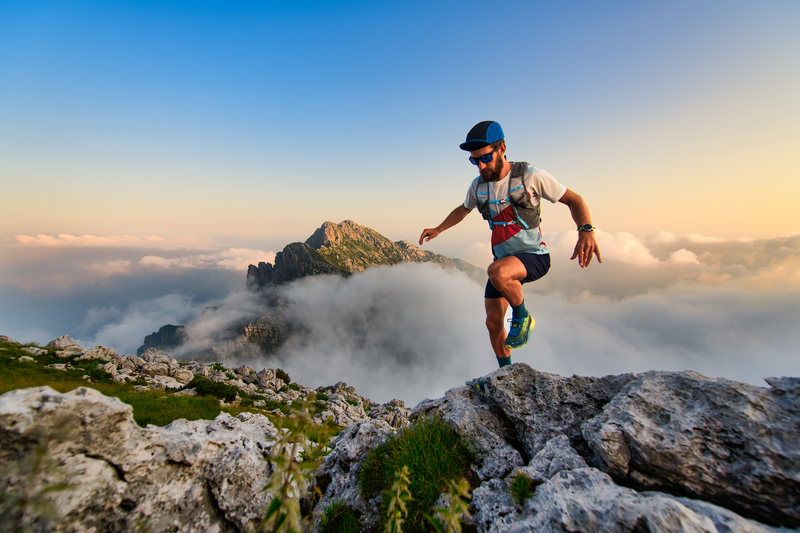
94% of researchers rate our articles as excellent or good
Learn more about the work of our research integrity team to safeguard the quality of each article we publish.
Find out more
ORIGINAL RESEARCH article
Front. Environ. Sci.
Sec. Environmental Policy and Governance
Volume 13 - 2025 | doi: 10.3389/fenvs.2025.1511882
This article is part of the Research Topic Climate Risk and Green and Low-Carbon Transformation: Economic Impact and Policy Response View all 15 articles
The final, formatted version of the article will be published soon.
You have multiple emails registered with Frontiers:
Please enter your email address:
If you already have an account, please login
You don't have a Frontiers account ? You can register here
This study aims to explore and validate the construction and effectiveness of a horizontal carbon ecological compensation mechanism in the Yellow River Basin (YRB). The aim is to enhance the ecological and environmental management capabilities within the YRB. Using data from 2013 to 2022, we calculated the net carbon emissions across the provinces in the YRB, distinguishing between carbon surplus and carbon deficit provinces. These calculations form the basis for constructing an evolutionary game model, which is used to analyse the dynamic evolutionary game under the introduction of incentive and punishment mechanisms, identifying key factors affecting the horizontal carbon ecological compensation mechanism in the YRB. This study advocates for the horizontal carbon ecological compensation mechanism in the YRB through a voluntary trading approach. By quantifying the influencing factors and employing machine learning techniques, we predict the net carbon emissions under the voluntary trading scenario, serving as evidence of the effectiveness of the ecological compensation mechanism. The results of our study demonstrate that with market-based incentives and proper guidance, the control and conservation of carbon emissions in the YRB can be significantly enhanced, providing a theoretical foundation for horizontal carbon ecological compensation within the basin. The innovation of this study lies in the development of a horizontal ecological compensation mechanism based on carbon emission trading and the application of machine learning methods to verify its effectiveness. This mechanism not only offers a new solution for the ecological protection of the YRB but also provides a reference model for the ecological governance of other river basins.
Keywords: Yellow River Basin, Carbon ecological compensation, evolutionary game theory, machine learning, policy impact
Received: 15 Oct 2024; Accepted: 25 Feb 2025.
Copyright: © 2025 Lu and Zhao. This is an open-access article distributed under the terms of the Creative Commons Attribution License (CC BY). The use, distribution or reproduction in other forums is permitted, provided the original author(s) or licensor are credited and that the original publication in this journal is cited, in accordance with accepted academic practice. No use, distribution or reproduction is permitted which does not comply with these terms.
* Correspondence:
Xiaofei Zhao, Lanzhou University of Finance and Economics, Lanzhou, China
Disclaimer: All claims expressed in this article are solely those of the authors and do not necessarily represent those of their affiliated organizations, or those of the publisher, the editors and the reviewers. Any product that may be evaluated in this article or claim that may be made by its manufacturer is not guaranteed or endorsed by the publisher.
Research integrity at Frontiers
Learn more about the work of our research integrity team to safeguard the quality of each article we publish.