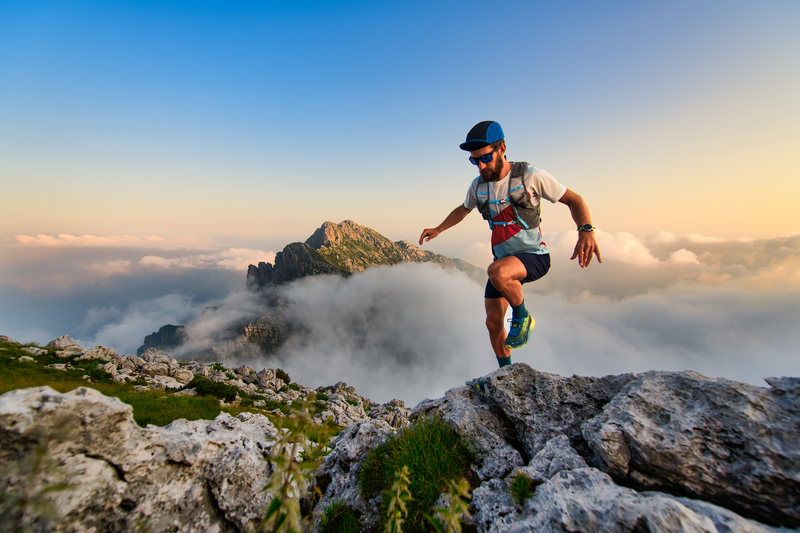
95% of researchers rate our articles as excellent or good
Learn more about the work of our research integrity team to safeguard the quality of each article we publish.
Find out more
ORIGINAL RESEARCH article
Front. Environ. Sci. , 25 October 2024
Sec. Freshwater Science
Volume 12 - 2024 | https://doi.org/10.3389/fenvs.2024.1488955
A comprehensive understanding of the prevailing pollution and other impairments to reservoirs is necessary at the national level to analyze patterns and causes as well as allocate national resources effectively. Most of these impairments are associated with sedimentation, nutrient contamination, and other pollution factors. The costs involved in conducting on-site surveys at multiple locations are potential obstacles for obtaining empirical data on large-scale spatial impairments. In such cases, inputs from specialists may be required to offset the absence of empirical data for determining the impairment statuses of thousands of geographically dispersed reservoirs. Thus, our aim was to examine whether expert opinion could offer a comprehensive review of the impairment statuses of numerous reservoirs in the United States. We designed and executed an internet-based survey of reservoir specialists to gather their ratings on visually evident elements of reservoir impairment. To evaluate the ratings, we searched for correlations with factors known to have impacts on reservoir impairment. Canonical correlation analyses indicated that nine metrics used by experts to rate impairment levels on an ordinal scale were correlated with metrics descriptive of the physical qualities of reservoirs, land use in catchments, and prevailing local climate. We thus conclude that expert opinions may facilitate assessments of impairment levels over large geographical areas. Lastly, we define the circumstances under which it is permissible to rely on expert opinions and propose criteria that could improve the quality of the data collected.
Sediments, fertilizers, pesticides, and heavy metals are the primary pollution impairments known to degrade reservoir ecosystems globally (Baker, 1992; Krithiga et al., 2022; Morin-Crini et al., 2022). Reservoirs are particularly vulnerable to these impairments because they are typically constructed to capture large volumes of water and have oversized catchments. Although sediment accumulation is a natural process, it is accelerated by land-use practices linked to agriculture, deforestation, mining, and urban runoff. Sedimentation can change the physical, chemical, and biological components of the ecosystem, resulting in degradation of beneficial uses such as drinking water, navigation, electricity production, flood control, and recreation. Similarly, nutrient accumulation occurs naturally over time but human activities have accelerated the rate and extent of eutrophication through both point-source discharges and non-point loadings of limiting nutrients, such as nitrogen and phosphorus, which have dramatic consequences for drinking water sources, fisheries, and recreational water bodies (Carpenter et al., 2001). Exposure to pesticides is increasing as agriculture continues to intensify, and pesticides have been shown to disrupt the reproductive functions in fish. Heavy metals originate from various sources, including mining, automobile emissions, landfill leaching, manufacturing, as well as domestic and industrial outflows. A common symptom of heavy-metal exposure in fish is immunosuppression that can lead to increased susceptibility to diseases (Bernier et al., 1995). In addition to heavy metals, a suite of other chemicals from industrial and domestic effluents has become increasingly prevalent in aquatic environments (Schwarzenbach et al., 2010); these include chlorides, sulfides, nitrates, oxides, pharmaceutics, and microplastics (Choudri et al., 2018; Jan et al., 2023).
