- 1Shanghai Key Laboratory of Atmospheric Particle Pollution and Prevention, Department of Environmental Science and Engineering, Institute of Atmospheric Sciences, Fudan University, Shanghai, China
- 2Collaborative Innovation Centre of Atmospheric Environment and Equipment Technology (CICAEET), Nanjing University of Information Science and Technology, Nanjing, China
- 3School of Geographic Sciences, East China Normal University, Shanghai, China
- 4Beijing Capital Air Environmental Science & Technology CO., LTD., Beijing, China
- 5Institute of Eco–Chongming (SIEC), Shanghai, China
The changes in global air pollutant concentrations influenced by the COVID-19 lockdown have been widely investigated. The lack of clarity regarding the individual contributions to restricted human activities (i.e., transportation) has limited the understanding of the health impacts of the lockdown. In this study, an efficient chemical transport model (GEOS-Chem) was employed to simulate the concentration changes in air pollutants (PM2.5, NO2, and O3) associated with emission reductions in land transportation and the corresponding health benefits. The simulated results suggested that transportation-related PM2.5, NO2, and O3 reduced by 20%, 36%, and 55%, respectively. The reduction in O3 concentrations presented regional variations, with percentages ranked as follows: China (67%) > India (56%) > Europe (−81%) > the US (−86%), indicating the various intensities of secondary transformations with spatial relevance. The health benefits were also simulated, and the all-caused mortalities were estimated to be 63,547 (95% CI: 47,597, 79,497), 52,685 (95% CI: 32,310, 73,059), and 231,980 (95% CI: 210,373, 253,586) for the reduced concentration of PM2.5, NO2, and O3 globally, respectively. Transportation-related O3 reduction contributed the largest proportion (∼67%) to global health benefits, further emphasizing the global relevance and severity of O3 pollution. Our study confirms that the health benefits of transportation emission reduction during the COVID-19 lockdown were considerable and provides relevant simulated data as supporting evidence. We suggest that further coordinated efforts to restrict certain pollutants worldwide should focus on controlling the global O3 concentrations to protect people from severe O3 exposure.
1 Introduction
Land transportation is a major global source of air pollutants. Numerous studies have demonstrated that emissions from road and rail transport sectors contribute significantly to acid deposition, air pollution, and climate change (AlKheder, 2024; Colvile et al., 2001; Rodríguez-Sánchez et al., 2024). For example, Li and Managi estimated that a 6.17 billion-kilometer (km) increase in on-road transportation per square kilometer could lead to a 1-μg/m³ increase in county-level PM2.5 concentrations across the contiguous United States (Li and Managi, 2021). Mertens et al. quantified that land transport emissions contribute to 18% of ozone concentrations in North America (Mertens et al., 2018). Additionally, there is growing concern about the impact of land transportation on urban air quality and human health (Allaouat et al., 2024; Priyan et al., 2024; Rajagopal et al., 2024; Sang et al., 2022). Stevenson et al. estimated that private motor vehicles are responsible for 826 disability-adjusted life years (DALYs) per 100,000 population (Stevenson et al., 2016). Given these significant impacts, it is crucial to quantify the contribution of the land transportation sector to air quality and human health, which would enable local governments to develop targeted strategies to mitigate these public health risks (Di et al., 2017).
A growing body of research has focused on the contribution of land transportation to air pollution (Shen et al., 2024; Tong et al., 2020; Xu et al., 2024; Yan et al., 2022; Zara et al., 2024). Tong et al. assessed the impact of on-road vehicles on PM2.5 emissions and human health in Beijing, finding that median vehicle-related PM2.5 concentrations in the city exhibited significant weekly variations, with higher values (2.68 μg/m³) on weekdays and lower values (1.82 μg/m³) on weekends (Tong et al., 2020). Later, Yan et al. reported that the vehicle-related contribution to PM2.5 levels increased from 34% to 63% between 2013 and 2020 (Yan et al., 2022). However, most current studies have focused primarily on the regional scale, with few exploring the global contribution of land transportation to air pollution (Bhardwaj et al., 2023; Jiang et al., 2022; Kim et al., 2024; Le Hong and Zimmerman, 2021). Quantifying the impact of land transportation on air quality at a global level is crucial for identifying hotspots and proposing stringent control measures to mitigate environmental and health damage.
The onset of the COVID-19 pandemic at the end of 2019 significantly reshaped normal social and economic activities through strict lockdown measures, including stay-at-home orders and road closures (Ansari and Ramachandran, 2024; Liu et al., 2021). These temporary lockdowns led to a substantial reduction in anthropogenic emissions, particularly those from land transportation. On a global scale, Hoang et al. confirmed that NOX emissions showed a 20% decrease in early 2020 compared with the same period in 2019 (Hoang et al., 2021). Moreover, land transportation emissions experienced a 50%–80% decrease around the world, significantly higher than reductions observed in other sectors (Doumbia et al., 2021). Furthermore, human health was also greatly impacted by the concentration of pollutants, which was widely predicted and simulated (Chen and Hoek, 2020; Kyrychenko, 2024; Schraufnagel et al., 2019). However, the health benefits of COVID-19 lockdown-resulted air quality shifts were only investigated regionally (i.e., in Eastern Indo-Gangetic Plain and China (Jain et al., 2024; Ye et al., 2021)). The abrupt COVID-19 event provided an unprecedented chance to quantify the significant air quality and health benefits of land transportation emission reduction, which could provide a scientific basis for the proposal of future emission control measures (Berman and Ebisu, 2020; Li et al., 2021; Ma et al., 2024).
It should be noted that although the lockdown of COVID-19 has resulted in many consequences for the global economy, health benefits were benefitted from these restrictions. The reduction in pollutant emissions was particularly important when considering the long-term health benefits. Emission reductions from numerous sources reduced their contribution to global complex pollution, thus leading to fewer cases of death in relation to specific source emissions (Jain et al., 2024; Liu et al., 2021; Sacks et al., 2020). Therefore, the investigation of health benefits resulting from global emission reductions is necessary to better understand the health effects of pollutants, which should also be part of the long-term effects of COVID-19 (Ansari and Ramachandran, 2024; Li R. et al., 2023; Ling et al., 2023; Mueller et al., 2023; Tong et al., 2020; Zhang et al., 2021). In this study, a chemical transport model was used to quantify the concentrations of PM2.5, NO2, and O3 associated with land transportation emissions from February to April in 2019 and 2020. Subsequently, the differences in absolute concentrations and health impacts of these air pollutants between 2019 and 2020 were calculated. Lastly, the health benefits resulting from the reduction in land transportation emissions were assessed.
2 Materials and methods
2.1 Field measurements
All the field measurements for atmospheric PM2.5, NO2, and O3 focus on East Asia, India, Europe, and the United States. The hourly ambient PM2.5, NO2, and O3 observations across China during 2019–2020 were downloaded from the website http://beijingair.sinaapp.com/. The observation network in China possesses more than 2000 monitoring sites, and these sites are mixed with urban, suburban, and rural regions (Supplementary Figure S1). The ambient PM2.5, NO2, and O3 levels were measured using a continuous monitoring system, the chemiluminescence method (TEI Model 42i from Thermo Fisher Scientific Inc., USA), and the UV spectrophotometry method (TEI model 49i from Thermo Fisher Scientific Inc., USA). The monthly PM2.5, NO2, and O3 concentrations in other countries of East Asia and Southeast Asia from 2019 to 2020 were collected from the Acid Deposition Monitoring Network in East Asia (EANET). The daily PM2.5, NO2, and O3 datasets were collected from the Central Pollution Control Board (CPCB) database (https://app.cpcbccr.com/ccr/#/caaqm-dashboard-all/caaqm-landing). The ground-level PM2.5, NO2, and O3 datasets in more than 100 sites across Europe during 2019–2020 were downloaded from the European Monitoring and Evaluation Programme (EMEP) (www.emep.int). The daily ambient PM2.5, NO2, and O3 datasets in more than 200 sites during 2019–2020 across the United States were downloaded from the website https://www.epa.gov/.
