- 1School of Public Administration, Nanjing Agricultural University, Nanjing, China
- 2China Academy of Resources, Environment and Development, Nanjing Agricultural University, Nanjing, China
- 3Rural Economic Research Center, Ministry of Agriculture and Rural Affairs of the People’s Republic of China, Beijing, China
Integrating territorial spatial planning with the spatial autocorrelation of cultivated land quality indices can enhance the optimization of farmland preservation and food security. This study combines the “three lines” demarcation—basic farmland protection line, ecological protection line, and urban development boundary—with farmland spatial autocorrelation to propose an improved model for optimizing farmland layout. We employed a four-dimensional farmland quality evaluation system that includes the natural quality index, utilization management index, ecological environment index, and economic value index. Using Liuhe District as a case study, we applied the optimal combination weighting method for quality assessment and conducted spatial autocorrelation analysis to simulate the indices’ relationships at the plot scale. The results indicate that: (1) The natural quality index is high in the west and low in the east, while the other indices are high in the center and low at the margins. (2) Each quality index exhibits positive geographic autocorrelation, with Moran’s I values of 0.89, 0.67, 0.65, and 0.83, respectively. (3) The optimization scheme increased permanent basic farmland by 123.15 hm² and improved the quality grade by 1.45, classifying land into four primary categories focused on protection and development. The permanent basic farmland protection zone has the highest quality and non-agricultural construction should be prohibited on it. The urban development buffer zone, which has poor farmland quality and a locational advantage, is ideal for urban growth. The ecological environmental protection zone, despite its poor farmland quality, should be focused on conservation. The comprehensive adjustment zone should address land obstructions and facilitate a transition to permanent basic farmland.
1 Introduction
Farmland is the material foundation for human survival and development, being crucial for ensuring national food security, encouraging sustainable socioeconomic development, and maintaining national security and social stability (Xin and Li, 2018). According to the United Nations Food and Agriculture Organization, the amount of global arable land has been decreasing since the 1960s, with a reduction of approximately 30 million hectares between 2015 and 2020 (Mottaleb et al., 2023). At the same time, as the world’s largest developing country, China sustains nearly 20% of the global population with only 9% of the world’s arable land and 6% of its freshwater, which places tremendous pressure on its arable land (Zhu et al., 2022). Alongside the reduction in arable land area, problems such as environmental degradation and declining land quality have become increasingly prominent. According to the “China Natural Resources Bulletin 2023,” the current cultivated land area in China is 127.58 million hectares (Liu et al., 2024). Based on the national standard “Grade of Cultivated Land Quality” (GB/T 33469-2016), the cultivated land is classified into 13 grades from high to low, with the average grade of cultivated land quality in China calculated to be 9.96 (Liu et al., 2023). This area decreased by 127,000 ha due to construction, ecological land conversion, and other factors. The overall low quality and spatial imbalance of arable land are both increasingly evident. The reduction in arable land not only threatens food production and supply, but it also can lead to environmental degradation (Wang, 2015). The reasons for the gradual reduction in arable land are varied, and include urban expansion, infrastructure development, land degradation, and natural disasters (Kuang Y. P. et al., 2022). Therefore, optimizing the layout of arable land and improving land use efficiency have become pressing issues that need to be addressed. Through the scientifically rational optimization of arable land layout, we can effectively protect existing land resources and improve overall land quality and output levels, thereby providing a solid guarantee for food security. Additionally, optimizing the spatial layout of arable land plays an important role in improving rural environments and promoting ecological health.
China has implemented various policy measures for farmland protection, among which national spatial planning plays a key role. In 2018, the Central Committee of the Communist Party of China and the State Council issued the “Opinions on Establishing a National Spatial Planning System and Supervising Its Implementation,” which proposed optimizing the spatial development pattern of land, delineating areas of permanent basic farmland, and implementing the strictest possible farmland protection system (Lichtenberg and Ding, 2008). Furthermore, in 2019, the General Office of the Central Committee of the Communist Party of China and the General Office of the State Council issued the “Guiding Opinions on the Overall Delineation and Implementation of the Three Control Lines in the Land and Space Planning”, emphasizing the significance of the permanent basic farmland protection red line, the ecological protection red line and the urban development boundary (hereinafter referred to as the “three lines”) to ensure national food security, promote the concentrated protection of cultivated land and promote the high-quality protection and utilization of cultivated land (Zhang et al., 2019). National spatial planning aims to achieve the sustainable use and rational allocation of land resources through scientific land use planning and management, ensuring the stability of the quantity of arable land and the improvement of its quality (Ye et al., 2024). However, current farmland protection planning has somewhat overlooked the spatial correlation of farmland quality, resulting in significant overlap between arable land areas, ecologically fragile areas, urban expansion zones, and heavy metal pollution zones. Specifically, the spatial autocorrelation of farmland quality means that high-quality farmland tends to cluster in certain areas, while low-quality farmland is distributed in other areas (Pang et al., 2023). Ignoring this can lead to the uneven distribution of resources and the reduced effectiveness of farmland protection. In light of this, combining national spatial planning with the spatial autocorrelation characteristics of farmland quality to implement differentiated farmland protection strategies not only helps improve the scientific and effective protection and utilization of farmland, but it also provides strong support for national food security and ecological protection. This approach can serve as a reference for promoting coordinated regional economic development, fine-tuning farmland management, and ensuring food security.
Many extensive studies have been conducted on the subject of optimizing the layout of cultivated land, with most research primarily focusing on the following aspects: theoretical frameworks for farmland protection (Ma et al., 2020), the evaluation and monitoring of farmland quality (Chen Y. M. et al., 2021), spatial optimization models and methods (Gao et al., 2017), and the impact and practical application of policies (Qie et al., 2023). First, regarding theoretical frameworks, researchers have generally found that farmland protection is not only about protecting and maintaining the quantity of farmland, but also about improving the quality of this land and ensuring its sustainable use (Tang et al., 2021). Consequently, the concept of farmland protection has gradually shifted from focusing on single-dimensional quantity protection to integrated protection that balances both quantity and quality (Qi et al., 2024). Some scholars have proposed the concept of “farmland ecosystem service value,” emphasizing that farmland is not only the foundation of food production, but that it also has ecological and social service functions (Song et al., 2022). This provides a broader perspective for research on optimizing farmland spatial layout. Second, the evaluation and monitoring of farmland quality are essential foundations for achieving the optimal spatial layout of arable land (Cheng et al., 2017). Extensive research has been conducted in this area, mainly focusing on constructing indicator systems, developing evaluation methods, and investigating their applications. Common evaluation indicators include soil fertility, soil structure, irrigation conditions, and environmental conditions (Liang et al., 2022; Chen Y. et al., 2021; Kuang L. H. et al., 2022). In recent years, the application of remote sensing technology and Geographic Information Systems (GIS) has provided strong support for the large-scale dynamic monitoring of farmland quality (Yan et al., 2017). Third, in terms of spatial optimization models and methods, researchers have developed various models and algorithms for use in guiding the optimization of farmland spatial layout. Commonly used models include multi-objective planning models (Wang et al., 2014), genetic algorithms (Wang et al., 2014), and simulated annealing algorithms (Gao et al., 2017). These models and algorithms can determine optimal farmland layout schemes by considering multiple factors such as the quantity, quality, and ecological protection of farmland. In addition, optimization methods based on big data and artificial intelligence technologies (Liu et al., 2011) have also begun to be applied in farmland spatial layout research, demonstrating broad application prospects. Lastly, policy impacts and practical applications are important aspects of research on optimizing the spatial layout of farmland. The academic community has extensively studied the effects of various policy measures, analyzing their effectiveness and shortcomings in practical applications. On the one hand, research indicates that government-implemented farmland protection policies have effectively curbed the loss of farmland, although there is still room for improvement in enhancing land quality (Zhang et al., 2018). On the other hand, some local governments have accumulated rich experience in farmland protection practices, providing useful references for other regions (Ippolito et al., 2021).
Farmland quality encompasses natural, economic, locational, and ecological dimensions. Current research on optimizing farmland spatial layout is mostly based on the evaluation of farmland quality and considers factors such as soil fertility, infrastructure completeness, locational conditions, and policy conditions. However, a widely accepted theoretical consensus has yet to be reached. In the early 1920s, the United States proposed the Land Capability Classification System, which became the first international framework for identifying high-quality farmland (Taveira et al., 2021). In 1981, based on this framework, the United States proposed the LESA (Land Evaluation and Site Assessment) system (Qian F. et al., 2021), which became a widely recognized international standard for farmland quality assessment. In summary, the aforementioned research lays the foundation for scientifically evaluating farmland quality and optimizing the spatial layout of arable land. However, there are still some deficiencies: (1) While existing research has established a research approach to evaluating farmland quality based on natural quality, locational conditions, and landscape conditions (Chen et al., 2016; Guo and Han, 2021), most studies on the spatial autocorrelation of farmland quality lack depth. (2) Although a few studies have noted the impact of the spatial autocorrelation of farmland quality on the optimization of the spatial layout of farmland and have conducted spatial correlation analysis on natural attributes, grading, and usage conditions (Han et al., 2022), they do not sufficiently consider its correlation with the ecological environment. (3) Existing research mainly delineates farmland protection zones from the perspectives of food security, comprehensive food view, and form and structure (Badenhausser et al., 2012; Huo et al., 2022), but it does not combine the spatial autocorrelation attributes of farmland quality with national spatial planning to deeply analyze the impact of the correlations of natural, economic, utilization, and ecological dimensions with the spatial layout of farmland. (4) Most existing studies analyze the spatial autocorrelation of farmland quality at the village scale with counties as the research object (Liu J. et al., 2022; Tan et al., 2020), while studies using farmland plots as the research unit are relatively rare.
In light of this, this study incorporates the ecological environment as the ‘fourth dimension’ within the spatial autocorrelation analysis framework of farmland quality. Using the delineation results from the national spatial planning of Liuhe District, Jiangsu Province, China, this research adopts an improved spatial autocorrelation model. Farmland plots serve as the research unit to explore the spatial correlation patterns among several key factors: the natural quality, management utilization, economic value, and ecological environment index of farmland. This analysis aims to identify effective protection strategies for permanent basic farmland. The national spatial planning of Liuhe District follows the “three-line’ system, which delineates three key control lines: the ecological protection red line, the permanent basic farmland line, and the urban development boundary line. This study utilizes the results of these delineations to inform the directions for urban development expansion and the timing and challenges of ecological space remediation. Ultimately, this work seeks to provide valuable references for enhancing farmland quality and optimizing its layout.
2 Study area and data collection
Liuhe District is part of the city of Nanjing in Jiangsu Province, China, located between 31°13′–31°26′N and 118°41′–119°21′E and covering an area of 14,710,000 hm2. This area is a nationally recognized agricultural base (Figure 1). According to the 2020 land use change data for Liuhe District, it has 57509.88 ha of arable land that has an average quality grade of 5.18. Since the implementation of the “Twelfth Five-Year Plan,” Liuhe District has established a comprehensive routine soil environmental quality monitoring system through 632 arable land quality monitoring units. In recent years, the ecological environment of the arable land in Liuhe District has been severely damaged due to the excessive use of chemical fertilizers and sewage irrigation, as well as the misuse of pesticides and agricultural films, posing a significant threat to farmland quality.
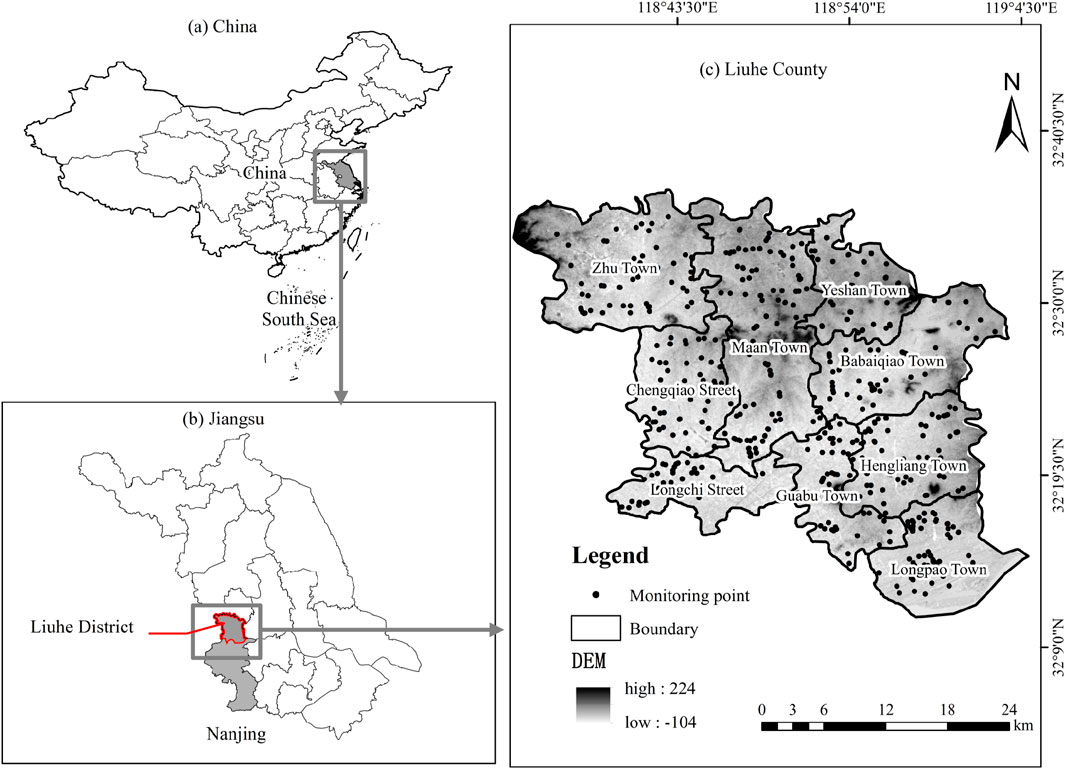
Figure 1. Maps showing the location of the Liuhe District and its current administrative divisions. (A) Location of Jiangsu Province in China; (B) the location of Liuhe District in Jiangsu Province and Nanjing City; (C) the digital elevation model (DEM) of Liuhe District.
