- Hebei Bureau of Geology and Mineral Resources Exploration and Development, Land and Resources Exploration Center, Shijiazhuang, China
Introduction: As an important part of terrestrial ecosystem, vegetation plays a vital role in the terrestrial carbon cycle. As one of China’s three major urban clusters, the Beijing-Tianjin-Hebei region has experienced significant changes in vegetation due to climate change and large-scale ecological restoration efforts. Climate change and human activities are two factors that have important effects on the carbon cycles of terrestrial ecosystems. Quantitative assessment of the relative effects of climate change and human activities on vegetation dynamics is of paramount importance for regional sustainable development and ecological security.
Methods: In this study, based on ground meteorological data and remote sensing data including NDVI, an improved Carnegie-Ames-Stanford Approach model was employed to estimate the net primary productivity (NPP) of vegetation in the region from 2000 to 2020. Utilizing methods such as trend analysis, Mann-Kendall significance test, and correlation analysis, we investigated the spatiotemporal patterns of vegetation NPP and its correlations with precipitation, temperature, and solar radiation. Furthermore, the partial derivative trend residuals method was adopted to separate and quantify the impacts of climate change and human activities on vegetation NPP in different years.
Results: The results show that: (1) from 2000 to 2020, the overall trend of vegetation NPP in the Beijing-Tianjin-Hebei region exhibited an increasing trend, with a spatial distribution pattern showing a step-like distribution from low to high to low from northwest to southeast. The multi-year average NPP was 384.90 g Cm−2, with an annual average growth rate of 3.00 g Cm−2. The NPP values of different vegetation types from large to small were: forests (578.40 g C m−2), shrubland (386.57 g C m−2), grassland (380.17 g C m−2), and cropland (324.91 g C m−2). (2) The proportion of regions with a positive correlation coefficient between average temperature and vegetation NPP in the Beijing-Tianjin-Hebei region from 2000 to 2020 was 69.85%, while the proportions of regions with positive correlations between precipitation and solar radiation with vegetation NPP were 92.1% and 89.19%, respectively. This indicates that vegetation NPP in most regions is positively correlated with precipitation, solar radiation, and temperature, with precipitation exerting a greater influence on vegetation NPP than temperature and solar radiation. (3) Vegetation improvement in the Beijing-Tianjin-Hebei region is mainly driven by both climatic factors and human activities, while vegetation degradation is primarily caused by human factors. The relative contributions of climate change and human activities to changes in vegetation NPP in the study area are 45.11% and 54.89%, respectively. The areas where they positively contribute account for 48.64% and 73.95% of the total study area, respectively, with the proportions of areas with relative contribution rates >60% being 45.86% and 28.86%, respectively.
Discussion: The findings of this study can provide scientific basis for vegetation restoration, low-carbon development, and ecosystem management decision-making in the Beijing-Tianjin-Hebei region.
1 Introduction
As an important part of terrestrial ecosystem, vegetation plays an important role in climate regulation and maifntenance of terrestrial carbon balance (Peng et al., 2012; Bai et al., 2023; Beltrán-Sanz et al., 2022). Net primary productivity (NPP) of vegetation refers to the portion of the total organic matter produced by plants through photosynthesis in a given unit of time and area, after subtracting the amount lost through autotrophic respiration (Field et al., 1995). Its magnitude not only directly reflects the productive capacity of vegetation under natural environmental conditions but also effectively indicates the vegetation’s response to its surrounding environment and environmental changes (Running et al., 2004; Gao et al., 2022). Quantifying and assessing the relative impacts of climate change and human activities on NPP is crucial for evaluating the carbon sink function of ecosystems and maintaining the stability of regional ecosystems.
Although numerous quantitative studies have assessed changes in NPP globally, the relative impact of climate change and human activities on vegetation remains uncertain, particularly at regional scale. Typically, changes in vegetation NPP within a region are often influenced by both climate factors, particularly temperature and precipitation (Zhu et al., 2017), and human activities such as reforestation and grassland restoration (Hong et al., 2021), ecological engineering (Liu et al., 2019; Yang et al., 2014), changes in land use practices (Haberl et al., 2005), and overgrazing (Yang et al., 2023). Existing studies indicate that the primary factor contributing to the increase in NPP in southwest China is the implementation of ecological projects such as reforestation and grassland restoration (Hong et al., 2021). In contrast, in North China, human activities play a dominant role in both vegetation improvement and degradation.
In recent years, studies aimed at identifying the causes of vegetation changes due to climate change and human activities have primarily utilized methods such as the coefficient of variation (Li et al., 2016), geographical detector (Zhao et al., 2020; Zuo and Gap, 2020), regression analysis (Xie et al., 2014), and the residual trend method (Tong et al., 2019). These approaches are used to spatially quantify and distinguish the impacts of human activities and climate change on NPP. The residual trend method quantitatively assesses the relative impact of human activities by calculating the difference between actual and potential vegetation productivity (Zhou et al., 2015). Potential vegetation productivity refers to the NPP of vegetation influenced solely by natural conditions (such as temperature and precipitation), representing the highest possible state of vegetation succession in the absence of human interference. In contrast, actual vegetation productivity reflects the combined effects of climate change and human activities on NPP. The difference between potential and actual productivity is defined as the impact value of human activities. This method, due to its clear biological significance and simple calculation process, has become a primary approach for quantitatively studying the driving forces behind changes in vegetation NPP (Yin et al., 2020; Tong et al., 2020; Li et al., 2014). However, when deriving potential NPP through the regression of meteorological factors and NPP, the influence of human activities can easily be confounded (Wessels et al., 2012). This is because long-term changes in meteorological factors themselves can be influenced by human activities, such as increased atmospheric CO2 concentrations due to human activities. Therefore, to improve the accuracy of assessments of the impacts of climate change and human activities on NPP, it is essential to minimize the influence of human activities on climate change as much as possible.
As one of the world’s most densely populated and highly urbanized regions, Beijing-Tianjin-Hebei region holds a significant position in China’s economic development. In recent years, with the accelerating pace of industrialization and the continuous increase in urbanization, this region has garnered widespread attention from scholars due to its susceptibility to ecological and environmental issues. These issues include vegetation degradation, frequent heavy pollution events, and water shortages, which are exacerbated by the combined impacts of climate change and human activities (Xin et al., 2024; Wu et al., 2023). At the same time, the spatial distribution of NPP in this region is strongly correlated with climate change (Zhang et al., 2018). The spatial patterns of NPP degradation are often influenced by factors such as urban sprawl, the density of transportation networks, regional economic development strategies, and industrial layout (Lv et al., 2017). Currently, there has been a lack of in-depth quantitative research on the relative contributions and spatial heterogeneity of vegetation NPP changes in the Beijing-Tianjin-Hebei region. This is particularly crucial given the intensifying interactions between climate change and human activities. Therefore, this study aims to conduct a scientific evaluation of the driving mechanisms behind vegetation NPP changes in this region. Based on the spatiotemporal characteristics of NPP simulated by the CASA model, this research employs the Partial Derivative Residual Trend method to separate and quantify the impacts of climate change and human activities on vegetation NPP. Investigating the spatial heterogeneity of these impacts is essential for uncovering the driving mechanisms of vegetation changes in the region. Additionally, the findings will contribute to promoting coordinated economic and social development in the Beijing-Tianjin-Hebei region and improving ecological management and protection measures.
2 Materials and methods
2.1 Study area
The Beijing-Tianjin-Hebei region (including Hebei Province, Beijing, and Tianjin) is located in the northern heartland of China, spanning longitudes 113°04′to 119°53′E and latitudes 36°01′to 42°37′N (Figure 1). The region covers a total area of 217,300 km2, accounting for 2.2% of the nation’s total land area. It serves as the country’s political, cultural, international exchange, and technological innovation center (Ren et al., 2023). The region features a varied topography, with high terrain in the northwest and low terrain in the southeast. The northern part is dominated by the Yanshan Mountains, while the southern part is part of the North China Plain, with the Taihang Mountains to the west and the Bohai Bay to the east. This diverse landscape is the only area in the country that encompasses plateaus, mountains, hills, basins, plains, and coastal regions, exhibiting a typical semi-circular stepped topography. The climate is a typical warm temperate semi-humid continental monsoon climate (Xu et al., 2021), with high temperatures and abundant rainfall from June to September, influenced by Pacific moisture. From November to January, the region experiences cold and dry conditions due to the Mongolian-Siberian cold front. The annual average temperature is 15°C–16°C, with annual sunshine hours ranging from 2,500 to 2,900 h and total annual radiation between 5,000 and 5,800 MJ/m2. The annual average precipitation ranges from 304 to 750 mm, with a distribution pattern of more rainfall in the southeast and less in the northwest. The frost-free period lasts from 20 to 200 days. The region is rich in ecological resources and diverse plant species. Urbanization is low in the northern towns and high in the central cities, although ecological degradation is severe. There is a significant regional variation in vegetation, with a gradual decrease in natural vegetation from north to south (Wu et al., 2023). The northern plateau region is primarily grassland and forest, including white pine forests, huashan pine forests, liaodong oak forests, and shrublands of thorny bushes and jujube trees. The central and southern regions are characterized by temperate deciduous broadleaf forests, while the southern plains are mainly used for crop cultivation.