2.2 GEOS-Chem simulation
GEOS-Chem (v13.4.0) was employed to estimate PM2.5, NO2, and O3 concentrations during February–April in 2019 and 2020. This model comprises a detailed simulation of tropospheric NOx–VOC–O3–aerosol chemistry mechanism (Mao et al., 2010; Park et al., 2004). Wet deposition includes the processes of sub-grid scavenging in convective updrafts, in-cloud rainout, and below-cloud washout (Liu et al., 2001). Dry deposition was calculated on the basis of a resistance-in-series model (Wesely, 2007). This model was driven by MERRA-2 assimilated meteorological factors (Li L. et al., 2023; Ou et al., 2022; Su et al., 2023). A global simulation was conducted at a spatial resolution of 2 × 2.5 (Ling et al., 2023; Qiu et al., 2020; Weagle et al., 2018). The anthropogenic emission inventory, including land transportation emissions in 2019 (0.5°), was collected from the Community Emissions Data System (CEDS, https://github.com/JGCRI/CEDS). Afterward, the daily emissions during February–April 2020 were calculated based on the value in 2019 and updated adjustment factor (for each source) proposed by Doumbia et al. (2021). Natural emissions include open biomass burning, lightning, and soil emissions. Open fire emissions derived from the Global Fire Emissions Database (GFED) in 2019 and 2020 were used for simulations (Chen et al., 2023). Lightning NOX emissions were estimated using the average of LIS/OTD satellite observations during 1995–2013 (Hudman et al., 2012; Murray et al., 2012). For the isolation of land transportation contribution, we calculated the total concentrations of air pollutants derived from all the sources and then subtracted the concentrations derived from all the sources excluding land transportation emissions. Finally, the concentrations derived from land transportation alone could be determined. The modeling performance of the contribution from individual sources cannot be validated, and thus, we only assessed the overall predictive accuracy of air pollutants from all the sources. In our study, some statistical indicators (supporting information) were applied to evaluate the predictive accuracy of the chemical transport model based on the ground-level observations.
2.3 Health effect assessment
In our study, the premature mortality associated with short-term PM2.5, NO2, and O3 exposures was estimated. The premature mortality linked with excessive air pollutant exposure was calculated based on the following formula, as previously recommended by Manojkumar and Srimuruganandam (2021) and Sacks et al. (2020).
where H denotes the premature all-cause mortality, owing to excessive PM2.5, NO2, and O3 exposures. x0 represents the baseline mortality.
3 Results and discussion
3.1 Model evaluation
The modeling performance of three pollutants—PM2.5, NO2, and O3—was evaluated using observed concentrations from field measurements (Section 2.1) and simulated concentrations from GEOS-Chem (Section 2.2). Ground-level observations of PM2.5, NO2, and O3 from over 2,000 cities worldwide were used to assess the predictive accuracy of the GEOS-Chem model. Notably, as there were insignificant differences between the correlations for February–April 2019 and 2020, the evaluation focused on each individual pollutant, with the results presented in Figure 1. The correlation coefficients (R values) between the observed and simulated concentrations for PM2.5, NO2, and O3 were 0.61, 0.65, and 0.72, respectively, for the period of February–April in 2019 and 2020. Furthermore, the root mean square error (RMSE) values were 3.89 μg m⁻³ for PM2.5, 6.68 μg m⁻³ for NO2, and 34.3 μg m⁻³ for O3, indicating good model performance. The mean absolute error (MAE) was calculated as 2.91 μg m⁻³ for PM2.5, 3.52 μg m⁻³ for NO2, and 28.2 μg m⁻³ for O3. In addition, the mean bias (MB), mean normalized bias (MNB), and mean normalized error (MNE) were determined to be −0.06 μg m⁻³, 0.05, and 0.42 for PM2.5; −2.98 μg m⁻³, −0.20, and 0.39 for NO2; and −23.6 μg m⁻³, −0.22, and 0.36 for O3. The MNB and MNE values were well within the thresholds recommended by the Epa (2007), which are ±60% for MNB and 75% for MNE. This suggests that the model results are robust, and the predicted concentrations of PM2.5, NO2, and O3 are reliable.
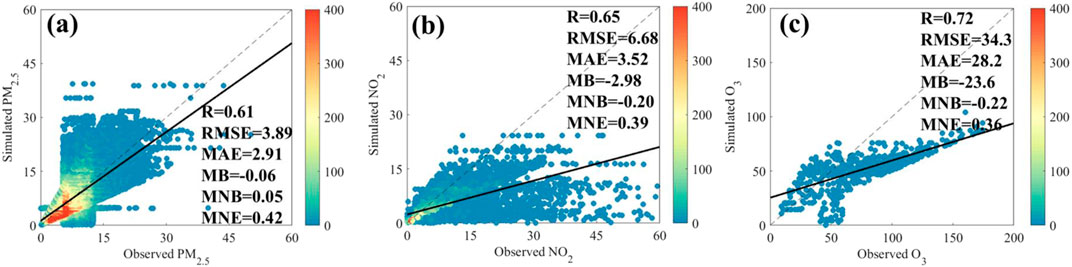
Figure 1. Modeling accuracy of estimated PM2.5 (A), NO2 (B), and O3 (C) levels during February–April in 2019 and 2020 globally (Unit: μg/m3).
Moreover, the model’s accuracy was comparable to previous studies. For instance, Balamurugan et al. reported an average R value of 0.55 for PM2.5 between in situ measurements and GEOS-Chem simulations in 10 German cities before the COVID-19 pandemic (January–May 2019) (Balamurugan et al., 2022). Similarly, Kong et al. found an average R value of 0.67 for NO2 in the North China Plain in 2010, while Lu et al. reported R values of 0.72 and 0.65 for NO2 in China in 2019 and 2020, respectively (Kong et al., 2020; Lu et al., 2024). Although the correlation for O3 was 0.53 from February to March 2019 over China, as simulated by Lu et al., this was likely due to the exclusion of significantly reduced NOX emission sites and the limited number of ground observation stations (Lu et al., 2024). In comparison, the R value for O3 in this study was higher, adding reliability to the model predictions. These results also surpass those of Sun et al. and Li et al., who reported R values of 0.65 (2019), 0.63 (2020), and 0.69 for O3, respectively (Li R. et al., 2023; Sun et al., 2024a). Overall, the model-predicted concentrations of air pollutants were both credible and satisfactory.
3.2 Impact of land transportation emissions on air pollutants around the world
The PM2.5, NO2, and O3 concentrations derived from land transportation emissions were estimated by subtracting the concentrations excluding land transportation emissions from the total concentrations. The results indicated that the transportation-related PM2.5 levels varied between 0.01 and 14.5 μg/m3 with a median of 0.46 μg/m3 during February–April 2019 (Supplementary Figure S2). The transportation-derived PM2.5 concentrations varied between 0.01 and 13.3 μg/m3 with a median of 0.28 μg/m3 during February–April 2020 (Figure 2). The transportation-related NO2 levels ranged from 0.02 to 9.66 μg/m3 with a median of 0.15 μg/m3 during February–April 2019 (Supplementary Figure S3). The transportation-related NO2 concentrations varied between 0.01 and 7.34 μg/m3 with a median of 0.09 μg/m3 during February–April 2020 (Figure 3). The O3 concentrations associated with land transportation ranged from 0.35 to 35.1 μg/m3 with a median of 7.68 μg/m3 during February–April 2019 (Supplementary Figure S4). The transportation-derived O3 levels varied between 0.26 and 30.9 μg/m3 with a median of 2.78 μg/m3 during February–April 2020 (Figure 4).
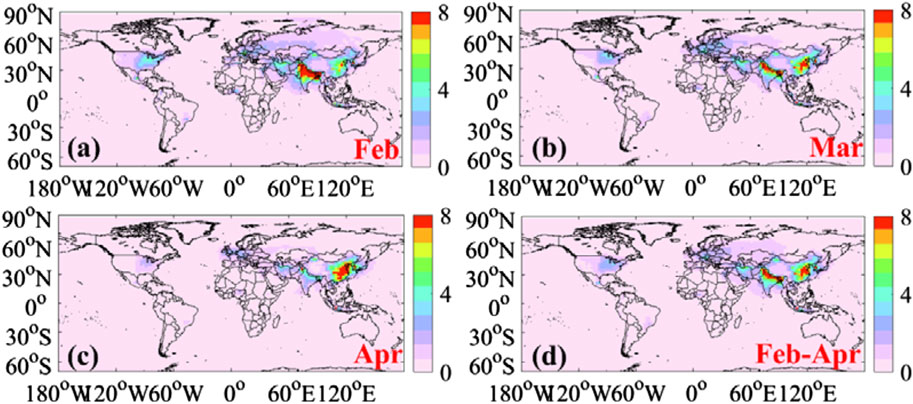
Figure 2. Spatial distributions of PM2.5 levels from land transportation emissions in February (A), March (B), and April (C) in 2020. (D) Mean concentrations of PM2.5 derived from land transportation emissions during February–April 2020.
