- 1Xingzhi College, Zhejiang Normal University, Jinhua, China
- 2School of Management, Zhejiang University of Technology, Hangzhou, China
- 3College of Economics and Management, Zhejiang Normal University, Jinhua, China
Improving urban land use eco-efficiency (ULUEE) is of great significance for promoting high-quality economic development and promoting the modernization of harmonious coexistence between humans and nature. In this study, the super efficiency SBM model with undesirable output was used to measure the level of ULUEE at the county scale in Zhejiang province from 2006 to 2022. Based on this, the spatial-temporal evolution and spatial agglomeration characteristics were analyzed by using spatial analysis techniques, kernel density analysis, and spatial autocorrelation model. Finally, the driving mechanisms were revealed by using the geographical detector model and GWR model. The results were as follows: (1) From 2006 to 2022, the ULUEE of Zhejiang province rose from 0.34 to 0.73, with an average annual growth rate of 2.44%. The degree of efficiency differences between counties gradually converged. (2) The ULUEE at the county level exhibited a significant spatial positive correlation, with Moran’s I index increasing from 0.3219 to 0.3715. On the local scale, the cold spot significant area was mainly distributed in the north and south of Zhejiang province, and significant spatial and temporal variations were observed within the hot spot significant area. (3) The results of factor detection showed that altitude (X1), topographic relief (X2), and forest cover (X3) always played a strong role in affecting ULUEE. Among the socioeconomic factors, foreign trade (X8) had the strongest explanatory power in the early period, and GDP per capita (X5) and industrial structure (X6) played the strongest role in the later period. The explanatory power of all influencing factors decreased over time. (4) At the local scale, GDP per capita (X5), industrial structure (X6), and fiscal expenditure scale (X7) presented positive effects on ULUEE, and development vitality (X9) presented a negative effect. Future endeavors should encompass a multifaceted approach, which includes the facilitation of industrial modernization and the enhancement of external economic engagement. Concurrently, it is imperative to capitalize on the region’s inherent economic strengths and to foster a low-carbon, environmentally sustainable economic model.
1 Introduction
Human activities have now warmed global temperatures by an average of 1.1°C, CO2 concentrations are at their maximum in nearly 2 million years, and all regions of the world are facing unprecedented changes in the climate system (Agnew, 2024). The high incidence of extreme weather is leading to excess deaths in the population (Lou et al., 2023), melting glaciers, and rising sea levels (Nerem et al., 2018). To promote sustainable development, scientific regulation of greenhouse gas emissions and slowing down the pace of global warming have become important issues facing the world today. China is the largest carbon emitter in the world since 2016. As a responsible country, China proposed to achieve the goal of carbon peaking in 2030 and carbon neutrality in 2060. In addition, China has accelerated ecological civilization construction, proposing an ambitious blueprint for building a beautiful China since 2012. A series of significant measures have been implemented to reduce pollutant emissions, protect ecological environments, and enhance ecosystem service levels, yielding substantial achievements. In 2022, China put forward the modernization road of harmonious coexistence of humans and nature, which means abandoning the unsophisticated model and enhancing the quality of economic and social systems. Land is the spatial carrier of socioeconomic activities and an important carbon source, enhancing ULUEE can facilitate high-quality socioeconomic development and address resource and environmental challenges. However, since the reform and opening up, China’s rapid industrialization and urbanization have led to the continuous and disorderly expansion of urban land. Numerous farmlands, forests, and wetlands have experienced encroachment, leading to the disruption of the functionality of territorial ecosystems (Liu et al., 2014). Simultaneously, the issue of urban land idleness is rather pronounced, and inefficient land use significantly hinders sustainable development. Consequently, achieving ecological land use has become imperative for China’s sustainable development.
Extensive research has been conducted on ULUEE. In terms of research contents, scholars primarily focus on the measurement (Lu et al., 2020; Liu et al., 2021), spatiotemporal changes, and convergence characteristics of ULUEE (Ma D. L. et al., 2023; Yu et al., 2019; Yang and Wu, 2019). Scholars have identified various factors influencing ULUEE, such as land use management policy (Den and Gibson, 2020), land urbanization (Tang et al., 2020), land marketization (Yu and Luo, 2023), population urbanization (Zhou Y. et al., 2020), environmental regulation (Yang et al., 2019), urban shrinkage (Sun and Zhou, 2023),new-type urbanization (Zhao et al., 2018), urban agglomerations (Huang et al., 2018), technological innovations (Chen et al., 2020). Additionally, some scholars have investigated agricultural land use eco-efficiency (Hu et al., 2022). In terms of research scale, studies on urban land eco-efficiency primarily concentrate on the national level (Zhou L. et al., 2020; Liu et al., 2022; Fan and Jiang, 2023), urban agglomerations (Ge et al., 2021; Ge et al., 2023; Hong and Mao, 2023), river basins (Chen et al., 2022; Cui et al., 2023; Li et al., 2023; Ma D. L. et al., 2023), provincial areas (Liu et al., 2019; Zhang and Lu, 2022), and prefecture-level (Huntington et al., 2021). In terms of research methods, the data envelopment analysis (DEA) (Cook and Seiford, 2009), stochastic frontier approach (SFA) (Liu et al., 2020), slacks-based measure model (SBM) (Fan and Jiang, 2023), epsilon-based measure model (EBM) (Cui et al., 2023), Super-EBM model (Li et al., 2023; Ma D. L. et al., 2023; Ma et al., 2024), Malmquist index method (Zhao et al., 2018) were mainly utilized. To analyze the driving mechanism of influencing factors, the Tobit regression model (Chen et al., 2022), geographically weighted regression model (GWR) (Huntington et al., 2021), geographically and temporally weighted regression model (GTWR) (Ge et al., 2023; Li et al., 2023), fixed effects model (Zhou et al., 2018; Hong and Mao, 2023) were mostly used.
The existing studies have the following deficiencies. (1) The county serves as the basic unit of China’s national governance and is the primary financial unit, spatial unit, and industrial carrier for the country’s socioeconomic development. Enhancing county-level ULUEE can positively contribute to green and sustainable development, but relevant research is insufficient. (2) At the measurement level of ULUEE, the targeted evaluation index system for the national dual-carbon goal and ecological civilization construction is relatively lacking, and there is an urgent need to incorporate undesirable outputs such as PM2.5, CO2, SO2, etc., (3) The traditional efficiency measurement model cannot differentiate the disparity between the research units when the efficiency value is over 1, and the super-efficiency SBM model can overcome this deficiency (Li and Shi, 2014), which can better reveal the differences in ULUEE among counties.
Zhejiang Province is a demonstration area of the common wealth and a pioneer area of ecological civilization construction in China, which has achieved outstanding results in socio-economic development for many years. However, the development mode of high energy consumption, high pollution, and low income still exists in some areas. Exploring the driving mechanism of ULUEE at the county level and putting forward targeted suggestions for improvement will help to support the national strategic goal of high-quality development. Therefore, this study adopts the super-efficient SBM model approach considering undesirable outputs to measure ULUEE at the county level in Zhejiang province from 2006 to 2022 and reveals its spatial clustering characteristics by using GIS spatial analysis. In addition, the geodetector model and GWR model are utilized to analyze the driving mechanisms at global and local scales and finally put forward targeted countermeasure suggestions.
2 Study area and data sources
2.1 Study area
Zhejiang Province is located in the south of China’s Yangtze River Delta urban agglomeration, with a land area of 105,500 km2. The topography of Zhejiang Province exhibits a stepped inclination from southwest to northeast, characterized by a distinctive landscape progression. The southwestern region is predominantly mountainous, transitioning to hilly terrain in the central area, and culminating in low-lying alluvial plains in the northeastern sector (Figure 1). In 2022, the GDP of Zhejiang province was 7,771.5 billion yuan, ranking fourth in the country. The permanent population is 65.77 million, and the urbanization rate is 73.4%. The air conditions in Zhejiang Province have continued to improve in recent years, with an average annual concentration of PM2.5 of 39 μg/m3 in 2022. Zhejiang Province comprises 90 districts and counties. Among them, Hangzhou boasts a highly developed digital economy and a concentration of high-tech industries, Taizhou and Ningbo cluster High-end manufacturing industries, and Lishui forms a highly effective eco-industrialization model. Conversely, Jiaxing and Wenzhou encounter challenges related to industrial upgrading and idle land use. As a leading demonstration area for ecological civilization construction and shared prosperity in China, Zhejiang Province strives to improve the ULUEE to promote coordinated development of efficient resource utilization, ecological and environmental protection, and high-quality economic development.