The spatial data were sourced from the year 2017 and include a comprehensive set of resources. Notably, the “three-line” national spatial planning results encompass the red line of ecological protection, the permanent basic farmland, and control line of urban development boundary. These three lines serve as critical boundaries for economic restructuring, industrial planning, and urbanization processes. Specifically, they implement the strictest ecological environment protection system, cultivated land protection system, and land-saving system. In Nanjing, these lines further delineate the cultivated land protection space, ecological protection space, and urban development space. However, due to the complexities of long-term land space planning and the variability in cultivated land quality, there are instances where low-quality cultivated land is designated within the cultivated land protection space, while high-quality cultivated land is occupied by urban development. To address this issue, this study, based on Nanjing’s ecological protection red line, permanent basic farmland, and urban development boundary, conducted an evaluation of cultivated land quality and spatial autocorrelation analysis. This analysis allowed for the adjustment of low-quality cultivated land located within the cultivated land protection red line to the ecological protection space and urban development space. Conversely, high-quality cultivated land situated in the ecological protection and urban development spaces was regulated back into the cultivated land protection space, aiming to optimize the spatial layout of cultivated land protection. Additionally, the spatial dataset incorporates high-standard basic farmland results, the agricultural land classification database, basic farmland database, soil survey data, elevation data, and data from 632 monitoring points provided by the Nanjing Agriculture and Rural Affairs Bureau. Firstly, to ensure the authenticity of the data, we compiled all relevant datasets and standardized them by converting measurement units to a consistent format. This process involved identifying discrepancies among the units used across different datasets, applying conversion factors where necessary, and verifying the accuracy of the standardized data through cross-referencing with established benchmarks. Then, to facilitate spatial analysis, all spatial data were uniformly converted to the CGCS2000 coordinate system, specifically using the 3-Degree GK Zone 27 projection. This coordinate system is designed to enhance spatial accuracy and consistency across datasets, ensuring reliable results in subsequent analyses. Finally, exploratory data analysis was used to remove extreme values from key indicators, such as soil quality metrics and crop yields, in order to eliminate their potential impact on the results of the spatial correlation analysis.
Indicators such as terrain slope, effective soil layer thickness, soil texture configuration, bulk density, pH, organic matter, farmland shelterbelt, drainage capacity, irrigation capacity, available phosphorus, available potassium, safe utilization rate, and biodiversity were sourced from the agricultural land classification database of Liuhe District. Grain crop yield and cost data were obtained from the “2021 Liuhe District Statistical Yearbook,” and the data for mercury, cadmium, chromium, and lead contents were obtained through spatial interpolation from the 632 monitoring points in Liuhe District. Finally, the textual data involved in this study consist of several key governmental and planning documents. Specifically, the “Revised Plan for the Overall Land Use Planning of Liuhe District, Nanjing” provides comprehensive guidelines for land use within the Liuhe District, detailing zoning regulations, protected areas, and development plans. This document is crucial for understanding the spatial distribution and potential changes in farmland use within the study area. The “2021 Nanjing Basic Farmland Protection Report” offers insights into the current status of basic farmland protection, including acreage, protection measures, and challenges faced. It serves as a benchmark for assessing the effectiveness of farmland preservation policies. Additionally, the “High-standard Farmland Construction Plan of Jiangsu Province (2014–2020)” outlines the objectives, strategies, and achievements of upgrading farmland to higher standards of productivity and sustainability. This plan is instrumental in identifying trends and best practices in farmland development. Lastly, the “Nanjing Basic Farmland Quality Monitoring Report (2015–2017)” presents detailed data on soil quality, crop yields, and other relevant indicators, providing a quantitative basis for evaluating farmland health and productivity. Collectively, these textual data sources contribute to the research by offering a multifaceted view of farmland use, protection, and improvement in Liuhe District, Nanjing, and enable a comprehensive analysis of the study’s objectives.
3 Method
3.1 Comprehensive evaluation of farmland quality
3.1.1 Index selection
This study references the “2021 Nanjing Basic Farmland Protection Report” to describe farmland quality using four aspects: natural quality, utilization management, economic value, and the ecological environment (Table 1) (Cracolici et al., 2010). (1) The natural quality index characterizes the intrinsic conditions of farmland, which are crucial for productivity (Qian F. K. et al., 2021); the terrain slope, effective soil layer thickness, soil texture configuration, bulk density, pH, and organic matter content were chosen to reflect the natural quality of farmland (Zhang R. Q. et al., 2022). (2) The utilization management index reflects the level of management by farmers. Therefore, farmland shelterbelt, irrigation capacity, drainage capacity, available phosphorus, and available potassium were selected to indicate differences in utilization management levels, including shelterbelt networks, irrigation, drainage channels, and fertilization practices. (3) The economic value index is the sum of benchmark crop yields, converted using yield ratio coefficients, that were obtained under the natural quality conditions of the farmland, as well as the average utilization conditions of the land use zone and average economic conditions of the land economic zone (Equations 1–4) (Pandit et al., 2013). It can also be interpreted as the maximum economic yield level that farmland within the classification unit can achieve under current technical and economic conditions (Zhou et al., 2022). The land economic coefficient is the ratio of the effective yield to the total yield of specific crops in the plot, and it can be used to describe differences in production capacity when the economic development level and input–output intensity are the same (Equation 3) (Kuethe et al., 2014). (4) Additionally, existing studies rarely consider the impact of the spatial aggregation of farmland ecological environment quality on farmland spatial layout (Baek et al., 2022; Li et al., 2022; Maddison, 2009). To address this gap, this study incorporates an ecological environment index into the analysis framework of farmland quality spatial autocorrelation. This index includes indicators such as the safe utilization rate, biodiversity, and heavy metal content (mercury, cadmium, chromium, and lead) (Liang et al., 2022). By integrating these indicators, we aim to capture the ecological factors influencing farmland layout and quality, thus enhancing the robustness of our spatial analysis.
where EIi is the economic value index of the ith farming unit. NQIi is the natural quality index of the ith farming unit. KLi is the utilization management index of the ith farming unit. UMIi is the utilization management index of the ith farming unit. UMImax is the maximum utilization management index among all of the farming units in the Liuhe District. KCi is the land economic coefficient of the ith farming unit. aij is the yield-cost index of the jth crop on the ith farming unit. Aij is the maximum yield-cost index of the jth crop among all of the farming units. Yj is the actual yield of the jth crop. Cj is the actual cost of the jth crop.
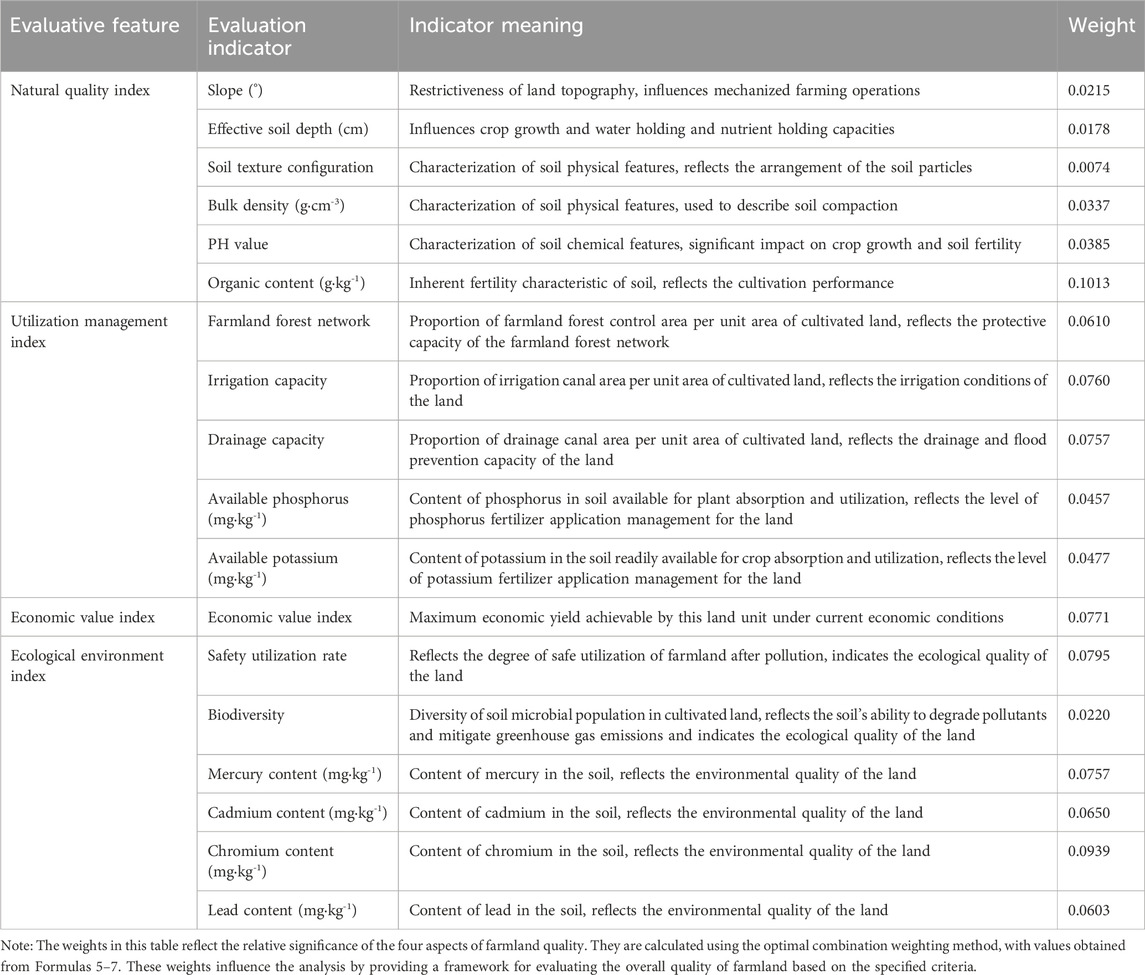
Table 1. Considering the natural quality index, utilization management index, economic value index and ecological environment index of the four one of the cultivated land comprehensive quality evaluation system.
3.1.2 Cultivated land quality comprehensive score calculation
This study uses the weighted index sum method (Kuang L. H. et al., 2022) to comprehensively evaluate the natural quality index, utilization management index, economic value index, and ecological environment index of each plot, and employs the natural breaks method to categorize them into five grades: Excellent, Good, Average, Bad, and Poor. The method for determining the weights in the evaluation index system is the optimal combination weighting method (Hammitt and Zhang, 2013; Liu Y. L. et al., 2022), which generally involves the following steps (Equations 5–7): (1) The expert scoring method, the analytic hierarchy process, the entropy weight method, and principal component analysis are used to determine the four types of weights and then construct the weight matrix WC; (2) The sum of squared deviations between standardized indicators is constructed pairwise and the sum of squared deviations matrix Z1 is constructed; (3) Based on the principle of maximizing the sum of squared deviations, the product of the weight matrix WC and the sum of squared deviations matrix Z1 are calculated, as in Equation 6, and then the maximum eigenvector of the resulting matrix is determined; and (4) The maximum eigenvector is normalized to obtain the correction coefficients for each weight, and then weighted calculations are performed to obtain the optimal combination weighting weights Wi.
where Iq is the qth quality indicator. Gi is the score of the ith indicator. Wi is the optimal combination weight for the ith indicator. J (WC) is the weight matrix WC’s objective function, which maximizes the sum of the squared deviations. gi is the standardized score of each indicator. n is the quantity of evaluation indicators. m is the quantity of the farming units.
3.2 Spatial autocorrelation analysis
3.2.1 Local spatial autocorrelation model
The local spatial autocorrelation model is one of the most widely used methods in theoretical geography, and it primarily identifies the clustering and differentiation characteristics of spatial units (Shaker, 2018). This study employed the local spatial autocorrelation model (Engen et al., 2002) to describe the spatial correlation characteristics between farmland patches and their adjacent patches in terms of land quality, simulating the spatial distribution of global farmland patch aggregation and differentiation. The specific calculation formula for the local indicators of spatial association (LISA) (Guo et al., 2013) is as follows Equations 8, 9:
Where Wij is the component in the spatial geographical matrix. xi and xj are the scores of the standardized quality index for individual units. S2 is the variance of the land quality coefficients.