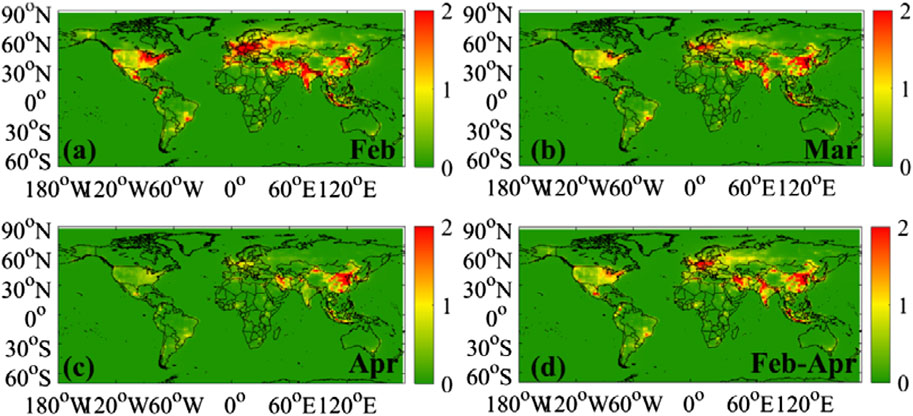
Figure 3. Spatial distributions of NO2 levels from land transportation emissions in February (A), March (B), and April (C) in 2020. (D) Mean concentrations of NO2 derived from land transportation emissions during February–April 2020.
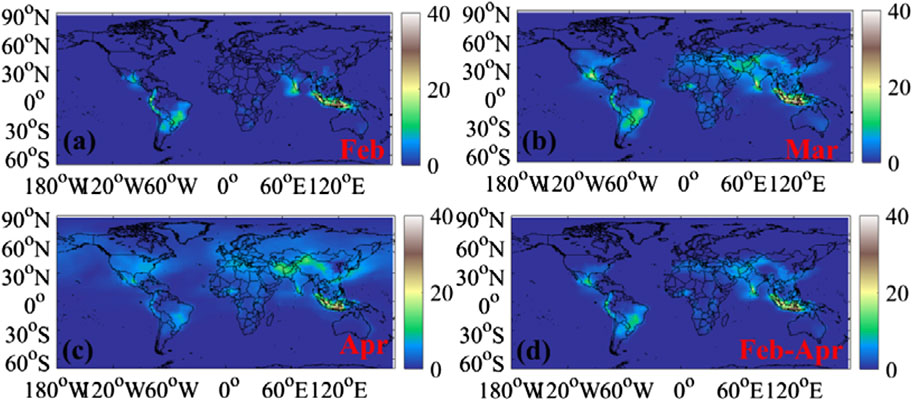
Figure 4. Spatial distributions of O3 levels from land transportation emissions in February (A), March (B), and April (C) in 2020. (D) Mean concentrations of O3 derived from land transportation emissions during February–April 2020.
The estimated transportation-derived PM2.5, NO2, and O3 levels exhibited significant spatial variations on a global scale. At the spatial scale, the transportation-related PM2.5 concentrations followed this order: India [4.19 ± 2.12 (2019) and 4.25 ± 2.66 (2020) μg/m3] > China (3.69 ± 1.68 and 2.89 ± 1.45 μg/m3) > Europe (3.54 ± 1.78 and 1.00 ± 0.48 μg/m3) > the US (1.17 ± 0.65 and 0.72 ± 0.42 μg/m3), which was in good agreement with the spatial distribution of total PM2.5 concentrations (Lim et al., 2020). The transportation-related NO2 levels in 2019 followed this order: Europe (1.47 ± 0.86 μg/m3) > China (1.15 ± 0.66 μg/m3) > India (1.06 ± 0.58 μg/m3) > the US (0.57 ± 0.35 μg/m3), while the transportation-derived NO2 levels in 2020 followed this order: China (0.85 ± 0.52 μg/m3) > India (0.83 ± 0.55 μg/m3) > Europe (0.63 ± 0.42 μg/m3) > the US (0.44 ± 0.28 μg/m3). The results suggested that Europe suffered from serious NO2 pollution derived from land transportation emissions during the business-as-usual period (Cooper et al., 2022; Sun et al., 2024b). This phenomenon is not surprising since the field measurements suggested that the NOX control is not as efficient as once thought, especially in Europe, where the transportation contribution to NOX concentrations is still dominant (Ntziachristos et al., 2016; Ramacher et al., 2020; Vestreng et al., 2009). Transportation-related O3 levels in 2019 displayed the highest concentrations in the US (12.4 ± 6.58 μg/m3), followed by India (11.1 ± 5.84 μg/m3) and Europe (11.0 ± 6.42 μg/m3), and the lowest concentration observed in China (10.1 ± 4.96 μg/m3). However, the transportation-derived O3 levels in 2020 showed the highest values in India (4.84 ± 2.65 μg/m3), followed by China (3.34 ± 2.12 μg/m3) and Europe (2.09 ± 1.12 μg/m3), and the lowest value in the US (1.74 ± 0.96 μg/m3). The marked decrease in transportation-derived O3 levels in the US compared with other countries during the COVID-19 lockdown might be contributed to more rapid decreases in NOX and VOC emissions than in other regions (Shakoor et al., 2020; Sicard et al., 2020). As recommended by Mertens et al., the transportation contribution toward ozone net production has reached 21% in North America, higher than 13% globally (Mertens et al., 2018). Such research studies emphasized the importance of precursors on the secondary formation of ozone globally.
The transportation-related PM2.5, NO2, and O3 concentrations not only displayed remarkable spatial differences but also suffered from marked variations during the COVID-19 period. The mean concentrations of transportation-derived PM2.5, NO2, and O3 decreased by 20%, 36%, and 55%, respectively. Furthermore, the decreasing ratios of air pollutants in different regions often suffered from significant spatial discrepancies. In China, PM2.5, NO2, and O3 concentrations reduced by 21%, 26%, and 67%, respectively. In India, PM2.5, NO2, and O3 levels decreased by 1%, 21%, and 56%, respectively. In the United States and Europe, the transportation-related O3 levels [−81% (Europe) and −86% (the US)] experienced more rapid decreases compared with PM2.5 [−72% (Europe) and −38% (the US)] and NO2 [−57% (Europe) and −23% (the US)]. More significant decreases in transportation-related air pollutant concentrations in the United States and Europe after the COVID-19 outbreak might be associated with dense road networks and land transportation emissions during the non-lockdown period (Gaubert et al., 2021; Keller et al., 2021; Miyazaki et al., 2021), as shown in Figure 5. In addition, it should be noted that the decrease in transportation-related O3 was significantly higher than the reductions in PM2.5 and NO2, which was in contrast with the trends observed for shipping-related air pollutants (Sun et al., 2024a). In general, the transportation-related NOX emission reduction was much greater than that of VOCs due to different source apportionments (Lidén et al., 1999; Liu et al., 2016; Shao et al., 2016; Xu et al., 2018; Zhang et al., 2020; Zhao et al., 2019), and thus, the O3 might increase, especially in VOC-limited regions (Grange et al., 2021; Wang et al., 2023). However, the transportation-related O3 concentrations displayed decreases in both VOC- and NOX-limited areas during the COVID-19 period. It was assumed that the deep emission reduction in VOC and NOX could facilitate the decreases in O3 concentrations (Liu and Shi, 2021; Sillman, 1999; Xiang et al., 2020).
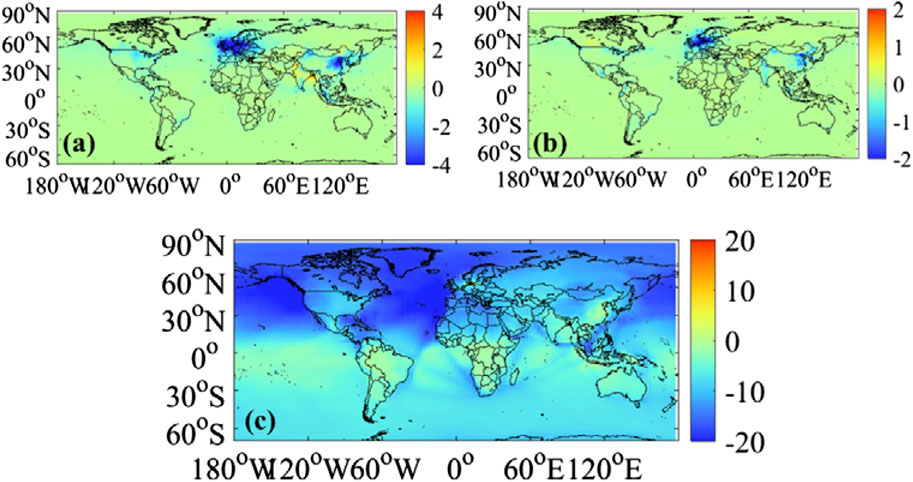
Figure 5. Spatial distributions of PM2.5 (A), NO2 (B), and O3 (C) concentrations before and during the COVID-19 period derived from land transportation emissions on a global scale.
