- School of Architecture and Urban Planning, Shandong Jianzhu University, Jinan, China
The global climate crisis is escalating, and how to reduce land use carbon emission (LUCE) while promoting social and economic development is a global issue. The purpose of this study was to investigate the spatio-temporal evolution characteristics and influencing factors of LUCE at the county scale. To accomplish this goal, based on Zibo County land use data and societal energy consumption statistics, for predicting the net LUCE in 2010, 2015, and 2020. GIS spatial analysis and spatial autocorrelation model were utilized to investigate the spatio-temporal evolution characteristics of LUCE. The geographical and temporal weighted regression (GTWR) model was used to investigate the influencing factors and spatial differences. The findings demonstrate that: (1) the rate of land use change in Zibo City decreased between 2010 and 2020, with the overall land use motivation falling from 0.14% to 0.09%. The area of arable land, forest land, and grassland decreased, while the amount of water, developed land, and unutilized land increased. Between 2010 and 2020, net carbon emissions in Zibo City increased significantly, from 3.011 × 107tC to 3.911 × 107tC. The spatial distribution of LUCE followed a clear pattern of “elevated in the east and diminished in the west, elevated in the south and diminished in the north.” The spatial agglomeration characteristics of LUCE are obvious, and the overall trend of the Moran I value is falling, from 0.219 to 0.212. The elements that determine LUCE vary greatly by location, with the most major influences being, in descending order, energy consumption per unit of GDP, urbanization rate, land-use efficiency, and population size. The energy consumption per unit of GDP has the greatest impact on Linzi District, with coefficients ranging from 55.4 to 211.5. The study clearly depicts the spatio-temporal distribution of carbon emissions resulting from land use in Zibo City and the factors that contribute to them. Simultaneously, it provides a scientific framework for improving land use structure and implementing low-carbon programs throughout the region.
1 Introduction
In terms of the environment, global warming and frequent natural disasters, economic development has resulted in a considerable increase in carbon emissions (Jia et al., 2023; Huang et al., 2024). As a result, tackling the climate change caused by these emissions has become a critical global concern (Meng et al., 2023). Land is the physical medium for human society and environmental processes, and it is inextricably linked to carbon emissions (Xia et al., 2023). According to research, land use and cover changes account for roughly one-third of total human-caused carbon emissions (Zhang et al., 2018). This ranks land use changes as the second most major source of carbon emissions, after fossil fuel combustion (Chen et al., 2023; Zhao et al., 2023). These changes have an important influence on the rise in carbon emissions (Yu et al., 2013; Li et al., 2022).
Currently, China leads the world in carbon dioxide emissions and energy consumption (Lin et al., 2021; Chen et al., 2022). As a result, reducing carbon emissions in China has emerged as a critical international issue. Hence, the Chinese government has regularly expressed China’s plans to cut carbon emissions and combat climate change in major forums to reach a carbon dioxide emission peak by 2030 and be carbon neutral by 2060 (Wang et al., 2022; Yan et al., 2022). Exploring solutions for reducing carbon emissions from land use is crucial for achieving green and low-carbon development goals.
Recently, domestic and international academics have conducted substantial research on carbon emissions from land use. The primary focus of this research is on measuring carbon emissions, as well as the spatial and temporal patterns and factors that influence them. The primary goal of this research is to account for carbon emissions. According to the Intergovernmental Panel on Climate Change (IPCC) carbon emissions inventory, the carbon emissions factor technique has been widely used in carbon emissions accounting due to its scientific, systematic, and simple calculation qualities, as demonstrated by prior research (Han and Ge, 2023). For example, Zhang et al. (2022) used the carbon emissions factor approach to calculate carbon emissions from various land use types in the Yellow River Delta. Their research found that arable land and land earmarked for building were the biggest sources of carbon emissions. Forest land, grassland, watersheds, and undeveloped areas, on the other hand, served as significant carbon sinks in the metropolis (Zhang et al., 2022). Similarly, Meng et al. (2023) used the carbon emissions coefficient approach to quantify carbon emissions from land use in nine provinces of the Yellow River Basin. They discovered that carbon emissions from construction land had a major impact on regional carbon emissions (Meng et al., 2023).
