- 1School of Statistics, Renmin University of China, Beijing, China
- 2School of Statistics, Dongbei University of Finance and Economics, Dalian, China
- 3School of Statistics and Mathematics, Central University of Finance and Economics, Beijing, China
Green technology has received continuous attention and facing challenges of transformation because of the growing environmental concern, especially the carbon emissions resulting in global warming. In the Industry 4.0 era, green technology transforms into smart green technology benefits from the convergence with digital technologies. However, little research investigates the role of green and digital technology convergence (GDC) on carbon emission reduction. This study proposes a novel measurement of the GDC level of enterprise based on the network method and examines its pattern and dynamic. Moreover, we empirically analyze the impact of GDC on carbon emission reduction. Results show that GDC has a significant carbon reduction effect. Moreover, GDC reduces enterprises’ carbon emissions by improving green innovation quality. Furthermore, an enterprise’s technology convergence capability moderates the focal relationship. The heterogeneity analysis results reveal that the carbon reduction effect of GDC is stronger in large, state-owned, non-labor-intensive and heavy-pollution enterprises. The findings contribute to a better understanding of the fusion of greening and digitalization and provide useful insights for sustainable development policy-making.
1 Introduction
Global warming will cause vast damage to natural ecologies, disrupting human living environments. The climate risk also may threaten economic and social development, such as triggering systematic risks in the global banking system (Wu et al., 2024; Liu B. et al., 2024). Carbon emission is a notable reason for global warming. Numerous countries have implemented policies aimed at reducing carbon emissions. As the world’s largest developing country and the biggest emitter of carbon dioxide, China places significant emphasis on addressing climate change and is actively engaged in various efforts to reduce emissions. China declared its “carbon peaking” and “carbon neutrality” goals and plans to reach peak carbon use by 2030 and become carbon neutral by 2060. Various industrial processes will emit much carbon dioxide into the atmosphere. Therefore, enterprises bear important responsibilities for carbon emission reduction (Luo et al., 2023). To reduce carbon emissions, green technological innovation is one of the critical factors (Weina et al., 2016; Nikzad and Sedigh, 2017). However, in the Industry 4.0 era, green technology innovation has faced challenges in transformation. Due to digital technology’s universality and penetration characteristics, its convergence with green technology facilitates the transformation. However, few studies have discussed the convergence of green and digital technology and its carbon reduction effect.
Extensive studies have shed light on the various drivers of carbon emission reduction. For example, in recent studies, Ren et al. (2024a) investigated the impact of economic freedom on corporate carbon emissions and revealed potential mechanisms regarding resource utilization and industrial agglomeration. Ren et al. (2024b) studied the role of climate vulnerability in global carbon emissions and found a significant spatial convergence pattern. Moreover, from the perspective of information disclosure, Wang et al. (2024) explored the influence of climate risk disclosure on carbon emissions. They found that this relationship is moderated by executives’ environmental experience, investor attention, and government environmental regulation. Furthermore, Sun et al. (2023) found that the development of ICT can help reduce global carbon emissions, especially in middle-income countries. There are also earlier studies documenting the various sources affecting carbon emission reduction, such as digital financial inclusion (Wang et al., 2022) and political connections (Wang et al., 2023). In addition, literature has also focused on the positive role of green technology innovation in carbon emission reduction (see e.g., Weina et al., 2016; Nikzad and Sedigh, 2017).
However, we noticed that there is no consensus in the existing literature on the relationship between green technology and carbon emissions. Braungardt et al. (2016) pointed out that the carbon reduction effects of green technology may be outweighed by the increase in carbon emissions associated with economic growth, as the rebound effect could lead to higher energy consumption. Weina et al. (2016) also found in their study that although green technology has improved environmental productivity, it has not resulted in significant carbon emission reductions. Lin and Ma (2022) highlighted that green technology has no significant carbon reduction effect in a study of 264 cities in China. The reason for this debate is the difference between the quality of green technology innovation and carbon reduction efficiency (Acemoglu et al., 2012; Jaffe et al., 2002). Thus, green technology needs to transform to improve quality and efficiency. Digitalization is a critical direction for green technology transformation in the era of Industry 4.0 (Cao et al., 2024).
The critical role of digital technology adoption in business transformation has been widely discussed in literature. Recent studies document that Ran et al. (2024) unveiled the effect of digital technology adoption in promoting the dual circulation of enterprise. Liu Y. et al. (2024) found that digital technology application moderates the relationship between servitization and service business focus. Lu et al. (2023) revealed the moderating effect of digital technology adoption on the relationship between supply chain governance and resilience performance. In addition, studies have investigated the impact of digital transformation on green technology from the perspective of strategic decision-making, and believe that digital transformation of enterprises can promote green technology innovation (Tang et al., 2023; Han et al., 2024). However, there is still a lack of research revealing the role of digital technology in achieving environmental goals from the perspective of technology convergence (Loeser et al., 2017).
According to the technology convergence theory, green and digital technology convergence (GDC) represents the integration of technological knowledge across these two different disciplines and the blurring of their boundaries (see e.g., Hacklin, 2008; Sung et al., 2010; Curran, 2013; Kim and Lee, 2017; Feng et al., 2020; San Kim and Sohn, 2020). The convergence of green and digital technology is a complex process. The changes caused by the converging process mainly lie in two aspects. On the one hand, the integration of green technology and digital technology allows for the unified digital encoding of technological knowledge and information, enhancing the level of information sharing and knowledge integration in enterprises’ green technology, thereby improving technological efficiency (Goldfarb and Tucker, 2019). On the other hand, the convergence of digital technology further enables real-time monitoring, control, and optimization of the application processes of green technology to reduce energy waste.
Studies have presented operational frameworks for measuring technology convergence using the co-occurrence of technology classifications of patents (Curran and Leker, 2011; Jeong et al., 2014), as patent reflects the firms’ technological trajectories and demonstrate their technological changes. The International Patent Classification (IPC) established by the World Intellectual Property Organization (WIPO) provides an international standard for technology classification. On this basis, GDC relationships can be constructed according to the co-occurrence of both green and digital technology in the firms’ patents. The co-occurrence relationships embedded in the numerous patents will form a complex technology convergence network. Therefore, social network analysis is an effective tool for measuring and analyzing GDC (Curran, 2013).