3.3 Health benefits of transportation-related PM2.5, NO2, and O3 exposures
Based on Equations 1, 2 from Section 2.3, the all-cause mortalities attributable to PM2.5, NO2, and O3 levels induced by transportation emissions were estimated. These methods, previously applied for assessing shipping emissions (Contini and Merico, 2021; Tian et al., 2013; Zhang et al., 2021), offer insights into the health impacts of air pollution. In total, transportation-related PM2.5 exposure resulted in 243,431 (95% CI: 196,813, 290,048) and 179,884 (95% CI: 149,216, 210,551) deaths globally in 2019 and 2020, respectively. Among the most affected regions, India showed the highest mortality rates, with 55,513 (95% CI: 52,846, 58,179) and 53,191 (95% CI: 51,301, 55,080) cases in early 2019 and 2020, respectively. China followed closely, recording 58,816 (95% CI: 57,633, 59,998) cases in 2019 and 49,709 (95% CI: 48,033, 51,385) in 2020. The slight decline in India’s numbers between 2019 and 2020 is attributed to the late imposition of COVID-19 lockdown measures (starting late-March 2020) (Sharma et al., 2020). Meanwhile, China’s decrease in both PM2.5 levels and related mortalities reflects the earlier implementation of lockdown measures, leading to improved air quality (Chen et al., 2020; He et al., 2020). Europe recorded similar PM2.5-related mortalities in early 2019, with 51,993 (95% CI: 35,101, 68,884) deaths, compared to a significant decrease in 2020 with 18,635 (95% CI: 11,631, 25,638) cases. The United States experienced the lowest numbers, with 17,481 (95% CI: 10,555, 24,408) in 2019 and 12,134 (95% CI: 7,233, 17,034) in 2020. The health benefits from the reduction in transportation-related PM2.5 emissions were estimated based on the decreased number of cases, as shown in Table 1. The reduction in mortalities amounted to 9,107 (95% CI: 8,613, 9,601) in China, 5,348 (95% CI: 3,322, 7,374) in the United States, 33,358 (95% CI: 23,471, 43,246) in Europe, 2,322 (95% CI: 1,545, 3,098) in India, and 63,547 (95% CI: 47,597, 79,497) globally.
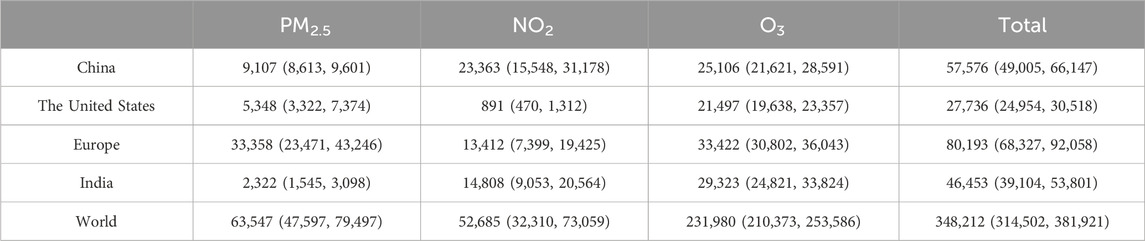
Table 1. Health benefits (95% CI: lower, upper) associated with PM2.5, NO2, and O3 induced by land transportation emission reduction during the COVID-19 period.
The all-cause mortalities and health benefits associated with transportation-related NO2 emissions were also calculated. Globally, transportation-related NO2 exposure resulted in 154,195 (95% CI: 90,311, 218,079) and 101,510 (95% CI: 58,000, 145,020) cases in early 2019 and 2020, respectively. In China, the estimated mortalities were 84,759 (95% CI: 51,886, 117,631) in 2019 and 49,709 (95% CI: 48,033, 51,385) in 2020. Similarly, in India, NO2-related all-cause mortalities were 54,967 (95% CI: 30,795, 79,140) in 2019 and 40,159 (95% CI: 21,742, 58,576) in 2020 during the February–April period. In Europe, the number of cases attributed to NO2 exposure from transportation emissions was 16,040 (95% CI: 8,787, 23,293) in 2019, decreasing to 2,628 (95% CI: 1,388, 3,868) in 2020. The United States exhibited the lowest health benefits, with 1,501 (95% CI: 796, 2,207) cases in 2019 and 610 (95% CI: 326, 895) in 2020. Globally, the reduction in NO2-related mortalities due to decreased transportation emissions was estimated at 52,685 (95% CI: 32,310, 73,059). Regionally, the health benefits were estimated as follows: China, 23,363 (95% CI: 15,548, 31,178); the United States, 891 (95% CI: 470, 1,312); Europe, 13,412 (95% CI: 7,399, 19,425); and India, 14,808 (95% CI: 9,053, 20,564).
The ambient O3 concentrations affected by the COVID-19 lockdown were also simulated, and the resulting health benefits from transportation emissions were estimated to be 25,106 (95% CI: 21,621, 28,591) in China, 21,497 (95% CI: 19,638, 23,357) in the United States, 33,422 (95% CI: 30,802, 36,043) in Europe, and 29,323 (95% CI: 24,821, 33,824) in India. During the lockdown period, our simulations indicated a slight increase in O3 concentration globally, consistent with previous research (Bi et al., 2022; Deroubaix et al., 2021; Keller et al., 2021). Globally, the total O3-related health benefits were estimated at 231,980 (95% CI: 210,373, 253,586), making it the most significant of the three pollutants examined. Summarizing the health benefits of all three pollutants, the transportation-related benefits were 57,576 (95% CI: 49,005, 66,147) in China, 27,736 (95% CI: 24,954, 30,518) in the United States, 80,193 (95% CI: 68,327, 92,058) in Europe, and 46,453 (95% CI: 39,104, 53,801) in India. Notably, while Europe represents approximately 9.5% of the global population, it accounted for over 24.1% of the health benefits, particularly with 52.5% of the PM2.5-related benefits and 25.5% of the NO2-related benefits. This highlights the substantial health benefits of reduced transportation emissions and emphasizes the severe situation of transportation emissions in Europe (Matthias et al., 2021; Ntziachristos et al., 2016; Rodríguez-Sánchez et al., 2024). Similarly, the United States, representing 4.2% of the global population, contributed 8.0% of the total health benefits.
It is important to acknowledge that the relative risk (RR) values used to estimate health impacts can vary significantly across different regions (Chen and Sun, 2021). As a result, this introduces uncontrolled uncertainties into the simulation process. Future simulations should focus on determining region-specific RR values, particularly in countries with smaller populations, to improve the accuracy of predictions.
4 Conclusions and implications
In this study, the GEOS-Chem model was employed to assess the health impacts associated with the reduction in transportation emissions by removing the corresponding contributions during February–April of both 2019 and 2020, enabling the quantification of the additional effects of the COVID-19 lockdown. Initially, transportation-related emissions were included in the pollutant simulations but were subsequently excluded for a separate simulation. The difference between these simulations was considered the health benefit derived from the reduction in transportation emissions. Therefore, the change in transportation emissions between 2019 and 2020 accounted for the health benefit differences observed between these 2 years. The simulation of selected pollutants in this study demonstrated strong agreement with corresponding observations (R = 0.61 for PM2.5, 0.65 for NO2, and 0.72 for O3).
According to the simulation, significant spatial variations were observed in transportation-related PM2.5, NO2, and O3 levels. The estimated PM2.5 concentrations followed this order: India > China > Europe > the United States in both 2019 and 2020, a spatial distribution consistent with the findings of Lim et al. (2020). The predicted NO2 concentrations presented a different pattern between 2019 and 2020. When comparing the influence of excluding transportation emissions, the results showed that the world (36%) > China (26%) > India (21%) > the United States (−23%) > Europe (−57%). This suggests that the COVID-19 lockdown caused a significant decrease in NO2 levels in China and India, while globally, NO2 concentrations were suppressed except in Europe and the United States. The lockdowns, which began in early March 2020 in Europe and mid-March in the United States—coinciding with the same period in India—led to varying impacts on NO2 levels (Berman and Ebisu, 2020; Matthias et al., 2021; Nigam et al., 2021; Sharma et al., 2020). The industrial emissions in China and India contributed to higher NO2 levels than those in Europe and the United States, where transportation emissions dominated. As a result, the decrease in NO2 concentrations in China and India was less pronounced compared to the steep declines in Europe and the United States, where transportation was the primary source of NO2 emissions. Regarding O3, the reduction in transportation-related emissions caused a larger decrease in O3 levels compared to PM2.5 and NO2, which contrasts with patterns observed for shipping emissions (Sun et al., 2024a). O3 levels are generally controlled by photochemical reactions, as explained by the Empirical Kinetic Modeling Approach (EKMA) (Martinez et al., 1983), which suggests that reducing NOX and VOCs emissions may improve O3 concentrations. This was further supported by the observed higher O3 concentrations during the lockdown period compared to pre-lockdown levels (Figure 4). Overall, effective O3 pollution control requires a comprehensive approach, addressing both NOX and VOC emissions alongside the local and long-range transport of these pollutants.