The effects of pollutants can be invisible to the naked eye, may not be obvious immediately, and are often difficult to document. This is particularly true in reservoirs as these aquatic systems are effectively islands of diverse sizes and accessibilities embedded in continental land masses (Magnuson et al., 1998). Such isolation promotes diversity of aquatic environments and also their impacts. Owing to the dispersed nature of reservoir resources, pollutants may go unmonitored, unregulated, and unmanaged. Some reservoir systems may be managed with sparse monitoring data, while others are managed through regional general policies. Personnel and funds are prioritized to concentrate on key high-visibility reservoir systems. Often, expert opinions may be the best available and actionable information regarding complex environmental systems (Krueger et al., 2012).
Expert opinions are often sought by regulatory agencies when there are insufficient empirical data to quantify a problem. Evidence-based management centered on the monitoring of key metrics is considered as the gold standard of empirical evidence. However, when backed with direct field monitoring experience, expert opinions represent an alternative form of environmental knowledge that can be complementary or alternative to empirical evidence. According to Failing and Gregory (2003) collective expert knowledge remains underutilized because scientists have a propensity to shun the knowledge living in scientific minds as well as coming from experience and extensive acquaintance with an environment. Inputs from experts are sometimes necessary to overcome the lack of empirical data for managing thousands of geographically scattered reservoirs. However, when considering such expert knowledge, we should rely on evidence and careful reasoning as well as seek to eliminate any potential sources of bias (Brownstein et al., 2018).
The aim of our study was to examine whether expert opinions could be used to gain an understanding of the pollution impairment statuses of reservoir resources over wide geographical areas of the United States. We employed a two-step methodology in this study. First, we designed and conducted an online survey of reservoir specialists to obtain their ratings of visually evident elements of reservoir impairment. Second, to evaluate these ratings, we searched for relationships with factors that are known to impact the impairments, such as the physical qualities of reservoirs, land use in catchments, and prevailing local climate. Large-scale spatial evaluations made feasible by relying on expert opinions could provide insights into reservoir sustainability challenges relevant to impairments that are only apparent when the reservoir resources are considered over wide geographical areas.
We included reservoirs within the continental United States that impound a river and have surface areas equal to or exceeding 100 ha in surface area. Natural lakes with dams or water control structures were excluded from this study. A sampling frame of nearly 4,600 reservoirs was identified using the National Inventory of Dams (NID) database (https://nid.sec.usace.army.mil/#/). Because the NID does not distinguish dams constructed to impound rivers from those constructed to manage water levels in natural lakes, we relied on the LAGOS-US RESERVOIR database (Rodriguez et al., 2023) to eliminate natural lakes that had dams installed.
Impairment status was obtained through an online survey of a panel of natural resources specialists familiar with the reservoirs under their supervision (Krogman and Miranda, 2016). These specialists were frequently state agency biologists who were matched to a roster of reservoirs by agency administrators. The survey comprised over fifty questions pertaining to a variety of reservoir environmental issues. We selected nine issues concerning various facets of pollution and impairment typically associated with reservoirs (Table 1). The impairment status was scored by the respondents on a six-point ordinal impairment scale with ratings from 0 to 5, where 0 = no impairment, 1 = low impairment, 2 = low-to-moderate impairment, 3 = moderate impairment, 4 = moderate-to-high impairment, and 5 = high impairment. An expanded explanation was provided for each question to promote respondent consistency. The participants were advised to interpret the scale in accordance with the generally acknowledged criteria for high impairment in their areas of the country.