The Moran’s Index (Moran’s I) ranges from [−1,1] (Chen and Shen, 2020). Depending on its sign, spatial autocorrelation can be described as high-high (HH) or low-low (LL) for positive correlations, and as high-low (HL) or low-high (LH) for negative correlations (Pang et al., 2023). Positive correlations indicate the spatial clustering of farmland patches, while negative correlations represent spatial differentiation (Das and Ghosh, 2017). In this study, the Z-test statistic, commonly referred to as the Zi standard score, was employed to determine the significance of global spatial autocorrelation. The Zi score quantifies the number of standard deviations a data point is from the mean, allowing for the assessment of spatial relationships. A Zi greater than 1.96 indicates a strong positive correlation between the observed values, suggesting that the overall spatial pattern of farmland patches exhibits spatial clustering. Conversely, a Zi less than −1.96 indicates a strong negative correlation, meaning that the overall spatial pattern demonstrates spatial differentiation. When the absolute value of Zi (|Zi|) is less than 1.96, it indicates that the spatial autocorrelation of farmland patches is not significant, and instead, they exhibit a random distribution in space.
3.2.2 Determination and improvement of geographical weight matrix
1. Determination of geographical weight matrix. The inverse distance spatial weight matrix is based on the premise that the strength of spatial autocorrelation effects depends on the distance between farmland patches (Carrijo and da Silva, 2017). Specifically, the influence of adjacent patches on an observed plot diminishes as the distance increases, which aligns with the first law of geography: “all spatial units are correlated, and the correlation between closer spatial units is stronger than that between farther spatial units.” This principle underpins the spatial autocorrelation analysis of cultivated land quality in this study. Traditional methods typically calculate spatial correlations using the distances between the centroids of farmland blocks, which may overlook the complexities introduced by varying plot sizes (Aghadadashi et al., 2019). For instance, when larger plots are adjacent to smaller ones, the centroid distance may not accurately reflect the quality correlations between these plots (Raty and Kangas, 2007). By employing an inverse distance spatial weight matrix, this study enhances the precision of the spatial autocorrelation results for farmland plots in Liuhe District using a Local Indicators of Spatial Association (LISA) model (Ferrari et al., 2021). This model serves as a foundational tool for optimizing the spatial layout of farmland protection across the entire region.
2. Improvement of the geographical weight matrix. Previous studies have often relied on centroid distances without sufficiently considering the correlation among farmland patches within specific threshold distance ranges (Li et al., 2021). The traditional construction process for inverse distance spatial weight matrices can be subjective in determining distance thresholds, which impacts the scientific rigor of optimizing farmland layouts (Krisztin and Piribauer, 2023). To address these limitations, this study enhances the inverse distance spatial weight matrix by incorporating the areas of farmland plots. This approach utilizes incremental spatial autocorrelation analysis to objectively establish distance thresholds, thereby improving the model’s accuracy. The specific steps involved are as follows:
① Improvement of the Inverse Distance Spatial Weight Matrix. To enhance accuracy, the area of farmland units in Liuhe District is normalized (Equation 10). This normalization adjusts for discrepancies in plot sizes, ensuring that the geographical weight reflects the relative influence of each plot more accurately.
where Mij denotes the elements of the land parcel area matrix M, Mjmax and Mjmin are the maximum and minimum areas of adjacent farming units within the threshold distance range for land unit i, respectively, and Mi is the area of land unit i. Within different distance threshold ranges, farmland unit x has multiple adjacent units. When farmland unit j has only one adjacent unit or when all adjacent units have equal areas (i.e., when Mjmax = Mjmin), Mij is set to 1. If farmland unit j has more than one adjacent unit and their areas are not equal, the standardized area is calculated according to Equation 10. Subsequently, the improved inverse distance spatial weight matrix is adjusted based on the calculated standardized areas of farmland units, as detailed in the following computational process (Equation 11):
where Wij is the component of the improved distance-weighted geographical weight matrix W, which has been modified by the standardized area of the farming unit to reflect the geographic influence of farming unit j on farming unit i.
② The optimal threshold distance is determined. The spatial layout optimization of farmland in this study is based on the overlay and coupling of the spatial autocorrelation results of the farmland’s natural quality, utilization management, economic value, and ecological environment indexes (Balthazar et al., 2015). Accurate simulation of the spatial autocorrelation results for each farmland quality index can only be achieved after determining the optimal threshold range. Given this, the incremental autocorrelation analysis method was used with the ArcGIS platform to simulate the spatial clustering and dispersion characteristics of farmland units across different threshold ranges. The variation in the Zi index was used to determine the optimal threshold distance. Generally, as the threshold distance gradually increases, the Zi index also increases, indicating an increasing spatial clustering tendency of the farmland units. Within a specific threshold distance range, if the Zi index exhibits a peak, the corresponding threshold distance represents the most significant distance for the spatial clustering process of the farmland units, and thus it is the optimal threshold distance.
3.3 Improved spatial autocorrelation significance test based on a spatial econometric model
In this study, we utilized the Geoda 1.6.7 platform to apply three spatial econometric models: the first-order spatial autocorrelation model, the geographical lag model, and the spatial error model, to analyze the spatial autocorrelation of farming parcels in Liuhe District (Ling et al., 2022). The primary objective of employing these models is to compare their regression parameters and identify the one that best fits the data. The first-order spatial autocorrelation model evaluates how the values of farming parcel attributes correlate with those of neighboring parcels, while the geographical lag model considers the influence of neighboring parcels on the value of a given parcel, and the spatial error model addresses potential spatial autocorrelation in the error terms. To assess the significance and reliability of the model results, we compared them using several statistical criteria, including the Akaike Information Criterion (AIC), Bayesian Information Criterion (BIC), Hannan-Quinn Criterion (HQC), log-likelihood (Log L) value, and Moran’s I index. Lower AIC and BIC values indicate a more suitable model, while higher Log L values reflect a better fit. Additionally, the Moran’s I index quantifies the spatial autocorrelation, confirming significant clustering or dispersion among farming parcels. By systematically comparing these models based on these criteria, we aim to identify the most appropriate model for capturing the spatial autocorrelation of farmland quality in Liuhe District.
3.4 Optimization of the spatial layout of farmland conservation
The differences in the quality among farmland units are caused by the continuous interactions of multiple factors, and each farmland unit responds differently to the same environmental changes (Xu et al., 2022). Therefore, the strategies for improving the farmland quality in the same plot can lead to significant variations in the quality of the neighboring farmland units (Song and Zhang, 2017). According to polarization theory, when farmland units are at the same polarization level, the farmland quality tends to be homogeneous, while at different polarization levels, the farmland quality tends to be heterogeneous (Liu W. et al., 2022). When the quality of a particular farmland unit changes, it has two distinct effects on the surrounding units: a diffusion effect that promotes and positively influences the farmland quality of the adjacent units, and a backflow effect that inhibits and negatively influences the farmland quality of the adjacent units. In this study, the geographic self-correlation of the natural quality index, utilization management index, economic value index, and ecological environment index were analyzed within the “three lines” of territorial spatial planning under the supervision of polarization theory. With the intention of suggesting practical solutions for maximizing the spatial arrangement of farmland conservation, the effects of changes in a particular farmland quality coefficient on the farmland quality of the adjacent units were evaluated.
4 Results
4.1 Geographical distribution features of farmland quality index
This research employs the natural quality index, utilization management index, economic value index, and ecological environmental index to construct a comprehensive farmland quality evaluation system. The farmland’s comprehensive quality score was determined using a weighted index based on standardized farmland categorization criteria. Figure 2 illustrates the geographical distribution of the four aforementioned dimensions of the farmland quality index.
The natural quality index exhibits a spatial distribution pattern of high values in the west and low values in the east. High-score regions, characterized by paddy fields and optimal farming conditions, are mainly situated around Chengqiao Street, Zhuzhen, and northern Ma’an Town. Low-score areas are primarily in Babaiqiao Town, central Ma’an Town, and the region between the hills and alluvial land in western Hengliang Town, and they are dominated by dryland farming and unfavorable natural conditions.
For the utilization management index, the cultivated land quality in Liuhe District shows a pattern of high density in the center and low density on the periphery. High-score regions are mostly in Ma’an Town, western Babaiqiao Town, and the intersection of Hengliang and Guabu Towns. These areas feature extensive irrigation canals, drainage facilities, and advanced management practices. In contrast, low-score areas are mainly in western Babaiqiao Town, the intersection of Zhuzhen and Ma’an Town, and Longchi Street. These areas are marked by inadequate field infrastructure and lower levels of agricultural practices, such as fertilization.
Regarding the economic value index, low-score land parcels cover a larger area than high-score ones, and the quality of land in Liuhe District is high in the central region and low on the borders. High-score regions, which are primarily in Ma’an Town, western Babaiqiao Town, and central Zhuzhen, consist mainly of black and heavy soil, offering higher agricultural economic benefits. Conversely, low-score regions are mainly in northern Ma’an Town, the junction of Chengqiao Street and Ma’an Town, and western Zhuzhen, and they are predominantly composed of mid-layer lime soil and loess. These areas have lower input intensity and agricultural economic sustainability, representing a high input-low output model with poor economic returns.
The ecological environment index reveals a distinctive geographical distribution in Liuhe District, with high-score farmland in the center and low-score farmland on the edges, and a larger area of high-score parcels. High-score areas are mainly concentrated in Ma’an Town, Longpao Town, and Yeshan Town, characterized by paddy fields and high soil biodiversity, promoting the effective decomposition of soil microorganisms and nutrient conversion. In contrast, low-score areas are mainly clustered in Zhuzhen, Chengqiao Street, Babaiqiao Town, Longchi Street, and the industrial park area in Hengliang Town. These regions have less diverse soils and face significant risks to the ecological integrity of cultivated land. In some areas, levels of heavy metals such as lead, cadmium, and chromium exceed the established limits.
4.2 Improved spatial autocorrelation analysis test results
4.2.1 Determination of the optimal threshold distance
The Incremental Geographical Autocorrelation Analysis tool in ArcGIS 10.2 was utilized to examine the spatial relationships and clustering patterns of farmland quality indexes in Liuhe District across various threshold distances. This tool is designed to analyze spatial autocorrelation by calculating the Moran’s I statistic at different distance thresholds. The Moran’s I statistic is a measure of spatial autocorrelation, indicating the degree to which similar values cluster together or disperse over space. By examining the spatial relationships at incremental distances, this tool allows for the identification of spatial patterns that may not be apparent at a single distance threshold, offering a more nuanced understanding of how farmland quality indexes are spatially distributed. The analysis started with a threshold distance of 150 m, incrementing by 100 m until reaching a final distance of 3,050 m. At each distance, the tool recalculates Moran’s I, identifying at which threshold distance the spatial clustering is most pronounced. This process helps determine the distance at which the spatial autocorrelation is strongest and provides insights into the geographic scale of clustering. The results are illustrated in Figure 3, highlighting how spatial correlations change with varying distances.
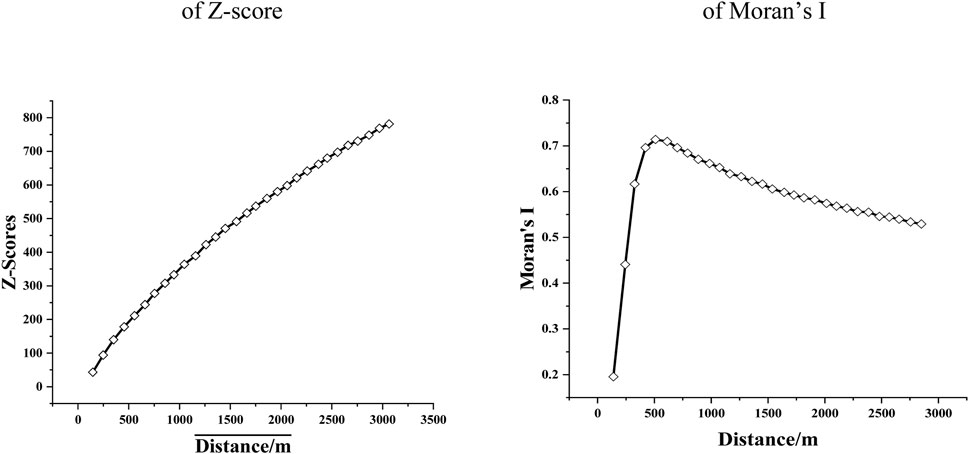
Figure 3. Results of incremental spatial auto-correlation analysis. (A) The results of incremental spatial autocorrelation analysis of Z-score (B) The results of incremental spatial autocorrelation analysis of Moran’s I.