The health benefits of reducing PM2.5, NO2, and O3 emissions due to transportation-related activities were evaluated across key global regions. The all-cause mortalities associated with these pollutants were simulated to be 65,347 (95% CI: 47,597, 79,497) for PM2.5, 52,685 (95% CI: 32,310, 73,059) for NO2, and 231,980 (95% CI: 210,373, 253,586) for O3. Among the regions studied, Europe saw the greatest health benefits, with estimated reductions in mortalities at 80,193 (95% CI: 68,327, 92,058), followed by China [57,576 (95% CI: 49,005, 66,147)], India [46,453 (95% CI: 39,104, 53,801)], and the United States [27,736 (95% CI: 24,954, 30,518)]. Although previous studies have investigated this by regional or source differences (Cesaroni et al., 2012; Host et al., 2020; Liu et al., 2021; Pappin et al., 2016; Zhang et al., 2021), the transportation emission reduction-related health benefits were derived globally in this study, providing a valuable perspective on the long-term effect of the COVID-19 lockdown.
The findings from this research also hold significant global implications for policy-making. First, the positive health impacts observed from the reduction of transportation emissions demonstrate that limiting vehicle usage can substantially protect populations from pollutant exposure. This underscores the importance of implementing stricter emission standards for fuel-powered vehicles and encouraging the adoption of cleaner, alternative energy vehicles worldwide. As transportation is one of the major sources of pollution globally, future efforts must focus on imposing greater restrictions on emissions in this sector. Moreover, even during the global lockdown in April 2020, when PM2.5 and NO2 concentrations were at their lowest, O3 levels peaked globally—except in South America, where high cloud cover and frequent rainfall likely contributed to lower ozone concentrations (Cazorla et al., 2021; Gaubert et al., 2021). Of particular concern is the fact that transportation-related ozone exposure accounted for most health benefits across the three selected pollutants, emphasizing the critical role of transportation-emitted precursors (such as VOCs and NOX) in ozone formation. These precursors should be strictly regulated in future policies.
It is important to acknowledge the limitations to this study. Transportation emissions globally can influence several other factors, such as aerosol optical depth, surface temperature, and the local meteorological transformations that occur in response to the absence of these emissions. Additionally, the health impacts associated with reduced transportation emissions may extend beyond immediate respiratory conditions, potentially affecting crop growth, local photosynthesis, and even the long-term effects of COVID-19 infections. To better estimate health benefits and minimize uncertainties, future studies should incorporate more accurate observations and detailed variable data in modeling efforts. Furthermore, identifying effective strategies for managing secondary pollutants like O3 is crucial for safeguarding human health worldwide. Furthermore, the health benefits from other specific sources remain uninvestigated (i.e., industrial emissions). As the most important factor in emission reduction, the COVID-19 lockdown plays a significant role in global pollution levels and climate change (Abdullah et al., 2024; Liu et al., 2024; Tautan et al., 2024). More research studies are recommended on the concentration reduction of pollutants to gain a better understanding of regional secondary transformation and global pollution formation.
Data availability statement
The raw data supporting the conclusions of this article will be made available by the authors, without undue reservation.
Author contributions
YZ: conceptualization, data curation, formal analysis, investigation, methodology, validation, writing–original draft, and writing–review and editing. YC: conceptualization, formal analysis, methodology, validation, and writing–original draft. FZ: conceptualization, formal analysis, validation, and writing–original draft. HF: conceptualization, funding acquisition, resources, supervision, writing–original draft, and writing–review and editing.
Funding
The author(s) declare that financial support was received for the research, authorship, and/or publication of this article. This work was supported by the National Key R&D Program of China (grant no. 2022YFC3701102), the National Natural Science Foundation of China (grant nos 22466020, 22376029, 22176038, 91744205, and 21777025) and the Natural Science Foundation of Shanghai City (grant no. 22ZR1404700).
Conflict of interest
Author FZ was employed by Beijing Capital Air Environmental Science & Technology Co., Ltd.
The remaining authors declare that the research was conducted in the absence of any commercial or financial relationships that could be construed as a potential conflict of interest.
Generative AI statement
The author(s) declare that no Generative AI was used in the creation of this manuscript.
Publisher’s note
All claims expressed in this article are solely those of the authors and do not necessarily represent those of their affiliated organizations, or those of the publisher, the editors, and the reviewers. Any product that may be evaluated in this article, or claim that may be made by its manufacturer, is not guaranteed or endorsed by the publisher.
Supplementary material
The Supplementary Material for this article can be found online at: https://www.frontiersin.org/articles/10.3389/fenvs.2024.1519984/full#supplementary-material
References
Abdullah, M. A., Chuah, L. F., Abdullah, S. B., Bokhari, A., Syed, A., Elgorban, A. M., et al. (2024). From port to planet: assessing NO2 pollution and climate change effects with Sentinel-5p satellite imagery in maritime zones. Environ. Res. 257, 119328. doi:10.1016/j.envres.2024.119328
AlKheder, S. (2024). Alternate green and carsharing mobility options: a strategy to fight climate change in oil producing countries. Phys. Chem. Earth, Parts A/B/C 136, 103694. doi:10.1016/j.pce.2024.103694
Allaouat, S., Yli-Tuomi, T., Tiittanen, P., Kukkonen, J., Kangas, L., Mikkonen, S., et al. (2024). Long-term exposures to low concentrations of source-specific air pollution, road-traffic noise, and systemic inflammation and cardiovascular disease biomarkers. Environ. Res. 262, 119846. doi:10.1016/j.envres.2024.119846
Ansari, K., and Ramachandran, S. (2024). Global changes in aerosol single scattering albedo during COVID-19. Atmos. Environ. 333, 120649. doi:10.1016/j.atmosenv.2024.120649
Balamurugan, V., Chen, J., Qu, Z., Bi, X., and Keutsch, F. N. (2022). Secondary PM2.5 decreases significantly less than NO2 emission reductions during COVID lockdown in Germany. Atmos. Chem. Phys. 22, 7105–7129. doi:10.5194/acp-22-7105-2022
Berman, J. D., and Ebisu, K. (2020). Changes in U.S. air pollution during the COVID-19 pandemic. Sci. Total Environ. 739, 139864. doi:10.1016/j.scitotenv.2020.139864
Bhardwaj, C., Axsen, J., and Crawford, C. (2023). Simulating long-term emissions from private automated vehicles under climate policies. Transp. Res. Part D Transp. Environ. 118, 103665. doi:10.1016/j.trd.2023.103665
Bi, Z., Ye, Z., He, C., and Li, Y. (2022). Analysis of the meteorological factors affecting the short-term increase in O3 concentrations in nine global cities during COVID-19. Atmos. Pollut. Res. 13, 101523. doi:10.1016/j.apr.2022.101523
Cazorla, M., Herrera, E., Palomeque, E., and Saud, N. (2021). What the COVID-19 lockdown revealed about photochemistry and ozone production in Quito, Ecuador. Atmos. Pollut. Res. 12, 124–133. doi:10.1016/j.apr.2020.08.028
Cesaroni, G., Boogaard, H., Jonkers, S., Porta, D., Badaloni, C., Cattani, G., et al. (2012). Health benefits of traffic-related air pollution reduction in different socioeconomic groups: the effect of low-emission zoning in Rome. Occup. Environ. Med. 69, 133–139. doi:10.1136/oem.2010.063750
Chen, H., and Sun, J. J. E. s.F. (2021). Significant increase of the global population exposure to increased precipitation extremes in the future, 9.e2020EF001941
Chen, J., and Hoek, G. (2020). Long-term exposure to PM and all-cause and cause-specific mortality: a systematic review and meta-analysis. Environ. Int. 143, 105974. doi:10.1016/j.envint.2020.105974
Chen, R., Yin, P., Meng, X., Wang, L., Liu, C., Niu, Y., et al. (2018). Associations between ambient nitrogen dioxide and daily cause-specific mortality: evidence from 272 Chinese cities. Epidemiology 29, 482–489. doi:10.1097/ede.0000000000000829
Chen, S., Yang, J., Yang, W., Wang, C., and Bärnighausen, T. (2020). COVID-19 control in China during mass population movements at New Year. lancet 395, 764–766. doi:10.1016/s0140-6736(20)30421-9
Chen, W., Lu, X., Yuan, D., Chen, Y., Li, Z., Huang, Y., et al. (2023). Global PM2. 5 prediction and associated mortality to 2100 under different climate change scenarios. Environ. Sci. and Technol. 57, 10039–10052. doi:10.1021/acs.est.3c03804
Colvile, R., Hutchinson, E. J., Mindell, J., and Warren, R. (2001). The transport sector as a source of air pollution. Atmos. Environ. 35, 1537–1565. doi:10.1016/s1352-2310(00)00551-3
Contini, D., and Merico, E. (2021). Recent advances in studying air quality and health effects of shipping emissions, 12, 92.