2.2 Data description
The foundational data required for this study includes remote sensing data, land use data, and meteorological data. The NDVI data used to estimate vegetation NPP was sourced from the MODIS product MOD13A3 dataset provided by NASA/EOS LPDAAC (https://lpdaac.usgs.gov/), with a temporal resolution of 1 month and a spatial resolution of 250 m. Preprocessing, including image mosaicking, clipping, and maximum value compositing, was conducted using the MODIS Reprojection Tools (MRT) and ArcGIS software. NDVI data were filtered to remove anomalies, resulting in monthly NDVI data for the study area from 2000 to 2020. The land use data was obtained from the CLCD (China Land Cover Dataset) (https://cds.climate.copernicus.eu; Yang and Huang, 2021). This dataset is based on all available Landsat data on Google Earth Engine (GEE), combined with stable samples extracted from the China Land Use/Cover Dataset (CLUD) and visually interpreted samples from Google Earth. Using a random forest classifier, annual land use data from 2000 to 2020 with a spatial resolution of 30 m was generated, achieving an overall accuracy of 80%.The meteorological data were sourced from the China Meteorological Data Center (http://data.cma.cn/site/index.html), including average temperature (TEM) (°C), total precipitation (PRE) (mm), and sunshine duration (SUN) (h) data. Data from 69 meteorological stations within and around the Beijing-Tianjin-Hebei region were interpolated monthly from 2000 to 2020 into gridded meteorological data at a 250 m resolution using kriging. The administrative boundary data for the Beijing-Tianjin-Hebei region was obtained from the National Basic Geographic Information Center (http://ngcc.sbsm.gov.cn).The above datasets were all resampled to raster data with a spatial resolution of 250 m, consistent with the projection information of the NDVI data.
2.3 Methodology
2.3.1 NPP estimation
In this study, the CASA (Carnegie-Ames-Stanford Approach) model established by Potter in 1993 (Potter et al., 1993) was used to estimate the actual productivity of vegetation. The main input parameters for the model are the absorbed photosynthetically active radiation (APAR) and the light use efficiency (
In the equation, x represents the position of the pixel; t represents time;
In the equation,
2.3.2 Theil-sen slope trend analysis
The Theil-Sen slope trend analysis is a robust non-parametric statistical method used to detect trends, widely applied in meteorological and vegetation data analysis. One of the main advantages of this method is that it does not require the data to follow a specific distribution (Thiel, 1950). Additionally, the Mann-Kendall significance test is employed to robustly quantify long-term trends in meteorological and vegetation data (Hamed and Rao, 1998; Zhang et al., 2012). Based on the significance difference test’s p-values (p = 0.10, p = 0.05, p = 0.01) and combined with the trend of NPP changes, the results are categorized into eight situations: extremely significant increase, significant increase, slightly significant increase, non-significant increase, extremely significant decrease, significant decrease, slightly significant decrease, and non-significant decrease.
2.3.3 Stability analysis
The coefficient of variation (CV) is a statistical measure that assesses the degree of variability in the observed sequence values and can effectively reflect the differences in spatial data over time series, as well as evaluate the stability of data time series (Mou et al., 2023). In this study, the stability of NPP was assessed using the coefficient of variation method, with stability grading criteria based on references (Equation 3) Liu et al. (2019) and Sun et al. (2019). The calculation formula is as follows:
In the equation,
2.3.4 Correlation analysis
The variation of NPP is influenced by multiple factors such as climate, leading to a complex coupling process (Yan et al., 2021). In this study, Pearson correlation coefficient was employed to calculate the correlation between NPP and meteorological factors including temperature, precipitation, and total solar radiation, and to analyze their relationships (Equation 4). The calculation formula is as follows:
In the equation,
2.3.5 Separation and quantification of climate change and human activities influence
This study employs the partial trends residual method to separate and quantify the influence of climate change and human activities on vegetation NPP variation (Zhang et al., 2022). The contributions of climate change and human activities to vegetation NPP variation are denoted as
In the equations,
By performing subtractive calculations between
Finally, based on
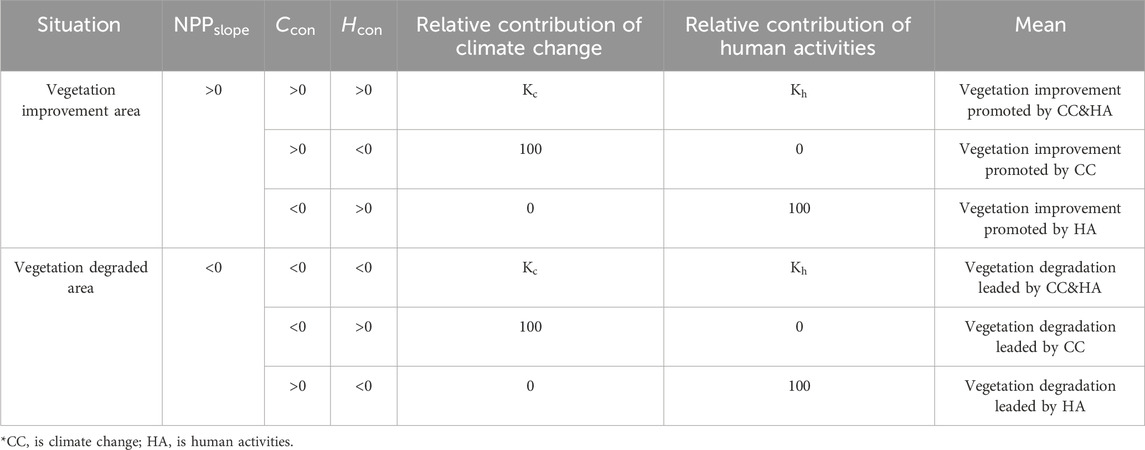
Table 1. Methods for assessing the relative contributions of climate change and human activities to vegetation change under different scenarios.
3 Results
3.1 Spatiotemporal characteristics of NPP in Beijing-Tianjin-Hebei region in 2000–2020
The overall trend of vegetation NPP in the Beijing-Tianjin-Hebei region from 2000 to 2020 showed a fluctuating increase (Figure 2). The annual mean of NPP ranged between 341.62 and 443.62 g C m−2, with a multi-year average of 384.90 g C m−2. The highest value was recorded in 2020, reaching 443.62 g C m−2, while the lowest occurred in 2006, at 341.62 g C m−2. The linear trend rate was 3.00 g C m−2 (p < 0.01), indicating a significant recovery in vegetation productivity in the region. Similarly, the 21-year average NPP of four vegetation cover types—cropland, forests, grassland, and shrubland—also showed a fluctuating growth trend. The NPP values of different vegetation types, from highest to lowest, were forests (578.40 g C m−2), shrubland (386.57 g C m−2), grassland (380.17 g C m−2), and cropland (324.91 g C m−2). Among these, forests showed the highest linear trend rate of 3.88 g C m−2 (p < 0.01), suggesting that the most pronounced recovery in vegetation potential occurred in forested areas.