2.2 Research methodology
2.2.1 Super-SBM model
The traditional DEA method cannot incorporate undesirable output to evaluate the efficiency of DMU. Therefore, this study used the super-SBM model, which can consider the undesirable output and distinguish the units when the efficiency value equal to 1 (Huang et al., 2021). Assume there are j = 1, 2, 3 … … N DMUs. Each of them utilizes an input vector
which
Subject to:
where
2.2.2 Spatial autocorrelation model
Spatial autocorrelation measures the potential interdependence between the same spatial attribute values, including global and local autocorrelation. Global spatial autocorrelation is used to judge whether attribute values are spatially clustered. Still, it cannot accurately measure the specific cluster region, so it is necessary to use local spatial autocorrelation to determine the cluster region (Ye et al., 2018). The formula is as Equation 4:
where i and j denote different cities,
where n denotes the number of counties,
2.2.3 Kernel density analysis
Kernel density analysis is an essential non-parametric estimation method to study data distribution characteristics. This method does not require the prior information of the model and can describe the distribution form of random variables by estimating their continuous density curves, thus possessing robustness (Liu et al., 2023).
In this paper, the Gaussian kernel function is used to construct kernel function K(x). The estimation formulas are shown in Equations 6, 7. f(x) is the density function of random variable X. N, Xi, and h denote the number of observations, independent and identically distributed observations, and broadband, respectively.
2.2.4 Geodetector model
Geodetector is used to detect spatial differentiation and reveal the driving forces behind it, including factor detection, interactive detection, and ecological detection (Wang et al., 2010). The calculation formula for factor detection is shown as Equations 8, 9:
where h = 1, … , L is the strata of variable Y or factor X, that is, classification or partition, Nh and N are the number of units in layer h and the whole region, respectively. Moreover,
The interaction detector is used to identify the interaction between different risk factors Xs, that is, to assess whether the cooperation of factors X1 and X2 will increase or decrease the explanatory power of the dependent variable Y or whether the effects of these factors on Y are independent of each other. Interactions are divided into five types: nonlinear-weaken, uni-weaken, bi-enhance, independent, and nonlinear-enhance.
2.2.5 GWR model
The GWR model is a spatial analysis technique that replaces global parameter estimation with local parameter estimation during regression calculations, which can reflect the non-stationarity of parameters in different spaces so that the relationship between variables can vary with spatial location. Therefore, it can be used to explore the spatial heterogeneity of the mechanism of action of the influencing factors (Cao et al., 2019). The formula is as Equation 10:
where
2.3 Index system construction
2.3.1 Evaluation index system of county-level ULUEE
In this study, we selected urban land, labor, and capital as input indicators. Specifically, county urban construction land, urban employment in secondary and tertiary industries, and fixed investment in secondary and tertiary sectors are input variables (Sun and Zhou, 2023). The desirable output index considers economic, social, and ecological aspects. The GDP of secondary and tertiary industries and financial budget revenue are selected as representative indicators for economic output (Liu et al., 2021). The disposable income of urban residents is used as social output, while ecological production is represented by NDVI(Liu et al., 2021). PM2.5, CO2, and SO2 are selected as the main undesirable output indicators (Zhao et al., 2018; Ma L. D. et al., 2023), considering the objective phenomenon of environmental pollution caused by land use (Table 1).
2.3.2 Index system of influencing factors of county-level ULUEE in Zhejiang province
Previous research has demonstrated that the ULUEE was influenced by natural geographical conditions and socioeconomic development. Considering the mountainous natural environment in Zhejiang province and referring to the relevant research, we have selected elevation (X1) (Cao et al., 2019; Li et al., 2024), topographic relief (X2) (Yu and Liu, 2024), forest coverage rate (X3) (Zhu et al., 2019; Xue et al., 2023) and precipitation (X4) (Li et al., 2024) as indicators at the physical geography level. GDP per capita (X5) (Zhou et al., 2018; Cao et al., 2019; Sun and Wang, 2022), industrial structure (X6) (Bai et al., 2018; Sun and Wang, 2022), fiscal expenditure scale (X7) (Zhu et al., 2019; Xue et al., 2023), foreign trade (X8) (Zhou et al., 2018; Ma M. et al., 2023) and development vitality (X9) (Li et al., 2024) were selected as indicators at the socio-economic level (Table 2).
2.4 Data resources
The data sources utilized in this research are detailed in Tables 1, 2. Missing data were addressed through imputation methods. The NDVI dataset was derived from MODIS 16-day 250 m continuous time-series NDVI and EVI data products and was generated using the Maximum Value Composite (MVC) method. Artificial intelligence techniques were employed to produce CO2, SO2, and PM2.5 measurements from various big data sources, including ground-based observations, satellite remote sensing products, atmospheric reanalysis, and model simulation data. The Chinese land use data was primarily interpreted using Landsat 8 OLI satellite imagery with 30 m resolution spectral bands, supplemented by GF-2 satellite imagery. The interpretation was conducted using an object-oriented, human-computer interactive land-use interpretation platform, maintaining a 30 m spatial resolution. Topographic relief is calculated as the product of the elevation difference between the highest and lowest points in a region and the area of non-flat terrain, representing regional topographic characteristics (Feng et al., 2008). This metric was derived from DEM data using GIS spatial analysis methods. The nighttime light index was computed using an autoencoder-based cross-sensor approach, integrating data from DMSP-OLS and NPP-VIIRS sensors. To ensure spatial consistency across all datasets, preprocessing steps including clipping, projection, resampling, and normalization were performed. All data were projected to the WGS84_Albers coordinate system with a standardized 1,000 m grid resolution.
3 Results and analysis
3.1 Spatial-temporal changes of ULUEE in Zhejiang province
3.1.1 Temporal evolution analysis
As shown in Figure 2, the ULUEE of Zhejiang Province increased from 0.34 in 2006 to 0.73 in 2022, with an average annual growth rate of 2.44%. To further investigate the temporal evolution of ULUEE, kernel density estimation was performed (Figure 3). From 2006 to 2022, the kernel density curves demonstrated a progressive rightward shift, indicating a gradual improvement in Zhejiang Province’s ULUEE levels. The continuous decline in the primary peak amplitude suggests a reduction in absolute efficiency disparities among counties. The curves exhibit pronounced right-skewed tailing with expanding distributional spread, indicating that counties with higher ULUEE showed an upward trend, with their deviation from the provincial average increasing year by year. From 2006 to 2022, the kernel density curves consistently exhibited a double-peak pattern, indicating a polarization in county-level ULUEE across Zhejiang Province. However, the primary peak gradually decreased in height, while the secondary peak steadily increased, suggesting that the disparities in ULUEE among counties in Zhejiang Province have progressively diminished.
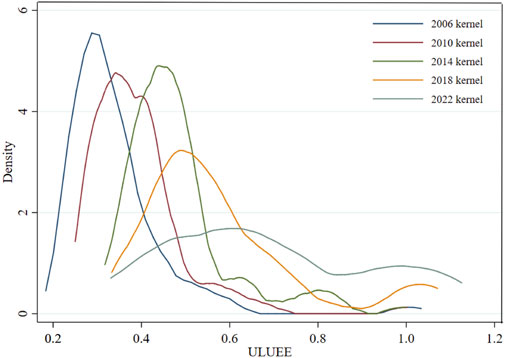
Figure 3. Kernel density curve changes of county-level ULUEE in Zhejiang province from 2006 to 2022.
At the municipal level, the ULUEE demonstrated an increasing trend across all cities over time (Figure 4). Zhoushan, Ningbo, and Hangzhou exhibited the most rapid growth rates. Notably, Zhoushan’s ULUEE value increased from 0.33 in 2006 to 1.04 in 2022, representing a remarkable growth rate of 213%, making it the only municipality to exceed a value of 1.0 in 2022. Lishui, benefiting from its abundant natural resources of mountains and waters, led the municipal ULUEE rankings in 2006 and 2010 with efficiency values of 0.47 and 0.53, respectively. Zhoushan, leveraging its advantageous marine resource endowment and successful ecological industrialization model, has maintained the leading position in ULUEE since 2014. Its efficiency value progressively increased from 0.76 in 2014 to 1.04 in 2022, significantly outperforming other municipalities. In contrast, Jiaxing consistently recorded the lowest efficiency levels during the study period, with ULUEE values rising modestly from 0.25 in 2006 to 0.43 in 2022. This relatively poor performance can be attributed to its dominant textile and garment industry, which ranks as the second most polluting sector after the petroleum industry, characterized by high water and energy consumption. To address these challenges, Jiaxing needs to continue advancing industrial structure upgrades, promote ecological transformation of industries, optimize urban land use structure, and facilitate high-quality economic and social development.