Figure 3A shows a parabolic increase in the Z-score as the threshold distance extends from 100 to 3,050 m, rising from 44.23 at 150 m to 779.51 at 3000 m. Additionally, as the distance increases, the Z-score increment gradually diminishes. The absence of a peak value within the 150–3,050 m range suggests pre-existing clustering in the data. Figure 3B indicates a linear distribution of Moran’s I, characterized by a rapid rise followed by a gradual decline from 150 to 3,050 m. Moran’s I attains its maximum value of 0.73 at a threshold distance of 550 m. Beyond 550 m, Moran’s I gradually decreases, with the rate of decrease showing a declining trend. Moran’s I signifies the correlation between variables. From the incremental autocorrelation results, it can be inferred that the data exhibits the strongest correlation at a threshold distance of 550 m. Furthermore, the corresponding P-score for each threshold distance was consistently 0, confirming the accuracy and feasibility of the analysis results.
4.2.2 Improved spatial autocorrelation analysis test results
Utilizing the optimal threshold distance, we constructed an improved inverse distance geographical weight matrix (Wij) and a traditional inverse distance geographical weight matrix (wij) using ArcGIS 10.2 and Geoda 1.6.7 software. To conduct spatial fitting analysis, we employed a first-order spatial autoregressive model (FAR), a spatial autoregressive model (SAR), and a spatial error model (SEM). The fitness of the improved spatial autocorrelation model was evaluated using information criteria (AIC, BIC, HQ, and Log L) and Moran’s I value. The results of the simulated data for the three spatial models (FAR, SAR, and SEM), using cultivated land natural indices as an example, are shown in Table 2.
The information criteria and Moran’s I index results for the three spatial models (FAR, SAR, and SEM) are presented in Table 2, revealing noticeable differences between the improved inverse distance geographical weight matrix (Wij) and the traditional inverse distance geographical weight matrix (wij). With the exception of the Log L for the FAR model and the BIC for the SAR model, the other criteria information results and Moran’s I values under Wij are more reasonable. This indicates that the spatial error model aligns better with reality, validating the logic of the geographic self-correlation analysis using the improved inverse distance weight matrix.
4.3 Analyzing the moran dispersion diagram and Moran’s I
This study employed an improved spatial weight-based local spatial error model to analyze the geographic associations and variations between cultivated land units in Liuhe District. Figure 4 presents the Moran scatter plots for each index of cultivated land conditions. Overall, the Moran’s I scatter plots for all of the indexes are predominantly in the first and third quadrants, indicating that there is significant positive spatial autocorrelation for all four indexes. The scatter plots for the natural quality index and ecological environment index are closely aligned with the fitted line, whereas those for the utilization management index and economic value index are more dispersed. This indicates that the positive spatial correlation is stronger for the natural quality index and ecological environment index than that of the utilization management index and economic value index. This demonstrates that ecological and natural quality conditions exhibit a stronger intrinsic correlation than utilization and economic value conditions. Model significance tests reveal that the geographic self-correlation of the natural quality index, utilization management index, economic value index, and ecological environment index for Liuhe District’s farmland have Moran’s I values of 0.89, 0.67, 0.65, and 0.83, respectively. All four indexes approach 1, indicating a strong positive spatial correlation for Liuhe District’s cultivated land quality indicators. The strengths of correlation, in descending order, are the natural quality index, ecological environment index, utilization management index, and economic value index.
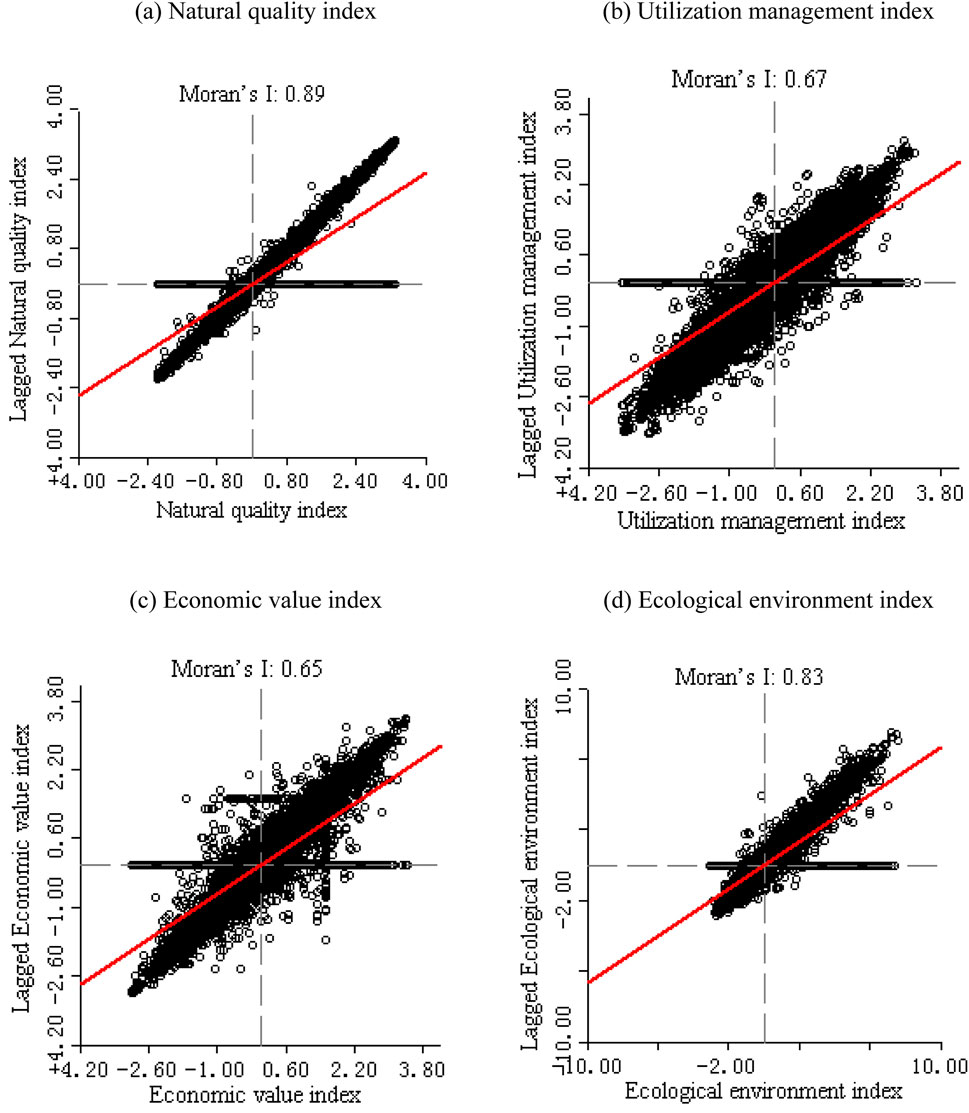
Figure 4. Moran’s I scatter plot of the farming units quality index in Liuhe District. (A) Natural quality index (B) Utilization management index (C) Economic value index (D) Ecological environment index.
4.4 Analysis of local geographical self-correlation
To capture the influence of neighboring units on the geographic characteristics of farmland’s natural quality, utilization, economic value, and ecological conditions within the optimal distance threshold, this study employed an improved inverse distance geographical weight matrix based on cultivated land area. The quality of farming parcels in Liuhe District was mapped using a local geographical self-correlation model (Figure 5). The research results indicate the presence of a high positive geographical self-correlation for the natural quality index, utilization management index, economic value index, and ecological environment index, all at a 95% confidence level. Table 3 provides the total area and percentage of each land category.
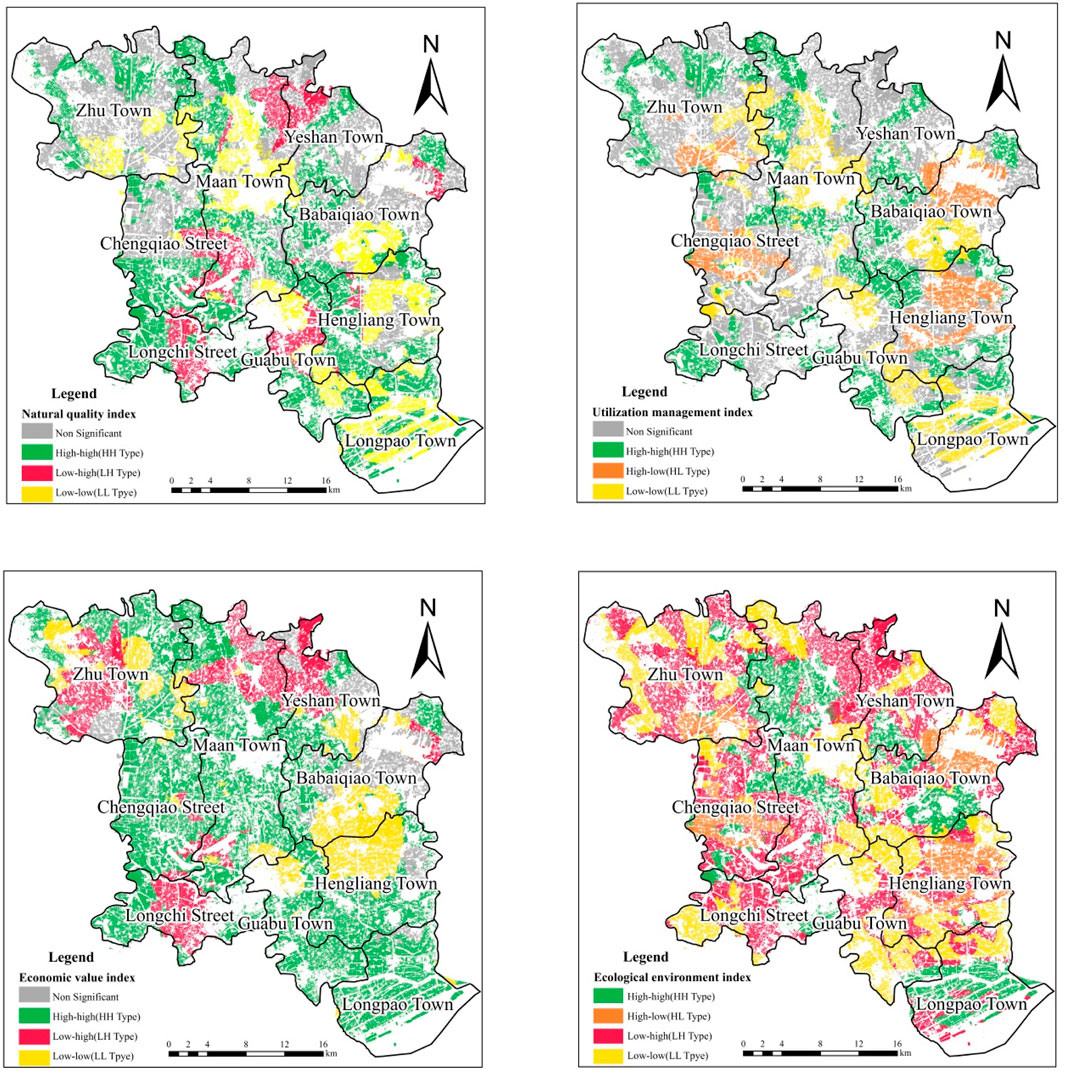
Figure 5. Local spatial aggregation geographical features of the farmland parcel quality index in Liuhe District.
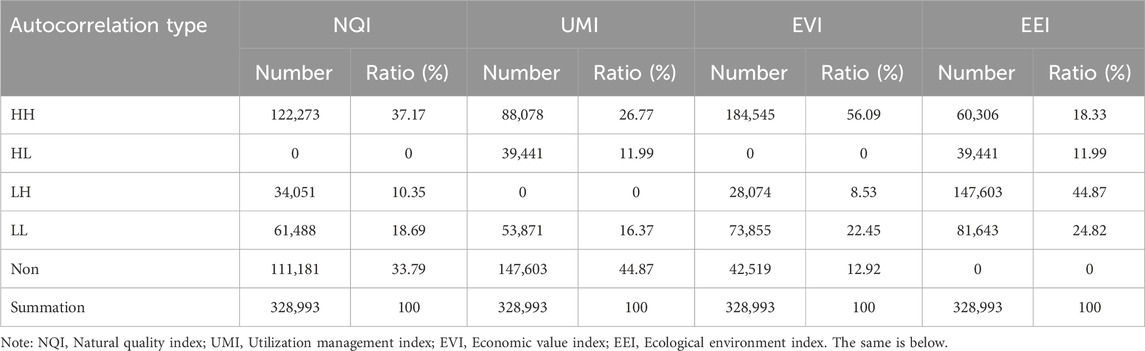
Table 3. Summary of local geographical self-correlation types and proportion of the farming parcel quality index in the Liuhe District.