Table 1. Impairment variables included in the online survey. Data were collected using a six-point ordinal impairment scale ranging from 0 to 5, where 0 = no, 1 = low, 2 = low-to-moderate, 3 = moderate, 4 = moderate-to-high, and 5 = high impairments. The description for each metric was available to the respondents of the survey. The participants were advised to interpret the scale anchored on the regional high impairment criteria.
The link for the online survey was distributed to the specialists. After an introduction outlining the purpose of the survey as well as the voluntary and confidential nature of the responses, the respondents were asked to complete the survey for reservoirs under their oversight. Reservoirs that the respondents were unfamiliar with, including those owned privately or not included in regular monitoring, were excluded to discourage speculation outside of the respondents’ expertise. Non-respondents were contacted multiple times to encourage participation. We concluded the survey after 3 months when the rate of return from the respondents had declined to nearly zero.
We examined correlations between the ratings and three elements relevant to impairment: (1) physical characteristics of the reservoirs, (2) land cover in the catchments, and (3) local climate. The physical characteristics were represented using seven descriptors of reservoir attributes (Table 2) available in a dataset compiled by Rodgers (2017). The land cover in the catchments represented nine major classes used commonly for regional and other large-scale spatial applications (Table 2), which was also sourced from Rodgers (2017). The selection of local climate variables encompassed representations of temperature and precipitation patterns (Table 2), which were derived from a pool of 19 bioclimatic indicators proposed by Fick and Hijmans (2017). These indicators were constructed on the basis of monthly measurements of temperature and rainfall.
Table 2. Mean and coefficient of variability (CV) for the reservoir attributes, local climate, and watershed landcover covariates included in the analysis of 1,090 U.S. reservoirs.
We applied canonical correlation analysis (CCA) to assess the relationships between the impairment ratings and the three sets of reservoir attributes anticipated to influence impairment. CCA reconstructs each set of variables into multiple pairs of synthetic variables. Each synthetic variable is generated such that it yields the largest possible canonical correlation between the two corresponding sets of variables. The canonical correlation is a Pearson r between a pair of synthetic variables. Any residual variance unexplained by the first pair of synthetic variables was used to create a second pair that was orthogonal (i.e., uncorrelated) to the previous pairs. Analogous to the principal component analysis, this process is repeated until all the variability is explained by subsequent pairs of synthetic variables. To meet the assumptions of the CCA, transformations were applied to most variables, including monotonic transformation to ordinal data, logarithmic transformation to skewed physical characteristics, and arcsine transformation to percentage data. Additionally, the reservoir physical characteristics, land cover, and climate variables were standardized to mean = 0 and SD = 1 values to account for the differences in magnitudes and units.
To assess the resulting canonical correlations, we first evaluated the overall canonical model using Wilks’ lambda (λ) to determine correlations between two sets of variables. In a multivariate procedure, λ is used to determine the proportion of variability in one set of variables that cannot be accounted for by the variations in the second set of variables. If λ was statistically significant (p ≤ 0.05), we examined the pairs of synthetic variables produced by the CCA. Each successive pair progressively accounts for a smaller proportion of the total variation in the overall canonical correlation until the pairs are unable to sufficiently explain the variation to justify interpretation. Lastly, for the pairs having correlations ≥0.5 or ≤ -0.5, we assessed the metrics associated with each synthetic variable in terms of the correlation intensity (also ≥0.5 or ≤ -0.5) and directionality. Analyses were conducted with the cancorr procedure (SAS Institute Inc, 2023).
Complete response data on the nine impairment ratings were obtained from 203 specialists for 1,090 reservoirs distributed across the United States (Figure 1). This sample represents nearly 25% of the approximately 4,600 reservoirs having areas ≥100 ha identified in the NID database. The highest rated metric was sedimentation (Table 1), with 29.6% of the reservoirs being scored as 4 or 5 (i.e., above average to high impairment). Next, in decreasing order were non-point-source pollution (21.6% scored as 4 or 5), excessive nutrients (21.5%), and inorganic turbidity (20.3%). Thermal pollution (3.9% scored as 4 or 5) and point-source pollution (5.8%) generally received low effect ratings.