3.1.2 Spatial evolution analysis
As time progressed, a distinct upward trend in efficiency values was observed across counties (Figure 5). This temporal progression demonstrates a systematic improvement in land resource utilization across the province’s administrative regions. We divided ULUEE into five categories: low-value zone (0.2–0.4), lower-middle value zone (0.4–0.6), middle-value zone (0.6–0.8), upper-middle value zone (0.8–1.0), and high-value zone (1.0–1.2).
In 2006, 74 counties (82.2%) were in the low-value zone, 15 counties (16.7%) in the lower-middle zone, and only Chun’an County reached the high-value zone. By 2010, the number of counties in the low-value zone decreased to 57 (63.3%), while those in the lower-middle zone increased to 29 (32.2%). Three counties were classified in the middle-value zone, with Chun’an County remaining the sole representative of the high-value zone. A dramatic shift occurred in 2014, with only 15 counties (16.7%) remaining in the low-value zone, while 62 counties (68.9%) moved to the lower-middle zone. Eight counties (8.9%) occupied the middle-value zone, and five counties reached the high-value zone, including the newly added districts of Yuecheng, Dinghai, Putuo, and Haishu, alongside Chun’an County. The 2018 data revealed further evolution, with only two counties remaining in the low-value zone. The lower-middle zone contained 53 counties (58.9%), while the middle-value zone experienced substantial growth to 23 counties (25.6%). Notably, four counties emerged in the upper-middle zone, and eight counties achieved high-value status, including new additions such as Beilun District, Yiwu City, Yuhang District, and Wenling City. By 2022, Zhejiang province demonstrated significant advancement in ULUEE, driven by enhanced green development and ecological civilization initiatives. The distribution pattern showed greater differentiation, with most counties concentrated in the middle and high-value zones. Counties with ULUEE exceeding 1.0 in 2022 were predominantly clustered in Zhoushan, Lishui, and Hangzhou cities.
3.2 Spatial agglomeration characteristics of ULUEE in Zhejiang province
3.2.1 Global autocorrelation analysis
The global Moran’s I for ULUEE across Zhejiang Province’s counties was calculated using ArcGIS 10.6. Results revealed that the global Moran’s I exhibited a fluctuating upward trend from 2006 to 2022, with all values achieving statistical significance at the 1% level (Table 3). This pattern indicates significant positive spatial autocorrelation in ULUEE at the county level. From 2006 to 2014, the Moran’s I index decreased from 0.3219 to 0.2316, indicating a reduction in spatial autocorrelation. However, from 2018 to 2022, the index increased substantially to 0.3715, demonstrating enhanced spatial clustering and club convergence characteristics.
3.2.2 Local autocorrelation analysis
From 2006 to 2018, the number of significant cold spots for ULUEE decreased from 17 to 9. However, by 2022, the number increased to 14. Notably, the counties of Nanxun in Huzhou City and Haiyan, Jiashan, Nanhu, Pinghu, and Xiuzhou in Jiaxing City have consistently remained within the significant cold spot category. The persistent presence of these cold spots can be attributed to Jiaxing’s failure to timely upgrade its industrial structure in light of ongoing market economic developments, alongside the notable inefficiencies in land use. In terms of spatial distribution, significant cold spots have gradually evolved from being concentrated in the northern part of Zhejiang Province to coexisting in both the northern and southern regions. The north has exhibited a trend of continuous contraction, while the south has shown a pattern of initial contraction followed by subsequent expansion. From 2006 to 2022, the number of significant hot spots across the counties remained relatively stable. However, their spatial distribution underwent marked changes. The distribution shifted from being mainly concentrated in the cities of Hangzhou, Lishui, and Quzhou in the western and southwestern regions of Zhejiang to a gradual contraction and relocation towards Ningbo and Zhoushan in the northeastern region. Hangzhou has consistently maintained its status as a significant hot spot due to its advantages in the digital economy and high-tech industry agglomeration. Moreover, with advancements in economic and social development, Zhoushan and Ningbo have effectively leveraged their marine resource endowments for rapid transformation and growth, thus becoming significant hot spots as well (Figure 6).
In 2006, significant cold spots were predominantly concentrated in 17 counties across northern Zhejiang Province, encompassing regions within Jiaxing, Hangzhou, Huzhou, and Ningbo. Notably, all seven counties in Jiaxing and six counties in Hangzhou were classified as cold spots. Conversely, significant hot spots were primarily clustered in 11 counties across western and southwestern Zhejiang, specifically within Hangzhou, Lishui, Quzhou, and Wenzhou. Among these, Lishui contained six counties within the significant hot spot zone, while Wenzhou’s representation was limited to Taishun County. By 2010, the significant cold spots had consolidated into 15 counties, with 11 in northern Zhejiang (Jiaxing, Hangzhou, and Huzhou) and four newly designated counties in southern Wenzhou. This distribution reflected a pattern of northern contraction and southern expansion. The hot spot configuration remained relatively stable, encompassing 10 counties across western and southwestern regions, with Lishui maintaining five counties in the significant hot spot category. In 2014, there were 13 significant cold spots, comprising nine counties in the north and four in Wenzhou. The northern region continued its contracting trend while the southern distribution remained stable. The significant hot spots shifted to include nine counties across western Hangzhou, southwestern Quzhou and Lishui, and northeastern Ningbo and Zhoushan, with Zhoushan contributing three counties to this classification. In 2018, cold spots were primarily concentrated in seven northern counties and two southern counties, demonstrating simultaneous contraction in both regions. The significant hot spots encompassed six counties across western Hangzhou and northern Shaoxing. Notably, Shaoxing emerged as a new hot spot through effective land stock activation, improved urban land use coordination, and promotion of intensive, green development. By 2022, significant cold spots expanded to 14 counties, distributed across northern, southern, and eastern regions. This pattern reflected stability in the north, expansion in the south, and the emergence of new eastern zones. Wenzhou contained seven cold spot counties, and Linhai City in Taizhou was newly designated as a cold spot. Significant hot spots were primarily distributed across northern Hangzhou, southern Lishui, and northeastern Ningbo and Zhoushan, with all four counties in Zhoushan classified as significant hot spots.
3.3 Driving mechanism analysis
The geodetector model was conducted to investigate the driving force of these driving factors at a global scale from 2006 to 2022. Additionally, the GWR model was employed to examine the direction and intensity of driving factors across Zhejiang Province’s 90 counties.
3.3.1 Global scale
3.3.1.1 Factor detection
From 2006 to 2022, statistical analysis revealed that a greater number of natural geographical factors demonstrated statistical significance compared to socioeconomic factors (Table 4). Over time, the q-values of all driving factors showed a declining trend, indicating a diminishing influence of these factors. Among the natural geographical factors, precipitation (X4) ceased to be significant in later periods, while elevation (X1), topographic relief (X2), and forest coverage rate (X3) maintained substantial impacts on ULUEE throughout the study period. Notably, elevation (X1) exhibited the strongest driving force, with explanatory power exceeding 0.5 in both 2006 and 2010. This pattern suggests that county-level ULUEE in Zhejiang Province is largely constrained by natural geographical conditions, with this relationship becoming more pronounced over time. Regarding socioeconomic factors, foreign trade (X8) demonstrated the strongest explanatory power in the early stages and remained the only consistently significant socioeconomic factor throughout the 5 years. As time progressed, GDP per capita (X5) and industrial structure (X6) emerged as increasingly influential socioeconomic factors with substantial explanatory power.
In 2006, all nine variables demonstrated significant influence on ULUEE. Development vitality (X9) and forest coverage rate (X3) emerged as the most influential factor, with q-values of 0.6566 and 0.5244, respectively. A high-vitality state facilitates the elimination of outdated production capacities and reduces pollutant emissions. Simultaneously, it enhances corporate efficiency through technological empowerment. These factors contribute to increasing the expected land output while mitigating negative environmental impacts. A high forest coverage rate indicates superior ecological benefits within a county. Healthy forest ecosystems not only produce and protect soil but also diminish natural disaster risks and mitigate environmental pollution. This multifaceted role of forests underscores their importance in maintaining ecological balance and promoting sustainable development.
In 2010, eight factors overall showed substantial effects on ULUEE. Among them, development vitality (X9) maintained the strongest explanatory power (q = 0.5498), followed by precipitation (X4) with a q-value of 0.5037. Development vitality emerged as the most explanatory socioeconomic factor; however, its q-value decreased compared to 2006, indicating a decline in its influence. Precipitation (X4) emerged as the most influential natural geographic factor, attributed to its role in air purification and vegetation growth promotion.