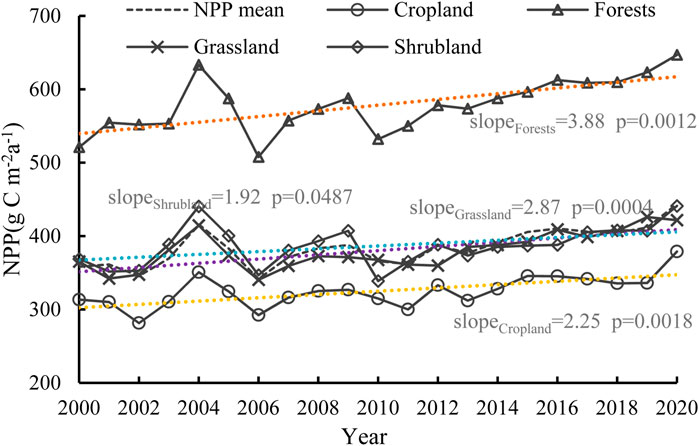
Figure 2. Inter-annual variation of NPP of different vegetation types in Beijing-Tianjin-Hebei from 2000 to 2020 computed using CASA model.
The spatial distribution of average vegetation NPP in the Beijing-Tianjin-Hebei region from 2000 to 2020 is showed in Figure 3A. It is evident from the figure that there was significant spatial heterogeneity in the distribution of vegetation NPP across the region. Apart from the northwest corner, the overall trend showed a stepwise distribution pattern of low-high-low from northwest to southeast. Over the past 21 years, the regional average NPP ranged between 0 and 854.44 g C m−2, predominantly concentrated between 200 and 600 g C m−2, covering 83.41% of the total study area. Areas with NPP < 200 g C m−2 accounted for 5.02% of the total area, mainly distributed in the central areas of each county in the study area and southwestern Zhangjiakou. Areas with NPP > 600 g C m−2 accounted for 10.07% of the total area, mainly distributed throughout Chengde city, northern parts of Beijing, Qinhuangdao, Baoding, and eastern parts of Zhangjiakou. These areas exhibit higher vegetation coverage and higher NDVI values, leading to higher NPP values.
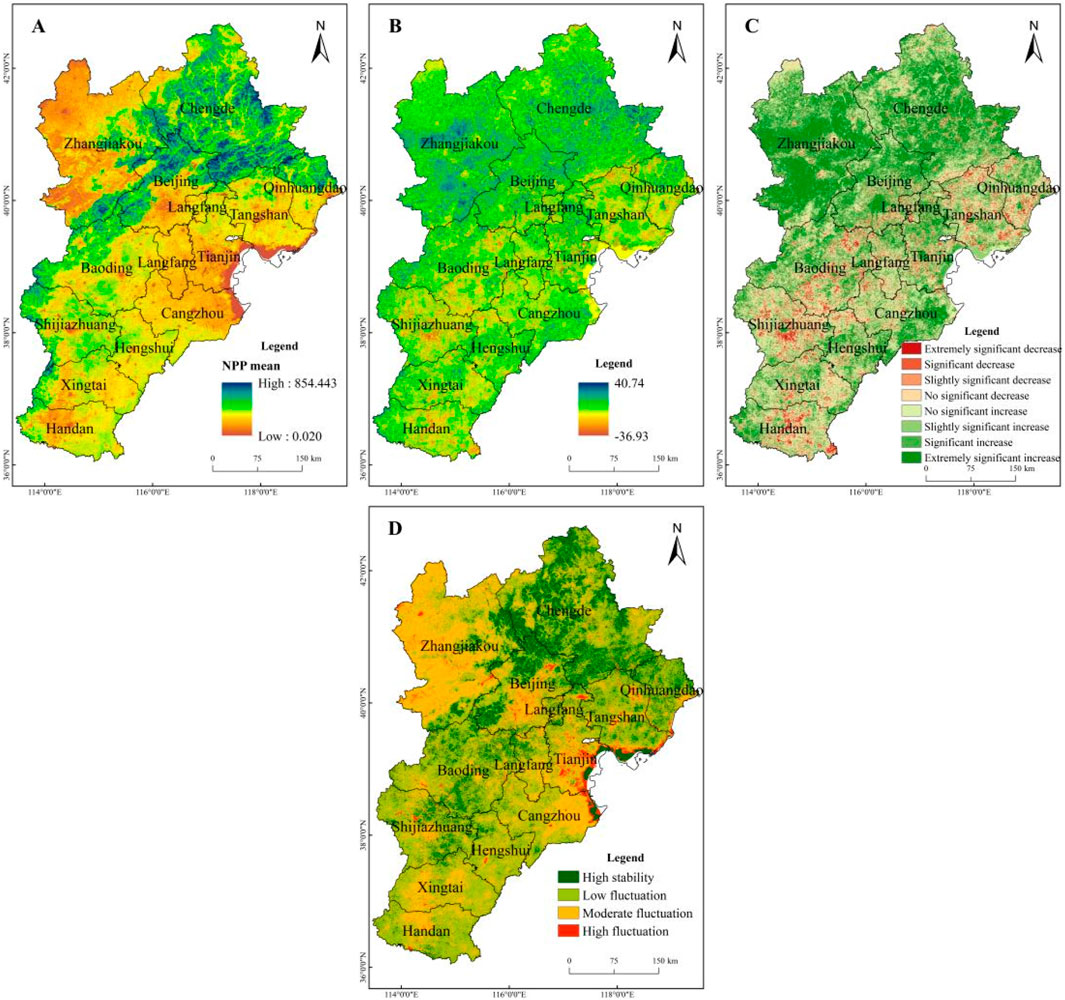
Figure 3. Spatial distribution (A), change trend (B), significance test (C) and stability analysis (D) of mean NPP in Beijing-Tianjin-Hebei Region from 2000 to 2020.
The spatiotemporal trends of NPP in the Beijing-Tianjin-Hebei region from 2000 to 2020 are depicted in Figure 3B, while the results of the Mann-Kendall (MK) test are shown in Figure 3C. It is evident from the figures that the regional average NPP has generally improved during the period from 2000 to 2020. Areas with improved NPP cover approximately 79.32% of the total study area, with significantly improved areas accounting for 54.13%. These significant improvements are mainly observed in Zhangjiakou, Chengde, northern parts of Beijing and Tianjin, and northern parts of Qinhuangdao. The significant improvement in vegetation coverage in these areas is attributed to large-scale implementation of key ecological projects at the national, provincial, and municipal levels, such as the control of sand sources in Beijing and Tianjin, returning farmland to forests, ecological water source protection forests in the Beijing-Tianjin region, and national reserve forests (Zhang et al., 2016). Areas experiencing vegetation degradation cover approximately 20.68% of the total study area, with significantly degraded areas accounting for 6.99%. These degraded areas are mainly distributed in Shijiazhuang, Handan, Tangshan, and eastern parts of Baoding. The low vegetation coverage in these areas is primarily due to the significant impact of urban development and construction activities, resulting in a noticeable decrease in NPP.
Figure 3D illustrated the spatial distribution of the coefficient of variation of NPP in the study area over the 21-year period. It is observed that the vegetation NPP in the Beijing-Tianjin-Hebei region remained stable during this period. Areas characterized by high stability and low variability cover approximately 65.10% of the total area. Regions with moderate variability cover 32.45% of the total study area, mainly distributed in Zhangjiakou, Beijing, Tianjin, central parts of Cangzhou, and western parts of Xingtai and Handan. Among these, fluctuations in NPP in Zhangjiakou, Beijing, and Tianjin are influenced by the implementation of ecological protection projects, while the relatively flat terrain and lower elevation in the eastern parts of Cangzhou and western parts of Xingtai and Handan, where the primary vegetation type is grassland, are prone to fluctuations due to external environmental factors. Areas with high variability cover 2.45% of the total area of the Beijing-Tianjin-Hebei region, mainly distributed in Tangshan, southern parts of Tianjin and Beijing, and eastern parts of Cangzhou. The significant fluctuations in vegetation in these areas are attributed to urban expansion and human activities.