Figure 1. Locations of the 1,090 reservoirs distributed throughout the conterminous United States and evaluated by experts for impairment status in this study. Each point represents a single reservoir.
The CCA revealed various associations between the set of impairment descriptors and the physical characteristics of reservoirs, land cover in reservoir catchments, and local climate. For the impairment concerning physical characteristics of the reservoirs, the full model of the CCA across all functions was statistically significant (λ = 0.47, F(54, 4349) = 6.1, p < 0.001). For the impairment concerning land cover in catchments, the full model CCA across all functions was statistically significant (λ = 0.37, F(81, 4100) = 7.8, p < 0.001). For the impairment regarding local climate, the full model CCA across all functions was also statistically significant (λ = 0.43, F(72, 6534) = 7.8, p < 0.001). Because the Wilks’s λ value represents the variance unexplained by the model, (1– λ) yields the full model effect size in an R2 metric. Thus, the full models accounted for 53% of the variance shared with the physical characteristics of the reservoirs, 63% of the variance shared with the land cover in the catchments, and 57% of the variance shared with the local climate.
The physical characteristics of the reservoirs were canonically correlated with the impairment metrics (Figure 2). The first two canonical functions had significant (p ≤ 0.05) correlations ≥0.5 and were interpreted. Here, the first canonical function had a correlation of 0.59 and demonstrated an association between most impairment metrics and mostly three reservoir attributes (Figure 2A); in this canonical function, all impairment metrics except for three (i.e., algae, thermal, and contaminants) were positively correlated with drainage area, maximum discharge, and drainage/surface area ratio. Thus, the impairment levels increased with catchment size, catchment size relative to reservoir size, and extent of discharge from the reservoir, which are indicative of the amount and rate of water flowing into a reservoir. The second canonical function had a correlation of 0.51 and demonstrated inverse associations between nutrients, algae, and organic turbidity as well as reservoir depth, volume, and discharge (Figure 2B). Thus, the impairment levels decreased in deep reservoirs with large volumes and high flow-through rates. Thermal pollution and contaminants were not related with the considered physical characteristics of the reservoirs.
Figure 2. Canonical correlation analysis. The orange rectangles represent the impairment metrics, and beige rectangles indicate the reservoir attributes: (A) canonical axis 1; (B) canonical axis 2. The center values represent the Pearson correlations between each of the canonical axes; the value next to each rectangle represents the Pearson correlation between each variable and its canonical axis. Thus, high Pearson correlations identify variables that have high influences on the canonical axes.
The climate conditions were next canonically correlated with the impairment metrics (Figure 3). The first canonical function had significant (p < 0.05) correlations ≥0.5 and was interpreted. This first function had a correlation of 0.59 and demonstrated an association between most of the impairment metrics and four climatic conditions (Figure 3). In the first canonical function, all impairment metrics except for three (i.e., thermal, contaminants, and point-source) were positively correlated with temperature seasonality and annual range as well as inversely correlated with minimum temperature during the coldest month and precipitation during the driest month. The three impairments that were seemingly unassociated with climate showed no strong correlations with the climate variables in the two remaining significant canonical factors (not shown).
Figure 3. Canonical correlation analysis. The orange rectangles represent the impairment metrics, and blue rectangles show the climate attributes defined in Table 2. Only canonical axis 1 is shown here. The center values represent the Pearson correlations between each of the canonical axes; the value next to each rectangle represents the Pearson correlation between each variable and its canonical axis. Thus, high Pearson correlations indicate variables that have high influences on the canonical axis.
The landcover composition was canonically correlated with impairment metrics (Figure 4). Only the first canonical function had a significant (p < 0.05) correlation ≥0.5 and was interpreted. The first function, with a correlation of 0.68, provided evidence that increasing the proportions of crops and pastures in a watershed increased the nutrients, sediments, organic and inorganic turbidities, and non-point-source pollution. The opposite effects were facilitated by the forested catchments.