Cooper, M. J., Martin, R. V., Hammer, M. S., Levelt, P. F., Veefkind, P., Lamsal, L. N., et al. (2022). Global fine-scale changes in ambient NO2 during COVID-19 lockdowns. Nature 601, 380–387. doi:10.1038/s41586-021-04229-0
Deroubaix, A., Brasseur, G., Gaubert, B., Labuhn, I., Menut, L., Siour, G., et al. (2021). Response of surface ozone concentration to emission reduction and meteorology during the COVID-19 lockdown in Europe, 28.e1990
Di, Q., Wang, Y., Zanobetti, A., Wang, Y., Koutrakis, P., Choirat, C., et al. (2017). Air pollution and mortality in the medicare population. N. Engl. J. Med. 376, 2513–2522. doi:10.1056/nejmoa1702747
Doumbia, T., Granier, C., Elguindi, N., Bouarar, I., Darras, S., Brasseur, G., et al. (2021). Changes in global air pollutant emissions during the COVID-19 pandemic: a dataset for atmospheric modeling. Earth Syst. Sci. Data 13, 4191–4206. doi:10.5194/essd-13-4191-2021
Epa, U. (2007). Guidance on the use of models and other analyses for demonstrating attainment of air quality goals for ozone, PM2. 5, and regional haze. US Environmental Protection Agency, Office of Air Quality Planning and Standards.
Gaubert, B., Bouarar, I., Doumbia, T., Liu, Y., Stavrakou, T., Deroubaix, A., et al. (2021). Global changes in secondary atmospheric pollutants during the 2020 COVID-19 pandemic. J. Geophys. Res. Atmos. 126, e2020JD034213. doi:10.1029/2020jd034213
Grange, S. K., Lee, J. D., Drysdale, W. S., Lewis, A. C., Hueglin, C., Emmenegger, L., et al. (2021). COVID-19 lockdowns highlight a risk of increasing ozone pollution in European urban areas. Atmos. Chem. Phys. 21, 4169–4185. doi:10.5194/acp-21-4169-2021
Hang, Y., Meng, X., Li, T., Wang, T., Cao, J., Fu, Q., et al. (2022). Assessment of long-term particulate nitrate air pollution and its health risk in China. Iscience 25, 104899. doi:10.1016/j.isci.2022.104899
He, G., Pan, Y., and Tanaka, T. (2020). The short-term impacts of COVID-19 lockdown on urban air pollution in China. Nat. Sustain. 3, 1005–1011. doi:10.1038/s41893-020-0581-y
Hoang, V.-T., Colson, P., Levasseur, A., Delerce, J., Lagier, J.-C., Parola, P., et al. (2021). Clinical outcomes in patients infected with different SARS-CoV-2 variants at one hospital during three phases of the COVID-19 epidemic in Marseille, France. Infect. Genet. Evol. 95, 105092. doi:10.1016/j.meegid.2021.105092
Host, S., Honoré, C., Joly, F., Saunal, A., Le Tertre, A., and Medina, S. (2020). Implementation of various hypothetical low emission zone scenarios in Greater Paris: Assessment of fine-scale reduction in exposure and expected health benefits. Environ. Res. 185, 109405. doi:10.1016/j.envres.2020.109405
Hudman, R., Moore, N., Mebust, A., Martin, R., Russell, A., Valin, L., et al. (2012). Steps towards a mechanistic model of global soil nitric oxide emissions: implementation and space based-constraints. Atmos. Chem. Phys. 12, 7779–7795. doi:10.5194/acp-12-7779-2012
Jain, R. R., Sahni, B. K., Mishra, I., and Izhar, S. (2024). COVID-19 lockdown impact on air quality and associated health benefit in two contrasting urban cities in Eastern Indo Gangetic Plain. Atmos. Environ. X 23, 100290. doi:10.1016/j.aeaoa.2024.100290
Jiang, Z., Yu, D., Luan, S., Zhou, H., and Meng, F. (2022). Integrating traffic signal optimization with vehicle microscopic control to reduce energy consumption in a connected and automated vehicles environment. J. Clean. Prod. 371, 133694. doi:10.1016/j.jclepro.2022.133694
Keller, C. A., Evans, M. J., Knowland, K. E., Hasenkopf, C. A., Modekurty, S., Lucchesi, R. A., et al. (2021). Global impact of COVID-19 restrictions on the surface concentrations of nitrogen dioxide and ozone. Atmos. Chem. Phys. 21, 3555–3592. doi:10.5194/acp-21-3555-2021
Kim, C., Butt, A. A., Harvey, J. T., and Ostovar, M. (2024). Environmental impacts from traffic on highway construction work zones: framework and simulations. Int. J. Sustain. Transp. 18, 680–694. doi:10.1080/15568318.2024.2392624
Kong, L., Tang, X., Zhu, J., Wang, Z., Fu, J. S., Wang, X., et al. (2020). Evaluation and uncertainty investigation of the NO2, CO and NH3 modeling over China under the framework of MICS-Asia III. Atmos. Chem. Phys. 20, 181–202. doi:10.5194/acp-20-181-2020
Kyrychenko, O. (2024). Health benefits of air pollution reduction: evidence from economic slowdown in India. Econ. Hum. Biol. 55, 101437. doi:10.1016/j.ehb.2024.101437
Le Hong, Z., and Zimmerman, N. (2021). Air quality and greenhouse gas implications of autonomous vehicles in Vancouver, Canada. Transp. Res. Part D Transp. Environ. 90, 102676. doi:10.1016/j.trd.2020.102676
Li, C., and Managi, S. (2021). Contribution of on-road transportation to PM2. 5. Sci. Rep. 11, 21320. doi:10.1038/s41598-021-00862-x
Li, C., Shang, H., Cui, Z., Dai, Z., and Ma, Z. (2021). COVID-19 as a factor influencing air quality? A city study in China. Aerosol Air Qual. Res. 21, 210080. doi:10.4209/aaqr.210080
Li, L., Che, H., Su, X., Zhang, X., Gui, K., Zheng, Y., et al. (2023a). Quantitative evaluation of dust and black carbon column concentration in the MERRA-2 reanalysis dataset using satellite-based component retrievals. Remote Sens. 15, 388. doi:10.3390/rs15020388
Li, R., Zhang, L., Gao, Y., and Wang, G. (2023b). Different response mechanisms of N-bearing components to emission reduction across China during COVID-19 lockdown period. J. Geophys. Res. Atmos. 128. doi:10.1029/2023jd039496
Lidén, R., Vasilyev, A., Stålnacke, P., Loigu, E., and Wittgren, H. B. (1999). Nitrogen source apportionment—a comparison between a dynamic and a statistical model. Ecol. Model. 114, 235–250. doi:10.1016/s0304-3800(98)00146-x
Lim, C.-H., Ryu, J., Choi, Y., Jeon, S. W., and Lee, W.-K. (2020). Understanding global PM2. 5 concentrations and their drivers in recent decades (1998–2016). Environ. Int. 144, 106011. doi:10.1016/j.envint.2020.106011
Ling, C., Cui, L., and Li, R. (2023). Global impact of the COVID-19 lockdown on surface concentration and health risk of atmospheric benzene. Atmos. Chem. Phys. 23, 3311–3324. doi:10.5194/acp-23-3311-2023
Liu, B., Liang, D., Yang, J., Dai, Q., Bi, X., Feng, Y., et al. (2016). Characterization and source apportionment of volatile organic compounds based on 1-year of observational data in Tianjin, China. Environ. Pollut. 218, 757–769. doi:10.1016/j.envpol.2016.07.072
Liu, C., and Shi, K. (2021). A review on methodology in O3-NOx-VOC sensitivity study. Environ. Pollut. 291, 118249. doi:10.1016/j.envpol.2021.118249
Liu, F., Wang, M., and Zheng, M. (2021). Effects of COVID-19 lockdown on global air quality and health. Sci. Total Environ. 755, 142533. doi:10.1016/j.scitotenv.2020.142533
Liu, H., Jacob, D. J., Bey, I., and Yantosca, R. M. (2001). Constraints from 210Pb and 7Be on wet deposition and transport in a global three-dimensional chemical tracer model driven by assimilated meteorological fields. J. Geophys. Res. Atmos. 106, 12109–12128. doi:10.1029/2000jd900839