3.2 Relationship between NPP and meteorological factors in Beijing-Tianjin-Hebei region
The spatial and temporal variation of temperature, precipitation, and solar radiation, along with their spatial correlation with vegetation NPP, were shown in Figure 4. The overall spatial distribution of temperature in the Beijing-Tianjin-Hebei region displayed a pattern of higher temperatures in the southeast and lower temperatures in the northwest (Figure 4A). The annual average temperature ranged from 3.73°C to 14.23°C, with the lowest temperatures observed in northern Zhangjiakou and Chengde, while the highest temperatures were concentrated in the southeastern plains. In terms of trends, the region showed a significant warming trend overall (Figure 4B), with the most pronounced increases occurring in the southeastern part of the region and southern Chengde, where the warming rate exceeded 0.04°C per year in some areas. There were also regions where temperatures decreased, particularly in eastern Baoding, western Langfang, and northern Chengde, though the areas affected were small, and the decrease was not significant. Temperature plays a crucial role in plant growth, with suitable temperatures promoting the accumulation of NPP. The analysis of the correlation between temperature and NPP in the study area (Figure 4C) reveals that, overall, NPP in the Beijing-Tianjin-Hebei region shows a positive correlation with temperature. Regions with an insignificant positive correlation account for 61.00% of the study area, primarily located in northern areas such as Zhangjiakou, Chengde, Beijing, Tangshan, and Tianjin. Areas with an insignificant negative correlation account for 28.41%, mainly distributed in the southern part of the study area. Regions where the correlation passed the significance test cover 10.59% of the total area, indicating that the relationship between temperature and NPP in the region is not very strong. Areas with significant and highly significant positive correlations account for 8.85% of the study area, mostly located in the eastern regions where adequate moisture and favorable hydrothermal conditions support robust vegetation growth. Meanwhile, areas with significant and highly significant negative correlations are smaller, covering 1.74% of the region, concentrated in eastern Handan, Xingtai, Hengshui, and central Cangzhou. In these areas, higher temperatures increase plant transpiration, with most of the organic matter produced being consumed for autotrophic respiration, limiting nutrient storage in the plants.
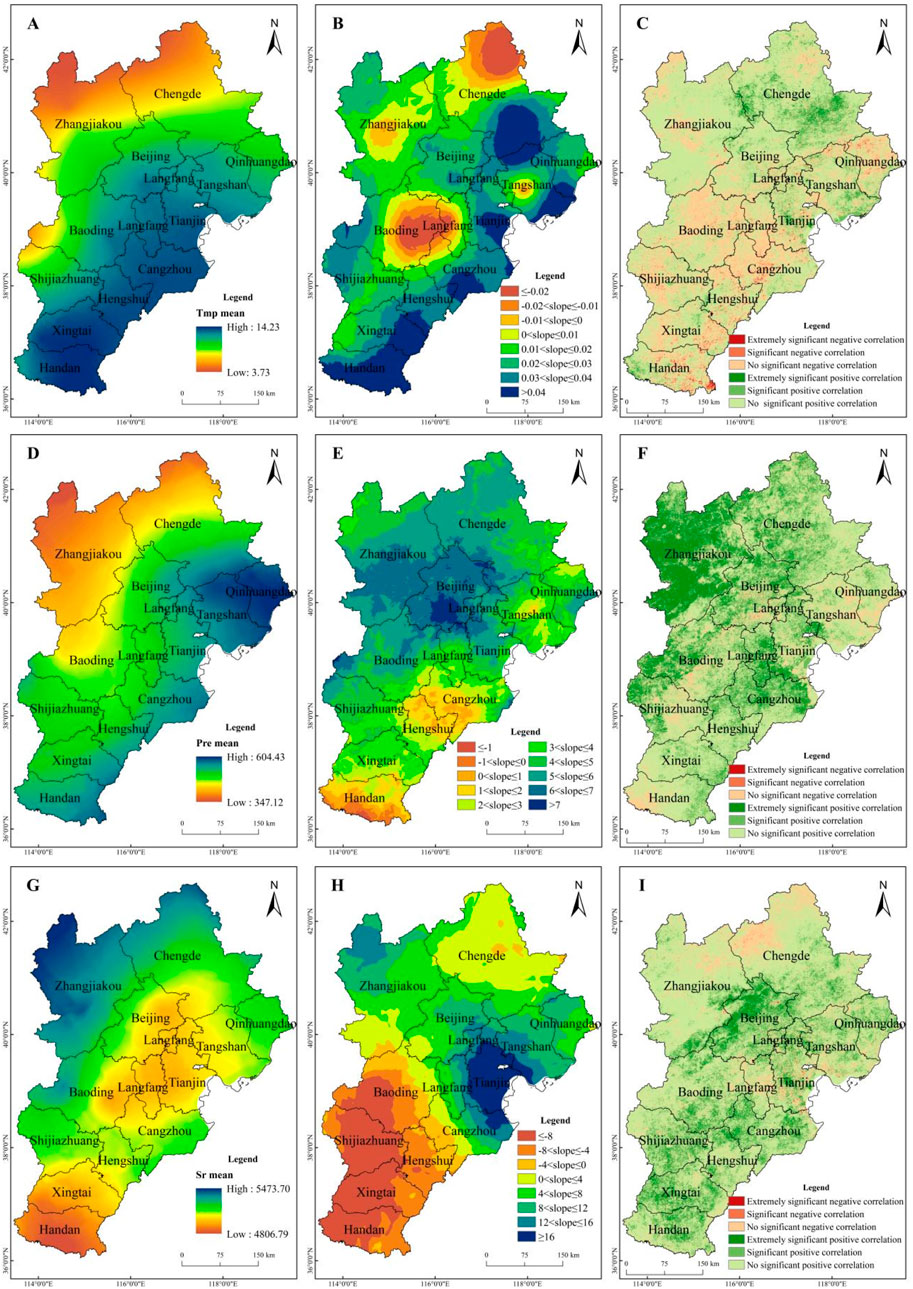
Figure 4. Spatial distribution (A, D, G) and change trend (B, E, H) of air temperature, precipitation and solar radiation in Beijing-Tianjin-Hebei region from 2000 to 2020 and their correlation with NPP (C, F, I).
The spatial distribution of precipitation in the Beijing-Tianjin-Hebei region was similar to that of temperature, generally showing a decreasing trend from the coastal areas to the inland regions (Figure 4D). The annual precipitation ranged from 346.27 to 608.89 mm, with the highest values concentrated in Qinhuangdao, Tangshan, Tianjin, and Cangzhou, and the lowest values in northern Zhangjiakou and Chengde. These low-precipitation areas were located in the Bashang grasslands, where monsoonal climate and topographic factors contributed to lower precipitation levels. In terms of trends, precipitation in the study area also exhibited a clear increasing trend (Figure 4E), particularly in the northern part of the region, with the most significant increase observed in Beijing, where the upward trend exceeded 5 mm per year. However, some areas, such as Handan, western Cangzhou, and northern Hengshui, experienced a decreasing trend in precipitation, although this decline was less than 2 mm per year. An analysis of the correlation between precipitation and vegetation NPP (Figure 4F) revealed that areas with a positive correlation coefficient accounted for 92.10% of the region, while areas with a negative correlation accounted for only 7.90%, indicating that the majority of the region’s NPP was positively correlated with annual precipitation. The regions with an insignificant negative correlation covered 7.78% of the study area, primarily distributed along the coastal areas of Qinhuangdao, western Handan, and eastern Tangshan. Regions with a significant or highly significant negative correlation were extremely small, accounting for only 0.12%, and were scattered around the eastern border of Beijing and Langfang. On the other hand, regions with an insignificant positive correlation accounted for 49.44% of the study area and were widely distributed. Areas with a significant positive correlation covered 19.77% of the study area, mainly located in Chengde, northwestern Beijing, as well as Cangzhou and Langfang, where favorable hydrothermal conditions promoted vegetation growth. Regions with a highly significant positive correlation were concentrated in the Bashang Plateau and the high-altitude forested areas of the Yanshan and Taihang mountain ranges, covering 22.89% of the study area. This indicated that precipitation had a strong positive impact on vegetation growth in these regions, with vegetation responding more intensely to changes in rainfall.