Figure 4. Canonical correlation analysis. The orange rectangles represent the impairment metrics, and green rectangles show the landcover attributes defined in Table 2. Only canonical axis 1 is shown here. The center values represent the Pearson correlations between each of the canonical axes; the value next to each rectangle represents the Pearson correlation between each variable and its canonical axis. Thus, high Pearson correlations indicate variables that have high influences on the canonical axis.
Expert opinions incorporate qualitative information from personnel who are most familiar with a system. Although expert opinions are sometimes considered less robust than quantitative data collected from field surveys, they are valuable alternatives in situations where in situ data are unavailable, including the large-scale spatial assessment presented here. However, where available, we suggest prioritizing empirical evidence over expert opinions in the management of reservoir impairments. This study offers insights into the general patterns of reservoir impairment that can be used to guide future research. Our analysis indicates that expert opinions on impairment aspects were often correlated with covariates that were anticipated to influence impairments, including the local climate, reservoir attributes, and surrounding landcover, thereby supporting the use of expert opinions in ecological research. It is understandable that certain impairments showed weak correlations with the covariates investigated herein as they are likely associated with reservoir characteristics that were not captured in our covariate selection. Although some impairments did not show correlations with the covariates under consideration, it does not imply that the expert judgments were incorrect. Indeed, it is not unreasonable to assume that the impairments with limited correlations to the covariates are estimated with a precision level comparable to those that are correlated, considering that they originate from the same survey and respondents.
The correlations that we identified between the impairments and selected covariates were consistent with the results of empirical studies, thereby providing support for reliance on expert judgment. Davies et al. (2010), Wallace et al. (2018), and Lee et al. (2022) showed that impairment levels were related to the catchment sizes, as predicted by the first canonical function of the reservoir physical features in our study. Similarly, reduced nutrients and algae in deeper, high-volume lakes were previously reported by Taranu and Gregory-Eaves (2008) and Miranda (2011, 2017), as suggested in this study by the second canonical function of reservoir physical features. Generally and consistent with the observed canonical correlations between the impairments and climate metrics, it has been documented that temperature and precipitation variations affect pollutant toxicities (Noyes et al., 2009). The composition of a watershed region, particularly its agricultural composition, has frequently been observed to correspond with the impairment levels, and this association was well-represented by the expert opinions. Thus, we conclude that expert opinions may be used to assess reservoir impairment levels over wide geographical areas. Below, we explore when and how these opinions may be applicable.
Relying on expert opinions rather than empirical surveys may be appropriate in some scenarios (Drescher et al., 2013). For example, when empirical data are scarce or unavailable, expert opinions can provide valuable insights based on knowledge and experience (Ryder et al., 2010; Runge et al., 2020). The U.S. Environmental Protection Agency recurrently conducts an onsite National Lake Assessment of the lakes and reservoirs in the country (USEPA, 2024). This national survey is arguably one of the most extensive aquatic surveys globally, yet it lacks the reservoir breadth attained through our online expert opinion survey likely because of its different objectives. Moreover, when a study has broad spatial applicability over multiple jurisdictions that are fragmented or have limited standardization among their monitoring programs (Hughes et al., 2000), it may be challenging to gather a complete dataset (Krogman and Miranda, 2016). In situations where urgent decisions are necessary and there is insufficient time to collect relevant empirical data, expert opinions can provide immediate guidance (Bolam et al., 2019; Regan et al., 2005; Schmoldt et al., 2001). Expert opinions can offer a more complete picture in complex situations when empirical surveys based on limited quantitative metrics may not be able to cover all pertinent elements or subtleties. Thus, experts can help interpret events and circumstances while providing context that might not be apparent from raw survey results. Lastly, in the early stages of research where empirical data are collected, expert opinions can help shape the hypotheses, design of the empirical studies, and identify the key metrics of investigation. Although expert opinions are valuable in these situations, it is essential to consider the potential biases and ensure that the qualifications and experiences of the experts are relevant to the specific context. The potential biases in expert opinions related to probabilistic onsite surveys may include limited expert familiarity with a reservoir, focus on aspects of the environment that the expert finds more relevant, anchoring opinion relative to different standards, and possibly overconfidence. Balancing expert opinions with empirical data, where available, can provide a more robust basis for decision-making.