Liu, X., Zhang, L., Du, Y., Yang, X., He, X., Zhang, J., et al. (2024). Spatiotemporal variations and the ecological risks of microplastics in the watersheds of China: implying the impacts of the COVID-19 pandemic. Sci. Total Environ. 952, 175988. doi:10.1016/j.scitotenv.2024.175988
Lu, Z., Wang, J., Wang, Y., Henze, D. K., Chen, X., Sha, T., et al. (2024). Aggravated surface O3 pollution primarily driven by meteorological variations in China during the 2020 COVID-19 pandemic lockdown period. Atmos. Chem. Phys. 24, 7793–7813. doi:10.5194/acp-24-7793-2024
Ma, Y., Nobile, F., Marb, A., Dubrow, R., Kinney, P. L., Peters, A., et al. (2024). Air pollution changes due to COVID-19 lockdowns and attributable mortality changes in four countries. Environ. Int. 187, 108668. doi:10.1016/j.envint.2024.108668
Manojkumar, N., and Srimuruganandam, B. (2021). Health benefits of achieving fine particulate matter standards in India – a nationwide assessment. Sci. Total Environ. 763, 142999. doi:10.1016/j.scitotenv.2020.142999
Mao, J., Jacob, D. J., Evans, M., Olson, J., Ren, X., Brune, W., et al. (2010). Chemistry of hydrogen oxide radicals (HO x) in the Arctic troposphere in spring. Atmos. Chem. Phys. 10, 5823–5838. doi:10.5194/acp-10-5823-2010
Martinez, J. R., Maxwell, C., Javitz, H. S., and Bawol, R. (1983). “Performance evaluation of the empirical kinetic modeling approach (EKMA),” in Air pollution modeling and its application II, 199–211.
Matthias, V., Quante, M., Arndt, J. A., Badeke, R., Fink, L., Petrik, R., et al. (2021). The role of emission reductions and the meteorological situation for air quality improvements during the COVID-19 lockdown period in central Europe. Atmos. Chem. Phys. 21, 13931–13971. doi:10.5194/acp-21-13931-2021
Mertens, M., Grewe, V., Rieger, V. S., and Jöckel, P. (2018). Revisiting the contribution of land transport and shipping emissions to tropospheric ozone. Atmos. Chem. Phys. 18, 5567–5588. doi:10.5194/acp-18-5567-2018
Miyazaki, K., Bowman, K., Sekiya, T., Takigawa, M., Neu, J. L., Sudo, K., et al. (2021). Global tropospheric ozone responses to reduced NO x emissions linked to the COVID-19 worldwide lockdowns. Sci. Adv. 7, eabf7460. doi:10.1126/sciadv.abf7460
Mueller, N., Westerby, M., and Nieuwenhuijsen, M. (2023). Health impact assessments of shipping and port-sourced air pollution on a global scale: a scoping literature review. Environ. Res. 216, 114460. doi:10.1016/j.envres.2022.114460
Murray, L. T., Jacob, D. J., Logan, J. A., Hudman, R. C., and Koshak, W. J. (2012). Optimized regional and interannual variability of lightning in a global chemical transport model constrained by LIS/OTD satellite data. J. Geophys. Res. Atmos. 117. doi:10.1029/2012jd017934
Nigam, R., Pandya, K., Luis, A. J., Sengupta, R., and Kotha, M. (2021). Positive effects of COVID-19 lockdown on air quality of industrial cities (Ankleshwar and Vapi) of Western India. Sci. Rep. 11, 4285. doi:10.1038/s41598-021-83393-9
Ntziachristos, L., Papadimitriou, G., Ligterink, N., and Hausberger, S. (2016). Implications of diesel emissions control failures to emission factors and road transport NOx evolution. Atmos. Environ. 141, 542–551. doi:10.1016/j.atmosenv.2016.07.036
Ou, Y., Li, Z., Chen, C., Zhang, Y., Li, K., Shi, Z., et al. (2022). Evaluation of MERRA-2 aerosol optical and component properties over China using SONET and PARASOL/GRASP data. Remote Sens. 14, 821. doi:10.3390/rs14040821
Pappin, A. J., Hakami, A., Blagden, P., Nasari, M., Szyszkowicz, M., and Burnett, R. T. (2016). Health benefits of reducing NO x emissions in the presence of epidemiological and atmospheric nonlinearities. Environ. Res. Lett. 11, 064015. doi:10.1088/1748-9326/11/6/064015
Park, R. J., Jacob, D. J., Field, B. D., Yantosca, R. M., and Chin, M. (2004). Natural and transboundary pollution influences on sulfate-nitrate-ammonium aerosols in the United States: implications for policy. J. Geophys. Res. Atmos. 109. doi:10.1029/2003jd004473
Priyan, S., Guo, Y., McNabola, A., Broderick, B., Caulfield, B., O'Mahony, M., et al. (2024). Detecting and quantifying PM2.5 and NO2 contributions from train and road traffic in the vicinity of a major railway terminal in Dublin, Ireland. Environ. Pollut. 361, 124903. doi:10.1016/j.envpol.2024.124903
Qiu, Y., Ma, Z., Li, K., Lin, W., Tang, Y., Dong, F., et al. (2020). Markedly enhanced levels of peroxyacetyl nitrate (PAN) during COVID-19 in Beijing. Geophys. Res. Lett. 47, e2020GL089623. doi:10.1029/2020gl089623
Rajagopal, K., Ramachandran, S., and Mishra, R. K. (2024). Size resolved particle contribution to vehicle induced ultrafine particle number concentration in a metropolitan curbside region. Atmos. Environ. 337, 120773. doi:10.1016/j.atmosenv.2024.120773
Ramacher, M. O. P., Matthias, V., Aulinger, A., Quante, M., Bieser, J., and Karl, M. (2020). Contributions of traffic and shipping emissions to city-scale NOx and PM2.5 exposure in Hamburg. Atmos. Environ. 237, 117674. doi:10.1016/j.atmosenv.2020.117674
Rodríguez-Sánchez, A., Santiago, J. L., Vivanco, M. G., Sanchez, B., Rivas, E., Martilli, A., et al. (2024). How do meteorological conditions impact the effectiveness of various traffic measures on NOx concentrations in a real hot-spot? Sci. Total Environ. 954, 176667. doi:10.1016/j.scitotenv.2024.176667
Sacks, J. D., Fann, N., Gumy, S., Kim, I., Ruggeri, G., and Mudu, P. (2020). Quantifying the public health benefits of reducing air pollution: critically assessing the features and capabilities of WHO’s AirQ+ and U.S. EPA’s environmental benefits mapping and analysis program—community edition (BenMAP—CE). Atmos. (Basel). 11, 516. doi:10.3390/atmos11050516
Sang, S., Chu, C., Zhang, T., Chen, H., and Yang, X. (2022). The global burden of disease attributable to ambient fine particulate matter in 204 countries and territories, 1990–2019: a systematic analysis of the Global Burden of Disease Study 2019. Ecotoxicol. Environ. Saf. 238, 113588. doi:10.1016/j.ecoenv.2022.113588
Schraufnagel, D. E., Balmes, J. R., de Matteis, S., Hoffman, B., Kim, W. J., Perez-Padilla, R., et al. (2019). Health benefits of air pollution reduction. Ann. Am. Thorac. Soc. 16, 1478–1487. doi:10.1513/annalsats.201907-538cme
Shakoor, A., Chen, X., Farooq, T. H., Shahzad, U., Ashraf, F., Rehman, A., et al. (2020). Fluctuations in environmental pollutants and air quality during the lockdown in the USA and China: two sides of COVID-19 pandemic. Air Qual. Atmos. and Health 13, 1335–1342. doi:10.1007/s11869-020-00888-6
Shao, P., An, J., Xin, J., Wu, F., Wang, J., Ji, D., et al. (2016). Source apportionment of VOCs and the contribution to photochemical ozone formation during summer in the typical industrial area in the Yangtze River Delta, China. Atmos. Res. 176, 64–74. doi:10.1016/j.atmosres.2016.02.015
Sharma, S., Zhang, M., Anshika, , Gao, J., Zhang, H., and Kota, S. H. (2020). Effect of restricted emissions during COVID-19 on air quality in India. Sci. Total Environ. 728, 138878. doi:10.1016/j.scitotenv.2020.138878