Based on accounting methods, researchers conduct extensive investigations into the geographical and temporal evolution characteristics, as well as the influencing variables, of land use carbon emissions. Existing research by professionals and scholars has analyzed carbon emissions at many scales, including provincial area (Chuai et al., 2015; Dong et al., 2015), city cluster (Zhang et al., 2018; Li L. et al., 2023), city area (Huang et al., 2021; Tang et al., 2022), and metropolitan area (Wang et al., 2023), to analyze carbon emissions’ spatial and temporal patterns. Commonly used methods in these studies include spatial autocorrelation analysis (Wei et al., 2023) and standard deviation ellipse modeling (Cai and Li, 2024). For instance, Cui et al. (2018) employed the spatial autocorrelation method, utilizing Landsat image data and socio-economic statistics, to examine the spatial attributes of carbon emissions in the Yangtze River Delta. The study revealed a noteworthy positive correlation between carbon emissions and regional land use (Cui et al., 2018). Scholars primarily focus on research methods and models when studying the factors that influence a phenomenon. Some commonly used methods and models include the logarithmic mean division index (LMDI) model (Zhang et al., 2021; Zhao et al., 2021; Liu C. X. et al., 2023), geographically weighted regression (GWR) model (Fang et al., 2023), spatio-temporal geographic weighted regression (GTWR) model (Tian et al., 2022; Yang et al., 2024), geographic detector (Song et al., 2022; Zhang et al., 2024) and stochastic impacts by regression on population, affluence, and technology (STIRPAT) model (Zhang and Xu, 2017; Yang and Liu, 2023). For instance, Liu S. T. et al. (2023) employed the LMDI decomposition model to investigate the drivers of carbon emissions from agricultural land in China, and the findings revealed that the level of the agricultural economy plays a significant role in the increase in carbon emissions. Yang and Liu. (2023) conducted a study using a spatio-temporal geographic weighted regression model to examine the factors that influence carbon emissions in Xinjiang. The study revealed that factors such as population size, urbanization rate, and land use efficiency have a significant impact on carbon emissions (Liu S. T. et al., 2023b). Similarly, Guo et al. (2023) employed the STIRPAT model to assess the degree of influence of carbon emissions factors in the production-life-ecological space in Heilongjiang Province (Guo et al., 2023). Additionally, Chen et al. utilized the LMDI model to analyze the factors affecting LUCE in the Northeastern Black Soil Region. Their findings indicated that per capita gross domestic product is decisive in carbon emissions (Chen et al., 2023).
Although domestic and foreign scholars have gradually improved their study on land use carbon emissions, the following shortcomings remain: (1) In terms of research methodology, most scholars use geographic probes, geographically weighted regression, and other models to analyze land use carbon emissions based on the spatial distribution scale, and few scholars have carried out research on land use carbon emissions in both the temporal and spatial dimensions. (2) In terms of the scale of the research, scholars have focused on the geographical areas of provinces, municipalities, urban agglomerations, and metropolitan areas. Provinces, cities, urban agglomerations, metropolitan areas, and other geographical areas to carry out research for the county unit of land use carbon emission mechanisms, effects, and factors affecting relatively few studies, ignoring the spatial heterogeneity within the region, the county is China’s basic administrative unit, and there have been studies showing that counties have a strong potential for carbon reduction; (3) in the content of the study, land use change is one of the important sources of global carbon emissions, but from a land use change is one of the important sources of global carbon emissions, but fewer scholars have conducted research on carbon emissions from the perspective of land use change.
Shandong Province has developed the “Shandong Province ecological and environmental protection industry high-quality development “311” project 3-year action plan (2023–2025) “in the context of “double carbon”. As a modern industrial city and one of the three major ecological and environmental protection industry clusters, Zibo City’s government has issued the “Zibo City Deepening the Conversion of Old and New Dynamics to Promote Green, Low-Carbon, and High-Quality Development Three-Year Action Plan (2023–2025),” which promotes the city’s green, low-carbon growth trend (Zhang and Zhang, 2023). Industrial cities emit more carbon than average cities. And, being a typical contemporary industrial city and a major petrochemical base in China, Zibo City has particular characteristics as a research subject.
In the selection of influential factors, it is found by observing previous studies that these influencing factors are always related to population (Wu et al., 2024), land (Li and Lin, 2022), economy (Zhou and Cao, 2022), and energy (Cao and Yuan, 2019). For example, Cai and Li, (2024) found that the level of urbanization, population size, and economic volume have a significant impact on carbon emissions based on a geodetector study Cai and Li, 2024, and Zhao et al., (2021) used the LMDI model to study the influencing factors of carbon emissions in the Pearl River Delta, and the study showed that the land use change, energy structure, energy efficiency, economic development, and population all contribute to the increase of carbon emissions from land use. Most of these scholars studied the influencing factors of the study area from a certain dimension of time or space, while this paper is based on the GTWR model, which makes it easier to reveal the spatial and temporal heterogeneity of the influencing factors of carbon emissions. Carbon emissions in Zibo City County vary spatially and temporally, and these variations are influenced by a variety of causes. Combining existing studies and Zibo City’s regional characteristics, taking data accessibility into account, this paper screens the influencing factors of land use carbon emissions from the perspectives of population size, land use, socioeconomics, and energy consumption, respectively. Finally, four significant influencing factors were identified: population size, urbanization rate, land use efficiency, and energy use per unit of GDP.