Adhering to a few criteria may improve the quality of the impairment data obtained through expert opinions (Ericsson et al., 2006; Davis and Ruddle, 2010). It is important that the experts possess adequate understanding of the impairments coupled with local knowledge though extensive experience working at the reservoirs in question. Verifications may be achieved by examining the educational credentials, career track records, and possibly published works of the experts. Occasionally, it may be possible to seek multiple opinions to obtain a range of perspectives, possibly from different agencies managing the reservoir. This may help to mitigate individual biases and provide a broader understanding of the issue. Where possible, we also recommend comparing the expert opinions with available empirical data, as we have considered in this study. There are many potential conflicts of interest or biases that could influence expert opinions, including personal beliefs and professional affiliations. Even if the data are limited, such comparisons can highlight the areas of agreement and discrepancy, providing checkpoints on how and what kinds of experts are selected and questioned to achieve a more balanced view.
The raw data supporting the conclusions of this article will be made available by the authors, without undue reservation.
LM: conceptualization, formal analysis, funding acquisition, supervision, and writing–original draft. DS: formal analysis and writing–review and editing. RK: data curation, methodology, and writing–review and editing.
The authors declare that financial support was received for the research, authorship, and/or publication of this article. Funding was provided by the U.S. Fish and Wildlife Service through the Reservoir Fish Habitat Partnership.
The authors thank Bob Hughes for the constructive review. Any use of trade, firm, or product names is for descriptive purposes only and does not imply endorsement by the U.S. Government.
The authors declare that the research was conducted in the absence of any commercial or financial relationships that could be construed as a potential conflict of interest.
All claims expressed in this article are solely those of the authors and do not necessarily represent those of their affiliated organizations, or those of the publisher, the editors, and the reviewers. Any product that may be evaluated in this article, or claim that may be made by its manufacturer, is not guaranteed or endorsed by the publisher.
Baker, L. A. (1992). Introduction to nonpoint source pollution in the United States and prospects for wetland use. Ecol. Eng. 1, 1–26. doi:10.1016/0925-8574(92)90023-u
Bernier, J., Brousseau, P., Krzystyniak, K., Tryphonas, H., and Fournier, M. (1995). Immunotoxicity of heavy metals in relation to Great Lakes. Environ. Health Perspect. 103 (S9), 23–34. doi:10.1289/ehp.95103s923
Bolam, F. C., Grainger, M. J., Mengersen, K. L., Stewart, G. B., Sutherland, W. J., Runge, M. C., et al. (2019). Using the value of information to improve conservation decision making. Biol. Rev. 94, 629–647. doi:10.1111/brv.12471
Brownstein, N., Louis, T., O’Hagan, A., and Pendergast, J. (2018). The role of expert judgment in statistical inference and evidence-based decision-making. Am. Statistician 73 (S1), 56–68. doi:10.1080/00031305.2018.1529623
Carpenter, S. R., Cole, J. J., Hodgson, J. R., Kitchell, J. F., Pace, M. L., Bade, D., et al. (2001). Trophic cascades, nutrients, and lake productivity: whole-lake experiments. Ecol. Monogr. 71, 163–186. doi:10.2307/2657215
Choudri, B. S., Charabi, Y., and Ahmed, M. (2018). Effects of pollution on freshwater organisms. Water Environ. Res. 90, 1723–1747. doi:10.2175/106143018x15289915807399
Davies, B. R., Biggs, J., Williams, P. J., Lee, J. T., and Thompson, S. (2010). A comparison of the catchment sizes of rivers, streams, ponds, ditches and lakes: implications for protecting aquatic biodiversity in an agricultural landscape. Pond Conservation Eur., 7–17. doi:10.1007/978-90-481-9088-1_2
Davis, A., and Ruddle, K. (2010). Constructing confidence: rational skepticism and systematic enquiry in local ecological knowledge research. Ecol. Appl. 20, 880–894. doi:10.1890/09-0422.1
Drescher, M., Perera, A. H., Johnson, C. J., Buse, L. J., Drew, C. A., and Burgman, M. A. (2013). Toward rigorous use of expert knowledge in ecological research. Ecosphere 4, 1–26. doi:10.1890/es12-00415.1
Ericsson K. A., Charness N., Feltovitch P. J., and Hoffman R. R. (2006). The Cambridge handbook of expertise and expert performance (New York, New York, USA: Cambridge University Press).