Shen, Y., de Hoogh, K., Schmitz, O., Gulliver, J., Vienneau, D., Vermeulen, R., et al. (2024). Europe-wide high-spatial resolution air pollution models are improved by including traffic flow estimates on all roads. Atmos. Environ. 335, 120719. doi:10.1016/j.atmosenv.2024.120719
Sicard, P., De Marco, A., Agathokleous, E., Feng, Z., Xu, X., Paoletti, E., et al. (2020). Amplified ozone pollution in cities during the COVID-19 lockdown. Sci. Total Environ. 735, 139542. doi:10.1016/j.scitotenv.2020.139542
Sillman, S. (1999). The relation between ozone, NOx and hydrocarbons in urban and polluted rural environments. Atmos. Environ. 33, 1821–1845. doi:10.1016/s1352-2310(98)00345-8
Song, J., Wang, Y., Zhang, Q., Qin, W., Pan, R., Yi, W., et al. (2023). Premature mortality attributable to NO2 exposure in cities and the role of built environment: a global analysis. Sci. Total Environ. 866, 161395. doi:10.1016/j.scitotenv.2023.161395
Stevenson, M., Thompson, J., de Sá, T. H., Ewing, R., Mohan, D., McClure, R., et al. (2016). Land use, transport, and population health: estimating the health benefits of compact cities. lancet 388, 2925–2935. doi:10.1016/s0140-6736(16)30067-8
Su, X., Huang, Y., Wang, L., Cao, M., and Feng, L. (2023). Validation and diurnal variation evaluation of MERRA-2 multiple aerosol properties on a global scale. Atmos. Environ. 311, 120019. doi:10.1016/j.atmosenv.2023.120019
Sun, W., Jiang, W., and Li, R. (2024a). Global health benefits of shipping emission reduction in early 2020. Atmos. Environ. 333, 120648. doi:10.1016/j.atmosenv.2024.120648
Sun, W., Lu, K., and Li, R. (2024b). Global estimates of ambient NO2 concentrations and long-term health effects during 2000–2019. Environ. Pollut. 359, 124562. doi:10.1016/j.envpol.2024.124562
Tautan, M., Zoran, M., Radvan, R., Savastru, D., Tenciu, D., and Stanciu, A. (2024) The effects of air quality and the impact of climate conditions on the first COVID-19 wave in wuhan and four European metropolitan regions, 15, 1230.
Tian, L., Ho, K.-f., Louie, P. K. K., Qiu, H., Pun, V. C., Kan, H., et al. (2013). Shipping emissions associated with increased cardiovascular hospitalizations. Atmos. Environ. 74, 320–325. doi:10.1016/j.atmosenv.2013.04.014
Tong, R., Liu, J., Wang, W., and Fang, Y. (2020). Health effects of PM2. 5 emissions from on-road vehicles during weekdays and weekends in Beijing, China. Atmos. Environ. 223, 117258. doi:10.1016/j.atmosenv.2019.117258
Vestreng, V., Ntziachristos, L., Semb, A., Reis, S., Isaksen, I. S. A., and Tarrasón, L. (2009). Evolution of NOx emissions in Europe with focus on road transport control measures. Atmos. Chem. Phys. 9, 1503–1520. doi:10.5194/acp-9-1503-2009
Wang, D., Zhou, J., Han, L., Tian, W., Wang, C., Li, Y., et al. (2023). Source apportionment of VOCs and ozone formation potential and transport in Chengdu, China. Atmos. Pollut. Res. 14, 101730. doi:10.1016/j.apr.2023.101730
Weagle, C. L., Snider, G., Li, C., Van Donkelaar, A., Philip, S., Bissonnette, P., et al. (2018). Global sources of fine particulate matter: interpretation of PM2. 5 chemical composition observed by SPARTAN using a global chemical transport model. Environ. Sci. and Technol. 52, 11670–11681. doi:10.1021/acs.est.8b01658
Wesely, M. (2007). Parameterization of surface resistances to gaseous dry deposition in regional-scale numerical models. Atmos. Environ. 41, 52–63. doi:10.1016/j.atmosenv.2007.10.058
Xiang, S., Liu, J., Tao, W., Yi, K., Xu, J., Hu, X., et al. (2020). Control of both PM2. 5 and O3 in Beijing-Tianjin-Hebei and the surrounding areas. Atmos. Environ. 224, 117259. doi:10.1016/j.atmosenv.2020.117259
Xu, S., Sun, C., and Liu, N. (2024). Road congestion and air pollution -Analysis of spatial and temporal congestion effects. Sci. Total Environ. 945, 173896. doi:10.1016/j.scitotenv.2024.173896
Xu, W., Zhao, Y., Liu, X., Dore, A. J., Zhang, L., Liu, L., et al. (2018). Atmospheric nitrogen deposition in the Yangtze River basin: spatial pattern and source attribution. Environ. Pollut. 232, 546–555. doi:10.1016/j.envpol.2017.09.086
Yan, R.-H., Peng, X., Lin, W., He, L.-Y., Wei, F.-H., Tang, M.-X., et al. (2022). Trends and challenges regarding the source-specific health risk of PM2. 5-bound metals in a Chinese megacity from 2014 to 2020. Environ. Sci. and Technol. 56, 6996–7005. doi:10.1021/acs.est.1c06948
Yang, J., Sakhvidi, M. J. Z., de Hoogh, K., Vienneau, D., Siemiatyck, J., Zins, M., et al. (2021). Long-term exposure to black carbon and mortality: a 28-year follow-up of the GAZEL cohort. Environ. Int. 157, 106805. doi:10.1016/j.envint.2021.106805
Ye, T., Guo, S., Xie, Y., Chen, Z., Abramson, M. J., Heyworth, J., et al. (2021). Health and related economic benefits associated with reduction in air pollution during COVID-19 outbreak in 367 cities in China. Ecotoxicol. Environ. Saf. 222, 112481. doi:10.1016/j.ecoenv.2021.112481
Zara, M., van der A, R., Ding, J., Stavrakou, T., and Boersma, F. (2024). OMI-based emission source classification in East China and its spatial redistribution in view of pollution control measures. Environ. Monit. Assess. 196, 323. doi:10.1007/s10661-024-12421-8
Zhang, G., Xu, H., Wang, H., Xue, L., He, J., Xu, W., et al. (2020). Exploring the inconsistent variations in atmospheric primary and secondary pollutants during the 2016 G20 summit in Hangzhou, China: implications from observations and models. Atmos. Chem. Phys. 20, 5391–5403. doi:10.5194/acp-20-5391-2020
Zhang, Y., Eastham, S. D., Lau, A. K. H., Fung, J. C. H., and Selin, N. E. (2021). Global air quality and health impacts of domestic and international shipping. Environ. Res. Lett. 16, 084055. doi:10.1088/1748-9326/ac146b
Keywords: COVID-19, transportation emission, GEOS-Chem, health benefits, atmosphere pollutants
Citation: Zhao Y, Chen Y, Zhuo F and Fu H (2024) Global health benefits associated with a substantial decrease in land transportation emissions during the COVID-19 period. Front. Environ. Sci. 12:1519984. doi: 10.3389/fenvs.2024.1519984
Received: 30 October 2024; Accepted: 21 November 2024;
Published: 09 December 2024.
Edited by:
Nana Wu, North Carolina State University, United StatesReviewed by:
Mohamed Ahmed Ibrahim Ahmed, Assiut University, EgyptZirui Liu, Chinese Academy of Sciences (CAS), China
Copyright © 2024 Zhao, Chen, Zhuo and Fu. This is an open-access article distributed under the terms of the Creative Commons Attribution License (CC BY). The use, distribution or reproduction in other forums is permitted, provided the original author(s) and the copyright owner(s) are credited and that the original publication in this journal is cited, in accordance with accepted academic practice. No use, distribution or reproduction is permitted which does not comply with these terms.
*Correspondence: Hongbo Fu, fuhb@fudan.edu.cn