In view of this, this paper analyzes the land use changes and spatial and temporal characteristics of carbon emissions in Zibo City using three periods of land use remote sensing data and social energy consumption data in 2010, 2015, and 2020, and analyzes the carbon emission agglomeration characteristics based on the county unit and 1 km grid scale, and finally analyzes the carbon emission influencing factors of Zibo City’s land use by the spatiotemporal geographical Finally, the GTWR model was used to assess the factors that influence carbon emissions from land use in Zibo City.
In recent years, Zibo city districts and counties have a large gap in the level of development and are faced with industrial transformation, large resource consumption, technological backwardness, and other low-carbon development problems. This paper analyzes the spatial and temporal characteristics of land use carbon emissions and influencing factors based on the perspective of land use change and proposes scientific references for the development of carbon emission reduction.
2 Materials and methods
2.1 Study area
Zibo City is located in Shandong Province’s center region and is part of the Shandong Peninsula City Cluster. It is located west of the provincial capital of Jinan, north of Binzhou, east of Weifang, and south of Linyi. The city’s geographical coordinates are 35°55′20″ to 37°17′14″ north and 117°32′15″ to 118°31′00″ east. The State Council recognizes Zibo City as a modern industrial city, with petrochemicals being the leading industry. The city’s topography features higher heights in the south and lower elevations in the north, as well as mountainous hills in the south. The Yellow River also flows through the northern part of the city (Figure 1). Zibo’s GDP (gross domestic product) was CNY 456.18 billion in 2023, representing a 5.5% increase over the previous year. The territory is now divided into five districts: Linzi, Zichuan, Boshan, Zhangdian, and Zhoucun. Additionally, there are three counties in total: Huantai, Gaoqing, and Yiyuan. Zibo covers 596,500 ha and has a population of 4.7 million residents.
2.2 Research data
The data used in this analysis include land use data, energy consumption data, GDP statistics for districts and counties, and Zibo City’s urbanization rate from prior years. This study’s land use data is based on 2010–2020 land use raster data provided by the Chinese Academy of Sciences Resource and Environment Science and Data Centre. The data has a resolution of 30 m. The 2010–2020 Zibo Statistical Yearbook provides data on energy consumption and GDP for districts and counties (Table 1). To maintain data comparability across years, this analysis uses the administrative division of 2022 as a reference point.
2.3 Methods
Scholars at home and abroad have conducted a large number of studies focusing on the fields of land use carbon emissions accounting, spatial and temporal evolution characteristics of land use carbon emissions, influencing factors of land use carbon emissions, and low carbon land use optimization, with different authors selecting different research methods based on the actual situation of the research object (Figure 2). In the research method of spatial and temporal evolution characteristics, the spatial autocorrelation model can analyze the internal and external characteristics of the observational data of the study area from both global and local aspects, reflecting the spatial clustering characteristics, and the standard deviation ellipse model is often used to analyze the distribution and development trend of a set of data; in the research method of influencing factors, the geodetector explores the relationship between two variables by examining the degree of coupling between the two variables, and the LMDI model can effectively identify and quantify the degree of contribution of each influencing factor, the GWR model is used to explore the characteristics of spatial changes and influencing factors at a certain scale, and the GTWR elaborates the spatial-temporal evolution characteristics of the study content in both time and space dimensions, which is more useful for revealing the spatial-temporal heterogeneity of the different influencing factors within the study area.
Therefore, considering the reality of the study area and the environmental background, the spatial autocorrelation model is more useful for exploring the characteristics of carbon emissions agglomeration in Zibo City, while the time span of the study is large, it is more reasonable to choose the GTWR model to explore the spatiotemporal heterogeneity of the study area in both time and space dimensions.
2.3.1 Land-use dynamics approach
This paper examines the land use dynamics to analyze the changes in land use types in the study area. Land use dynamics refers to the extent of change in the area of each land use type over a specific period of time, reflecting the speed and intensity of the change (Li X. P. et al., 2023). A higher land use dynamics indicates a more significant change in land use types. Hence, the land use dynamics can serve as a clear indicator of the overall transformation of Zibo City or specific land use categories during the course of the study period.