Based on the above analysis, this study aims to investigate the impact of GDC on carbon emission reduction and the influencing mechanisms hidden behind the Chinese listed companies for 2006–2021. The main contributions of this study are therefore as follows: 1) a novel measurement of the convergence of green and digital technology is proposed in this study; this not only unveils the transformation of green technology to be smart or digital but also offers new evidence for the GDC level and patterns in China; 2) the influencing mechanism between GDC and carbon emission reduction is systematically analyzed in this study; this unveils the role of green innovation quality and technology convergence capability and provides management and policy insights for stakeholders; 3) the heterogeneity effects of GDC is closely examined and expands the understanding of the carbon emission reduction effects of GDC.
The remainder of this study is organized as follows. Section 2 is the theoretical mechanisms which reviews the relevant literature and develops our research hypotheses. Section 3 presents the data source and measurement of GDC. Section 4 provides the variable definitions and econometrics model. Section 5 reports the results of empirical studies and the conclusions and policy implications are concluded in Section 6.
2 Theoretical mechanisms
2.1 Green and digital technology convergence and carbon emission reduction
Studies define technology convergence as the phenomenon of the blurring of boundaries between at least two different areas of technology (Kodama, 1995; Curran and Leker, 2011; Kim et al., 2014). At the innovation level, converging fields appear to be characterized by greater novelty and more breakthrough outcomes (Schumpeter, 1939; No and Park, 2010; Karvonen and Kässi, 2013). Among the converging fields, digital technology is a general technology that influences almost all technologies (Nambisan et al., 2019). Convergence occurs when digital technology is embedded into technologies in other sectors (Park and Heo, 2020). The transformation brought about by digital technologies towards intelligence is amplified by these technological integrations (Schwab, 2017). The convergence of digital technology reflects its disruptive impact on reorganizing and transforming innovation processes (e.g., Curran, 2013).
The technological convergence theory provides theoretical foundations for the carbon emission reduction effect of GDC. The technology convergence theory explains the process of the convergence of green and digital technology, which also unveils the changes of green technology to be smarter after convergence. In the context of GDC, the integration of digital technology enables real-time monitoring, control, and optimization of the application processes of green technology to reduce energy waste. In addition, the convergence of digital technology promotes digitized monitoring of energy consumption, accurate measurements, and predictions of emissions, and planning and implementation of efficiency improvements, which enable the achievement of on-demand supply and efficient production and reduce energy consumption from the source. The convergence of digital technologies enables value realization through energy conservation, reduction in consumption and emissions, quality improvement, and efficiency enhancement (Noussan and Tagliapietra, 2020).
Moreover, GDC creates new knowledge with the integration of knowledge in green and digital technology fields, which improves the efficiency of green technology implementation. Recent studies have recognized the relationship between the digitalization of green technology and carbon emissions, but they only examined a specific green technology area. For example, existing research has documented the fusion of digital technologies in environmental, energy, and resource-related fields (Zhang et al., 2023; Zeng and Yang, 2023). In terms of energy production and management, this fusion has led to the development of photovoltaics, wind power, and other types of renewable energy, which have further led to diverse complementary fields of clean energy and decarbonization (Chen et al., 2021). In addition, the integration of green technology and digital technology allows for the unified digital encoding of technological knowledge and information, enhancing the level of information sharing and knowledge integration in enterprises’ green technology, thereby improving technological efficiency (Goldfarb and Tucker, 2019).
Therefore, we propose the following hypothesis,
H1. GDC reduces the carbon emission intensity of enterprises.
2.2 Green innovation quality channel
The knowledge-based view holds that the convergence of digital technologies will broaden the breadth and depth of knowledge in green technology innovation, thereby improving the quality of green technology innovation. Yoo et al. (2012) argued that the availability of digital technology has led to generativity and convergence characteristics in innovation, largely due to the integration of knowledge (Brynjolfsson and McAfee, 2014; Di Vaio et al., 2021). Moreover, digital technology has helped expand the breadth and depth of external knowledge acquisition, enabling them to achieve breakthroughs through existing technological structures and domain limitations and acquire cutting-edge knowledge efficiently (Radicic and Petković, 2023; Yang et al., 2022). Therefore, digital technology can dismantle enterprises’ path dependence on previous technological trajectories, enabling them to achieve green knowledge integration across different knowledge domains, reconstruct knowledge repositories, and enhance the quality of green innovation (Ning et al., 2023).
Enhancing the quality of green innovation can significantly contribute to carbon emission reduction. Green technology encompasses a wide range of solutions, including pollution control, recycling, ecological treatment, purification, monitoring, and various evaluation techniques. From a green innovation perspective, advancements in clean recycling technologies can reduce the consumption of fossil fuels like coal and oil, increase resource and energy efficiency and play a crucial role in lowering CO2 emissions. Innovations in pollution control and ecological technologies can effectively eliminate or transform pollutants generated during production, thus reducing direct emissions of substances such as CO2. Green product innovation focuses on meeting environmental standards throughout the product’s entire life cycle, aiming to deliver environmental benefits at every stage (Zhang et al., 2020). Consequently, the improvement of green technology innovation quality will better play these carbon reduction effects. At the same time, by increasing the efficiency of green technology innovation, it is possible to achieve greater carbon reduction with the same level of innovation input, thereby weakening the rebound effect.
H2. GDC enhances the quality of an enterprise’s green innovations reducing its carbon emission intensity.
2.3 Moderating effects of technology convergence capability
According to the technological convergence theory, the converging fields can produce greater novelty and more breakthrough outcomes (Schumpeter, 1939; No and Park, 2010; Karvonen and Kässi, 2013). Kodama (1991) highlighted the significance of technological convergence capability for achieving breakthroughs by building on existing technologies and merging different technologies. This convergence broadens the scope of technological impact, frequently resulting in the creation of advanced products. (Adner and Levinthal, 2002; Gauch and Blind, 2015). At the technical level, technological problems are becoming increasingly complex, and it is necessary to integrate technologies from different disciplines to solve these problems (Hacklin and Wallin, 2013). Converged fields seem to have greater innovation and breakthrough results (No and Park, 2010; Karvonen and Kässi, 2013). In addition, technology convergence can open up new technological fields and generate opportunities for technological recombination. At the enterprise level, technology convergence provides a wide range of technological solutions (Nordmann, 2004; Roco and Bainbridge, 2002), enhancing the innovation capabilities of enterprises (Karvonen and Kässi, 2013).
