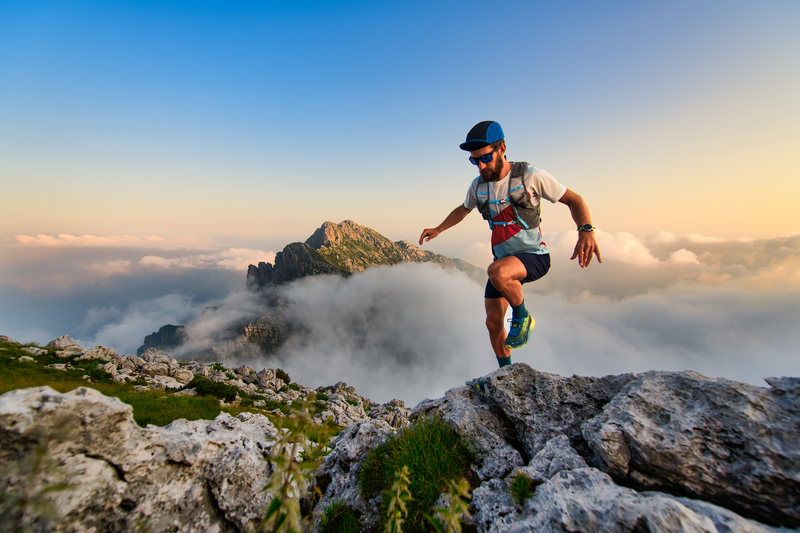
94% of researchers rate our articles as excellent or good
Learn more about the work of our research integrity team to safeguard the quality of each article we publish.
Find out more
ORIGINAL RESEARCH article
Front. Environ. Sci.
Sec. Big Data, AI, and the Environment
Volume 13 - 2025 | doi: 10.3389/fenvs.2025.1549209
This article is part of the Research Topic Dust and Polluted Aerosols: Sources, Transport and Radiative Effects Volume II View all articles
The final, formatted version of the article will be published soon.
You have multiple emails registered with Frontiers:
Please enter your email address:
If you already have an account, please login
You don't have a Frontiers account ? You can register here
With the rapid advancement of industrialization and the prevalent occurrence of haze weather, P M 2.5 contamination has emerged as a significant threat to public health and environmental sustainability. The concentration of P M 2.5 exhibits intricate dynamic attributes and is profoundly correlated with meteorological conditions as well as the concentrations of other pollutants, thereby substantially augmenting the complexity of predictive endeavors. Consequently, a novel predictive methodology has been developed, integrating time series frequency domain analysis with the decomposition of deep learning models. This approach facilitates the capture of interdependencies among high-dimensional features through time series decomposition, employs Fourier Transform to mitigate noise interference, and incorporates sparse attention mechanisms to selectively filter critical frequency components, thereby enhancing time-dependent modeling.Importantly, this technique effectively reduces computational complexity from O(L 2 ) to O(L log L).Empirical findings substantiate that this methodology yields notably superior predictive accuracy relative to conventional models across a diverse array of real-world datasets. This advancement not only offers an efficacious resolution for P M 2.5 prediction tasks but also paves the way for innovative research and application prospects in the realm of complex time series modeling.
Keywords: Frequency, time series forecasting, Air Pollution, transformer, Sparse attention mechanism
Received: 24 Dec 2024; Accepted: 13 Feb 2025.
Copyright: © 2025 Qin, Wei, Gao, Chen, Zhang and Un. This is an open-access article distributed under the terms of the Creative Commons Attribution License (CC BY). The use, distribution or reproduction in other forums is permitted, provided the original author(s) or licensor are credited and that the original publication in this journal is cited, in accordance with accepted academic practice. No use, distribution or reproduction is permitted which does not comply with these terms.
* Correspondence:
Baozhong Wei, Guangxi Police College, Nanning, Guangxi Zhuang Region, China
Caifeng Gao, Guangxi Police College, Nanning, Guangxi Zhuang Region, China
Xiaolong Chen, Faculty of Humanities and Social Sciences, Macao Polytechnic University, Macao, Macau Region, China
Hongfeng Zhang, Faculty of Humanities and Social Sciences, Macao Polytechnic University, Macao, Macau Region, China
Cora Un, Faculty of Humanities and Social Sciences, Macao Polytechnic University, Macao, Macau Region, China
Disclaimer: All claims expressed in this article are solely those of the authors and do not necessarily represent those of their affiliated organizations, or those of the publisher, the editors and the reviewers. Any product that may be evaluated in this article or claim that may be made by its manufacturer is not guaranteed or endorsed by the publisher.
Research integrity at Frontiers
Learn more about the work of our research integrity team to safeguard the quality of each article we publish.