The term “single dynamic attitude” refers to the measurement of the extent and rate of change of a specific land use type within a particular timeframe in the study area. On the other hand, the “comprehensive land use dynamic attitude” is used to assess the overall change of land use in the study area over a specific period of time. This is calculated using the following formula (Equations 1, 2) (Han and Ge, 2023):
Where
2.3.2 Methodologies for measuring LUCE
This study employs the carbon emissions coefficient approach, which is extensively utilized to compute the carbon emissions of various land use kinds. Drawing on extensive research conducted by numerous researchers, the land use types are classified into two categories: carbon source land and carbon sink land (Huang and Zhou, 2022; Cheng et al., 2024). Carbon source land comprises arable land and construction land, whereas carbon sink land encompasses arable land, forest land, grassland, watershed, and unutilized land. The carbon emissions factor method can directly measure arable land, forest land, grassland, watershed, and unutilized land. However, the carbon emissions from construction land primarily stem from human production and life. This can be indirectly calculated using energy consumption data (Li X. et al., 2023). Obtaining energy consumption statistics for development land in the county is challenging. However, this study manages to acquire it by using the proportion allocation of the county to the city’s GDP (Liu C. X. et al., 2023).
2.3.2.1 Methodologies for measuring direct LUCE
The carbon emissions from land usage on cultivated land, forest land, grassland, aquatic areas, and vacant land have shown consistent stability over an extended period. These emissions can be accurately quantified using the carbon emissions coefficient approach. The calculating formula is as follows (Yan et al., 2022):
Where Cz indicates direct LUCE(Unit:tC); i represents land use type;
2.3.2.2 Methodologies for measuring indirect LUCE
Land serves as both a natural source and sink for carbon, as well as a medium for human activities that generate carbon emissions. Indirect carbon emissions are primarily determined based on energy usage statistics. Based on the current circumstances in Zibo City, a total of 10 energy sources have been chosen for computation. These include raw coal, coke, natural gas, crude oil, petrol, diesel, kerosene, fuel oil, liquefied petroleum gas and electricity. The calculating formula is as follows (Yan et al., 2022):
Where
2.3.3 Exploratory spatial data analysis methods
Spatial autocorrelation theory, unlike previous spatial analysis methods, is employed to assess if the spatial arrangement of variables exhibits clustering. It is a prominent approach for quantitatively addressing the issue of spatial correlation in geographic variables. Moreover, it is better suited for uncovering the spatial distribution characteristics of the data and facilitates visual representation. This theory comprises two components: global spatial autocorrelation and local spatial autocorrelation (Zhang et al., 2022).
Global spatial autocorrelation is employed to evaluate the general pattern and trend of the data in order to ascertain if a phenomenon is concentrated in a particular place. On the other hand, the local Moran index is utilized to quantify the areas where high and low attribute values cluster together. This article uses global spatial autocorrelation to examine carbon emissions in Zibo City, while local spatial autocorrelation is utilized to assess the grid units at a 1 km size in the Zibo area.
Spatial autocorrelation is typically quantified using the Moran’s I index, which measures global spatial autocorrelation. The index is constructed as follows (Equations 5, 6) (Wu et al., 2022):
Where
The value of Moran’s I is between (−1,1). The closer the value is to 1, the stronger the spatial positive correlation is; 0 means that there is no spatial autocorrelation; the closer the value is to −1, the stronger the spatial negative correlation is (Tan et al., 2023).
2.3.4 Spatio-temporal geographically weighted regression (GTWR) models
Presently, the prevailing models used for the dissection of influencing components encompass the LMDI decomposition model, the STIRPAT model, the GWR model, and others. This work differs from prior studies by utilizing the approach developed by Huang et al. to include temporal characteristics into the conventional geographically weighted regression model, resulting in the proposal of a GWTR model (Zhou et al., 2021; Rong et al., 2023). The model offers a more accurate explanation of spatial phenomena and effectively addresses the issue of temporal and spatial non-stationarity. It describes the spatio-temporal evolution of the study content in both time and space dimensions and is valuable for uncovering the spatio-temporal heterogeneity of various influencing factors within the study area.
In order to account for the variations in socio-economic environment and natural ecological conditions across the study area, it is imperative to investigate the specific impact of different influencing factors in different regions, taking into consideration both spatial and temporal aspects. The expression for the spatio-temporal geographically weighted regression model (GTWR) is as follows (Equation 7) (Shi et al., 2022):
Where
3 Results
3.1 Land use change
The land uses raster data from Zibo City in 2010, 2015, and 2020 were evaluated with ArcGIS10.6 software. This analysis provided the current land use status of Zibo City for each year and looked into any changes that occurred.