The convergence of green and digital technology involves the intersection of two types of knowledge. The technological integration capability of enterprises facilitates innovation through increasing interdisciplinary knowledge (Kose and Sakata, 2019). And it reshapes innovation trends and creates new innovation pathways (No and Park, 2010; Kim et al., 2012; Curran, 2013). The ability to integrate technologies will strengthen the synergy between digital and green technologies within enterprises, amplifying their combined effect on reducing carbon emissions.
H3. Enterprise’s technology convergence capability moderates the relationship between green and digital technology convergence and carbon emission reduction.
We present our research framework in Figure 1.
3 Data and measurement of GDC
3.1 Data
For the empirical analysis, two data sets are combined: patent data and a firm’s basic information and financial data. In this study, listed companies in the A-share market are selected to examine the level and effect of GDC. First, patent data of the sample companies from the China National Intellectual Property Administration (CNIPA) database are used in the measurement of GDC. This study uses patents applied in China from 2006 to 2021. Second, the IPC classification provided by the CNIPA allocates lists of sub-class IPCs for each patent. Third, we identify the green patents based on the “International Patent Classification Green List” provided by the World Intellectual Property Organization (WIPO). The list includes seven categories of green technologies and the corresponding IPCs. Fourth, the digital technologies are identified following Bielig (2023). As a result, 139,069 green patents are collected from A-share-listed companies in China.
This study also uses data from the CSMAR database. We collect the basic information and financial data of the A-share listed companies from 2006 to 2021. We performed data cleansing before conducting the empirical work by deleting observations with missing key variables, companies labeled as “ST,” and companies in the financial industry. We then winsorized the continuous variables at 1%, resulting in a final dataset containing 22,946 valid observations from 2,193 listed companies.
3.1.1 Technology convergence network construction
The measurement of GDC is based on the analysis of the technology convergence network. Therefore, we construct the technology convergence network using patent data. A patent often covers multiple technical fields. Multiple technologies appearing concurrently in the same patent are called co-occurrence, which is a direct manifestation of technology convergence (Curran and Leker, 2011; Geum et al., 2012; Karvonen and Kässi, 2013).
Focusing on the co-classification situation of green patent data, we found that of all 139,067 green patents, 37,009 inventions were co-classified with digital technologies. Let
Furthermore, we build the technology convergence network according to the following steps. First, the four-digit IPC technology classes are nodes, and the edges represent the co-occurrence in one patent between technology classes
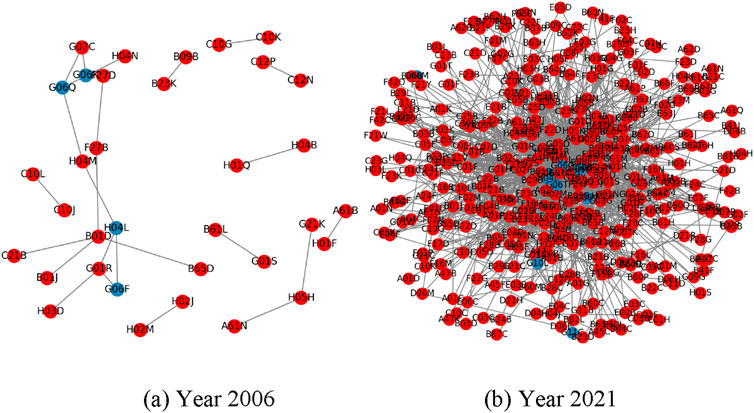
Figure 4. Technology convergence network of green innovation. Note: Nodes in red and blue denote nondigital technologies and digital technologies, respectively, in green innovation. (A) Year 2006 (B) Year 2021.
3.1.2 Measurement of GDC
Based on the technology convergence network, we calculate the GDC level of each enterprise. First, we obtain the shortest path of each IPC to the digital technology in the technology convergence network. Second, we calculate the proportion of each firm’s IPCs. Third, we take the proportion of IPC and their shortest path to digital technology into consideration and multiply them to evaluate GDC level. The process of measurement is illustrated in Figure 5.
In sum, we perform the GDC measurement by using the following equation:
where
4 Variables and methods
4.1 Variables
4.1.1 Dependent variable
Carbon emission intensity. We adopted the method of Konadu et al. (2022) to measure enterprises’ carbon emissions according to the “greenhouse gas accounting system.” As such, carbon emissions are divided into those that come directly or indirectly from sources owned or controlled by an enterprise. To capture these data, we referred to company annual reports. For companies that only disclosed different types of consumption, we calculated their total carbon emissions based on the fuel carbon emission coefficients from their reports2. We used the ratio of total carbon emissions to total operating revenue to measure carbon emission intensity (Li et al., 2021), representing tons of carbon emissions per million yuan of revenue.
4.1.2 Independent variable
GDC. Enterprises’ GDC was measured based on the technology convergence network (Kim et al., 2019; Kose and Sakata, 2019), which was constructed using IPCs as nodes and co-occurrence relationships as links. We calculated the shortest distance from the IPC classification number of the patent to the IPC classification number of the digital technology field for each industry. We then calculated the proportion of IPCs owned by each enterprise multiplied by the reciprocal of the shortest distance.
4.1.3 Mediating variable
Green innovation quality. As discussed in the hypothesis, GDC can achieve carbon reduction effects by improving the quality of green technologies. Griliches et al. (1987) showed that the value distribution of innovation is extremely skewed, with most of the influence concentrated on a few crucial and highly cited patents. Flammer and Kacperczyk (2016) used the number of patent citations to measure the quality of enterprise innovation. To this end, we used the number of patent citations, QS, to measure the quality of green innovations. To ensure robustness, we also used the ratio of the cumulative number of green patent citations to the total number of employees, QR, as an alternative measure.
4.1.4 Moderating variable
Technology convergence capability. Based on Kim et al. (2017), we measured firms’ technology convergence capability, TC, based on their centrality in the technology convergence network. The specific construction of TC requires three steps. First, we calculated the degree and page rank centralities for each IPC technology territory in the convergence network. Second, we compute the proportion of IPC per territory owned by the enterprise. The proportion of IPCs owned indicates the extent to which the enterprise uses these technologies (Scott, 2017). Third, the TC of each firm was measured by the aggregate product of the centrality index of the IPC in each territory and the proportion of IPCs owned by the enterprise. In the first stage, we used degree and page rank centrality, obtaining two alternative TC measurements: TC_degree and TC_page_rank.
