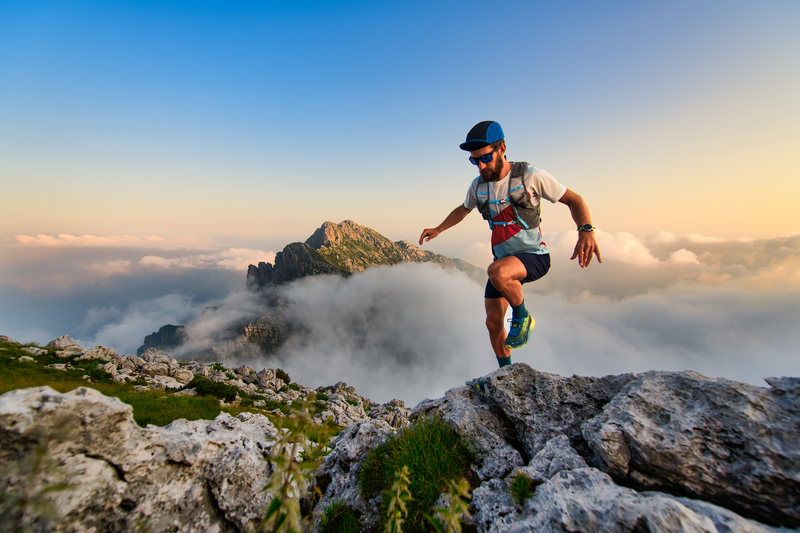
94% of researchers rate our articles as excellent or good
Learn more about the work of our research integrity team to safeguard the quality of each article we publish.
Find out more
ORIGINAL RESEARCH article
Front. Environ. Sci.
Sec. Big Data, AI, and the Environment
Volume 13 - 2025 | doi: 10.3389/fenvs.2025.1522933
This article is part of the Research Topic Integrating Deep Learning with Remote Sensing for Environmental Applications View all articles
The final, formatted version of the article will be published soon.
You have multiple emails registered with Frontiers:
Please enter your email address:
If you already have an account, please login
You don't have a Frontiers account ? You can register here
The acceleration of urbanization and the impact of climate change have led to an increasing frequency and intensity of grassland fires, posing severe challenges to resident safety and ecological protection. Traditional static evacuation route planning methods struggle to adapt in real-time to the dynamic changes in fire conditions during emergency management. To address this issue, this paper proposes a grassland fire evacuation route optimization strategy based on the GreyGNN-MARL model. By integrating Synthetic Aperture Radar (Sentinel-1 SAR) imagery, Graph Neural Networks (GNNs), Grey Wolf Optimization (GWO) algorithms, and Multi-Agent Reinforcement Learning (MARL), the model achieves intelligent planning and real-time adjustment of dynamic evacuation routes in fire scenarios. Experimental results demonstrate that this model significantly outperforms traditional methods in terms of evacuation time, risk avoidance success rate, and path safety, with evacuation time reduced by over 25% and risk avoidance success rate improved by approximately 18%. This model provides technical support for emergency management of grassland fires, helping to enhance evacuation efficiency and ensure safety, which is of great significance for smart cities and ecological protection. Future research will focus on further optimizing the model's computational efficiency and applicability for broader use in fire emergency management in complex environments.
Keywords: Fire Evacuation Route, SAR imagery, Pathfinding Optimization, deep learning, Multi-Hazard Ecosystem Resilience, Post-fire recovery, Climate-Adaptive Decision-making
Received: 13 Nov 2024; Accepted: 24 Feb 2025.
Copyright: © 2025 Zhang, Tan and Tiong. This is an open-access article distributed under the terms of the Creative Commons Attribution License (CC BY). The use, distribution or reproduction in other forums is permitted, provided the original author(s) or licensor are credited and that the original publication in this journal is cited, in accordance with accepted academic practice. No use, distribution or reproduction is permitted which does not comply with these terms.
* Correspondence:
Lingye Tan, Nanyang Technological University, Singapore, 639798, Singapore
Disclaimer: All claims expressed in this article are solely those of the authors and do not necessarily represent those of their affiliated organizations, or those of the publisher, the editors and the reviewers. Any product that may be evaluated in this article or claim that may be made by its manufacturer is not guaranteed or endorsed by the publisher.
Research integrity at Frontiers
Learn more about the work of our research integrity team to safeguard the quality of each article we publish.