4.4.1 Local spatial self-correlation of the farming parcels’ natural quality index
According to the simulation outcomes of the geographical self-correlation of the farming parcels natural quality index in the Liuhe District (Table 3; Figure 5A), the proportions of the HH-type and LL-type land parcels among the types of positive association are 37.17% and 18.69%, respectively. The HL-type and LH-type take up 0% and 10.35%, respectively, of the negative correlation styles. Overall, the positive correlation types are mainly concentrated in contiguous patterns in the central part of Liuhe, while the negative correlation types are scattered along the edges of the Liuhe District. The HH-type is particularly abundant in the western portion of Longchi Street, the southern portion of Chengqiao Street, and the junction of Ma’an Town and Babaiqiao Town. The middle region, specifically Longpao Town, Ma’an Town, and Hengliang Town, is where the LL-type is most prevalent. The LH-type can be observed along the eastern portion of Longchi Street, at the intersection of Chengqiao Street and Ma’an Town, in the central part of Guabu Town, and at the intersection of Ma’an Town and Yeshan Town.
The geographical features of the land parcels with greater quality are closely correlated with the spatial distribution of the positive correlation type of the cultivated land natural quality index in the Liuhe District. The positive correlation type is primarily found in the heart section of Liuhe, where the good inherent qualities of the cultivated land are prominent. The northern and southern zones, where the natural quality of the farmed land is typically minimal, have the highest amount of the negative correlation type. Among the positive correlation types, Hengliang Town stands out as having fertile soil, a good soil texture, an ideal effective soil depth, excellent water retention and fertility capabilities, strong land connectivity, a concentrated and contiguous distribution, and a higher level of natural quality. By contrast, among the negative correlation types, Yeshan Town and Longchi Street have poor soil texture and insufficient soil fertility. Some areas have uneven terrain, which is unfavorable for mechanized operations. The soil depth is inadequate, and the organic matter content is low. These areas are primarily located on both sides of the highways and far from the roads; they are thus heavily impacted by the cutting effect made by highways.
4.4.2 Local spatial self-correlation of the farming parcels’ utilization management index
According to the simulation results of the geographical self-correlation of the farming parcels’ management index in the Liuhe District (Table 3; Figure 5B), among the positive correlation types, the proportions of the HH-type and LL-type land parcels are 26.77% and 16.37%, respectively. The proportions of the HL-type and the LH-type, two types of negative correlation, are 11.99% and 0%, respectively. Overall, the positive correlation types are mainly concentrated in contiguous patterns in the central part of Liuhe, while the negative correlation types are scattered along the edges of Liuhe Town. Specifically, the HH-type is primarily distributed in the western part of Babaiqiao Town, the central part of Ma’an Town, and the southern part of Hengliang Town. The LL-type is primarily found in Longpao Town, Ma’an Town’s middle zone, and Babaiqiao Town’s southern region. The HL-type is mainly distributed in Zhuzhen Town, Babaiqiao Town, Chengqiao Street, and Hengliang Street in a clustered manner.
The location-based distribution of the positive self-correlation type of the Liuhe District farming parcels’ utilization management index significantly matches the geographic characteristics of the land parcels with the highest utilization management index. The southern part of Ma’an Town benefits from the Pingshan Lake water system, an abundance of water resources, dense irrigation and drainage channels in the fields, notable advantages in irrigation conditions, close proximity to villages, shorter distances for farming activities, facilitation of the input and output of agricultural production factors, and concentrated and contiguous cultivated land, all of which are suitable for large-scale automated agrarian production and other activities. The land parcels in Hengliang Town have smaller areas and less contiguity, severely impeding large-scale mechanized farming. There are multiple restrictive factors that limit the farming and utilization conditions, and the infrastructure for land utilization is not yet complete, resulting in a lower utilization management index. Although Gucheng Town has abundant water sources and good irrigation conditions, agricultural production is severely restricted due to its location in the Gangchong hilly area. Poor conditions exist for the use of farmland and unreasonable conditions exist for the laying of irrigation and drainage channels. The land parcels located in the north and away from both sides of the road, as well as those in the southern part of Dingbu Town, lack evident locational advantages, which severely reduces the output of agricultural production elements and shows inadequate levels of exploitation and management.
4.4.3 Local spatial self-correlation of the farming parcels’ economic value index
According to the simulation results of the geographical self-correlation of the farming parcels’ economic value index in the Liuhe District (Table 3; Figure 5C), among the positive correlation types, the proportions of the HH-type and LL-type land parcels are 56.09% and 22.45%, respectively. The proportions of the HL-type and the LH-type, two types of negative correlation, are 0% and 8.53%, respectively. Overall, the positive correlation types are mainly concentrated in contiguous patterns in the central part of Liuhe, while the negative correlation types are scattered along the edges of Liuhe Town. Specifically, the HH-type is primarily distributed in Ma’an Town, Chengqiao Street, and Guabu Town. The LL-type is mainly distributed at the intersection of Hengliang Town and Babaiqiao Town and in the central part of Zhuzhen Town. The LH-type is mainly found in the northern zone of Ma’an Town and Yeshan Town, the central part of Zhuzhen Town, and the eastern part of Longchi Street.
In the Liuhe District, the land parcels with a positive correlation with the economic value index are mainly distributed in high-value-added agricultural areas, along roadsides, and around Jinniu Lake. Among them, in the HH-type regions, Ma’an Town has predominantly medium-textured soil, good soil quality, fertility, and proximity to the center of the Liuhe District, which facilitates transportation and overall economic benefits. Longpao Town has a flat terrain in the riverine area, with several roads such as the Ningzhen Highway and Jiangbei Along-River Expressway crossing from east to west, enabling the efficient transportation of agricultural products and enhancing the economic benefits of farming parcels. The centrally positioned Ma’an Town benefits from its closeness to Liuhe’s city core and the Chengjia Lake water system, which assures irrigation and drainage while taking into account the town’s economic advantages. The land parcels located in the northern part of Ma’an Town and Yeshan Town, the central part of Zhuzhen Town, and the eastern part of Longchi Street do not have significant location advantages. These regions suffer from insufficient labor, fertilizer, and other material inputs per unit area of the field, relatively few crop varieties, and outdated field management technology. The improvement of the economic advantages of the farmland parcels is greatly hampered by the low input and poor output production style.
4.4.4 Local spatial self-correlation of the farming parcels’ ecological environment index
According to the simulation results of the geographical self-correlation of the farming parcels’ ecological environment index in the Liuhe District (Table 3; Figure 5D), among the positive correlation types, the proportions of the HH-type and LL-type land parcels are 18.33% and 24.82%, respectively. The proportions of the HL-type and the LH-type, two types of negative correlation, are 11.99% and 44.87%, respectively. Overall, the positive correlation types are predominantly concentrated in contiguous areas in the central part of the Liuhe District, while the negative correlation types exhibit a scattered distribution along the edges of Liuhe Town. The HH-type land parcels are primarily found in Longpao Town, the western part of Ma’an Town, and the southern section of Babaiqiao Town. The locations around Zhuzhen Town, the Hengliang-Guabu-Ma’an region, and the western portion of Longchi Street are where the LL-type land lots are primarily found. The HL-type land parcels are primarily located in the eastern section of Babaiqiao Town, the central area of Hengliang Town and Chengqiao Street, and the southern part of Zhuzhen Town. The LH-type land parcels are mainly distributed in areas far from the highways in Zhuzhen Town, the northern part of Yeshan Town, and the Chengqiao Street-Longchi Street area, and they are sporadically distributed in the eastern part of Babaiqiao and the southern part of Longpao Town.
The Liuhe District’s areas with positive self-correlation types for the ecological environment index are mainly found near rivers, lakes, and other bodies of water and away from industrial parks and roadways. In the HH-type areas, Longpao Town exhibits a high soil microbial diversity, facilitating effective biological nitrogen fixation and improving the microenvironment of the soil. Moreover, these areas are spatially distant from heavy industrial zones, resulting in lower levels of heavy metal pollution. In some regions, an ecological grid has even been established, indicating prominent ecological quality. In the eastern part of Babaiqiao Town, which is near the eastern industrial zone in the Liuhe District, the industrial pollution is severe, with some areas exhibiting excessive levels of heavy metals such as cadmium, chromium, and lead, posing a significant threat to the ecosystem of the farmland parcels. There are few soil microbial species in plots far from the road in Zhuzhen Town, which hinders decomposition of soil macromolecules. Consequently, these areas have lower levels of soil structure and fertility, resulting in poorer ecological and environmental quality.
4.5 Layout and optimization strategy of cultivated land protection zoning based on local spatial autocorrelation
4.5.1 Cultivated land protection zoning layout
According to theoretical geography, there exist interdependencies and potential diffusion or backflow effects between different regional units, which may reduce or amplify the quality differences among adjacent units (Zhang W. H. et al., 2022). In this study, the geographic autocorrelation of agricultural land quality’s positive correlation types was interpreted as a spatial diffusion effect, while the negative correlation types was interpreted as a backflow effect (Yuan et al., 2018). Among them, the HH-type represents spatial clusters of high-quality land where the land quality tends to be homogeneous, making it a core protection zone where all non-agricultural construction should be prohibited. The LL-type, on the other hand, reflects geographical clusters of low-quality land, where land quality also tends to be uniform; therefore ecological afforestation and grassland restoration should be carried out in a staged manner depending on the endowment of land quality. This type of land serves as an ideal area for non-agricultural development. The HL-type represents convex areas that typically exhibit a spatial distribution pattern where high-quality land is surrounded by low-quality land. Under the influence of backflow effects, the high-quality land in this area is prone to assimilation with adjacent low-quality land, resulting in the degradation of the LL-type. The LH-type is characterized by concave regions with a geographic distribution form in which high-quality land encircles low-quality land. The low-quality land in these areas is prone to assimilation with nearby high-quality land and becoming HH-type land due to the influence of diffusion processes. The specific zoning results and optimization strategies for each type are shown in Figure 6 and Table 4.
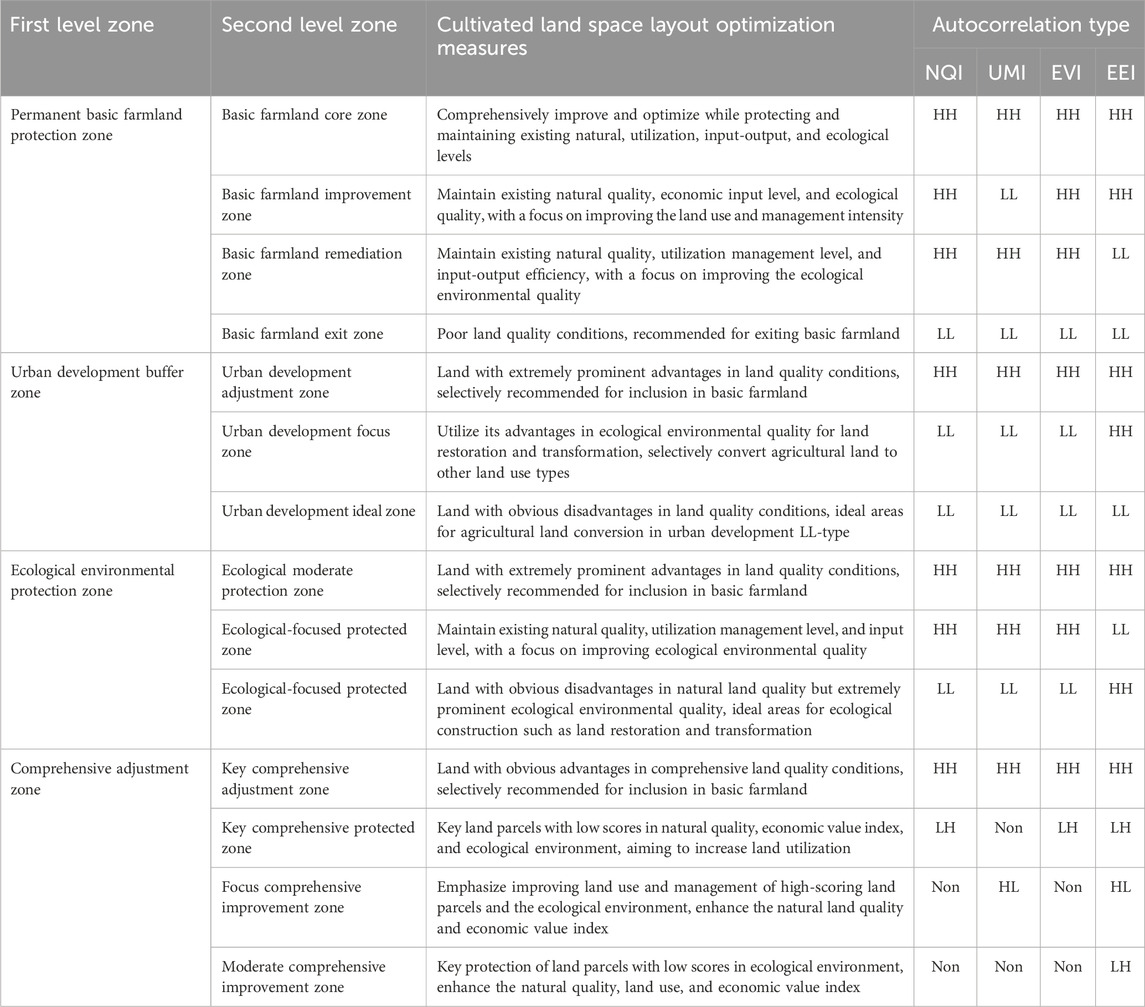
Table 4. Optimization strategy of cultivated land spatial layout in Liuhe District based on the combination of spatial autocorrelation analysis and territorial planning.