4.1.5 Control variables
To minimize bias caused by omitted variables, we control the following variables that may affect enterprises’ carbon emission intensity: the age of the enterprise (Age), which is the logarithm of the difference between the current and established year plus one; the size of the enterprise (Size), which is the logarithm of total assets; the asset-liability ratio (Leverage), which is the total liabilities divided by assets; Tobin’s Q, which is the development ability and market value of firms; Cashflow, which is the ratio of net cash flows from operating activities to total assets; operating income growth (Growth), which is the proportion of revenue growth over the previous year; total asset turnover rate (Turnover), which is the ratio of operating income to total assets; and concentration ratio (Top3), which is the shareholding ratio of the top three shareholders.
4.1.6 Descriptive statistics of variables
The descriptive statistics for each variable are presented in Table 1. Descriptive statistics show that the dependent variable carbon emission intensity has an average value of 47.360 and a standard deviation of 22.481. The minimum value of the independent variable GDC is 0. This is because some enterprises have not yet achieved the convergence of green and digital technology, especially in the earlier sample years. The moderating variable TC_degree and TC_page_rank also has a minimum value of 0, which reflects the existing of the technology convergence network not having the connected areas in the corresponding patent data for calculation.
4.2 Econometric models
To verify whether GDC reduces enterprise carbon emission intensity, the fixed-effect panel data model is constructed as follows:
where
To verify whether GDC reduces enterprise carbon emission intensity by improving the green innovation quality, a two-way effect model using the mediating variable as the explained variable was constructed as follows:
where
To further explore the moderating effect of technological convergence capability on enterprises’ carbon emission intensity, the intersection of GDC and technology convergence capability was introduced in the following regression equation:
where
5 Empirical analysis
5.1 Benchmark regression results
We used Equation 2 to evaluate the influence of GDC on carbon emission intensity using a two-way fixed-effects model. Table 2 lists the empirical results, indicating that GDC significantly reduced carbon emissions. Specifically, the regression model in Column (1) includes the independent variable and the year fixed-effects without control variables, indicating that GDC exhibited a prominently negative influence on carbon emission intensity. The model in Column (2) includes the firm fixed effects; GDC was still negatively correlated with carbon emission intensity. The models in Columns (3) and (4) add control variables on the base of Columns (1) and (2), respectively. Column (4) presents the results of the full model, indicating that GDC reduces firm carbon emission intensity. Taken together, these findings support Hypothesis 1. That is, GDC positively affects firms’ carbon emission reductions. This finding shows that the convergence of digital technology enables real-time monitoring, control, and optimization of the application processes of green technology to reduce energy waste, thereby reducing carbon emissions. Moreover, the integrated digital technology may contribute to the unified digital encoding of technological knowledge, which improves the efficiency of green technology applications.
5.2 Underlying mechanism
5.2.1 Mediating effect of green innovation quality
In this section, we explore whether GDC improves green innovation quality, leading to lower carbon emissions. Table 3 presents the results. The findings in Column 1 show that an increase in GDC implied a significant increase in green innovation quality (β = 0.346, p < 0.01). Column 2 shows that the green innovation citation scale had a significant negative effect on carbon emission intensity at the 1% level (β = −0.720). Moreover, GDC had a significant negative effect on carbon emission intensity at the 10% level (β = −0.192). Compared with the baseline regression in Table 2, the GDC coefficient decreased from −0.456 to −0.192, indicating that the green innovation citation scale positively mediated the relationship between GDC and carbon emission intensity. Finally, Columns 3 and 4 present similar results of the green innovation citation ratio as the mediating variable.
5.2.2 Moderating effect of technology convergence capability
As discussed previously in Section 2.3, social network theory states that companies in different positions within a network may have differentiated information and resources. Meanwhile, from an organizational learning perspective, companies with high centrality can access more timely information, further enhancing the potential and capacity for organizational learning (Brown and Duguid, 1991). Therefore, the position of a firm in the convergence network is highly correlated with GDC. Therefore, analyzing whether technology convergence capability affects the relationship between GDC and carbon emission intensity is necessary; hence, we measured technology convergence capability based on network centrality. In Columns (1) and (2) of Table 4, the coefficients of GDC were all negative and significant, indicating that GDC significantly reduced carbon emission intensity. This provides further support to Hypothesis 1. Moreover, the interaction term between GDC and technology convergence capability was significantly negative, meaning that a firm’s technology convergence capability moderates the relevance of GDC and carbon emission intensity, supporting Hypothesis 3 and demonstrating that companies possessing higher technology convergence capabilities have stronger carbon emission reduction effects.
5.3 Heterogeneous effects
Regarding the issue of carbon emissions, the special nature of state-owned enterprises in China suggests that they must undertake more corporate social responsibility than other firms. They also receive more support from relevant government and banking departments. Therefore, it is necessary to further explore whether GDC has a heterogeneous impact on carbon emission intensity.
5.3.1 Firm heterogeneity
We divided the entire sample into state-owned and non-state-owned enterprises for group regression. Column (1) of Table 5 indicates that the coefficient of GDC in the subsample of state-owned enterprises was negative and the estimated coefficient was significant at the 1% level. In Column (2), the coefficient of GDC in the subsample of private enterprises was positive but not significant, indicating that GDC has a more obvious effect on the carbon emission reduction of state-owned enterprises. This result could be attributed to the inherent political affiliations of state-owned enterprises. Under the goals of “carbon peaking” and “carbon neutrality,” state-owned enterprises have stronger environmental responsibilities and regulations and are more willing to improve their GDC to reduce emissions. In addition, GDC requires a large amount of funding, which state-owned enterprises have in abundance. Hence, they are more capable of achieving the transformation of green technology by integrating it with digital technologies.