In 2014, eight factors overall showed substantial effects on ULUEE. Among them, industrial structure (X6) emerged as the most influential factor, with the highest explanatory power (q = 0.3425). This prominence can be attributed to its role in determining land-use intensity, patterns, and structural composition. Elevation (X1) ranked second in explanatory power (q = 0.2148). The diverse topography of Zhejiang Province’s counties indicates that lower-elevation regions facilitate large-scale land utilization and superior transportation accessibility, enabling more extensive resource development. This positions elevation (X1) as the most influential natural geographic factor. Fiscal expenditure scale (X7) demonstrated the lowest explanatory power (q = 0.1089).
In 2018, five factors overall showed substantial influence on ULUEE. Industrial structure (X6) and elevation (X1) exhibited notably high driving intensities, with q-values of 0.3425 and 0.2148, respectively. Among these, elevation (X1) and topographic relief (X2) emerged as the most influential natural geographical factors, with q-values of 0.2560 and 0.2349. Ranking third was industrial structure (X6), with a q-value of 0.2224, representing the most explanatory socio-economic factor.
In 2022, seven factors overall show substantial influence on ULUEE. Elevation (X1) and GDP per capita (X5) demonstrated notably high driving intensities, with q-values of 0.2550 and 0.1786, respectively. GDP per capita, representing the regional economic development level, increasingly reveals its impact on land ecological utilization efficiency as social and economic development progresses, emerging as the most influential socio-economic factor.
3.3.1.2 Interaction detection
From 2006 to 2022, the interaction detection analysis revealed several notable trends (Figure 7). First, there was an observed increase in the number of nonlinear enhancement relationships over time, accompanied by a declining trend in q-values from the interaction detection results. The five socioeconomic factors demonstrated a pronounced nonlinear enhancement trend when interacting with other factors. Among the physical geographic factors, only precipitation (X4) exhibited nonlinear enhancement patterns in its interactions with other variables. These findings indicate that socioeconomic factors exerted a substantial influence on county-level ULUEE in Zhejiang Province. Moreover, when these socioeconomic factors interacted with physical geographic factors, their explanatory power for ULUEE significantly increased. This pattern underscores that the ULIUEE at the county level in Zhejiang Province is jointly constrained by both socioeconomic and physical geographic factors.
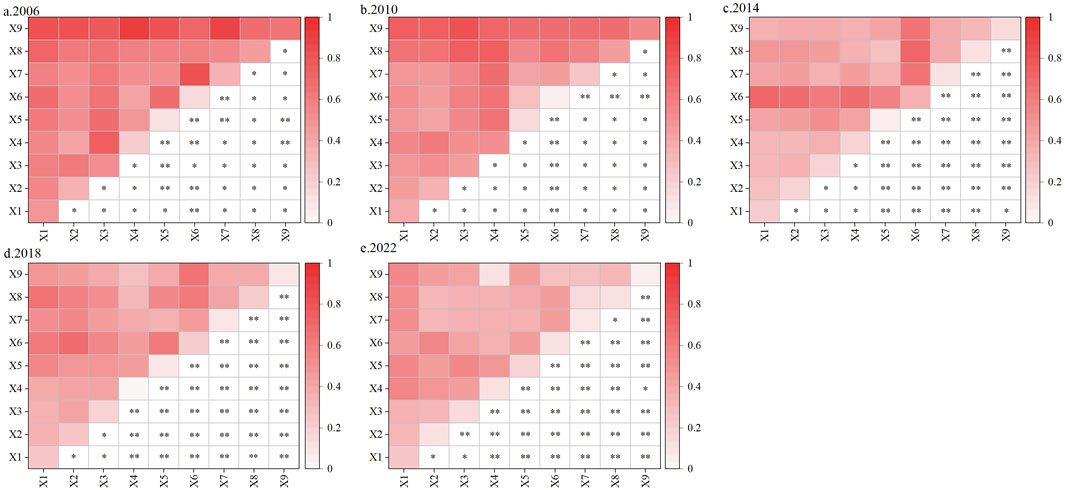
Figure 7. Interaction detection of influencing factors of county-level ULUEE from 2006 to 2022. Note: The * in the figure indicates bi-enhancement, and ** is non-linear enhancement.
In 2006, interaction detection results identified 25 bi-enhancement relationships and 11 nonlinear enhancement relationships. Foreign trade (X8) demonstrated bi-enhancement relationships in all its interactions with other factors, indicating that when foreign trade (X8) interacted with other variables, it strengthened the explanatory power for ULUEE at the county level in Zhejiang Province. GDP per capita (X5) and industrial structure (X6) exhibited numerous nonlinear enhancement relationships when interacting with other factors. This suggests that the ULUEE at the county level in Zhejiang Province results from the combined effects of regional natural environment and socioeconomic development. Considering natural environmental factors alone cannot adequately explain the underlying driving mechanisms. The interaction between development vitality (X9) and precipitation (X4) showed the strongest explanatory power, with a q-value of 0.9057.
In 2010, interaction detection analysis revealed 28 bi-enhancement relationships and eight nonlinear enhancement relationships. Industrial structure (X6) exhibited nonlinear enhancement relationships in all its interactions with other factors, while the remaining factor interactions demonstrated bi-enhancement relationships. The interaction between development vitality (X9) and forest coverage rate (X3) showed the strongest explanatory power, with a q-value of 0.8078.
In 2014, the analysis identified seven bi-enhancement relationships and 29 nonlinear enhancement relationships. GDP per capita (X5), industrial structure (X6), fiscal expenditure scale (X7), and foreign trade (X8) all displayed nonlinear enhancement relationships in their interactions with other factors. Development vitality (X9) showed nonlinear enhancement relationships with all factors except elevation (X1), with which it exhibited a bi-enhancement relationship. These findings suggest that interactions among natural geographical factors had relatively minor effects, whereas interactions between natural geographical and socioeconomic factors demonstrated nonlinear enhancement characteristics, which were more decisive in determining the ULUEE at the county level in Zhejiang Province. The strongest explanatory power was observed in the interaction between industrial structure (X6) and elevation (X1), with a q-value of 0.7156.
In 2018, interaction detection analysis revealed three bi-enhancement relationships and 33 nonlinear enhancement relationships. Precipitation (X4), GDP per capita (X5), industrial structure (X6), fiscal expenditure scale (X7), foreign trade (X8), and development vitality (X9) all exhibited nonlinear enhancement relationships in their interactions with other factors. The interactions among elevation (X1), topographic relief (X2), and forest coverage rate (X3) demonstrated bi-enhancement relationships exclusively. This pattern suggests that interactions among regional geographical elements were insufficient to fully explain the distributional characteristics of county-level ULUEE. The interaction between industrial structure (X6) and topographic relief (X2) demonstrated the strongest explanatory power, with a q-value of 0.6954.
In 2022, the analysis identified four bi-enhancement relationships and 32 nonlinear enhancement relationships. The interactions involving precipitation (X4), GDP per capita (X5), industrial structure (X6), fiscal expenditure scale (X7), foreign trade (X8), and development vitality (X9) predominantly showed nonlinear enhancement relationships, with two exceptions: the interaction between fiscal expenditure scale (X7) and foreign trade (X8), precipitation (X4) and development vitality (X9), which exhibited bi-enhancement relationships. The strongest explanatory power was observed in the interaction between GDP per capita (X5) and forest coverage rate (X3), with a q-value of 0.5630.
3.3.2 County scale
The GWR model was subsequently employed for local spatial regression analysis to investigate the spatial variations in both the direction and magnitude of the nine influencing factors across different counties. The analysis yielded an adjusted R2 value of 0.4252, indicating strong model fitness and validating the reliability of the factor detection results. While all independent variables exhibited spatial non-stationarity, their degrees of variation and characteristic patterns showed distinct differences (Figure 8).