In this study, we analyzed the geographical self-correlations of the farming parcel quality within the boundaries of the permanent basic farmland, the expansion of cities, and ecological redlines based on regional development theory and practice while taking into consideration the boundary-drawing results of the “three lines” in the territorial spatial planning of the Liuhe District. The 14 combined types resulting from the overlay of the spatial associations of the various land indices were classified into four primary categories: permanent basic farmland protection zones, urban development buffer zones, ecological environmental protection zones, and comprehensive adjustment zones. Further refinement into 14 secondary categories was conducted based on the difficulty and cost of optimizing the land quality. Strategies for optimizing the spatial land layout were developed according to the different types of spatial effects. This study reveals that the optimal spatial land layout scheme based on the “three lines” spatial planning demarcation results not only ensures the successful execution of terrestrial geographic planning, but also offers methods for optimizing the spatial land layout. It increases the area of permanent basic farmland by 1234.15 hm2, with a grade improvement of 1.45.
4.5.2 Strategy for optimizing the cultivated land protection zoning layout
The outcomes regarding territorial space planning were incorporated into this study’s research framework to optimize the layout of farmland by considering the geographic self-correlation of cultivated land quality. A thorough analysis of the four-in-one geographic self-correlation system, which takes into account the natural, utilization, economic, and ecological conditions, was conducted on the parcels that fell within the basic farmland red line, urban expanding line, and ecological red line established by national geographic planning. In this study, the protection zones for arable land were divided again, and the goals and challenges of optimizing the layout in each zone were reassessed. The key focus areas and optimization strategies for each zone are discussed below.
1. Permanent basic farmland protection zone: It is primarily distributed in the northern part of Hengliang Town, the central part of Zhuzhen Town, and the intersection of Babaiqiao Town and Ma’an Town. It has a total area of 16212.62 hm2 and accounts for 83.90% of the total area. The parcels within the permanent farmland protection zone not only fall within the boundary of the basic arable land as determined by the territorial spatial planning in Liuhe District, but also exhibit strong spatial diffusion effects in all of the farmland quality indices, indicating overall high-quality and barrier-free conditions. Among them, the basic farmland core zone, a highly concentrated area with superior natural quality, a high level of infrastructure completeness, a large economic value index, and excellent ecological environmental quality, should receive top priority for farmland preservation. The parcels within the basic farmland improvement zone and basic farmland remediation zone exhibit relatively weak spatial clustering advantages in terms of the utilization management level and ecological environmental quality, and therefore land quality improvement techniques focusing on field management facility construction and ecological remediation projects should be implemented in these zones, respectively. The parcels within the basic farmland exiting zone exhibit weakened advantages in farmland quality, utilization management level, economic values, and ecological environmental quality. Simultaneously, they exhibit a strong negative backflow effect, which affects the farmland quality of the surrounding parcels. These parcels should be withdrawn from the basic farmland in an orderly manner through land ownership transfer. In summary, the parcels within this region belong to the category of permanent basic farmland due to their prominent farmland quality advantages and non-agricultural construction activities should be prohibited in these parcels. For the parcels with significant negative spatial clustering effects and poor farmland quality, effective monitoring, withdrawal, and transfer through the basic farmland management platform should be implemented to prevent damage to the basic farmland.
2. Urban development buffer zone: It is primarily distributed in the central area of Liuhe County in Ma’an Town, the northern area around Jinniu Lake in Hengliang Town, and the southern area around the center of Babaiqiao Town. It makes up 6.49% of the total area and has a total size of 1255.08 hm2. The urban development buffer zone falls within the urban expansion boundary determined by the territorial planning in Liuhe District. The farmland is adjacent to the urban center and has prominent location advantages, but it exhibits obvious disadvantages in terms of the farmland quality. Among them, the farmland within the urban development adjustment zone has a superior farmland quality, and all of the farmland quality indices exhibit strong positive spatial diffusion effects. It is recommended to selectively include these parcels in the category of basic farmland. The parcels within the urban development focus zone exhibit strong positive spatial diffusion effects in terms of ecological environmental quality. These parcels should be improved through measures such as land reclamation for afforestation and grassland restoration projects to enhance the ecological quality of the surrounding parcels. The parcels within the urban development ideal zone, located near the urban periphery, exhibit strong negative spatial reflow effects for all of the farmland quality indices, highlighting their own poor farmland quality and causing a decline in the farmland quality in the surrounding parcels. These parcels should be given top priority for urban development and occupation. In conclusion, the farmland quality in the urban development buffer zone has declined, and all of the farmland quality indices exhibit strong negative spatial reflow effects. This zone offers the perfect location for urban growth.
3. Ecological environmental protection zone: It is primarily distributed in Longpao Town, the western part of the town center in Ma’an Town, and the western part of Babaiqiao. With a total size of 101.63 hm2, it makes up 0.53% of the entire area. The ecological environmental protection zone falls within the ecological red line mapped by the geographic planning of the Liuhe District, and the parcels exhibit prominent advantages in terms of the ecological environment. It is mainly distributed along rivers, lakes, and highways. Among them, the parcels within the ecological moderate protection zone exhibit prominent farmland quality advantages, and all of the farmland quality indices exhibit significant positive spatial diffusion effects. It is recommended to selectively include these parcels in the category of basic farmland. In terms of natural quality, field management, and economic output, the plots within the ecologically focused protection zone show considerable advantages, while the ecological environment index display a strong negative geographical backflow impact. It is recommended to improve their ecological environmental quality through a series of ecological remediation projects such as on-field ecological protection and heavy metal degradation techniques. The parcels within the ecological key protection zone exhibit poor farmland quality, and all of the quality indices exhibit strong negative spatial reflow effects. Due to the limited farmland productivity, the use of ecological afforestation and grassland restoration projects should be actively explored to form a grid for high-quality ecological protection in Liuhe. In summary, the parcels within the ecological environmental protection zone exhibit poor farmland quality, and all of the farmland quality indices exhibit strong negative reflow effects. These parcels should be selectively withdrawn in an orderly manner through land reclamation for afforestation and grassland restoration projects to fully realize their ecological protection value.
4. Comprehensive adjustment zone: In the Liuhe District’s territorial spatial design, the parcel of this zone lies outside the “three lines” delineation. It makes up 9.08% of the whole space and has an area of 1755.09 hm2; this zone is primarily found in Chengqiao Town, Zhuzhen Town’s eastern section, and Yeshan Town’s eastern section. Among them, the parcels within the key comprehensive adjustment zone exhibit superior farmland quality, and all of the farmland quality indices exhibit strong positive diffusion effects. These plots should be selectively categorized as basic farming land. Farmland units within the key comprehensive protected zone, focus comprehensive improvement zone, and moderate comprehensive improvement zone have relatively weaker advantages in terms of farmland quality, with more complex obstacles affecting farmland quality. The mechanisms and effects of the various limiting factors should be identified to achieve a transition of all of the farmland quality indices to HH-type. The specific measures include determining the sequence of remediation for each parcel based on the mechanisms, quantity, and remediation cost of the limiting factors; adopting measures such as soil transfer and improvement in parcels with poor farmland quality; accelerating the construction of field roads and ditches in parcels with inadequate infrastructure; optimizing land management models through measures such as farmland circulation and substitution in parcels with poor input-output levels; and actively exploring new technologies for soil pollution remediation and improving soil pollution control models in parcels with poor ecological conditions.
5 Discussion
5.1 Four-in-one farmland quality spatial autocorrelation analysis system
This study presents a novel, integrated approach to evaluating farmland quality by constructing a four-dimensional spatial autocorrelation system. Our approach expands the theoretical framework of farmland quality by coupling the spatial autocorrelation characteristics of natural quality, utilization management, economic value, and ecological environment indices. Unlike previous research that relied heavily on expert evaluation methods (Huang et al., 2017), our study provides a more comprehensive and data-driven perspective on optimizing farmland spatial layout. The coupling of farmland quality and spatial autocorrelation features is a significant advancement over existing methods that often prioritize weighted indices for assessing natural quality (Rotches-Ribalta et al., 2021), locational conditions (Baek et al., 2022), and economic value (Kuang Y. et al., 2022) without adequately considering the spatial autocorrelation characteristics of these indices.
Our findings reveal a high degree of spatial clustering in areas with positive spatial autocorrelation of the farmland quality index, particularly in regions with high-quality land parcels. This observation aligns with previous studies (Cheng et al., 2023). Specifically, regions with a positive spatial autocorrelation of natural quality are predominantly located in the central area of the Lihe District, where the natural advantages of farmland are most pronounced. Conversely, negative spatial autocorrelation is prevalent in the northern and southern areas, where natural quality is lower. The positive spatial autocorrelation of the utilization management index is concentrated in areas with abundant water resources, dense irrigation and drainage channels, and close proximity to agricultural activities. Negative spatial autocorrelation, on the other hand, is found in hill and ravine areas with poor infrastructure and accessibility. Similarly, the economic value index shows positive spatial autocorrelation in high-value agricultural zones and areas near roads, whereas negative spatial autocorrelation is associated with plots that suffer from insufficient labor, fertilizers, pesticides, and outdated management techniques. For the ecological environment index, positive spatial autocorrelation is evident in regions with diverse soil microbiota and minimal industrial and road pollution, while negative spatial autocorrelation occurs near industrial zones, where soil biodiversity is low and heavy metal contamination is significant. Consistent with prior research (Luo et al., 2022), our study underscores the critical role of spatial autocorrelation characteristics in optimizing farmland spatial layout, enhancing precise management of farmland protection, and ensuring food security. Accurate identification of these characteristics can harness the spatial diffusion effects of farmland quality while mitigating its potential negative spillover effects, ultimately enhancing overall farmland quality.
A noteworthy contribution of our study is the inclusion of the ecological environment quality as a fourth dimension in the spatial autocorrelation analysis of farmland quality. Previous studies have largely focused on natural quality, utilization management, and economic value, often overlooking the spatial autocorrelation characteristics of ecological environment quality. By integrating this dimension, we extend the theoretical framework of farmland spatial autocorrelation analysis and provide a more holistic approach to farmland protection. This integration allows for the accurate identification of ecological risk areas, thereby preventing ecological degradation and promoting sustainable farmland management. In conclusion, our study not only broadens the conceptualization of farmland quality but also offers practical insights into its spatial optimization. By incorporating the ecological environment quality into the analysis, we provide a comprehensive approach that addresses both the economic and ecological aspects of farmland management.
5.2 Combination of farmland quality spatial autocorrelation with territorial spatial planning
This study integrates territorial spatial planning with the spatial autocorrelation characteristics of farmland quality, enhancing both the theoretical understanding and practical applications for optimizing spatial layouts. This integration is significant for improving the territorial spatial planning system, strengthening farmland protection, and accelerating the modernization of agriculture and rural areas. Territorial spatial planning divides rural areas into production, living, and ecological spaces. However, existing spatial autocorrelation analyses of farmland quality have often overlooked ecological environmental factors (Das and Ghosh, 2017), failing to effectively align with territorial spatial planning frameworks (Yuan et al., 2018; Fahr and Sunde, 2006). Our research addresses this gap by coupling the spatial autocorrelation characteristics of natural quality, utilization management, economic value, and ecological environment. We then align these characteristics with the “three-line” delineation results of territorial spatial planning. Specifically, we categorize farmland within basic farmland protection zones into core, improvement, remediation, and exit zones. Land within urban construction spaces is categorized into urban development adjustment, emphasis, and ideal zones. Land within ecological protection zones is divided into moderate, emphasis, and key construction protection zones. Land outside the three-line delineation is classified into key comprehensive adjustment, protection, emphasis remediation, and moderate adjustment zones. This comprehensive categorization facilitates the precise management of farmland quality by spatially aligning farmland characteristics with appropriate planning and protection strategies. It allows for targeted interventions tailored to the unique needs and potentials of different areas, thereby optimizing the use of land resources and enhancing overall land management efficiency. Moreover, our study underscores the importance of spatial autocorrelation characteristics in the context of territorial spatial planning. Accurate identification and understanding of these characteristics enable policymakers and planners to harness the benefits of spatial diffusion effects while mitigating negative spillover effects. This approach not only enhances farmland quality but also supports sustainable development goals by balancing economic, social, and environmental objectives.
