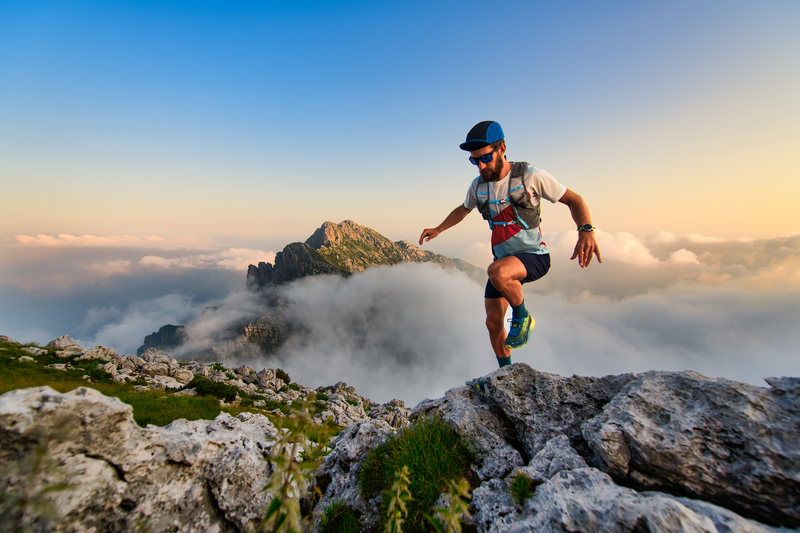
94% of researchers rate our articles as excellent or good
Learn more about the work of our research integrity team to safeguard the quality of each article we publish.
Find out more
ORIGINAL RESEARCH article
Front. Environ. Sci.
Sec. Environmental Informatics and Remote Sensing
Volume 13 - 2025 | doi: 10.3389/fenvs.2025.1526906
The final, formatted version of the article will be published soon.
You have multiple emails registered with Frontiers:
Please enter your email address:
If you already have an account, please login
You don't have a Frontiers account ? You can register here
Plant nitrogen (N) estimation using real-time and non-convection methods is very crucial for ecosystem management. This study aimed at estimating plant N concentration of indigenous vegetation in Luvuvhu River Catchment (LRC) using both field and remotely sensed data from Sentinel-2 imagery. The study used three different categories of spectral indices to fulfil its objectives. Red edge based, nitrogen related, and combined spectral indices based on Sentinel-2 data were subjected to stepwise regression on R studio software to determine which category of spectral indices is more efficient in estimating plant N concentration. Results have shown that combined spectral indices performed better with R 2 = 0.59, RMSE = 0.47% and MAE = 0.38%, followed by N based spectral indices with R 2 = 0.44, RMSE = 0.65% and MAE = 0.48%, and the last category is red edge based spectral indices with R 2 = 0.35, RMSE = 0.81% and MAE = 0.65%. The coefficients of the best performing model obtained from stepwise regression were used to compute multiple linear regression on QGIS to produce a map showing the concentration of plant N across the study area. Plant N varies with plant species and, the thematic map created show how plant N is distributed across the study area. With the help of this study, forest managers can better manage the natural vegetation by collaborating with forest communities. This possible partnership will create green jobs in addition to revenue. The link of the natural regeneration, reforestation, agroforestry and quantification by Sentinel-2 images for emission reduction will be beneficial for their livelihood. On a broader scale, participatory management is a good way to mitigate and adapt to climate change. On the other hand, the study suggests that more in-depth research should be conducted to explore further properties of red-edge indices for vegetation parameters prediction.
Keywords: Nitrogen, Sentinel-2, Spectral indices, broadband, narrowband
Received: 12 Nov 2024; Accepted: 21 Feb 2025.
Copyright: © 2025 Tshishonga and Malahlela. This is an open-access article distributed under the terms of the Creative Commons Attribution License (CC BY). The use, distribution or reproduction in other forums is permitted, provided the original author(s) or licensor are credited and that the original publication in this journal is cited, in accordance with accepted academic practice. No use, distribution or reproduction is permitted which does not comply with these terms.
* Correspondence:
Unarine Emmanuel Tshishonga, University of Venda, Thohoyandou, South Africa
Disclaimer: All claims expressed in this article are solely those of the authors and do not necessarily represent those of their affiliated organizations, or those of the publisher, the editors and the reviewers. Any product that may be evaluated in this article or claim that may be made by its manufacturer is not guaranteed or endorsed by the publisher.
Research integrity at Frontiers
Learn more about the work of our research integrity team to safeguard the quality of each article we publish.