The spatial distribution of solar radiation in the Beijing-Tianjin-Hebei region, as shown in Figure 4G, exhibited a decreasing trend from the northern to the southern parts of the region. The lowest levels of solar radiation were observed in Handan and Xingtai, while the highest levels were found in the northern Bashang region of Zhangjiakou and Chengde. This is due to the higher altitude of these areas, where the atmosphere has less of an attenuating effect on solar radiation, resulting in stronger radiation reaching the ground. Over the 21-year study period, solar radiation in the region also showed an upward trend (Figure 4H), with clear spatial differentiation. Specifically, the northeastern part of the region experienced an increase in solar radiation, while the southwestern part saw a decrease. The area experiencing increased solar radiation accounted for 67.17% of the total, more than twice the size of the area with decreasing radiation. The most significant increase, exceeding 12 MJ m−2, was observed in western Tianjin, western Tangshan, and the northern Bashang region of Zhangjiakou. The analysis of the correlation between solar radiation and vegetation NPP (Figure 4I) showed that areas with a positive correlation coefficient made up 89.29% of the region, while areas with a negative correlation accounted for 10.71%. This indicated that most of the region’s vegetation NPP was positively correlated with the annual total solar radiation. Areas with an insignificant positive correlation covered 62.28% of the study area, mainly distributed in Zhangjiakou, Chengde, Tangshan, Qinhuangdao, Shijiazhuang, and Hengshui. Areas with an insignificant negative correlation accounted for 10.45% of the region, primarily located in northern Chengde, scattered areas of Langfang, and parts of Tianjin. The correlation analysis showed that 27.27% of the region passed the significance test, indicating that the correlation between solar radiation and NPP was higher than that between temperature and NPP but lower than that between precipitation and NPP. Of this, areas with a significant or highly significant positive correlation made up 27.01% of the study area, concentrated in Beijing, eastern Langfang, eastern Cangzhou, and western Tangshan. In these areas, the combination of favorable water and heat conditions, along with increased solar radiation, promoted photosynthesis, contributing to improved vegetation growth.
3.3 The impact of climate change and human activities on vegetation NPP
3.3.1 The main driving factors influencing vegetation NPP changes in the Beijing-Tianjin-Hebei region
Figure 5 showed the spatial distribution of the dominant factors influencing vegetation NPP changes in the Beijing-Tianjin-Hebei region, while Table 2 presented the area proportions of these different dominant factors. As seen from Figure 5 and Table 2, the improvement in vegetation across the Beijing-Tianjin-Hebei region was primarily driven by the combined effects of climate change and human activities, accounting for 36.94% of the area, which was mainly located in Chengde, central Zhangjiakou, and northern Baoding. The second most dominant factor was human activities alone, which influenced 28.40% of the area, mainly distributed in northern Beijing, eastern Cangzhou, northern Zhangjiakou, and western Shijiazhuang and Baoding. Climate change alone accounted for 14.26% of the area, mainly in the southern part of the study region. Vegetation degradation in the region was predominantly caused by human activities, affecting 8.79% of the area, mostly in Tangshan, Langfang, and eastern Baoding. These areas experienced significant pressure on vegetation growth due to rapid socioeconomic development and the construction of the industrial zone surrounding the capital, which led to poor vegetation recovery and degradation. The combined effects of both climate change and human activities also contributed to vegetation degradation in 6.14% of the area, mainly in central Shijiazhuang, Baoding, and eastern Handan. Vegetation degradation caused solely by climate change accounted for 5.47% of the area, primarily located in the southern part of the study region. Overall, the combined effects of climate change and human activities were the main factors contributing to vegetation improvement in the Beijing-Tianjin-Hebei region from 2000 to 2020, while human activities were the leading cause of vegetation degradation.
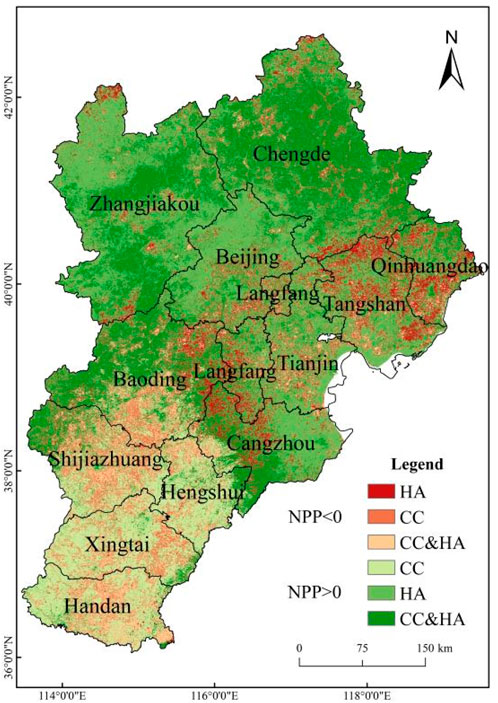
Figure 5. Drivers of NPP variability at each point according to the criteria in Table 1. *CC&HA indicates that NPP variability is driven by both climate change and human activities. CC means that NPP variability is only driven by climate change. HA indicates that NPP variability is driven by human activities alone.
3.3.2 The relative contributions of climate change and human activities to vegetation NPP
Figures 6A, B illustrated the contributions of climate change and human activities to vegetation NPP changes, while Figures 6C, D showed the relative contribution rates of climate change and human activities. Table 3 presented the relative contribution rates of climate change and human activities in areas of vegetation improvement and degradation. According to Figure 6, the mean contributions of climate change and human activities to vegetation NPP changes were 1.22 g C m⁻2 a⁻1 and 2.57 g C m⁻2 a⁻1, respectively. The areas with positive contributions from climate change and human activities accounted for 48.64% and 73.95% of the total study area, respectively. The relative contribution rates of climate change and human activities to vegetation NPP changes were 45.11% and 54.89%, respectively. Areas where the relative contribution rate of climate change exceeded 60% covered 28.86% of the total area, mainly distributed in eastern Chengde, Baoding, Hengshui, Handan, and western Cangzhou. Meanwhile, areas where the relative contribution rate of human activities exceeded 60% covered 45.86% of the total area, mainly located in Zhangjiakou, northwestern Beijing, eastern Cangzhou, most of Chengde, and scattered areas in Shijiazhuang and Baoding. The implementation of ecological projects and the enforcement of ecological protection policies in these regions significantly promoted vegetation growth, leading to a higher relative contribution of human activities. In terms of different vegetation types, human activities contributed most significantly to farmland, with a relative contribution rate of 43.29%. Forestland and grassland followed, with rates of 35.56% and 17.77%, respectively, while shrubland had the lowest rate, at 3.38%.
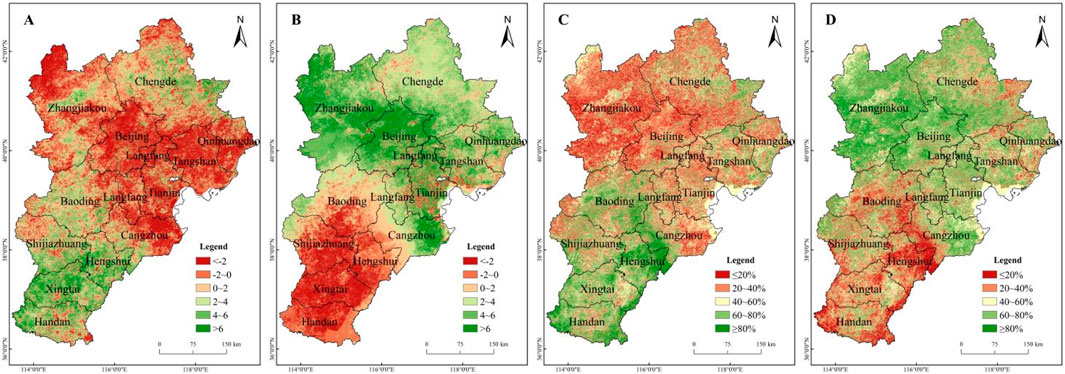
Figure 6. Spatial distributions of the contributions of climate (A) and human activities (B) to the vegetation NPP, and the contribution proportions of climate change (C) and human activities (D) to vegetation NPP.
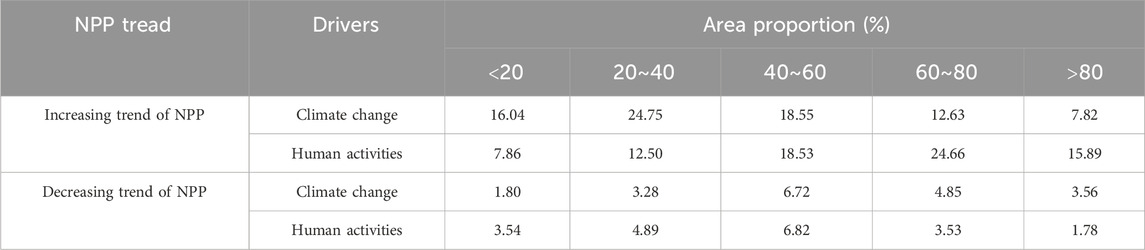
Table 3. The relative contribution rates of climate change and human activities in areas of vegetation improvement and degradation.