5.3 Improvement of the spatial autocorrelation model
In this study, we introduce an enhanced spatial autocorrelation analysis method for cultivated land quality, which is based on an improved spatial weight matrix. This method provides a more accurate and scientific approach to describing and analyzing the spatial clustering characteristics of cultivated land quality. While spatial autocorrelation models have been widely used in studies on cultivated land quality protection (Chen and Shen, 2020; Carrijo and da Silva, 2017; Cheng et al., 2016), the traditional focus has predominantly been on the spatial distance between patches when determining the spatial weight matrix. This conventional approach often neglects the influence of patch area on the estimation results, potentially compromising the accuracy of spatial simulation outcomes. To address this limitation, we optimized the spatial weight matrix by identifying the optimal threshold distance. This optimization significantly enhances the precision and scientific rigor of the spatial autocorrelation analysis. Utilizing Moran’s I and both global and local geographic autocorrelation models, we investigated the regional aggregation of parcel quality and its implications for improving farmland layout. To evaluate the significance of geographical self-correlation, we applied the information criteria (AIC, BIC, HQ, and Log L) as described by Shaker and Liu, and used Moran’s I values obtained from previous studies on 328,993 farmland units in the Liuhe District (Liu Y. L. et al., 2022). These methodological improvements contribute to a more nuanced understanding of farmland quality and provide valuable insights for optimizing farmland layout. Our findings underscore the importance of considering both spatial distance and patch area in the spatial weight matrix, which leads to more accurate spatial autocorrelation analyses. This refined approach not only improves the precision of spatial simulations but also offers robust support for effective farmland management and protection strategies. The enhanced accuracy of spatial analysis enables more informed decision-making, ultimately leading to better management practices that can enhance farmland quality and sustainability.
5.4 Limitations of this study
While our research provides valuable insights, it is important to acknowledge several limitations. First, although we developed a series of land remediation strategies based on the geographic distribution of farmland quality and spatial spillover effects, improving farmland quality is a long-term and gradual process. Our study does not fully consider how enhancements in the quality of one plot may influence the quality of adjacent plots over time. Future research should implement comprehensive, long-term assessment methods or utilize panel data to investigate this dynamic more thoroughly. Second, the definition of farmland quality is continuously evolving. In this study, we established a four-dimensional framework for evaluating farmland quality based on current production needs. However, it remains uncertain whether this framework will adequately address future requirements for farmland quality. Future studies should incorporate more comprehensive theoretical perspectives to deepen the understanding of farmland quality and progressively enhance the evaluation system. Additionally, there is a need to develop a multidimensional spatial autocorrelation analysis framework specifically tailored to farmland quality. Although these limitations do not undermine the significance of our findings, they highlight opportunities for advancing and refining methodologies used in farmland spatial planning and optimization in future research. Addressing these constraints can contribute to a more nuanced understanding of farmland quality and pave the way for more effective and robust land management and conservation policies.
6 Conclusion
Based on the outcomes of the “three lines” delineation of the land space planning of two belts, two wedges, two axes, and two cities in the Liuhe District, in this study we constructed an improved local geographic four-in-one self-correlation model to analyze the spatial agglomeration distribution features of arable land quality indicators in the permanent farmland conservation red line, urban expanding line, and ecological red line. The following are the research’s principal findings:
(1) This study developed a four-in-one spatial autocorrelation evaluation system for assessing the quality of farmland parcels based on natural quality, field management, economic input and output, and ecological environment, and it also examined the spatial distribution characteristics of the quality level of cultivated land. The natural quality index indicated a spatial distribution where the west was high while the east is low. Utilization management, economic value, and ecological environment all displayed the characteristics of a geographical distribution that is high in the middle with a low level on the periphery.
(2) In terms of spatial self-correlation, the vast majority of the geographical self-correlation types of farming parcels in Liuhe District are favorable. Both positive and negative correlation types were congruent with the spatial distribution of high- and low-quality farmland. The natural quality index, utilization management index, economic value index, and ecological environment index—all of which were geographical aggregation features—displayed Moran’s I values of 0.89, 0.67, 0.65, and 0.83.
(3) Based on the combination the territorial spatial planning with geographical self-correlation analysis, the area of permanent basic farmland increased by 123.15 hm2 and the quality grade was enhanced by 1.45. According to the difficulty and the cost of remediation, the parcels in Liuhe District were divided into 14 second-level classifications and four first-level classifications, including the permanent basic farmland protection zone, the urban development buffer zone, the ecological environmental protection zone, and the comprehensive adjustment zone. The permanent basic farmland protection zone’s total quality is the best of these, and it shows a high positive spatial association. It should be the focal point of cultivated land conservation, and non-agricultural building should be prohibited. The urban development buffer zone’s cultivated land is of poor overall quality, but its locational advantage is the most pronounced. The substantial negative spatial backflow that the cultivated land quality index displays makes this an excellent area for urban growth. The total quality disadvantage of the farmed land here is more apparent, while the ecological circumstances are more noticeable in the plots located in the ecological environmental protection zone. To create a global ecological network based on the ecological red line, projects to convert cropland to forests and grasslands should be carried out in a systematic way. The variables restricting the quality of the cultivated land are more complex in the plots situated in the comprehensive adjustment zone. Field remediation initiatives should be energetically implemented on the basis of fully identifying the mechanisms of the impeding factors in order to encourage the conversion of these plots into long-term basic farmland.
Data availability statement
The raw data supporting the conclusions of this article will be made available by the authors, without undue reservation.
Author contributions
HS: Conceptualization, Formal Analysis, Investigation, Software, Writing–original draft. GG: Funding acquisition, Resources, Supervision, Writing–review and editing. LJ: Funding acquisition, Project administration, Resources, Supervision, Writing–review and editing. ZC: Data curation, Formal Analysis, Investigation, Validation, Writing–review and editing.
Funding
The author(s) declare financial support was received for the research, authorship, and/or publication of this article. This research was supported by the National Social Science Fund Key project (Grant No. 20AGL024), the National Social Science Fund project (Grant No. 22BGY003) and the National Natural Science Foundation of China (Grant No. 42471301).
Conflict of interest
The authors declare that the research was conducted in the absence of any commercial or financial relationships that could be construed as a potential conflict of interest.
Publisher’s note
All claims expressed in this article are solely those of the authors and do not necessarily represent those of their affiliated organizations, or those of the publisher, the editors and the reviewers. Any product that may be evaluated in this article, or claim that may be made by its manufacturer, is not guaranteed or endorsed by the publisher.
References
Aghadadashi, V., Molaei, S., Mehdinia, A., Mohammadi, J., Moeinaddini, M., and Bakhtiari, A. R. (2019). Using GIS, geostatistics and Fuzzy logic to study spatial structure of sedimentary total PAHs and potential eco-risks; an Eastern Persian Gulf case study. Mar. Pollut. Bull. 149, 110489. doi:10.1016/j.marpolbul.2019.110489
Badenhausser, I., Gouat, M., Goarant, A., Cornulier, T., and Bretagnolle, V. (2012). Spatial autocorrelation in farmland grasshopper assemblages (orthoptera: acrididae) in western France. Environ. Entomol. 41, 1050–1061. doi:10.1603/en11256
Baek, S., Yoon, H., and Hahm, Y. (2022). Assessment of spatial interactions in farmland abandonment: a case study of Gwangyang City, Jeollanam-do Province, South Korea. Habitat Int. 129, 102670. doi:10.1016/j.habitatint.2022.102670
Balthazar, V., Vanacker, V., Molina, A., and Lambin, E. F. (2015). Impacts of forest cover change on ecosystem services in high Andean mountains. Ecol. Indic. 48, 63–75. doi:10.1016/j.ecolind.2014.07.043
Carrijo, T. B., and da Silva, A. R. (2017). Modified Moran's I for small samples. Geogr. Anal. 49, 451–467. doi:10.1111/gean.12130
Chen, L., Song, G., and Zou, C. (2016). Zoning of protected cultivated land based on improved local indicators of spatial association. Resour. Sci. 38, 1871–1882. doi:10.18402/resci.2016.10.05
Chen, Y., Yao, M., Zhao, Q., Chen, Z., Jiang, P., Chen, D., et al. (2021b). Delineation of a basic farmland protection zone based on spatial connectivity and comprehensive quality evaluation: a case study of Changsha City, China. Land Use Policy 101, 105145. doi:10.1016/j.landusepol.2020.105145
Chen, Y. H., and Shen, T. J. (2020). Unifying conspecific-encounter indexvand Moran's I index. Ecography 43, 1902–1904. doi:10.1111/ecog.05281
Chen, Y. M., Yao, M. R., Zhao, Q. Q., Chen, Z. J., Jiang, P. H., Li, M. C., et al. (2021a). Delineation of a basic farmland protection zone based on spatial connectivity and comprehensive quality evaluation: a case study of Changsha City, China. Land Use Policy 101, 105145. doi:10.1016/j.landusepol.2020.105145
Cheng, G., Zeng, X. K., Duan, L., Lu, X. P., Sun, H. C., Jiang, T., et al. (2016). Spatial difference analysis for accessibility to high level hospitals based on travel time in Shenzhen, China. Habitat Int. 53, 485–494. doi:10.1016/j.habitatint.2015.12.023
Cheng, Q., Jiang, P., Cai, L., Jinxia, S., Zhang, Y., Wang, L., et al. (2017). Delineation of a permanent basic farmland protection area around a city centre: case study of Changzhou City, China. Land Use Policy 60, 73–89. doi:10.1016/j.landusepol.2016.10.014
Cracolici, M. F., Cuffaro, M., and Nijkamp, P. (2010). The measurement of economic, social and environmental performance of countries: a novel approach. Soc. Indic. Res. 95, 339–356. doi:10.1007/s11205-009-9464-3
Das, M., and Ghosh, S. K. (2017). Measuring Moran's I in a cost-efficient manner to describe a land-cover change pattern in large-scale remote sensing imagery. Ieee J. Sel. Top. Appl. Earth Observations Remote Sens. 10, 2631–2639. doi:10.1109/jstars.2017.2660766
Engen, S., Lande, R., and Saether, B. E. (2002). Migration and spatiotemporal variation in population dynamics in a heterogeneous environment. Ecology 83, 570–579. doi:10.2307/2680036
Fahr, R., and Sunde, U. (2006). Regional dependencies in job creation: an efficiency analysis for Western Germany. Appl. Econ. 38, 1193–1206. doi:10.1080/00036840600685140
Ferrari, G., Ai, P., Alengebawy, A., Marinello, F., and Pezzuolo, A. (2021). An assessment of nitrogen loading and biogas production from Italian livestock: a multilevel and spatial analysis. J. Clean. Prod. 317, 128388. doi:10.1016/j.jclepro.2021.128388
Gao, B. B., Lu, A. X., Pan, Y. C., Huo, L. L., Gao, Y. B., Li, X. L., et al. (2017). Additional sampling layout optimization method for environmental quality grade classifications of farmland soil. Ieee J. Sel. Top. Appl. Earth Observations Remote Sens. 10, 5350–5358. doi:10.1109/jstars.2017.2753467
Guo, G., and Han, X. (2021). Spatial layout optimization of basic farmland considering food security and cultivated land quality at county scale. Trans. Chin. Soc. Agric. Eng. Trans. CSAE 37, 252–260. doi:10.11975/j.issn.1002-6819.2021.07.031
Guo, L., Du, S. H., Haining, R., and Zhang, L. J. (2013). Global and local indicators of spatial association between points and polygons: a study of land use change. Int. J. Appl. Earth Observation Geoinformation 21, 384–396. doi:10.1016/j.jag.2011.11.003