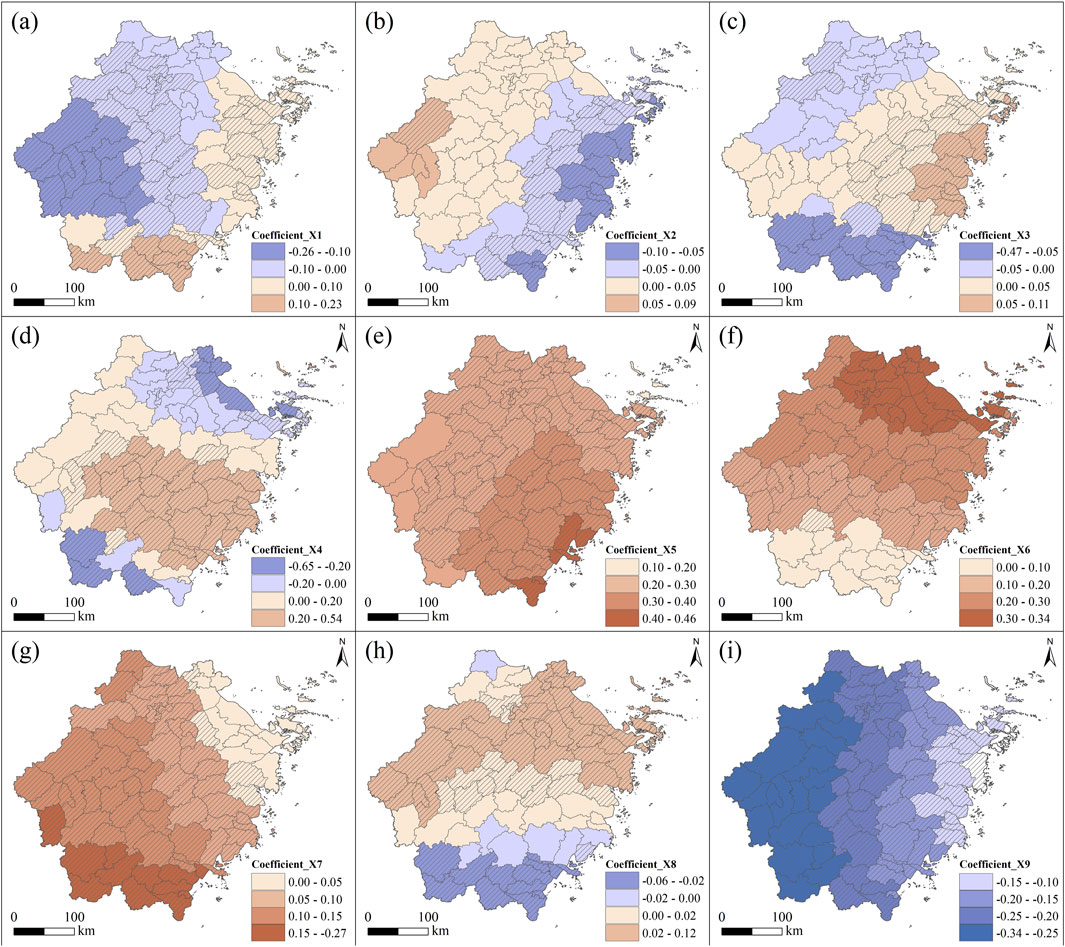
Figure 8. GWR model results of county-level ULUEE in Zhejiang province. Note: Counties denoted by diagonal lines represent passing the 5% significance test (p< 0.05).
The regression coefficients for elevation (X1) ranged from −0.26 to 0.23. Sixty-five counties passed the 5% significance test, with 26 demonstrating positive effects and 39 showing negative effects. In Ningbo, Wenzhou, Zhoushan, and Taizhou (specifically in Sanmen County, Jiaojiang District, Luqiao District, and Linhai City), elevation (X1) exhibits a significant positive effect. This suggests that in coastal counties, higher elevation is conducive to improving ULUEE. Conversely, in Hangzhou, Jinhua, Lishui, and Quzhou, a significant negative effect was observed, indicating that lower elevations in western Zhejiang are more favorable for ULUEE enhancement.
The regression coefficient for terrain ruggedness (X2) ranged between −0.1 and 0.09, characterized by a spatial distribution pattern of higher elevation in the west and lower elevation in the east. Thirty-four counties passed the 5% significance test, with only one demonstrating a positive effect and 33 showing negative effects. Chun’an County exhibited a significant positive effect. Surrounded by mountains and characterized by hilly terrain, Chun’an County is the sole special ecological function zone in Zhejiang Province and the only mountainous county in Hangzhou. Larger terrain ruggedness implies higher forest coverage and a superior ecological environment. Chun’an County leverages its ecological advantages for industrial development, thereby significantly promoting ULUEE. Conversely, significant negative effects were observed in Ningbo, Wenzhou, Taizhou, and Zhoushan. Coastal counties predominantly develop industrial economies based on marine resources, and flatter terrain facilitates industrial agglomeration and marine resource development, rendering terrain ruggedness (X2) a significant negative factor.
The regression coefficient for forest coverage (X3) ranged between −0.47 and 0.11. Forty-six counties passed the 5% significance test, with 29 demonstrating positive effects and 17 showing negative effects. Significant positive effects were primarily observed in Taizhou, Zhoushan, Ningbo, and Jinhua. Conversely, significant negative effects were predominantly found in Wenzhou and Lishui in southern Zhejiang. High forest coverage restricts available land resources for urban development, hindering industrial expansion and resource agglomeration. Consequently, this impedes further economic and social development, ultimately reducing the ULUEE.
The regression coefficient for precipitation (X4) ranged between −0.65 and 0.54. Out of 52 counties that passed the significance test at the 5% level, 35 exhibited a positive effect, while 17 showed a negative effect. Notably, significant positive effects were observed in Jinhua City, Lishui City, Taizhou City, and Wenzhou City. Abundant rainfall can promote vegetation growth and enhance the removal rate of PM2.5, thereby significantly improving the level of ULUEE. In contrast, significant negative effects were primarily found in Jiaxing City, Ningbo City, and Zhoushan City in the northeastern part of Zhejiang Province. Ningbo City and Zhoushan City are particularly affected by heavy rainfall brought on by typhoons, which have a considerable negative impact on social production, hence precipitation exhibits a significant negative effect in these areas.
The regression coefficient for GDP per capita (X5) ranged from 0.1 to 0.46, exhibiting a positive correlation with ULUEE across all counties, with a spatial distribution characterized by lower values in the northwest and higher values in the southeast. A total of 82 counties have passed the 5% significance test, all of which demonstrate a significant positive effect. This indicates that GDP per capita, as a crucial indicator of economic development, exerts a significant influence on the ULUEE.
The regression coefficients for industrial structure (X6) ranged from 0.0 to 0.34, exhibiting a positive correlation with ULUEE across all counties, with a spatial distribution characterized by higher values in the north and lower values in the south. Seventy-five counties have passed the 5% significance test, all of which show a significant positive effect. The upgrading of industrial structure, characterized by an increasing service sector, significantly promotes ULUEE in the associated counties.
The regression coefficients for the fiscal expenditure scale (X7) ranged from 0.0 to 0.2, exhibiting a positive correlation with ULUEE across all counties, with a spatial distribution characterized by lower values in the northeast and higher values in the southwest. Seventy-three counties have passed the 5% significance test, all of which demonstrated a significant positive effect. Counties with larger fiscal expenditure scales can concentrate financial advantages for the redevelopment of urban abandoned and idle land, thereby contributing to enhanced ULUEE.
The regression coefficients for foreign trade (X8) ranged from −0.06 to 0.12, exhibiting less variability compared to the other eight influencing factors, with only marginal spatial differences in effect, and its impact on ULUEE was positive in more than seven-ninths of the counties. Sixty-eight counties have passed the 5% significance test, with 54 showing a positive effect and 14 showing a negative effect. Significant positive effects were primarily observed in Hangzhou City, Jiaxing City, Jinhua City, Ningbo City, Quzhou City, Shaoxing City, and Zhoushan City. In contrast, significant negative effects were mainly found in Wenzhou City (10 counties and cities) and Lishui City (3 counties and cities). Wenzhou City’s private economy constitutes its main economic force, and a high total volume of import and export trade reflects the high scale and level of regional foreign trade. However, it also brings about higher negative externalities, such as environmental pollution, which suppresses the enhancement of ULUEE in Wenzhou City.
The regression coefficient of development vitality (X9) ranged from −0.34 to −0.1, exhibiting a consistently negative correlation with ULUEE across Zhejiang Province’s county domains. Spatially, the intensity of this effect demonstrated a gradient of Eastern < Central < Western regions. Notably, 88 counties passed the 5% significance test, all characterized as statistically significant negative impact zones. This finding suggests that as regional development vitality increases, human activity intensity escalates, consequently elevating undesired outputs, which substantially constrains the advancement of the ULUEE.