4 Discussion
4.1 The accuracy validation and spatio-temporal variation characteristics of NPP
The NPP is an important indicator of vegetation coverage and growth, playing a crucial role in climate regulation and maintaining terrestrial carbon balance (Brinkmann et al., 2011). The analysis of regional vegetation dynamics and their driving mechanisms has been a core focus in global change research and ecological civilization construction. Variations in methods, model structures, data sources, and spatiotemporal resolutions can lead to uncertainties in NPP estimation. In this study, NPP estimated using the CASA model closely aligns with the results of studies by Wang (2012), Liu (2008), Gao et al. (2022). Moreover, it demonstrates strong consistency with remote sensing products (Guo, 2023), indicating the CASA model’s high reliability in simulating vegetation NPP.
Through the study of the spatiotemporal distribution characteristics of vegetation NPP in the region from 2000 to 2020, it was found that the overall trend of vegetation NPP increased over time, consistent with previous research findings (Zhang, 2015; Wang et al., 2021). The reasons for this increase can be attributed to the implementation of ecological restoration projects, such as the Conversion of cropland to forest and grassland project since 2000, which improved the ecological quality of the region. Additionally, the establishment of various ecological security barriers has provided some protection to the environment in the region (Cui, 2011). In terms of spatial patterns, the NPP in the region exhibited a stepped distribution, decreasing from northwest to southeast, with high values in the Yanshan and Taihang mountains where forest vegetation predominates, and lower values in the Bashang Plateau and southeastern plains where grasslands and croplands are dominant. This pattern aligns with the findings of Lv et al. (2017). The overall vegetation in the region has been steadily recovering, especially in the sparsely populated areas of the Bashang Plateau and mountainous regions, where ecological engineering efforts have been concentrated. However, in parts of the central and coastal areas, urban expansion, agricultural irrigation, and other human activities have caused significant vegetation fluctuations, limiting recovery or even leading to degradation.
4.2 Analysis of driving mechanisms for vegetation dynamics in the Beijing-Tianjin-Hebei region
Climate and human activities are the fundamental driving forces controlling and influencing the spatial distribution and changes of vegetation (Yin et al., 2021). In this study, climate change drove an increase in NPP, accounting for 51.20% of the total. Among them, precipitation had a high explanatory power for the spatial distribution of NPP. The correlation analysis between NPP and precipitation showed that the interannual fluctuation of NPP was basically consistent with that of precipitation, indicating that vegetation is more sensitive to interannual changes in precipitation. This is consistent with the findings of Zhou et al. (2017) regarding the significant correlation between NPP and precipitation in temperate desert grasslands. Similarly, solar radiation also showed a high correlation with vegetation NPP, accounting for 27.27% of the total area through significance testing, lower than the correlation between precipitation and NPP but higher than that between temperature and NPP. This suggests that increased solar radiation benefits vegetation photosynthesis and storage, consistent with the results of Guo (2023).
The interannual fluctuation of NPP was positively correlated with temperature fluctuations overall, but only accounted for 10.79% of the study area through significance testing. The reason for this may be that suitable temperature and heat can promote vegetation growth to a certain extent, but continued warming can exacerbate drought and inhibit vegetation growth (Gao et al., 2019), even leading to withering and death of artificial vegetation due to the occurrence of dry soil layers (Chang et al., 2024). Under the background of global climate warming, the frequency, duration, intensity, and impact range of drought events have all shown a significant increasing trend (Liu et al., 2015). However, the occurrence of drought events has not changed the overall decreasing trend of NPP in the Beijing-Tianjin-Hebei region. This may be attributed to the ecological restoration projects implemented in the study area since 2000, such as returning farmland to forests and grasslands, desertification control, wetland protection, soil and water conservation, and governance.
Pixel-scale analysis revealed that human activities contributed to 67.34% of the NPP changes, with the comprehensive implementation of returning farmland to forests and grasslands in the Beijing-Tianjin-Hebei region since 2002, as well as the implementation of a series of large-scale ecological projects since 2010 and the enforcement of grazing bans. NPP has been significantly improved, and the negative impact of human activities on NPP in the Beijing-Tianjin-Hebei region has gradually weakened. In localized areas such as Tangshan, Langfang, and Baoding, NPP changes are mainly affected by human activities. Long-term and rapid urbanization activities and industrial zone construction have caused severe land pressure and landscape damage, resulting in weak vegetation stability. It is necessary to further strengthen the control of human activities and coordinate the relationship between economic development and environmental protection.
4.3 Limitations
The differences in research results may be related to various factors such as the scope of the study, the study period, and the quality of data used. Currently, large-scale studies based on remote sensing data are still limited by factors such as low spatiotemporal resolution and insufficient meteorological station coverage (Zhang et al., 2011). Additionally, while the residual analysis method has been widely used to separate the effects of human activities on vegetation NPP changes, it still has some limitations. For example, when simulating the impact of meteorological elements on vegetation NPP, only factors such as precipitation, temperature, and solar radiation are considered, while factors like relative humidity and wind speed may also affect vegetation NPP but are not fully accounted for. Similarly, when mentioning human activities, specific aspects such as vegetation restoration, agricultural technological advancements, transportation expansion, and urban sprawl are not explicitly considered (Liu et al., 2016). Quantitatively distinguishing the specific impacts and differences of human activities requires further research.
Due to variations in how vegetation growth responds to different influencing factors in different regions, these issues and limitations are difficult to resolve in large-scale studies, leading to some degree of uncertainty in the research results. Refining the driving factors of vegetation change, determining the relationship between each factor and vegetation change, and evaluating the accuracy of the results through field investigations will help reduce such uncertainties. Overall, further research is needed to better understand the effects of human activities and climate change on vegetation NPP changes and their driving mechanisms in the Beijing-Tianjin-Hebei region.
5 Conclusion
From 2000 to 2020, the annual average NPP in the Beijing-Tianjin-Hebei region showed an overall increasing trend, indicating an improvement in the ecosystem productivity of the area. The growth rates of NPP varied across different vegetation types, with the most significant increase observed in forests, reflecting effective forest ecosystem restoration. Spatially, NPP changes in the Beijing-Tianjin-Hebei region exhibited heterogeneity, with areas showing significant increases far outweighing those with decreases, suggesting a gradual enhancement of overall ecological quality. Analysis showed that vegetation NPP in the region is highly dependent on precipitation, which plays a critical role in promoting vegetation growth, while increased solar radiation also provides favorable conditions for photosynthesis. Overall, vegetation improvement in the Beijing-Tianjin-Hebei area was driven mainly by the combined impact of climatic factors and human activities, while vegetation degradation was primarily influenced by human factors. These findings highlight the pivotal role of human activities in regional ecological construction and provide a scientific basis for sustainable ecological management in the area.
Data availability statement
The original contributions presented in the study are included in the article/supplementary material, further inquiries can be directed to the corresponding author.
Author contributions
YZ: Conceptualization, Methodology, Writing–original draft. XQ: Data curation, Writing–review and editing.
Funding
The author(s) declare that financial support was received for the research, authorship, and/or publication of this article. This article is supported by the Hebei Natural Resources Projects (13000022P00EEC410094X, 13000024P00EEC410253A).
Acknowledgments
Thanks for the support of the leaders of the Land Resources Exploration Center of the Bureau of Geology and Mineral Resources Exploration and Development of Hebei Province.
Conflict of interest
The authors declare that the research was conducted in the absence of any commercial or financial relationships that could be construed as a potential conflict of interest.
Generative AI statement
The author(s) declare that no Generative AI was used in the creation of this manuscript.
Publisher’s note
All claims expressed in this article are solely those of the authors and do not necessarily represent those of their affiliated organizations, or those of the publisher, the editors and the reviewers. Any product that may be evaluated in this article, or claim that may be made by its manufacturer, is not guaranteed or endorsed by the publisher.