Hammitt, J. K., and Zhang, Y. F. (2013). Combining experts' judgments: comparison of algorithmic methods using synthetic data. Risk Anal. 33, 109–120. doi:10.1111/j.1539-6924.2012.01833.x
Han, S., Guo, G., and Shi, Y. (2022). Optimization of the spatial layout of arable land protection using “Three Lines” delineation of territorial spatial planning and spatial autocorrelation attributes of arable land quality. Trans. Chin. Soc. Agric. Eng. Trans. CSAE 38, 237–248. doi:10.11975/j.issn.1002-6819.2022.19.026
Huang, Z. Z., Chen, R. S., Xu, D., and Zhou, W. (2017). Spatial and hedonic analysis of housing prices in Shanghai. Habitat Int. 67, 69–78. doi:10.1016/j.habitatint.2017.07.002
Huo, K., Ruan, Y. F., Fan, H. Z., Guo, C. H., and Cai, H. (2022). Spatial-temporal variation characteristics of cultivated land and controlling factors in the Yangtze River Delta region of China. Front. Environ. Sci. 10, 871482. doi:10.3389/fenvs.2022.871482
Ippolito, T. A., Herrick, J. E., Dossa, E. L., Garba, M., Ouattara, M., Singh, U., et al. (2021). A comparison of approaches to regional land-use capability analysis for agricultural land-planning. Land 10, 458. doi:10.3390/land10050458
Krisztin, T., and Piribauer, P. (2023). A Bayesian approach for the estimation of weight matrices in spatial autoregressive models. Spat. Econ. Anal. 18, 44–63. doi:10.1080/17421772.2022.2095426
Kuang, L. H., Ye, Y. C., Zhao, X. M., Guo, X., and Xie, W. (2022b). Delineation of basic farmland based on the quality and spatial stability: a case study of Yingtan City, China. Environ. Sci. Pollut. Res. 29, 88737–88750. doi:10.1007/s11356-022-21982-5
Kuang, Y., Yang, J., and Abate, M.-C. (2022c). Farmland transfer and agricultural economic growth nexus in China: agricultural TFP intermediary effect perspective. China Agric. Econ. Rev. 14, 184–201. doi:10.1108/caer-05-2020-0076
Kuang, Y. P., Yang, J. L., and Abate, M. C. (2022a). Farmland transfer and agricultural economic growth nexus in China: agricultural TFP intermediary effect perspective. China Agric. Econ. Rev. 14, 184–201. doi:10.1108/caer-05-2020-0076
Kuethe, T. H., Hubbs, T., and Morehart, M. (2014). Farmland returns and economic conditions: a FAVAR approach. Empir. Econ. 47, 129–142. doi:10.1007/s00181-013-0730-5
Li, L., Tang, H., Lei, J., and Song, X. (2022). Spatial autocorrelation in land use type and ecosystem service value in Hainan Tropical Rain Forest National Park. Ecol. Indic. 137, 108727. doi:10.1016/j.ecolind.2022.108727
Li, X. D., Liu, T., Zhao, C. L., Shao, M. A., and Cheng, J. (2021). Land use drives the spatial variability of soil phosphorus in the Hexi Corridor, China. Biogeochemistry 155, 59–75. doi:10.1007/s10533-021-00812-9
Liang, J., Zheng, H. H., Cai, Z. Y., Zhou, Y. M., and Xu, Y. (2022). Evaluation of cultivated land quality in semiarid sandy areas: a case study of the horqin zuoyihou banner. Land 11, 1457. doi:10.3390/land11091457
Lichtenberg, E., and Ding, C. G. (2008). Assessing farmland protection policy in China. Land Use Policy 25, 59–68. doi:10.1016/j.landusepol.2006.01.005
Ling, C., Zhang, G. P., Deng, X. Y., Jiao, A. Y., Chen, C. Q., Li, F. J., et al. (2022). A study on the drivers of remote sensing ecological index of aksu oasis from the perspective of spatial differentiation. Water 14, 4052. doi:10.3390/w14244052
Liu, J., Zeng, S., Ma, J., Chang, Y., Sun, Y., and Chen, F. (2022a). The impacts of rapid urbanization on farmland marginalization: a case study of the yangtze river delta, China. Agriculture-Basel 12, 1276. doi:10.3390/agriculture12081276
Liu, J. P., Jin, X. B., Lin, J. H., Liang, X. Y., Zhang, X. L., and Zhou, Y. K. (2024). Identification and characteristic analysis of semi-natural habitats in China's economically developed areas: new insights to inform cultivated land system ecological conservation. J. Environ. Manag. 351, 119804. doi:10.1016/j.jenvman.2023.119804
Liu, S. C., Xiao, W., Ye, Y. M., He, T. T., and Luo, H. (2023). Rural residential land expansion and its impacts on cultivated land in China between 1990 and 2020. Land Use Policy 132, 106816. doi:10.1016/j.landusepol.2023.106816
Liu, W., Ma, L., Smanov, Z., Samarkhanov, K., and Abuduwaili, J. (2022c). Clarifying soil texture and salinity using local spatial statistics (Getis-Ord gi* and Moran's I) in Kazakh-Uzbekistan border area, central asia. Agronomy-Basel 12, 332. doi:10.3390/agronomy12020332
Liu, X. P., Li, X., Tan, Z. Z., and Chen, Y. M. (2011). Zoning farmland protection under spatial constraints by integrating remote sensing, GIS and artificial immune systems. Int. J. Geogr. Inf. Sci. 25, 1829–1848. doi:10.1080/13658816.2011.557380
Liu, Y. L., Li, J. L., Liu, C., and Wei, J. S. (2022b). Evaluation of cultivated land quality using attention mechanism-back propagation neural network. Peerj Comput. Sci. 8, e948. doi:10.7717/peerj-cs.948
Luo, G. L., Wang, B., Li, R. W., Luo, D. Q., and Wei, C. F. (2022). Study of the agglomeration characteristics of cultivated land in underdeveloped mountainous areas based on spatial auto-correlation: a case of pengshui county, chongqing, China. Land 11, 854. doi:10.3390/land11060854
Ma, L., Long, H. L., Tu, S. S., Zhang, Y. N., and Zheng, Y. H. (2020). Farmland transition in China and its policy implications. Land Use Policy 92, 104470. doi:10.1016/j.landusepol.2020.104470
Maddison, D. A. (2009). A spatio-temporal model of farmland values. J. Agric. Econ. 60, 171–189. doi:10.1111/j.1477-9552.2008.00182.x
Mottaleb, K. A., Kruseman, G., Frija, A., Sonder, K., and Lopez-Ridaura, S. (2023). Projecting wheat demand in China and India for 2030 and 2050: implications for food security. Front. Nutr. 9, 1077443. doi:10.3389/fnut.2022.1077443
Pandit, R., Polyakov, M., Tapsuwan, S., and Moran, T. (2013). The effect of street trees on property value in Perth, Western Australia. Landsc. Urban Plan. 110, 134–142. doi:10.1016/j.landurbplan.2012.11.001
Pang, R. R., Xu, H., Zhang, M. Y., and Qian, F. K. (2023). Spatial correlation and impact mechanism analysis of cultivated land fragmentation and quality in the Central Plain of Liaoning Province, Northeast China. Land Degrad. and Dev. 4797, 4623–4634. doi:10.1002/ldr.4797
Qi, Y., Lin, R. H., Zhang, J. Q., Lu, M. N., Zhang, Y. Y., Zhao, H. L., et al. (2024). Impact of farmland quality on farmland rents and its constraints: evidence from plots in six Chinese provinces. Land Use Policy 139, 107075. doi:10.1016/j.landusepol.2024.107075
Qian, F., Lal, R., and Wang, Q. (2021a). Land evaluation and site assessment for the basic farmland protection in Lingyuan County, Northeast China. J. Clean. Prod. 314, 128097. doi:10.1016/j.jclepro.2021.128097
Qian, F. K., Wang, W. W., Wang, Q. B., and Lal, R. (2021b). Implementing land evaluation and site assessment (LESA system) in farmland protection: a case-study in northeastern China. Land Degrad. and Dev. 32, 2437–2452. doi:10.1002/ldr.3922
Qie, L., Pu, L., Tang, P., Liu, R., Huang, S., Xu, F., et al. (2023). Gains and losses of farmland associated with farmland protection policy and urbanization in China: an integrated perspective based on goal orientation. Land Use Policy 129, 106643. doi:10.1016/j.landusepol.2023.106643
Raty, M., and Kangas, A. (2007). Localizing general models based on local indices of spatial association. Eur. J. For. Res. 126, 279–289. doi:10.1007/s10342-006-0147-1
Rotches-Ribalta, R., Ruas, S., Ahmed, K. D., Gormally, M., Moran, J., Stout, J., et al. (2021). Assessment of semi-natural habitats and landscape features on Irish farmland: new insights to inform EU Common Agricultural Policy implementation. Ambio 50, 346–359. doi:10.1007/s13280-020-01344-6
Shaker, R. R. (2018). Examining sustainable landscape function across the Republic of Moldova. Habitat Int. 72, 77–91. doi:10.1016/j.habitatint.2016.11.002
Song, H. S., and Zhang, M. (2017). Spatial spillovers of regional innovation: evidence from Chinese provinces. Emerg. Mark. Finance Trade 53, 2104–2122. doi:10.1080/1540496x.2017.1284061
Song, W., Zhang, H. Z., Zhao, R., Wu, K. N., Li, X. J., Niu, B. B., et al. (2022). Study on cultivated land quality evaluation from the perspective of farmland ecosystems. Ecol. Indic. 139, 108959. doi:10.1016/j.ecolind.2022.108959
Tan, Y. Z., Chen, H., Lian, K., and Yu, Z. N. (2020). Comprehensive evaluation of cultivated land quality at county scale: a case study of shengzhou, Zhejiang Province, China. Int. J. Environ. Res. Public Health 17, 1169. doi:10.3390/ijerph17041169
Tang, P., Feng, Y., Li, M., and Zhang, Y. Y. (2021). Can the performance evaluation change from central government suppress illegal land use in local governments? A new interpretation of Chinese decentralisation. Land Use Policy 108, 105578. doi:10.1016/j.landusepol.2021.105578
Taveira, L. R. S., Weindorf, D. C., De Menezes, M. D., de Carvalho, T. S., Da Motta, P. E. F., Teixeira, A. F. D., et al. (2021). Land use capability classification adaptation in low and intermediate technology farming systems: a soil erosion indicator. Soil Use Manag. 37, 164–180. doi:10.1111/sum.12555
Wang, L., Huang, N. S., Kuang, Y. Q., Zhou, J. H., Zhao, Y., Zhang, Z., et al. (2014). Optimized selection of suitable sites for farmland consolidation projects using multi-objective genetic algorithms. Int. J. Agric. Biol. Eng. 7, 19–27. doi:10.3965/j.ijabe.20140703.003
Wang, Y. M. (2015). Negotiating the farmland dilemmas: 'barefoot planners' in China's urban periphery. Environ. Plan. C-Government Policy 33, 1108–1124. doi:10.1068/c1302
Xin, L., and Li, X. (2018). China should not massively reclaim new farmland. Land Use Policy 72, 12–15. doi:10.1016/j.landusepol.2017.12.023
Xu, P., Jin, Z. H., Ye, X. X., and Wang, C. (2022). RETRACTED: efficiency measurement and spatial spillover effect of green agricultural development in China. Front. Environ. Sci. 10, 909321. doi:10.3389/fenvs.2022.909321
Yan, B. J., Yan, J. J., and Shi, W. J. (2017). Estimation of carrying capacity of livestock farm based on maximum phosphorus load of farmland and GIS spatial analysis technology. Curr. Sci. 112, 1931–1936. doi:10.18520/cs/v112/i09/1931-1936
Ye, Y., Qin, Y., Yu, R., and Wu, Q. (2024). Optimization of Chinese land spatial pattern in the transformation process of resource-based cities: a case study in Tongling City, China. Sci. Rep. 14, 6092. doi:10.1038/s41598-024-53546-7
Yuan, Y. M., Cave, M., and Zhang, C. S. (2018). Using Local Moran's I to identify contamination hotspots of rare earth elements in urban soils of London. Appl. Geochem. 88, 167–178. doi:10.1016/j.apgeochem.2017.07.011
Zhang, C., Wang, X., and Liu, Y. J. (2019). Changes in quantity, quality, and pattern of farmland in a rapidly developing region of China: a case study of the Ningbo region. Landsc. Ecol. Eng. 15, 323–336. doi:10.1007/s11355-019-00382-x
Zhang, R., Tian, Q., Jiang, L., Crooks, A., Qi, S., and Yang, R. (2018). Projecting cropping patterns around Poyang lake and prioritizing areas for policy intervention to promote rice: a cellular automata model. Land Use Policy 74, 248–260. doi:10.1016/j.landusepol.2017.09.040
Zhang, R. Q., Li, P. H., Xu, L. P., Zhong, S., and Wei, H. (2022a). An integrated accounting system of quantity, quality and value for assessing cultivated land resource assets: a case study in Xinjiang, China. Glob. Ecol. Conservation 36, e02115. doi:10.1016/j.gecco.2022.e02115
Zhang, W. H., Zhu, G. F., Qiu, D. D., Liu, Y. W., Sang, L. Y., Lin, X. R., et al. (2022b). Effects of agricultural activities on hydrochemistry in the Shiyang river basin, China. Environ. Sci. Pollut. Res. 30, 12269–12282. doi:10.1007/s11356-022-22914-z
Zhou, W., Zhao, L., Hu, Y. M., Liu, Z. H., Wang, L., Ye, C. D., et al. (2022). Cultivated land quality evaluated using the RNN algorithm based on multisource data. Remote Sens. 14, 6014. doi:10.3390/rs14236014
Keywords: farmland quality, spatial layout of cultivated land protection, territorial spatial planning, improved local spatial autocorrelation model, ecological environment index, Liuhe district
Citation: Shu H, Guancheng G, Junjie L and Chuan Z (2025) Optimization of the layout of cultivated land combining territorial spatial planning with quality index spatial autocorrelation characteristics: taking Liuhe District of Nanjing as an example. Front. Environ. Sci. 12:1496340. doi: 10.3389/fenvs.2024.1496340
Received: 18 September 2024; Accepted: 16 December 2024;
Published: 06 January 2025.
Edited by:
João Pedro Matos-Carvalho, Lusofona University, PortugalReviewed by:
Shicheng Li, China University of Geosciences Wuhan, ChinaFilipe Moutinho, NOVA University of Lisbon, Portugal
Afonso Oliveira, Lusofona University, Lisbon, Portugal in collaboration with reviewer FM
Copyright © 2025 Shu, Guancheng, Junjie and Chuan. This is an open-access article distributed under the terms of the Creative Commons Attribution License (CC BY). The use, distribution or reproduction in other forums is permitted, provided the original author(s) and the copyright owner(s) are credited and that the original publication in this journal is cited, in accordance with accepted academic practice. No use, distribution or reproduction is permitted which does not comply with these terms.
*Correspondence: Han Shu, aGFuc2h1QG5qYXUuZWR1LmNu; Guo Guancheng, Z2djQG5qYXUuZWR1LmNu