4 Discussions
4.1 Driving mechanism of county-level ULUEE in Zhejiang province
Urban land use systems function as complex, open systems characterized by extensive material cycling, energy flows, and information exchange with their external environment. The ULUEE is influenced by both regional natural environmental conditions and external socioeconomic forces. These dual driving forces collectively determine both the direction and intensity of evolution in ULUEE. In this study, a comprehensive evaluation index system was established. Based on the measurement of county-level ULUEE, the geodetector model and GWR model were employed to reveal the driving effects at global and local scales.
Overall analysis reveals that natural geographic factors, surpassing socioeconomic factors in number, demonstrated statistical significance. The declining q-values of driving factors indicate diminishing explanatory power as socioeconomic development progresses. Among natural geographic factors, precipitation (X4) exhibited strong explanatory power, while elevation (X1), topographic relief (X2), and forest coverage rate (X3) maintained consistent significant influences on ULUEE. The findings suggest that county-level ULUEE in Zhejiang Province is substantially constrained by natural geographic conditions, with this relationship becoming more pronounced over time. Among socioeconomic factors, foreign trade (X8) demonstrated the strongest initial explanatory power, while GDP per capita (X5) and industrial structure (X6) showed stronger explanatory power in later periods. The interaction detection results for the five socioeconomic factors exhibited a distinct non-linear enhancement trend when combined with other factors. Among natural geographic factors, only precipitation (X4) showed non-linear enhancement in interaction detection results. This indicates that socioeconomic factors strongly influence county-level ULUEE in Zhejiang Province, with their explanatory power significantly enhanced when interacting with natural geographic factors, reflecting the open and complex nature of urban land use systems. Regression analysis revealed that GDP per capita (X5), industrial structure (X6), and fiscal expenditure scale (X7) positively impacted county-level ULUEE in Zhejiang Province. Foreign trade (X8) showed both positive and negative effects, albeit with limited intensity, while development vitality (X9) demonstrated negative impacts. Natural geographic factors exhibited both positive and negative directional effects, indicating a more complex influence pattern compared to the generally consistent directional effects of socioeconomic factors. This suggests that adjusting socioeconomic factors could effectively regulate regional ULUEE.
4.2 Policy implications for improving county-level ULUEE of Zhejiang province
Due to the constraints imposed by its mountainous topography, Zhejiang Province faces significant urban land resource scarcity. Enhancing ULUEE has been identified as a critical factor in promoting sustainable development across the province. Based on the preceding analysis, the following policy recommendations are proposed.
(1) Enhance the utilization of existing construction Land. The optimization of existing construction land utilization requires a comprehensive approach focused on restructuring and promoting conservation-oriented, intensive land use. On the one hand, continue to push forward the reform of the land supply mechanism, establish an incentive mechanism for the comprehensive remediation and redevelopment and utilization of inefficient land, increase the renovation of old residential areas, old factory areas, and old commercial areas, and fully explore the potential of idle and inefficient land utilization, to increase the stock of land supply. On the other hand, the optimization of land use structure should be enhanced through upgrading of industrial structure, promoting the enhancement of eco-efficiency of land use, and advancing the digital transformation of traditional industries, to promote the reduction of pollution and carbon emissions.
(2) Advance ecological restoration and environmental protection. The enhancement of ecosystem stability requires a multifaceted approach encompassing several critical dimensions: ecosystem management, urban environmental governance, environmental enhancement initiatives, and technological integration. To improve ecosystem management, the government should strengthen ecosystem protection measures, promote harmonious development among ecological, productive, and residential spaces, and increase regional forest coverage rates. To strengthen urban environmental governance, the government should optimize urban land-use patterns, integrate economic, social, and ecological benefits, and enhance municipal environmental management capabilities. To improve environmental enhancement initiatives, the government should enhance the habitat quality and living environment standards, and implement beautification and greening programs. To enhance technological integration, we should leverage scientific and technological support for green, low-carbon development, deepen the application of digital technologies, particularly artificial intelligence, and establish an intelligent digital eco-civilization framework. These comprehensive approaches aim to create a sustainable balance between environmental preservation and urban development while incorporating advanced technological solutions.
(3) Further enhancement of Opening-up Policies. As a province characterized by a robust private economy, Zhejiang’s active participation in import and export trade significantly elevates regional economic performance and the ULUEE. The increased degree of economic openness facilitates the utilization of foreign capital and technology, thereby enhancing land productivity. Priority should be given to modernizing industrial and supply chains, continuing to strengthen the foundation for high-quality foreign trade development. The business environment should be optimized to attract foreign investment by benchmarking against successful international practices. The negative list for foreign investment access should be rationalized and reduced, directing foreign capital toward advanced manufacturing, modern services, high-tech industries, and energy conservation and environmental protection sectors.
(4) Innovate economic development models. It is necessary to adapt to local conditions, explore the advantages of location and special industries, and create green leading industries. Relying on local resources and location advantages to develop leading industries, the layout of high-tech industries, ecological industries, and actively explore the development mode of industrial integration to achieve high-quality economic and social development. For counties with relatively rich agricultural resources, it is necessary to make full use of the advantages of agricultural resources and ecological resources, on the one hand, vigorously develop green agricultural products planting, sightseeing agriculture, and picking agriculture, on the other hand, rely on the advantages of green water and green mountains, agricultural planting, etc., and vigorously develop rural tourism and leisure agriculture. At the same time, actively promote the integration of the digital economy and leading industries, increase the digital transformation and upgrading of industries, improve the efficiency of element allocation, and promote high-quality economic development.
(5) Promote the upgrading of industrial structure. Guide further optimization of the industrial structure, increasing the proportion of tertiary industry while reducing the share of primary and secondary industries, thereby establishing a modernized industrial pattern characterized by a “tertiary-secondary-primary” configuration. Continuously upgrade the internal structure of the industry. Industry remains the largest contributor to Zhejiang’s GDP. Using the development of advanced manufacturing as a key lever, continuously promote transformation and upgrading to achieve a metamorphosis of the industrial economy from “leading in speed” to “leading in quality.” Accelerate the development of modern service industries, vigorously develop the financial sector, real estate industry, and other service industries such as information transmission. Maintain high-speed growth of new momentum, promote the development of new industries, new forms of business, and new business models, leverage the advantages of digital economic development, drive deep bidirectional integration between advanced manufacturing and modern service industries, and cultivate new business forms and models of cross-industry integration.
4.3 Limitations and prospects
The key to evaluating the county-level ULUEE is to construct a proper index system. Based on previous studies, we selected labor, capital, and land as the input indicators (Zheng et al., 2023), considered economic, social, and ecological benefits in terms of desired outputs (Kong et al., 2023), and atmospheric pollution conditions in terms of undesirable outputs. The indicator system includes both statistical data remote sensing data and geospatial data, which can comprehensively reflect the ULUEE. In the selection of driving factors, most of the current studies choose socio-economic factors, however, the county-level ULUEE cannot be separated from the natural background conditions, so this paper also includes natural geographic factors. The results of the analyses show that the driving effect of the natural geographic factors is significant, and when the natural geographic factors and socio-economic factors interact, they are more capable of determining the level of county-level ULUEE. Consistent with previous studies, the level of economic development, industrial structure, and total amount of imports and exports had a significant effect on ULUEE.
However, there are some shortcomings in this study. Firstly, although 2006–2022 was chosen as the study interval, larger time scales are still necessary, such as conducting a study of the past 40 years, which can help to better reveal the evolution of the county-level ULUEE, and then discover the universal laws. In addition, although this study was refined to the county scale, it still did not break through the limitations of administrative boundaries, and future studies at the raster scale are needed to support the optimization of land space and precise governance.
5 Conclusion
(1) From 2006 to 2022, the county-level ULUEE of Zhejiang Province continued to improve, increasing from 0.34 to 0.73, with an average annual growth rate of 2.44%. At the same time, the absolute gap between counties decreased. At the municipal level, Zhoushan and Lishui had higher levels of the ULUEE, while Jiaxing was lower.
(2) From 2006 to 2022, the county ULUEE in Zhejiang Province showed a significant positive spatial correlation, with the global Moran’ I increasing from 0.3219 to 0.3715. At the local scale, the cold spot significant area gradually evolved from the northern part to the coexistence of the northern part and the southern part. At the same time, the northern part showed a continuous contraction, and the southern part shrank and then expanded. The number of counties in the hot spot area did not change much, but it showed obvious changes in space, and the distribution was gradually contracting from the main cluster in Hangzhou, Lishui, and Quzhou in western and southwestern Zhejiang while shifting to Ningbo and Zhoushan in northeastern Zhejiang.