References
Bai, X. Y., Zhang, S. R., Li, C. J., Xiong, L., Song, F. J., Du, C. C., et al. (2023). A carbon-neutrality-capacity index for evaluating carbon sink contributions. Environ. Sci. Ecotechnology 15, 100237. doi:10.1016/j.ese.2023.100237
Beltrán-Sanz, N., Raggio, J., Gonzalez, S., Dal Grande, F., Prost, S., Green, A., et al. (2022). Climate change leads to higher NPP at the end of the century in the Antarctic Tundra: response patterns through the lens of lichens. Sci. Total Environ. 835, 155495. doi:10.1016/j.scitotenv.2022.155495
Brinkmann, K., Dickhoefer, U., Schlecht, E., and Buerkert, A. (2011). Quantification of aboveground rangeland productivity and anthropogenic degradation on the Arabian Peninsula using Landsat imagery and field inventory data. Remote Sens. Environ. 115, 465–474. doi:10.1016/j.rse.2010.09.016
Chang, W. J., Cong, S. X., Wang, R. R., Ding, X. D., Yu, H. L., and Huang, J. Y. (2024). Quantitative analysis of NDVI changes in Mu Us Sandy Land by climate change and human activities. Arid. Land Geogr., 1–15. doi:10.12118/j.issn.1000-6060.2024.029
Cui, H. N. (2011). The analysis on ecological effect of land use change in poverty regions surrounding Beijing and Tianjin based on converting cultivated land back to forest or grassland. Baoding: Hebei Agricultural University.
Field, C. B., Randerson, J. T., and Malmström, C. M. (1995). Global net primary production: combining ecology and remote sensing. Remote Sens. Environ. 51, 74–88. doi:10.1016/0034-4257(94)00066-v
Gao, J. B., Jiao, K. W., and Wu, S. H. (2019). Revealing the climatic impacts on spatial heterogeneity of NDVI in China during 1982-2013. Acta Geogr. Sin. 74, 534–543. doi:10.1007/s11442-019-1682-2
Gao, X. X., Yu, C. W., Zhang, J., and Zhang, J. L. (2022). Quantitative assessment on the relative influence of climatic change and human activities on net primary productivity in Beijing-Tianjin-Hebei. Chin. J. Agro-meteorology. 43, 124–136. doi:10.3969/j.issn.1000-6362.2022.02.004
Gu, J., Li, X., Huang, C. L., Zhang, X. F., and Jin, X. (2013). Simulating net primary productivity of Chinese terrestrial vegetation during 2002-2010. J. Lanzhou Univ. Nat. Sci. 49, 203–213. doi:10.13885/j.issn.0455-2059.2013.02.017
Guo, H. (2023). Spatio-temporal variation of vegetation NPP in Beijing-Tianjin-Hebei region based on multi-source remote sensing data. Shijiazhuang, China: Hebei Normal University.
Haberl, H., Plutzar, C., Erb, K. H., Gaube, V., Pollheimer, M., and Schulz, N. B. (2005). Human appropriation of net primary production as determinant of avifauna diversity in Austria. Agric. Ecosyst. Environ. 110, 119–131. doi:10.1016/j.agee.2005.03.009
Hamed, K. H., and Rao, A. R. (1998). A modified Mann-Kendall trend test for autocorrelated data. J. Hydrology 204, 182–196. doi:10.1016/s0022-1694(97)00125-x
Hong, X. Q., Huang, Y., and Sun, T. (2021). Spatiotemporal evolution of vegetation net primary productivity in the karst region of southwest China from 2001 to 2018. Acta Ecol. Sin. 41, 9836–9846. doi:10.5846/stxb202009122381
Li, C. H., Zhao, J., Shi, Y. F., and Hu, X. F. (2016). The impact of human activities on net primary productivity based on the coefficient of variation: a case study of the Shiyang River Basin. Acta Ecol. Sin. 36, 4034–4044. doi:10.5846/stxb201411202301
Li, X. G., Liu, H. M., Wang, L. X., and Zhuo, Y. (2014). Vegetation cover change and its relationship between climate and human activities in Ordos plateau. Chin. J. Agrometeorology 35, 470–476. doi:10.3969/j.issn.1000-6362.2014.04.017
Liu, G. (2008). Estimation of forest net primary production of Jing-Jin-Ji region based on remote sensing. Beijing, China: Beijing Forestry University.
Liu, X. F., Zhu, X. F., Pan, Y. Z., Li, S. S., Ma, Y. Q., and Nie, J. (2016). Vegetation dynamics in Qinling-Daba Mountains in relation to climate factors between 2000 and 2014. J. Geogr. Sci. 26, 45–58. doi:10.1007/s11442-016-1253-8
Liu, Y., Li, Y., Li, S., and Motesharrei, S. (2015). Spatial and temporal patterns of global NDVI trends: correlations with climate and human factors. Remote Sens. 7, 13233–13250. doi:10.3390/rs71013233
Liu, Y. Y., Wang, Q., Zhang, Z. Y., Tong, L. J., Wang, Z. Q., and Li, J. L. (2019). Grassland dynamics in responses to climate variation and human activities in China from 2000 to 2013. Sci. Total Environ. 690, 27–39. doi:10.1016/j.scitotenv.2019.06.503
Liu, Y. Y., Zhang, Z. Y., Tong, L. J., Kjalifa, M., Wang, Q., Gang, C. C., et al. (2019). Assessing the effects of climate variation and human activities on grassland degradation and restoration across the globe. Ecol. Indic. 106, 105504. doi:10.1016/j.ecolind.2019.105504
Lv, G. X., Chen, Y. M., Zou, C. X., Feng, C. Y., and Hao, F. F. (2017). Spatial pattern and driving factors of vegetation degradation in Beijing-Tianjin-Hebei. J. Ecol. Rural Environ. 33, 417–425. doi:10.11934/j.issn.1673-4831.2017.05.005
Mou, Y. H., Huang, Y. Z., Liang, R., and Wei, C. G. (2023). Analysis of vegetation covering and landscape pattern in Wenshan based on MODIS NDVI, 38. Journal of Northwest Forestry University, 174–180+272. doi:10.3969/j.issn.1001-7461.2023.01.24
Peng, J., Liu, Z. H., Liu, Y. H., Wu, J. S., and Han, Y. N. (2012). Trend analysis of vegetation dynamics in Qinghai-tibet Plateau using hurst exponent. Ecol. Indic. 14, 28–39. doi:10.1016/j.ecolind.2011.08.011
Potter, C. S., Randerson, J. T., Field, C. B., Matson, P. A., Vitousek, P. M., Mooney, H. A., et al. (1993). Terrestrial ecosystem production:a process model based on global satellite and surface data. Glob. Biogeochem. Cycles 7, 811–841. doi:10.1029/93gb02725
Ren, Y. M., Liu, X. P., Xu, X. C., Sun, S. S., Zhao, L. F., Liang, X., et al. (2023). Multi-scenario simulation of land use change and its impact on ecosystem services in Beijing-Tianjin-Hebei region based on the FLUS-InVEST Model. Acta Ecol. Sin. 43, 4473–4487. doi:10.5846/stxb202201280269
Running, S. W., Nemani, R. R., Heinsch, F. A., Zhao, M., Reeves, M., and Hashimoto, H. (2004). A continuous satellite-derived measure of global terrestrial primary production. Bio-Science 54, 547–560. doi:10.1641/0006-3568(2004)054[0547:acsmog]2.0.co;2
Sun, R., Chen, S. H., and Su, H. B. (2019). Spatiotemporal variations of NDVI of different land cover types on the Loess Plateau from 2000 to 2016. Prog. Geogr. 38, 1248–1258. doi:10.18306/dlkxjz.2019.08.013
Thiel, H. (1950). A rank-invariant method of linear and polynomial regression analysis,I,II,III. Proc. Koninalike Nederl. Akademie van Weinenschatpen 53, 1397–1412.
Tong, L. J., Liu, Y. Y., Wang, Q., Zhang, Z. Y., Li, J., Sun, Z. G., et al. (2019). Relative effects of climate variation and human activities on grassland dynamics in Africa from 2000 to 2015. Ecol. Inf. 53, 100979. doi:10.1016/j.ecoinf.2019.100979
Tong, L. J., Liu, Y. Y., Zhang, Z. Y., Li, X. Y., Wang, Q., and Li, J. L. (2020). Quantitative assessment on the relative effects of climate variation and human activities on grassland dynamics in Northwest China. Res. Soil Water Conservation 27, 2020–2210. doi:10.13869/j.cnki.rswc.2020.06.028
Wang, J. J., Zhao, A. Z., Zhang, Z. J., Zhang, A. B., and Hu, X. F. (2021). Spatio-temporal evolution of vegetation net primary productivity and its driving factors in Beijing-Tianjin-Hebei region from 2000 to 2018. Ecol. Sci. 40 (1), 103–111. doi:10.14108/j.cnki.1008-8873.2021.01.014
Wang, L. Y. (2012). Net primary productivity estimation of vegetation in Hebei province using remote sensing. Shijiazhuang, China: Hebei Normal University.