Analyzing land use motivation in Zibo City reveals (Table 4) that the city’s overall attitude towards land use motivation decreased from 0.14% in 2010–2015 to 0.09% in 2015–2020. This suggests that Zibo City’s pace of land use change decreased between 2010 and 2020. Between 2010 and 2015, the amount of cultivated land, forest land, and grassland decreased, while the area of water, construction land, and unused land increased. The most common reason for using construction land was 0.71%. Between 2015 and 2020, the amount of farmed and unutilized land decreased, while the area of forest land, grassland, waterways, and construction land increased. The greatest increase in land use was recorded on unutilized land, reaching 1.58%. Overall, arable land, construction land, and unutilized land changed at a rapid pace between 2010 and 2020. Construction land saw the greatest gain, with a growth rate of 0.59%. Zibo City’s urbanization rate of Zibo City has been continuously increasing in recent years, resulting in increased demand for construction land due to economic development and an extension of the total area of construction land.
Table 5 shows that the types and areas of land use in Zibo City have changed to varied degrees (Figures 2–4). From 2010 to 2020, construction land experienced the greatest rise in the transfer area, growing by 65.168 km2. Water came next, increasing by 0.85 km2. The highest decline in transfer area was found in arable land, with a fall of 61.449 km2. Grassland experienced the second biggest drop, with a reduction of 2.416 km2. The smallest decrease in transfer area was observed in unutilized land, which decreased by 0.25 km2. Between 2010 and 2020, construction land area increased the most, owing mostly to the conversion of arable land. This represented 88.7% of the entire transfer of construction land area. Conversely, arable land area decreased the most, owing primarily to its conversion into construction land. This accounted for 77.7% of the total transfer of arable land area, including interconversion between arable and built-up land. The primary sources of forest land are grassland and cropland, while the primary sources of grassland are cropland and forest land. Farmland is the main contributor to water supply, and unutilized land is reduced in size through land use adjustments. Zibo City’s economic development and land use structure adjustment have accelerated urban construction, resulting in the expansion of construction land into non-construction areas such as cropland, forest land, and grassland, leading to an increase in the total area of construction land.
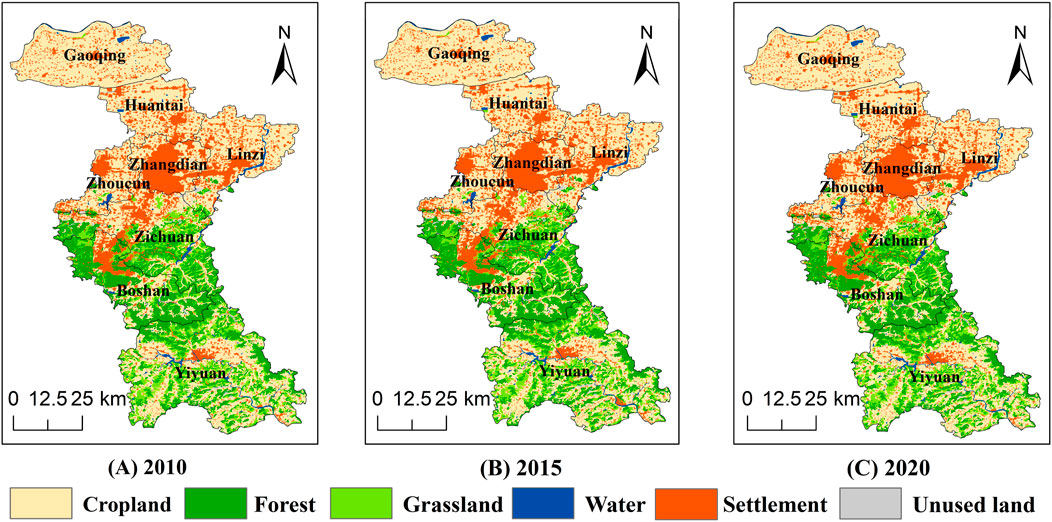
Figure 3. The present situation of land use in Zibo City from 2010 to 2020. (A) 2010, (B) 2015, (C) 2020.
In general, land use in Zibo City has changed significantly during the research period, with an increase in water and construction land and a decrease in arable land, forest land, grassland, and unutilized land. The overall trend in land use dynamics is downward, indicating that land use changes in Zibo City are gradually stabilizing.