(3) From 2006 to 2022, the number of natural geographic factors that passed the significance test was more than the socio-economic factors, and the q-value of the driving factors showed a decreasing trend. Among the natural geographic factors, precipitation (X4) had the strongest explanatory power in the early period, and elevation (X1), topographic relief (X2), and forest coverage rate (X3) always played a stronger role in ULUEE. Among the socio-economic factors, foreign trade (X8) had the strongest explanatory power in the early period, and GDP per capita (X5) and industrial structure (X6) had stronger explanatory power in the later period. The interaction detection results of the five socio-economic factors with other factors showed an obvious non-linear enhancement trend and only precipitation (X4) showed a non-linear enhancement trend in the interaction detection. In the regression analysis, GDP per capita (X5), industrial structure (X6), and financial expenditure scale (X7) showed positive effects on county-level ULUEE in Zhejiang Province, foreign trade (X8) had both positive and negative effects but with weaker intensity, and development vitality (X9) showed negative effects. The natural geography factors had both positive and negative effects. It showed that the county-level ULUEE of Zhejiang province was more complicated by the role of natural geographic factors, the effects of socio-economic factors are consistent, and the adjustment of socio-economic factors can effectively regulate the ULUEE of the region.
Data availability statement
The original contributions presented in the study are included in the article/supplementary material, further inquiries can be directed to the corresponding authors.
Author contributions
LF: Data curation, Formal Analysis, Investigation, Visualization, Writing–original draft, Writing–review and editing. LM: Project administration, Supervision, Validation, Writing–review and editing. ZH: Conceptualization, Investigation, Methodology, Project administration, Supervision, Writing–review and editing.
Funding
The author(s) declare that no financial support was received for the research, authorship, and/or publication of this article.
Conflict of interest
The authors declare that the research was conducted in the absence of any commercial or financial relationships that could be construed as a potential conflict of interest.
Publisher’s note
All claims expressed in this article are solely those of the authors and do not necessarily represent those of their affiliated organizations, or those of the publisher, the editors and the reviewers. Any product that may be evaluated in this article, or claim that may be made by its manufacturer, is not guaranteed or endorsed by the publisher.
References
Agnew, D. C. (2024). A global timekeeping problem postponed by global warming. Nature 628, 333–336. doi:10.1038/s41586-024-07170-0
Bai, Y., Deng, X., Jiang, S., Zhang, Q., and Wang, Z. (2018). Exploring the relationship between urbanization and urban eco-efficiency: evidence from prefecture-level cities in China. J. Clean. Prod. 195, 1487–1496. doi:10.1016/j.jclepro.2017.11.115
Cao, X., Liu, Y., Li, T., and Liao, W. (2019). Analysis of spatial pattern evolution and influencing factors of regional land use efficiency in China based on ESDA-GWR. Sci. Rep. 9 (1), 520. doi:10.1038/s41598-018-36368-2
Chen, M. J., Wang, Q. R., Bai, Z. K., Shi, Z. Y., Meng, P., and Hao, M. (2022). Green land use efficiency and influencing factors of resource-based cities in the yellow river basin under carbon emission constraints. BUILDINGS 12 (5), 551. doi:10.3390/buildings12050551
Chen, W. D., Si, W., and Chen, Z. M. (2020). How technological innovations affect urban eco-efficiency in China: a prefecture-level panel data analysis. J. Clean. Prod. 270, 122479. doi:10.1016/j.jclepro.2020.122479
Cook, W. D., and Seiford, L. M. (2009). Data envelopment analysis (DEA) - thirty years on. Eur. J. OPERATIONAL Res. 192 (1), 1–17. doi:10.1016/j.ejor.2008.01.032
Cui, X. H., Lin, M. Y., and Qiu, Y. (2023). Regional differences and dynamic evolution of urban land green use efficiency within the Yangtze River economic belt, China. Front. Environ. Sci. 10. doi:10.3389/fenvs.2022.1098924
Den, X. Z., and Gibson, O. (2020). Sustainable land use management for improving land eco-efficiency: a case study of Hebei, China. Ann. Operations Res. 290 (1-2), 265–277. doi:10.1007/s10479-018-2874-3
Fan, X. X., and Jiang, X. (2023). Regional differences and convergence of urban land green use efficiency in China under the constraints of carbon neutrality. Environ. Dev. Sustain. 26, 23499–23525. doi:10.1007/s10668-023-03607-0
Ge, K., Wang, Y., Ke, S. A., and Lu, X. H. (2023). Research on the spatiotemporal evolution and driving mechanism of coupling coordinating between green transition of urban land use and urban land use efficiency: a case study of the Yangtze River Delta Region in China. Environ. Sci. Pollut. Res. 31, 57002–57024. doi:10.1007/s11356-023-31072-9
Ge, K., Zou, S., Ke, S., and Chen, D. (2021). Does urban agglomeration promote urban land green use efficiency? Take the Yangtze River economic zone of China as an example. Sustainability 13 (19), 10527. doi:10.3390/su131910527
Hong, J. X., and Mao, Y. L. (2023). How does the green efficiency of urban land use evolve in the urban agglomeration of China's middle Yangtze River? Nat. Resour. Model. 37. doi:10.1111/nrm.12392
Hu, Y., Liu, X., Zhang, Z., Wang, S., and Zhou, H. (2022). Spatiotemporal heterogeneity of agricultural land eco-efficiency: a case study of 128 cities in the Yangtze River basin. Water 14 (3), 422. doi:10.3390/w14030422
Huang, X. B., Liu, M., Chen, R. D., Gao, S. Q., Xie, Q. Q., and Feng, Q. L. (2021). Evaluation and spatiotemporal characteristics of atmospheric environment efficiency in Shandong province based on super-SBM model. Environ. Res. Commun. 3 (11), 115002. doi:10.1088/2515-7620/ac3409
Huang, Y., Li, L., and Yu, Y. T. (2018). Do urban agglomerations outperform non-agglomerations? A new perspective on exploring the eco-efficiency of the Yangtze River Economic Belt in China. J. Clean. Prod. 202, 1056–1067. doi:10.1016/j.jclepro.2018.08.202
Huntington, H. P., Schmidt, J. I., Loring, P. A., Whitney, E., Aggarwal, S., Byrd, A. G., et al. (2021). Applying the food–energy–water nexus concept at the local scale. Nat. Sustain. 4 (8), 672–679. doi:10.1038/s41893-021-00719-1
Kong, F., Zhang, K., Fu, H., Cui, L., Li, Y., and Wang, T. (2023). Temporal-spatial variations and convergence analysis of land use eco-efficiency in the urban agglomerations of the yellow river basin in China. Sustainability 15 (16), 12182. doi:10.3390/su151612182
Li, C., Qiao, W., Gao, B., and Chen, Y. (2024). Unveiling spatial heterogeneity of ecosystem services and their drivers in varied landform types: insights from the Sichuan-Yunnan ecological barrier area. J. Clean. Prod. 442, 141158. doi:10.1016/j.jclepro.2024.141158
Li, H., and Shi, J. F. (2014). Energy efficiency analysis on Chinese industrial sectors: an improved Super-SBM model with undesirable outputs. J. Clean. Prod. 65, 97–107. doi:10.1016/j.jclepro.2013.09.035
Li, H. Y., Wang, Z. Q., Zhu, M. Y., Hu, C. X., and Liu, C. (2023). Study on the spatial-temporal evolution and driving mechanism of urban land green use efficiency in the Yellow River Basin cities. Ecol. Indic. 154, 110672. doi:10.1016/j.ecolind.2023.110672
Liu, F., Zhang, G. Y., Li, C. H., Ren, T., and Masi, D. (2023). Analysis of the temporal and spatial pattern and convergence characteristics of high-quality sustainable economic development of urban agglomeration. SUSTAINABILITY 15 (20), 14807. doi:10.3390/su152014807
Liu, J., Jin, X. B., Xu, W. Y., Fan, Y. T., Ren, J., Zhang, X. L., et al. (2019). Spatial coupling differentiation and development zoning trade-off of land space utilization efficiency in eastern China. LAND USE POLICY 85, 310–327. doi:10.1016/j.landusepol.2019.03.034