We also examine the heterogeneity between small and large enterprises3. The descriptive statistics of the GDC level for the small enterprise at the 25%, 50%, and 75% quantiles are 0, 0.002, and 0.016, respectively, while the corresponding values of large enterprises are 0, 0.006, and 0.117. The value 0 at the GDC level shows that there are enterprises that have not realized the convergence of green and digital technology in the early sample years. Moreover, the GDC level of small enterprises is lower than that of large enterprises. The regression results are reported in Columns (3)–(4) of Table 5. The coefficient of GDC in Column (3) is negatively significant, indicating that GDC reduces carbon emissions in large enterprises. However, the result in Column (4) shows that GDC has no significant influence on carbon emission reduction in small enterprises. The reason for this result may lie in the advantages of large enterprises in terms of capital, talent, and technology, resulting in a higher GDC degree and more noticeable digital carbon reduction effects.
5.3.2 Industry heterogeneity
Regarding industry heterogeneity, we investigate the carbon emission reduction effect of GDC at different industry pollution levels. We categorize industries into heavy-pollution and clean industries based on the “Guidelines for Environmental Information Disclosure of Listed Companies” issued by the State Environmental Protection Administration in September 2010. In this guideline, the thermal power, steel, cement, electrolytic aluminum, coal, metallurgy, chemicals, petrochemicals, building materials, papermaking, brewing, pharmaceuticals, fermentation, textiles, leather, and mining are defined as heavy-pollution industries, whereas other industries are referred to as “clean industries.” The results are presented in Columns (5) and (6) in Table 5. We find that the GDC has a significant carbon emission reduction effect in the heavy-pollution industries, while does not have a significant impact in clean industries. The reason behind this may be that the heavy-pollution industries are key targets monitored by China’s environmental protection departments, and green technology innovation is crucial for enterprises in these sectors. Therefore, heavy-pollution industries are more motivated to implement digital transformation of green technology innovation to improve carbon reduction efficiency.
We also examine the effects of GDC in the labor-intensive and non-labor-intensive industries for heterogeneity analysis. The descriptive statistics of the GDC level for the labor-intensive industries at the 25%, 50%, and 75% quantiles are 0, 0.001, and 0.056, respectively, while the corresponding values of non-labor-intensive enterprise are 0, 0.002, and 0.116. We find that the GDC level of labor-intensive industries is lower than that of non-labor-intensive industries. The regression results are reported in Columns (7) and (8) in Table 5. Results show that the coefficient of GDC is significant in non-labor-intensive industries while not significant in labor-intensive industries. In this regard, the possible explanation is enterprises in non-labor-intensive industries have stronger capital or technological capabilities and are more willing to adopt digital technologies. Thus, they can fully leverage the carbon reduction effects of GDC.
5.3.3 Technology field heterogeneity
This study explores the impact of GDC on carbon emission in different digital technology fields. The results in Columns (1)–(3) in Table 6 show that integrating enterprises with different digital technologies results in different carbon emission reduction effects. Specifically, integration with digital communication technology and computer technology significantly reduced enterprises’ carbon emission intensity. The potential reason may be that the digital communication and computer technology enable real-time monitoring, control, and optimization of the application of green technology to reduce energy waste.
5.4 Robustness tests
5.4.1 Alternative model: PSM method
As companies with high carbon emissions are more inclined to increase GDC, leading to possible selection bias, the coefficient results obtained from our regression may be higher than the average treatment effect (i.e., the carbon emission reduction effect of GDC might be underestimated). To alleviate potential endogeneity problems that occur due to reverse causality and self-selection effects, this article sets a dummy variable to measure the degree of GDC. If the GDC of an enterprise is greater than the mean, it is set to 1 and 0 if otherwise. We used a one-to-four matching method with all control variables as covariates. After matching, the absolute value of the standard deviation of each matching variable was less than 10%, and the treatment and control groups were equal in all observable characteristics. There was no significant difference, showing that the selection of matching variables and methods in this article was appropriate, and the nearest-neighbor matching and minimum radius estimation results were credible. Based on Table 7, the average treatment effect, ATT, calculated by the nearest-neighbor and minimum radius matching methods, was −0.845 and −0.846, respectively, which were smaller than the baseline regression coefficient and were significant at the 1% level. Hence, by keeping other conditions unchanged, GDC has a more obvious effect on reducing carbon emission intensity.
5.4.2 Alternative measurements of dependent and independent variables
This study used tons of carbon emissions per million assets as a replacement for the explained variable to conduct a robustness test. From the results listed in Column (1) in Table 8, after changing the measurement method, the coefficient of GDC remained significantly negative, indicating that a higher GDC can better reduce firms’ carbon emission intensity. In terms of the measurements of explanatory variables, Caviggioli (2016) argued that technology convergence relationships can last 3–5 years. Therefore, this study adopted a 3-year sliding window approach (2006–2008, 2007–2009, …, 2020–2022) to measure digitalization in green innovation. In Column (2), the coefficients were negative and significant, verifying that GDC significantly reduces carbon emission intensity.
5.4.3 Dividing different sample years
China ushered in the mobile Internet era in 2012, leading to rapid developments in the digital economy. Traditional industries began to become Internet-based. As digital technology began to have varying degrees of impact on various industries, the carbon emission reduction effect of digital technology after 2012 also changed compared with before 2012. Judging from the coefficient results listed in Columns (1) and (2) of Table 9, prior to 2012, digital technology did not significantly reduce enterprises’ carbon emission intensity, primarily because, at that early stage of development, digital technology did not improve production capacity and utilization efficiency. After 2012, GDC significantly reduced enterprises’ carbon emission intensity. Hence, as digital technology improves its energy efficiency and empowers the economy, it promotes the transformation of traditional industries into digital, intelligent, and environmentally friendly sectors. This leads to mutually beneficial economic situations where efficiency gains coexist with energy conservation and emission reduction efforts.
5.4.4 Eliminating the impact of industry changes
Due to major asset restructuring, expansion of business scale, and extended mergers and acquisitions, listed companies may have changed their industries. For example, a company may change from the civil engineering construction industry to an ecological protection and environmental governance industry, resulting in considerable changes in its carbon emission intensity, which may affect the regression results. To control for the impact of these types of industry changes, we eliminated companies that experienced industry changes and recalculated Equation 1. The results listed in Column (3) of Table 9 show that the significant negative impact of GDC on corporate carbon emission intensity remains, which is consistent with our baseline results, enhancing the credibility of the results.