3.2 Characteristics of the spatial and temporal evolution of LUCE
3.2.1 Characteristics of temporal evolution
The carbon emissions and carbon sequestration of six different land use types in Zibo City, including cropland, forest land, grassland, watershed, construction land, and unutilized land, were computed using Equations 3, 4, respectively. These land use types were then divided into two main categories: carbon sources and carbon sinks. Table 6 shows a large rise in carbon emissions from 2010 to 2020, from 3.017 × 107t to 3.918 × 107t. This amounts to an average annual growth rate of 9 × 105t. Notably, construction land is the most significant contributor to carbon emissions, accounting for more than 99% of the total. Carbon uptake at the carbon sinks increased gradually between 2010 and 2020, from 63,636.9t to 64,381.8t. The average annual growth rate was 74.49t. Forest land accounted for about 90% of total carbon uptake. To summarize, the rate at which carbon sinks are growing is significantly lower than the rate at which carbon emissions are increasing. Between 2010 and 2020, Zibo City’s net carbon emissions increased quickly from 3.011 × 107t to 3.911 × 107t. This indicates that the land in Zibo City has improved its capacity to absorb carbon, but it is still far from being able to meet the demands of carbon emissions. Additionally, the consumption of fossil energy in Zibo City has increased year by year during the study period, and the proportion of industrial output value has also risen. This is closely linked to Zibo’s positioning as a modern industrial city. In future carbon reduction targets, the government should prioritize the green transformation of enterprises, the research and development of low-carbon technology, and the optimization of land-use structures to enhance energy efficiency.
3.2.2 Characteristics of spatial evolution
To explore the changes in carbon sources and sinks in Zibo City between 2010 and 2020, we calculated the carbon emissions from land use in all districts and counties of Zibo City. We divided the districts and counties into five categories based on their carbon absorption and emissions levels: low value, sub-low value, medium value, sub-high value, and high value. We then used ArcGIS10.6 software to display and represent the data (Figure 5). Figure 5 (2a-2c) shows that the spatial distribution of carbon sinks in Zibo City is reasonably stable. The southern mountainous regions, including Zichuan District, Boshan District, and Yiyuan County, exhibit high carbon sink values due to their dense forest cover. Conversely, northern locations, such as Gaoqing County, Huantai County, and Zhangdian District, have poor carbon sink values because they are primarily agricultural and building terrain. This generates a spatial pattern in which, with the exception of Zhangdian District, Zhoucun District, and Huantai County, carbon sinks in Zibo City other districts and counties decreased between 2010 and 2020. Among them, Zichuan District had the largest decrease, with an average annual decrease of 89.57t. Figure 5 (2d-2f) clearly shows significant spatial changes in the amount of carbon sources in Zibo City County. The carbon sources in each district and county exhibited an upward trend, with Gaoqing County transitioning from a low-value area to a second-low-value area, Huantai County transitioning from a second-high-value area to a high-value area, and Yiyuan County and Zhoucun County transitioning from a second-low-value area to a medium-value area. Overall, the pattern observed is “high in the east, low in the west, and high in the north and south”. Notably, Huantai County experienced the largest increase in carbon emissions. Huantai County exhibits the most significant increase in carbon emissions, with an average annual rise of 1.298 × 105t. Figure 5 (2g-2i) illustrates that the net carbon emissions and carbon sources in Zibo City’s county area follow a similar pattern of change. The regions with high carbon values are primarily situated in Zhangdian and Linzi districts, as well as other central areas. Conversely, the regions with low carbon values are mainly found in the southern and northern areas, including Gaoqing County and Yiyuan County. During the study period, the carbon sink land in Zibo County, including forest land, grassland, waters, unused land, and other land, was unable to absorb the carbon emissions from cropland and construction land. As a result, the overall net carbon emissions increased, with the highest values primarily found in the central and eastern parts of Zibo. Zibo City’s net carbon emissions have continuously followed a regional trend, with higher emissions in the east and south and lower emissions in the west and north. Furthermore, the gap between net carbon emissions in the northern and southern areas has increased. Simultaneously, the carbon emissions coming from the energy consumption during land construction greatly outweigh those from arable land. From 2010 to 2020, carbon sinks only accounted for approximately 0.2% of carbon sources, indicating that relying solely on land carbon absorption is insufficient to offset the carbon emissions from energy consumption. As a result, in Zibo City, the primary focus of carbon reduction measures should be on energy consumption and industrial structure.