Failing, L., and Gregory, R. (2003). Ten common mistakes in designing biodiversity indicators for forest policy. J. Environ. Manag. 68, 121–132. doi:10.1016/s0301-4797(03)00014-8
Fick, S. E., and Hijmans, R. J. (2017). WorldClim 2: new 1-km spatial resolution climate surfaces for global land areas. Int. J. Climatol. 37, 4302–4315. doi:10.1002/joc.5086
Hughes, R. M., Paulsen, S. G., and Stoddard, J. L. (2000). EMAP-Surface Waters: a national, multiassemblage, probability survey of ecological integrity. Hydrobiologia 422/423, 429–443.
Jan, S., Mishra, A. K., Bhat, M. A., Bhat, M. A., and Jan, A. T. (2023). Pollutants in aquatic system: a frontier perspective of emerging threat and strategies to solve the crisis for safe drinking water. Environ. Sci. Pollut. Res. 30, 113242–113279. doi:10.1007/s11356-023-30302-4
Krithiga, T., Sathish, S., Renita, A. A., Prabu, D., Lokesh, S., Geetha, R., et al. (2022). Persistent organic pollutants in water resources: fate, occurrence, characterization and risk analysis. Sci. Total Environ. 831, 154808. doi:10.1016/j.scitotenv.2022.154808
Krogman, R. M., and Miranda, L. E. (2016). Rating US reservoirs relative to fish habitat condition. Lake Reserv. Manag. 32, 51–60. doi:10.1080/10402381.2015.1121307
Krueger, T., Page, T., Hubacek, K., Smith, L., and Hiscock, K. (2012). The role of expert opinion in environmental modelling. Environ. Model. and Softw. 36, 4–18. doi:10.1016/j.envsoft.2012.01.011
Lee, J., Bae, S., Lee, W. H., and Gil, K. (2022). Effect of surface area to catchment area ratio on pollutant removal efficiency in vegetation-type facilities. Ecol. Eng. 179, 106609. doi:10.1016/j.ecoleng.2022.106609
Magnuson, J. J., Tonn, W. M., Banerjee, A., Toivonen, J., Sanchez, O., and Rask, M. (1998). Isolation vs. extinction in the assembly of fishes in small northern lakes. Ecology 79, 2941–2956. doi:10.2307/176528
Miranda, L. E. (2011). Depth as an organizer of fish assemblages in floodplain lakes. Aquat. Sci. 73, 211–221. doi:10.1007/s00027-010-0170-7
Miranda, L. E. (2017). Reservoir fish Habitat management. Totowa, NJ, United States: Lightning Press.