6 Conclusion
We investigated the effects of GDC on carbon emission reductions in China using technology convergence network analysis and panel regression. We provided a novel metric for GDC and shed light on the mechanisms of GDC’s impact on carbon emission reduction. The results show that GDC significantly affects carbon emission intensity reduction. A series of robustness tests supported this result. Moreover, GDC reduces enterprise carbon emissions by improving the quality of green innovation. Furthermore, enterprises’ technology convergence capability significantly moderates the relationship between GDC and carbon emission reduction. Results of heterogeneity analysis show that GDC has a more pronounced effect on carbon emission reduction for state-owned, large, non-labor-intensive, and heavily polluting enterprises. Further improvements in carbon reduction effectiveness are needed for other types of enterprises.
Based on the above conclusions, this paper proposes the following policy recommendations:
(1) The government should actively promote the deep integration of green and digital technology in enterprises. On the one hand, the government should encourage companies to apply digital technologies and achieve intelligent green technology innovation through financial support and tax incentives. On the other hand, it should vigorously promote smart manufacturing or service production models to reduce resource waste and energy consumption, thereby enhancing the overall environmental benefits of enterprises. Additionally, the government should focus on improving the quality of green technology innovation through the transformation to intelligent green technologies, which will, in turn, enhance carbon reduction effectiveness.
(2) Enterprises that posit central network positions in the GDC network should be encouraged to leverage technological spillover effects. Occupying a favorable position in the network can amplify the carbon reduction effects of GDC. Therefore, the government should support collaborative innovation among enterprises, establish industry alliances and innovation cooperation platforms, and encourage companies from different sectors to achieve the transfer of green and digital technologies through innovation platforms, forming a collaborative innovation effect that reduces overall carbon emissions.
(3) Policy formulation should implement differentiated incentive mechanisms. For state-owned enterprises, large enterprises, enterprises in heavily polluting industries, and non-labor-intensive sectors, the government should set strict carbon emission standards and green production regulations to promote their active realization of GDC and facilitate carbon reduction. Conversely, for non-state-owned, small and medium-sized enterprises, clean industries, and labor-intensive industries, the government should support the construction of digital infrastructure to enhance their technological absorption capacity.
Data availability statement
The raw data supporting the conclusions of this article will be made available by the authors, without undue reservation.
Author contributions
JH: Writing–review and editing, Methodology, Writing–original draft, Software, Data curation. QS: Conceptualization, Writing–review and editing, Methodology. WW: Writing–review and editing, Methodology, Conceptualization.
Funding
The author(s) declare that financial support was received for the research, authorship, and/or publication of this article. Beijing Municipal Social Science Foundation (No. 22JJC033).
Conflict of interest
The authors declare that the research was conducted in the absence of any commercial or financial relationships that could be construed as a potential conflict of interest.
Publisher’s note
All claims expressed in this article are solely those of the authors and do not necessarily represent those of their affiliated organizations, or those of the publisher, the editors and the reviewers. Any product that may be evaluated in this article, or claim that may be made by its manufacturer, is not guaranteed or endorsed by the publisher.
Footnotes
1Refer to Kim et al. (2019) and Curran (2013) for a detailed construction of this network.
2The carbon emission coefficients are specified in the “IPCC National Greenhouse Gas Inventory Guidelines.”
3In this study, we used the logarithm of the total assets of an enterprise as proxy of firm size.
References
Acemoglu, D., Gancia, G., and Zilibotti, F. (2012). Competing engines of growth: innovation and standardization. Econ. Theory 147, 570–601.e3. doi:10.1016/j.jet.2010.09.001
Adner, R., and Levinthal, D. A. (2002). The emergence of emerging technologies. Calif. Manag. Rev. 45 (1), 50–66. doi:10.2307/41166153
Bielig, A. (2023). The propensity to patent digital technology: mirroring digitalization processes in Germany with intellectual property in a European perspective. J. Knowl. Econ. 14 (3), 2057–2080. doi:10.1007/s13132-022-00986-z
Braungardt, S., Elsland, R., and Eichhammer, W. (2016). The environmental impact of eco-innovations: the case of EU residential electricity use. Environ. Econ. Policy Stud. 18, 213–228. doi:10.1007/s10018-015-0129-y
Brown, J. S., and Duguid, P. (1991). Organizational learning and communities-of-practice: toward a unified view of working, learning, and innovation. Organ. Sci. 2 (1), 40–57. doi:10.1287/orsc.2.1.40
Brynjolfsson, E., and McAfee, A. (2014). The second machine age: work, progress, and prosperity in a time of brilliant technologies. WW Norton Company.
Cao, B., Li, L., Zhang, K., and Ma, (2024). The influence of digital intelligence transformation on carbon emission reduction in manufacturing firms. J. Environ. Manag. 367, 121987. doi:10.1016/j.jenvman.2024.121987
Caviggioli, F. (2016). Technology fusion: identification and analysis of the drivers of technology convergence using patent data. Technovation 55, 22–32. doi:10.1016/j.technovation.2016.04.003
Chen, X., Hu, D., Cao, W., Liang, W., Xu, X., Tang, X., et al. (2021). Path of digital technology promoting realization of carbon neutrality goal in China’s energy industry. Bull. Chin. Acad. Sci. 36 (9), 1019–1029. doi:10.16418/j.issn.1000-3045.20210807004
Curran, C. S., and Leker, J. (2011). Patent indicators for monitoring convergence–examples from NFF and ICT. Technol. Forecast. Soc. Change 78 (2), 256–273. doi:10.1016/j.techfore.2010.06.021
Di Vaio, A., Palladino, R., Pezzi, A., and Kalisz, D. E. (2021). The role of digital innovation in knowledge management systems: a systematic literature review. J. Bus. Res. 123, 220–231. doi:10.1016/j.jbusres.2020.09.042
Feng, S., An, H., Li, H., Qi, Y., Wang, Z., Guan, Q., et al. (2020). The technology convergence of electric vehicles: exploring promising and potential technology convergence relationships and topics. J. Clean. Prod. 260, 120992. doi:10.1016/j.jclepro.2020.120992
Flammer, C., and Kacperczyk, A. (2016). The impact of stakeholder orientation on innovation: evidence from a natural experiment. Manag. Sci. 62 (7), 1982–2001. doi:10.1287/mnsc.2015.2229
Funk, R. J., and Owen-Smith, J. (2017). A dynamic network measure of technological change. Manag. Sci. 63 (3), 791–817. doi:10.1287/mnsc.2015.2366
Gauch, S., and Blind, K. (2015). Technological convergence and the absorptive capacity of standardisation. Technol. Forecast. Soc. Change 91, 236–249. doi:10.1016/j.techfore.2014.02.022
Geum, Y., Kwak, R., and Park, Y. (2012). Modularizing services: a modified HoQ approach. Comput. Industrial Eng. 62 (2), 579–590. doi:10.1016/j.cie.2011.11.006
Goldfarb, A., and Tucker, C. (2019). Digital economics. J. Econ. literature 57 (1), 3–43. doi:10.1257/jel.20171452
Griliches, Z., Pakes, A., and Hall, B. H. (1987). The value of patents as indicators of inventive activity. Econ. Policy Technol. Perform., 97–124. doi:10.1017/cbo9780511559938.006
Hacklin, F. (2008). Management of convergence in innovation: strategies and capabilities for value creation beyond blurring industry boundaries. Springer Science Business Media.