3.3 Characteristics of spatial clustering of LUCE
3.3.1 Global spatial autocorrelation
A global spatial autocorrelation study of net carbon emissions in Zibo counties was conducted using ArcGIS10.6 software. Table 7 shows the results, including Moran’s I value, z value, and p-value for net carbon emissions in Zibo counties from 2010 to 2020. Table 3 shows that the Moran’s I values for 2010–2020 are consistently positive. This indicates that counties with high net carbon emissions in the Zibo county area are close to other high-value counties, while low-value counties are close to other low-value counties. Therefore, the net carbon emissions within the Zibo City area show a positive autocorrelation. The test statistic shows that all years from 2010 to 2020 meet the confidence requirement of P< 0.1. From 2010 to 2015, Moran’s I value gradually increased, indicating a rising geographical correlation of net carbon emissions in Zibo City. From 2015 to 2020, Moran’s I value decreased, indicating a weakening trend in the geographical correlation of net carbon emissions in Zibo County. In general, the net carbon emissions of Zibo counties show a noticeable clustering pattern, with some smoothness in the spatial correlation of these emissions. However, the overall trend of Moran’s index is a progressive drop, indicating a reduction in the clustering of net carbon emissions at the Zibo district and county levels. Because the global spatial autocorrelation only shows the worldwide characteristics of carbon emissions from land use in Zibo County, this research uses the local spatial autocorrelation to investigate the local spatial evolution characteristics of carbon emissions from land use in Zibo County.
3.3.2 Local spatial autocorrelation
This study uses ArcGIS10.6 software to examine the internal spatial relationships of net carbon emissions in Zibo City from 2010 to 2020. Zibo City’s net carbon emissions are divided into 1 km-scale grid cells for 2010, 2015, and 2020. Local spatial autocorrelation analysis is then applied to these grid cells. The findings indicate that the local spatial autocorrelation in Zibo City remained reasonably stable from 2010 to 2020. Table 8 and Figure 6 demonstrate that during the last decade, the areas with a high concentration of agglomeration have primarily been in Zhangdian District, Linzi District, Zichuan District, Boshan District, and other central areas of Zibo. This shows a very modest expansion compared to 2010, with Zhangdian District having the most coverage. The concentration of high-low agglomerations is primarily found in Yiyuan and Gaoqing counties. In 2020, the area of high-low agglomerations will gradually extend towards the central region of the country, as compared to 2010. Areas with low-high and high-low agglomeration are primarily found in Zhangdian District, Linzi District, Zichuan District, and other central areas of Zibo. These areas are now smaller than they were in 2010. The low-low agglomeration areas are mainly concentrated in Zichuan District, Boshan District, Yiyuan County, and other southern parts of Zibo. These areas are showing a slow expansion trend. The study demonstrates that the agglomeration effect of high-high and low-low agglomeration areas of net carbon emissions in Zibo City is strengthened. Furthermore, it has been noted that the majority of areas with high (low) values of net carbon emissions are located near together. This means that the disparities in net carbon emissions among the counties of Zibo have escalated.