Wessels, K. J., Bergh, F., and Scholes, R. J. (2012). Limits to detectability of land degradation by trend analysis of vegetation index data. Remote Sens. Environ. 125, 10–22. doi:10.1016/j.rse.2012.06.022
Wu, S., Tian, B., Gu, S. J., and Hu, Y. C. (2023). Spatial-temporal evolution of ecological vulnerability in Beijing-Tianjin-Hebei region based on PSRM model. Environ. Sci. 44, 5630–5640. doi:10.13227/j.hjkx.202210269
Xiao, X. M., Hollinger, D., Aber, J., Goltz, M., Davidson, E. A., Zhang, Q. Y., et al. (2004). Satellite-based modeling of gross primary production in an evergreen needle leaf forest. Remote Sens. Environ. 89, 519–534. doi:10.1016/j.rse.2003.11.008
Xie, B. N., Qin, Z. F., Wang, Y., and Chang, Q. R. (2014). Spatial and temporal variation in terrestrial net primary productivity on Chinese Loess Plateau and its influential factors. Trans. Chin. Soc. Agric. Eng. 30, 244–253. doi:10.3969/j.issn.1002-6819.2014.11.030
Xin, H. C., Guo, W., and Wang, H. F. (2024). Time series dynamic assessment of ecological environment quality in Beijing-Tianjin-Hebei region basedon GEE and RSEI. J. Northwest For. Univ. 39, 106–114. doi:10.3969/j.issn.1001-7461.2024.02.14
Xu, M. X., Li, J., Yao, L., and Wu, Q. Y. (2021). Analysis of the relationship between surface thermal environment and landscape change in Beijing-Tianjin-Hebei region under multiple scales. J. Xi’an Univ. Technol. 37, 43–52. doi:10.19322/j.cnki.issn.1006-4710.2021.01.006
Yan, J. X., Huang, H., Gao, Y. H., Wang, T. T., and Zhang, Y. (2021). Estimation and spatial-temporal dynamics of Long-term sequenced vegetation net primary productivity in Jilin province. J. Soil Water conservation 35, 172–180. doi:10.13870/j.cnki.stbcxb.2021.05.024
Yang, A. L., Zhang, X. P., Li, Z. X., Li, Y. C., and Nan, F. S. (2023). Quantitative analysis of the impacts of climate change and human activities on vegetation NPP on the Qilian Mountain National Park. Acta Ecol. Sin. 43, 1784–1792. doi:10.5846/stxb202202050295
Yang, H. F., Mu, S. J., and Li, J. L. (2014). Effects of ecological restoration projects on land use and land cover change and its influences on territorial NPP in Xinjiang, China. CATENA 115, 85–95. doi:10.1016/j.catena.2013.11.020
Yang, J., and Huang, X. (2021). The 30 m annual land cover dataset and its dynamics in China from 1990 to 2019. Earth Syst. Sci. Data 13 (8), 3907–3925. doi:10.5194/essd-13-3907-2021
Yin, Q. D., Liu, C. X., and Tian, Y. (2021). Spatio-temporal greenness and anthropogenic analysis in Shaanxi based on MODIS NDVI from 2001 to 2018. Acta Ecol. Sin. 41, 1571–1582. doi:10.5846/stxb201906291372
Yin, X. J., Zhu, H. H., Gao, G., Gao, J., Guo, L. J., and Wang, J. J. (2020). Effects of climate change and human activities on net primary productivity in the northern slope of Tianshan,Xinjing,China. Trans. CSAE 36, 195–202. doi:10.11975/j.issn.1002-6819.2020.20.023
Zhang, B. L., Jia, R. C., Liu, G. B., Xue, S., and Guo, T. Y. (2016). Remote sensor analysis of vegetation restoration in Green-for-grain project areas of Inner Mongolia. Environ. Sci. and Technol. 39, 187–193. doi:10.3969/j.issn.1003-6504.2016.01.030
Zhang, B. Q., Wu, P. T., and Zhao, X. N. (2011). Detecting and analysis of spatial and temporal variation of vegetation cover in the Loess Plateau during 1982-2009. Trans. CSAE 27, 287–293. doi:10.3969/j.issn.1002-6819.2011.04.051
Zhang, Q., Singh, V. P., Peng, J., Chen, Y. D., and Li, J. (2012). Spatial-temporal changes of precipitation structure across the Pearl River basin, China. J. Hydrology 440-441, 113–122. doi:10.1016/j.jhydrol.2012.03.037
Zhang, S. (2015). Research on spatio-temporal distribution of vegetation net primary productivity in Hebei province based on long time-series muti-source remote sensing data. Shijiazhuang: Hebei Normal University.
Zhang, X. R., Cao, Q., Ji, S. P., Chen, H., Zhang, T. J., and Liu, J. (2022). Quantifying the contributions of climate change and human activities to vegetation dynamic changes in the Yellow River Delta. Acta Sci. Circumstantiae 42 (1), 56–69. doi:10.13671/j.hjkxxb.2021.0492
Zhang, X. Y., Feng, Z. K., Zhang, X. L. F., and Fan, Z. X. (2018). Study on the relationship between net primary productivity and climate factors——a case study of Jing-Jin-Ji region. J. Central South Univ. For. and Technol. 38, 97–102. doi:10.14067/j.cnki.1673-923x.2018.08.015
Zhao, J. H., Zhou, H. R., Lu, Y. Y., and Sun, Q. X. (2020). Temporal-spatial characteristics and influencing factors of vegetation net primary production in the National Nature Reserve of Populus euphratica in Tarim from 2000 to 2015. Arid. land Geogr. 43, 190–200. doi:10.12118/j.issn.1000-6060.2020.01.22
Zhou, W., Gang, C. C., Zhou, F. C., Li, J. L., Dong, X. G., and Zhao, C. Z. (2015). Quantitative assessment of the individual contribution of climate and human factors to desertification in northwest China using net primary productivity as an indicator. Ecol. Indic. 48, 560–569. doi:10.1016/j.ecolind.2014.08.043
Zhou, W., Mou, F. Y., Gang, C. C., Guan, D. J., He, J. F., and Li, J. L. (2017). Spatio-temporal dynamics of grassland net primary productivity and their relationship with climatic factors from 1982 to 2010 in China. Acta Ecol. Sin. 37, 4335–4345. doi:10.5846/stxb201408291724
Zhou, Y. Y., Zhu, M. X., Guo, X. J., Li, K., Miao, J. X., Guo, J. J., et al. (2019). Relative effects of climate change and human activities on net primary productivity in Shule River Basin. Acta Ecol. Sin. 39, 5127–5137. doi:10.5846/stxb201809141984
Zhu, S. H., Yan, Y., Shao, H., and Li, C. F. (2017). The responses of the net primary productivity of the dryland ecosystems in central Asia to the CO2 and climate changes during the past 35 Years. J. Nat. Resour. 32, 1844–1856. doi:10.11849/zrzyxb.20160983
Zhu, W. Q., Pan, Y. Z., and Zhang, J. S. (2007). Estimation of net primary productivity of Chinese terrestrial vegetation based on remote sensing. J. Plant Ecol. 31, 413–424. doi:10.17521/cjpe.2007.0050
Keywords: climate change, human activities, vegetation NPP, Beijing-Tianjin-Hebei region, residual method
Citation: Zhao Y and Qie X (2024) Quantitative assessment of the relative impacts of climate change and human activities on net primary productivity of vegetation in the Beijing-Tianjin-Hebei region. Front. Environ. Sci. 12:1508433. doi: 10.3389/fenvs.2024.1508433
Received: 14 October 2024; Accepted: 18 November 2024;
Published: 05 December 2024.
Edited by:
Changchun Huang, Nanjing Normal University, ChinaReviewed by:
Xiaoyan Song, Northwest A and F University, ChinaZhenglei Xie, Nanjing Normal University, China
Copyright © 2024 Zhao and Qie. This is an open-access article distributed under the terms of the Creative Commons Attribution License (CC BY). The use, distribution or reproduction in other forums is permitted, provided the original author(s) and the copyright owner(s) are credited and that the original publication in this journal is cited, in accordance with accepted academic practice. No use, distribution or reproduction is permitted which does not comply with these terms.
*Correspondence: Xin Qie, MTEwMzQ1NjY1MUBxcS5jb20=