Hacklin, F., and Wallin, M. W. (2013). Convergence and interdisciplinarity in innovation management: a review, critique, and future directions. Serv. Industries J. 33 (7-8), 774–788. doi:10.1080/02642069.2013.740471
Han, Y., Li, Z., Feng, T., Qiu, S., Hu, J., Yadav, K. K., et al. (2024). Unraveling the impact of digital transformation on green innovation through microdata and machine learning. J. Environ. Manag. 354, 120271. doi:10.1016/j.jenvman.2024.120271
Jaffe, A. B., Newell, R. G., and Stavins, R. N. (2002). Environmental policy and technological change. Environ. Resour. Econ. 22, 41–69. doi:10.1023/A:1015519401088
Jeong, Y. K., Song, M., and Ding, Y. (2014). Content-based author co-citation analysis. J. Inf. 8 (1), 197–211. doi:10.1016/j.joi.2013.12.001
Karvonen, M., and Kässi, T. (2013). Patent citations as a tool for analysing the early stages of convergence. Technol. Forecast Soc. Change 80 (6), 1094–1107. doi:10.1016/j.techfore.2012.05.006
Kim, C., Kim, S., and Kim, M. S. (2012). Identifying relationships between technology-based services and ICTs: a patent analysis approach. Int. J. Inf. Commun. Eng. 5 (12), 1948–1952. doi:10.5281/zenodo.1083513
Kim, E., Cho, Y., and Kim, W. (2014). Dynamic patterns of technological convergence in printed electronics technologies: patent citation network. Scientometrics 98 (2), 975–998. doi:10.1007/s11192-013-1104-7
Kim, J., and Lee, S. (2017). Forecasting and identifying multi-technology convergence based on patent data: the case of IT and BT industries in 2020. Scientometrics 111 (1), 47–65. doi:10.1007/s11192-017-2275-4
Kim, K., Jung, S., and Hwang, J. (2019). Technology convergence capability and firm innovation in the manufacturing sector: an approach based on patent network analysis. Res. Dev. Manag. 49 (4), 595–606. doi:10.1111/radm.12350
Kim, K., Jung, S., Hwang, J., and Hong, A. (2017). A dynamic framework for analyzing technology standardisation using network analysis and game theory. Technol. Anal. Strateg. Manag. 30 (5), 540–555. doi:10.1080/09537325.2017.1340639
Kodama, F. (1991). Analyzing Japanese high technologies: the techno paradigm shift. London: England Pinter Publishers.
Konadu, R., Ahinful, G. S., Boakye, D. J., and Elbardan, H. (2022). Board gender diversity, environmental innovation and corporate carbon emissions. Technol. Forecast. Soc. Change 174, 121279. doi:10.1016/j.techfore.2021.121279
Kose, T., and Sakata, I. (2019). Identifying technology convergence in the field of robotics research. Technol. Forecast. Soc. Change 146, 751–766. doi:10.1016/j.techfore.2018.09.005
Li, C., Wan, J., Xu, Z., and Lin, T. (2021). Impacts of green innovation, institutional constraints and their interactions on high-quality economic development across China. Sustainability 13 (9), 5277. doi:10.3390/su13095277
Lin, B., and Ma, R. (2022). Green technology innovations, urban innovation environment and CO2 emission reduction in China: fresh evidence from a partially linear functional-coefficient panel model. Technol. Forecast. Soc. Change 176, 121434. doi:10.1016/j.techfore.2021.121434
Liu, B., Li, Z., and Fu, Y. (2024a). Financial performance response of manufacturers to servitization: curvilinear relationship mediated by service business focus and moderated by digital technology application. J. Serv. Theory Pract. 34, 571–597. doi:10.1108/jstp-07-2023-0200
Liu, Y., Wang, J., Wen, F., and Wu, C. (2024b). Climate policy uncertainty and bank systemic risk: a creative destruction perspective. J. Financial Stab. 73, 101289. doi:10.1016/j.jfs.2024.101289
Loeser, F., Recker, J., Brocke, J. V., Molla, A., and Zarnekow, R. (2017). How IT executives create organizational benefits by translating environmental strategies into Green IS initiatives. Inf. Syst. J. 27 (4), 503–553. doi:10.1111/isj.12136
Lu, Q., Wang, X., and Wang, Y. (2023). Enhancing supply chain resilience with supply chain governance and finance: the enabling role of digital technology adoption. Bus. Process Manag. J. 29 (4), 944–964. doi:10.1108/bpmj-11-2022-0601
Luo, G., Guo, J., Yang, F., and Wang, C. (2023). Environmental regulation, green innovation and high-quality development of enterprise: evidence from China. J. Clean. Prod. 418, 138112. doi:10.1016/j.jclepro.2023.138112
Nambisan, S., Wright, M., and Feldman, M. (2019). The digital transformation of innovation and entrepreneurship: progress, challenges and key themes. Res. Policy 48 (8), 103773. doi:10.1016/j.respol.2019.03.018
Nikzad, R., and Sedigh, G. (2017). Greenhouse gas emissions and green technologies in Canada. Environ. Dev. 24, 99–108. doi:10.1016/j.envdev.2017.01.001
Ning, J., Jiang, X., and Luo, J. (2023). Relationship between enterprise digitalization and green innovation: a mediated moderation model. J. Innovation Knowl. 8 (1), 100326. doi:10.1016/j.jik.2023.100326
No, H. J., and Park, Y. (2010). Trajectory patterns of technology fusion: trend analysis and taxonomical grouping in nanobiotechnology. Technol. Forecast Soc. Change 77 (1), 63–75. doi:10.1016/j.techfore.2009.06.006
Nordmann, A. (2004). Converging technologies, shaping the future of European societies. Luxembourg: Office for Official Publications of the European Communities.