Liu, S. C., Xiao, W., Li, L. L., Ye, Y. M., and Song, X. L. (2020). Urban land use efficiency and improvement potential in China: a stochastic frontier analysis. Land Use Policy 99, 105046. doi:10.1016/j.landusepol.2020.105046
Liu, Y., Fang, F., and Li, Y. (2014). Key issues of land use in China and implications for policy-making. Land Use Policy 40, 6–12. doi:10.1016/j.landusepol.2013.03.013
Liu, Y., Sun, H., Shi, L., Wang, H., Xiu, Z., Qiu, X., et al. (2021). Spatial-temporal changes and driving factors of land-use eco-efficiency incorporating ecosystem services in China. Sustainability 13 (2), 728. doi:10.3390/su13020728
Liu, Z., Lai, B., Wu, S., Liu, X., Liu, Q., and Ge, K. (2022). Growth targets management, regional competition and urban land green use efficiency according to evidence from China. Int. J. Environ. Res. Public Health 19 (10), 6250. doi:10.3390/ijerph19106250
Lou, S., Liu, Y., Bai, Y., Li, F., Lin, G., Xu, L., et al. (2023). Projections of mortality risk attributable to short-term exposure to landscape fire smoke in China, 2021-2100 a health impact assessment study. Lancet Planet. Health 7 (10), e841–e849. doi:10.1016/s2542-5196(23)00192-4
Lu, X., Qu, Y., Sun, P., Yu, W., and Peng, W. (2020). Green transition of cultivated land use in the yellow river basin: a perspective of green utilization efficiency evaluation. Land 9 (12), 475. doi:10.3390/land9120475
Ma, D. L., Zhang, J. W., Zhang, F. T., Xiao, Y. P., Tan, H. M., Guo, Z. M., et al. (2023a). What were the spatiotemporal evolution characteristics and the influencing factors of urban land green use efficiency? A case study of the Yangtze River Economic Belt. Environ. Monit. Assess. 195 (7), 806. doi:10.1007/s10661-023-11413-4
Ma, L., Xu, W., Zhang, W., and Ma, Y. (2024). Effect and mechanism of environmental regulation improving the urban land use eco-efficiency: evidence from China. Ecol. Indic. 159, 111602. doi:10.1016/j.ecolind.2024.111602
Ma, L. D., Xu, W. X., Hong, Y. X., He, S. C., Liu, C. J., and Ning, Q. (2023b). Can new urbanization and ecological environment achieve synergistic development? Empirical evidence from 63 counties in Zhejiang, China. PLOS ONE 18 (9), e0291867. doi:10.1371/journal.pone.0291867
Ma, M., Liu, Y., Wang, B., Yan, X., and Li, H. (2023c). Spatial-temporal evolution and driving mechanism of urban land use efficiency based on T-DEA model: a case study of anhui province, China. Sustainability 15 (13), 10087. doi:10.3390/su151310087
Nerem, R. S., Beckley, B. D., Fasullo, J. T., Hamlington, B. D., Masters, D., and Mitchum, G. T. (2018). Climate-change-driven accelerated sea-level rise detected in the altimeter era. Proc. Natl. Acad. Sci. U. S. A. 115 (9), 2022–2025. doi:10.1073/pnas.1717312115
Sun, J. N., and Zhou, T. (2023). Urban shrinkage and eco-efficiency: the mediating effects of industry, innovation, and land-use. Environ. Impact Assess. Rev. 98, 106921. doi:10.1016/j.eiar.2022.106921
Sun, Y., and Wang, N. (2022). Eco-efficiency in China’s Loess Plateau Region and its influencing factors: a data envelopment analysis from both static and dynamic perspectives. Environ. Sci. Pollut. Res. 29 (1), 483–497. doi:10.1007/s11356-021-15278-3
Tang, M. G., Li, Z., Hu, F. X., and Wu, B. J. (2020). How does land urbanization promote urban eco-efficiency? The mediating effect of industrial structure advancement. J. Clean. Prod. 272, 122798. doi:10.1016/j.jclepro.2020.122798
Wang, J. F., Li, X. H., Christakos, G., Liao, Y. L., Zhang, T., Gu, X., et al. (2010). Geographical detectors-based health risk assessment and its application in the neural tube defects study of the heshun region, China. Int. J. Geogr. Inf. Sci. 24 (1), 107–127. doi:10.1080/13658810802443457
Xue, S., Wang, C., Zhang, S., Weng, C., and Zhang, Y. (2023). Eco-efficiency of the urban agglomerations: spatiotemporal characteristics and determinations. Land 12 (7), 1275. doi:10.3390/land12071275
Yang, H. R., and Wu, Q. (2019). Land use eco-efficiency and its convergence characteristics under the constraint of carbon emissions in China. Int. J. Environ. Res. Public Health 16 (17), 3172. doi:10.3390/ijerph16173172
Yang, H. R., Zheng, H., Liu, H. G., and Wu, Q. (2019). NonLinear effects of environmental regulation on eco-efficiency under the constraint of land use carbon emissions: evidence based on a bootstrapping approach and panel threshold model. Int. J. Environ. Res. Public Health 16 (10), 1679. doi:10.3390/ijerph16101679
Ye, W. F., Ma, Z. Y., and Ha, X. Z. (2018). Spatial-temporal patterns of PM2.5 concentrations for 338 Chinese cities. Sci. Total Environ. 631-632, 524–533. doi:10.1016/j.scitotenv.2018.03.057
Yu, J. Q., Zhou, K. L., and Yang, S. L. (2019). Land use efficiency and influencing factors of urban agglomerations in China. LAND USE POLICY 88, 104143. doi:10.1016/j.landusepol.2019.104143
Yu, L., and Liu, K. (2024). Land green utilization efficiency and its driving mechanisms in the zhengzhou metropolitan area. Sustainability 16 (13), 5447. doi:10.3390/su16135447
Yu, Y. T., and Luo, N. S. (2023). DOes land marketization improve eco-efficiency? evidence from China. Technol. Econ. Dev. Econ. 29 (2), 539–563. doi:10.3846/tede.2023.18085
Zhang, R. T., and Lu, J. F. (2022). Spatial-temporal pattern and convergence characteristics of provincial urban land use efficiency under environmental constraints in China. Int. J. Environ. Res. PUBLIC HEALTH 19 (17), 10729. doi:10.3390/ijerph191710729
Zhao, Z., Bai, Y., Wang, G., Chen, J., Yu, J., and Liu, W. (2018). Land eco-efficiency for new-type urbanization in the Beijing-Tianjin-Hebei Region. Technol. Forecast. Soc. Change 137, 19–26. doi:10.1016/j.techfore.2018.09.031
Zheng, H., Wu, Y., He, H., Delang, C. O., Qian, J., Lu, J., et al. (2023). Urban land use eco-efficiency and improvement in the western region of China. J. Clean. Prod. 412, 137385. doi:10.1016/j.jclepro.2023.137385
Zhou, C., Shi, C., Wang, S., and Zhang, G. (2018). Estimation of eco-efficiency and its influencing factors in Guangdong province based on Super-SBM and panel regression models. Ecol. Indic. 86, 67–80. doi:10.1016/j.ecolind.2017.12.011
Zhou, L., Zhou, C., Che, L., and Wang, B. (2020a). Spatio-temporal evolution and influencing factors of urban green development efficiency in China. J. Geogr. Sci. 30 (5), 724–742. doi:10.1007/s11442-020-1752-5
Zhou, Y., Kong, Y., Wang, H. K., and Luo, F. Y. (2020b). The impact of population urbanization lag on eco-efficiency: a panel quantile approach. J. Clean. Prod. 244, 118664. doi:10.1016/j.jclepro.2019.118664
Keywords: urban land use eco-efficiency (ULUEE), super efficiency SBM model, geodetector model, sustainable development, Zhejiang province
Citation: Fan L, Ma L and Huang Z (2025) Spatial-temporal pattern and driving mechanism of urban land use eco-efficiency in mountainous counties based on multi-source data: a case study of Zhejiang province, China. Front. Environ. Sci. 12:1500948. doi: 10.3389/fenvs.2024.1500948
Received: 24 September 2024; Accepted: 16 December 2024;
Published: 07 January 2025.
Edited by:
Merja H. Tölle, University of Kassel, GermanyReviewed by:
Na Dong, Sun Yat-sen University, ChinaSvitlana Delehan, Uzhhorod National University, Ukraine
Copyright © 2025 Fan, Ma and Huang. This is an open-access article distributed under the terms of the Creative Commons Attribution License (CC BY). The use, distribution or reproduction in other forums is permitted, provided the original author(s) and the copyright owner(s) are credited and that the original publication in this journal is cited, in accordance with accepted academic practice. No use, distribution or reproduction is permitted which does not comply with these terms.
*Correspondence: Lindong Ma, bWFsaW5kb25nQHpqbnUuZWR1LmNu; Zhongwei Huang, aHp3QHpqbnUuZWR1LmNu