Morin-Crini, N., Lichtfouse, E., Liu, G., Balaram, V., Ribeiro, A. R. L., Lu, Z., et al. (2022). Worldwide cases of water pollution by emerging contaminants: a review. Environ. Chem. Lett. 20, 2311–2338. doi:10.1007/s10311-022-01447-4
Noyes, P. D., McElwee, M. K., Miller, H. D., Clark, B. W., Van Tiem, L. A., Walcott, K. C., et al. (2009). The toxicology of climate change: environmental contaminants in a warming world. Environ. Int. 35, 971–986. doi:10.1016/j.envint.2009.02.006
Regan, H. M., Ben-Haim, Y., Langford, B., Wilson, W. G., Lundberg, P., Andelman, S. J., et al. (2005). Robust decision-making under severe uncertainty for conservation management. Ecol. Appl. 15, 1471–1477. doi:10.1890/03-5419
Rodgers, K. D. (2017). A reservoir morphology database for the conterminous United States. U.S. Geol. Surv. data release. doi:10.5066/F7GF0RQZ
Rodriguez, L. K., Polus, S. M., Matuszak, D. I., Domka, M. R., Hanly, P. J., Wang, Q., et al. (2023). LAGOS-US RESERVOIR: a database classifying conterminous US lakes 4 ha and larger as natural lakes or reservoir lakes. Limnol. Oceanogr. Lett. 8, 267–285. doi:10.1002/lol2.10299
Runge, M., Converse, S. J., Lyons, J., and Smith, D. (2020). Structured decision making: case studies in natural resource management. doi:10.1353/book.74951
Ryder, D. S., Tomlinson, M., Gawne, B., and Likens, G. E. (2010). Defining and using ‘best available science’: a policy conundrum for the management of aquatic ecosystems. Mar. Freshw. Res. 61, 821–828. doi:10.1071/mf10113
Schmoldt, D. L., Mendoza, G. A., and Kangas, J. (2001). “Past developments and future directions for the AHP in natural resources,” in Natural resource and environmental decision making, 289–305. doi:10.1007/978-94-015-9799-9_18Anal. hierarchy process
Schwarzenbach, R. P., Egli, T., Hofstetter, T. B., von Gunten, U., and Wehrli, B. (2010). Global water pollution and human health. Annu. Rev. Environ. Resour. 35, 109–136. doi:10.1146/annurev-environ-100809-125342
Taranu, Z. E., and Gregory-Eaves, I. (2008). Quantifying relationships among phosphorus, agriculture, and lake depth at an inter-regional scale. Ecosystems 11, 715–725. doi:10.1007/s10021-008-9153-0
USEPA (2024). National Lakes Assessment: the fourth collaborative survey of lakes in the United States. Available at: https://nationallakesassessment.epa.gov/webreport.
Wallace, J. S., Garner, E., Pruden, A., and Aga, D. S. (2018). Occurrence and transformation of veterinary antibiotics and antibiotic resistance genes in dairy manure treated by advanced anaerobic digestion and conventional treatment methods. Environ. Pollut. 236, 764–772. doi:10.1016/j.envpol.2018.02.024
Keywords: lakes, pollution, environmental assessment, expert knowledge, large-scale analysis
Citation: Miranda LE, Shoemaker DJ and Krogman RM (2024) Assessment of pollution and other impairments of US reservoirs based on expert opinion. Front. Environ. Sci. 12:1488955. doi: 10.3389/fenvs.2024.1488955
Received: 03 September 2024; Accepted: 07 October 2024;
Published: 25 October 2024.
Edited by:
Paweł Tomczyk, Wroclaw University of Environmental and Life Sciences, PolandReviewed by:
Robert M. Hughes, Amnis Opes Institute, LLC, United StatesCopyright © 2024 Miranda, Shoemaker and Krogman. This is an open-access article distributed under the terms of the Creative Commons Attribution License (CC BY). The use, distribution or reproduction in other forums is permitted, provided the original author(s) and the copyright owner(s) are credited and that the original publication in this journal is cited, in accordance with accepted academic practice. No use, distribution or reproduction is permitted which does not comply with these terms.
*Correspondence: L. E. Miranda, c21pcmFuZGFAdXNncy5nb3Y=
Disclaimer: All claims expressed in this article are solely those of the authors and do not necessarily represent those of their affiliated organizations, or those of the publisher, the editors and the reviewers. Any product that may be evaluated in this article or claim that may be made by its manufacturer is not guaranteed or endorsed by the publisher.
Research integrity at Frontiers
Learn more about the work of our research integrity team to safeguard the quality of each article we publish.