Noussan, M., and Tagliapietra, S. (2020). The effect of digitalization in the energy consumption of passenger transport: an analysis of future scenarios for Europe. J. Clean. Prod. 258, 120926. doi:10.1016/j.jclepro.2020.120926
Park, C., and Heo, W. (2020). Review of the changing electricity industry value chain in the ICT convergence era. J. Clean. Prod. 258, 120743. doi:10.1016/j.jclepro.2020.120743
Radicic, D., and Petković, S. (2023). Impact of digitalization on technological innovations in small and medium-sized enterprises (SMEs). Technol. Forecast. Soc. Change 191, 122474. doi:10.1016/j.techfore.2023.122474
Ran, L., Wang, K., Sun, J., and Qu, Y. (2024). Can digital technology remove impediments in the dual circulation? An enterprise cycle efficiency perspective. Manag. Decis. Economics45 45 (3), 1594–1610. doi:10.1002/mde.4079
Ren, X., Li, W., Cheng, X., and Zheng, X. (2024a). Economic freedom and corporate carbon emissions: international evidence. Bus. Strategy Environ., 1–25. doi:10.1002/bse.3920
Ren, X., Xiao, Y., Xiao, S., Jin, Y., and Taghizadeh-Hesary, F. (2024b). The effect of climate vulnerability on global carbon emissions: evidence from a spatial convergence perspective. Resour. Policy 90, 104817. doi:10.1016/j.resourpol.2024.104817
Roco, M. C., and Bainbridge, W. S. (2002). Converging technologies for improving human performance: integrating from the nanoscale. J. Nanoparticle Res. 4, 281–295. doi:10.1023/a:1021152023349
San Kim, T., and Sohn, S. Y. (2020). Machine-learning-based deep semantic analysis approach for forecasting new technology convergence. Technol. Forecast. Soc. Change 157, 120095. doi:10.1016/j.techfore.2020.120095
Sun, X., Xiao, S., Ren, X., and Xu, B. (2023). Time-varying impact of information and communication technology on carbon emissions. Energy Econ. 118, 106492. doi:10.1016/j.eneco.2022.106492
Sung, K., Kim, T., and Kong, H. K. (2010). Microscopic approach to evaluating technological convergence using patent citation analysis. Proceedings Springer Berlin Heidelberg, 188–194.
Tang, L., Jiang, H., Hou, S., Zheng, J., and Miao, L. (2023). The effect of enterprise digital transformation on green technology innovation: a quantitative study on Chinese listed companies. Sustainability 15 (13), 10036. doi:10.3390/su151310036
Wang, X., Wang, X., Ren, X., and Wen, F. (2022). Can digital financial inclusion affect CO2 emissions of China at the prefecture level? Evidence from a spatial econometric approach. Energy Econ. 109, 105966. doi:10.1016/j.eneco.2022.105966
Wang, Z., Fu, H., Ren, X., and Gozgor, G. (2024). Exploring the carbon emission reduction effects of corporate climate risk disclosure: empirical evidence based on Chinese A-share listed enterprises. Int. Rev. Financial Analysis 92, 103072. doi:10.1016/j.irfa.2024.103072
Wang, Z. R., Fu, H. Q., and Ren, X. H. (2023). Political connections and corporate carbon emission: new evidence from Chinese industrial firms. Technol. Forecast. Soc. Change 188, 122326. doi:10.1016/j.techfore.2023.122326
Weina, D., Gilli, M., Mazzanti, M., and Nicolli, F. (2016). Green inventions and greenhouse gas emission dynamics: a close examination of provincial Italian data. Environ. Econ. Policy Stud. 18, 247–263. doi:10.1007/s10018-015-0126-1
Wu, B., Wen, F., Zhang, Y., and Huang, Z. J. (2024). Climate risk and the systemic risk of banks: a global perspective. J. Int. Financial Mark. Institutions Money 95, 102030. doi:10.1016/j.intfin.2024.102030
Yang, H., Li, L., and Liu, Y. (2022). The effect of manufacturing intelligence on green innovation performance in China. Technol. Forecast. Soc. Change 178, 121569. doi:10.1016/j.techfore.2022.121569
Yoo, Y., Boland, R. J., Lyytinen, K., and Majchrzak, A. (2012). Organizing for innovation in the digitized world. Organ. Sci. 23 (5), 1398–1408. doi:10.1287/orsc.1120.0771
Zeng, J. T., and Yang, M. R. (2023). Digital technology and carbon emissions: evidence from China. J. Clean. Prod. 430, 139765. doi:10.1016/j.jclepro.2023.139765
Zhang, H., Gao, S., and Zhou, P. (2023). Role of digitalization in energy storage technological innovation: evidence from China. Renew. Sustain. Energy Rev. 171, 113014. doi:10.1016/j.rser.2022.113014
Keywords: green technology, digital technology, technology convergence, carbon emission reduction, social network analysis
Citation: Hu J, Sun Q and Wang W (2024) The role of green and digital technology convergence on carbon emission reduction: evidence from China. Front. Environ. Sci. 12:1490657. doi: 10.3389/fenvs.2024.1490657
Received: 03 September 2024; Accepted: 15 October 2024;
Published: 24 October 2024.
Edited by:
Xiaohang Ren, Central South University, ChinaCopyright © 2024 Hu, Sun and Wang. This is an open-access article distributed under the terms of the Creative Commons Attribution License (CC BY). The use, distribution or reproduction in other forums is permitted, provided the original author(s) and the copyright owner(s) are credited and that the original publication in this journal is cited, in accordance with accepted academic practice. No use, distribution or reproduction is permitted which does not comply with these terms.
*Correspondence: Wenjing Wang, d2VuamluZ3dhbmdAY3VmZS5lZHUuY24=