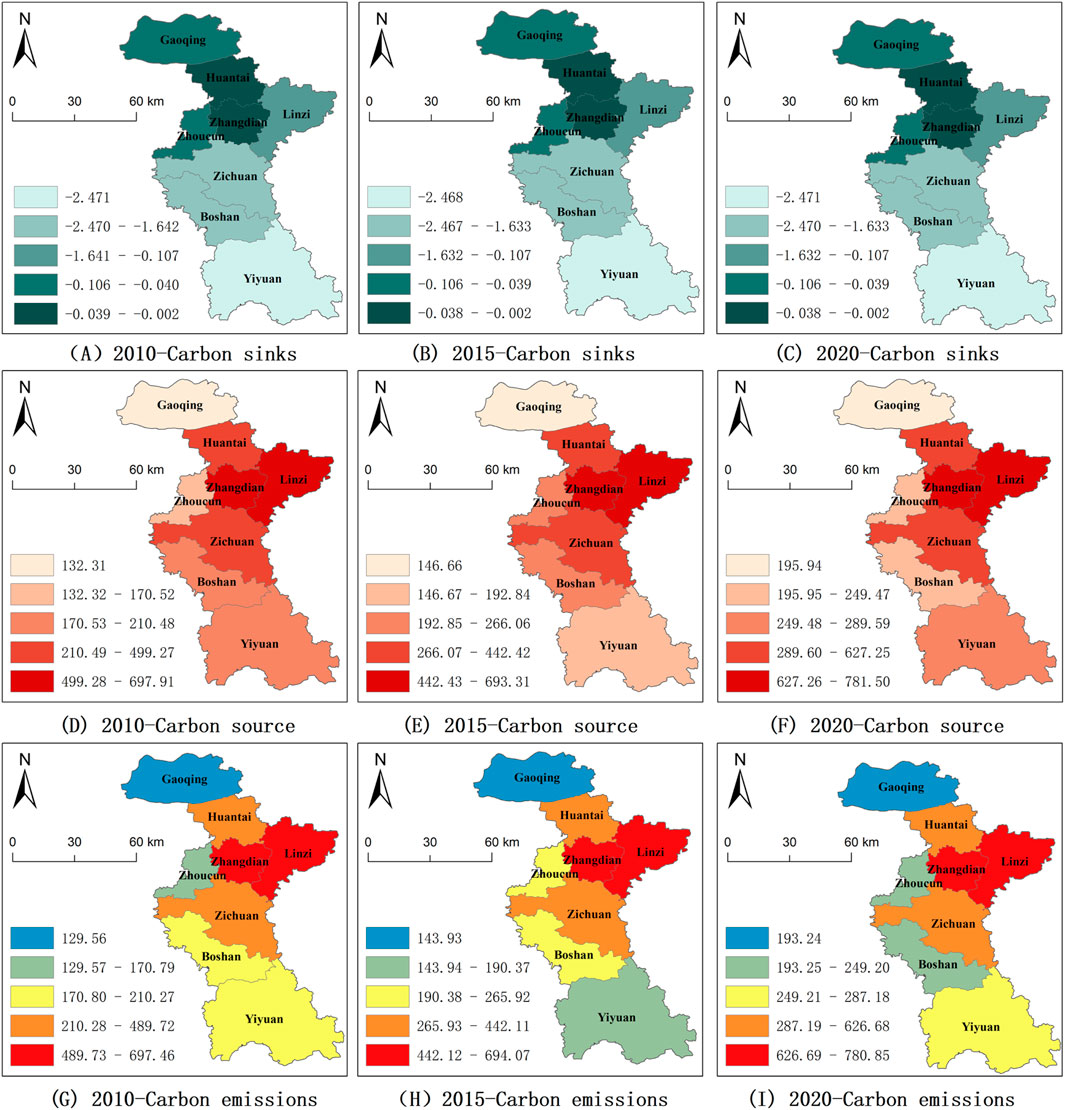
Figure 6. Spatial variation of carbon source and carbon sink of land use in Zibo City from 2010 to 2020. (Unit:104t). (A) 2010-Carbon sinks, (B) 2015-Carbon sinks, (C) 2020-Carbon sinks, (D) 2010-Carbon source, (E) 2015-Carbon source, (F) 2020-Carbon source, (G) 2010-Carbon emissions, (H) 2015-Carbon emissions, (I) 2020-Carbon emissions.
3.4 Analysis of factors affecting LUCE
3.4.1 Influence factor selection
To avoid the presence of a strong connection among the specified influencing factors, which could result in inaccurate estimation results for the model, a multiple covariance test on the selected components was performed using SPSS software. The Variance Inflation Factor (VIF) was less than 5, and the tolerance exceeded 0.1. These findings indicate that there was no significant multicollinearity among the influencing factors. Four key parameters that had a substantial impact were ultimately chosen: population size, urbanization rate, land use efficiency, and energy consumption per unit of GDP. The impact factors have the following meanings: the population size is represented by the total resident population of each district and county, while the urbanization rate is represented by the ratio of the resident population to the resident population of cities and towns. Land-use efficiency is characterized by the ratio of construction land to the total area of the land, as construction land is the main source of carbon emissions. Energy consumption per unit of GDP is represented by the ratio of total energy consumption to regional GDP, as carbon emissions from energy consumption contribute significantly to the overall increase in carbon emissions.
Furthermore, the GTWR model’s intricacy may increase the danger of overfitting when the sample size is insufficient. To solve this problem, first, the model and data were cross-validated by using Python software, the dataset was divided into a training set and test set, and the results were averaged after repeating several times, and finally, the results of the model performance evaluation were obtained, and the results showed that the model fit to the data reached 0.7320, which indicated that the model had a better explanation of the data, and avoided the appearance of overfitting. Second, based on a large number of literature readings, we found that most scholars use the GTWR model to analyze a small sample size (He and Yang, 2023; Yang et al., 2024), which to some extent indicates that GTWR does not need a large number of samples to support, and its advantage is that it simplifies the process of data collection and processing, and even without a large number of samples to support, it is still possible to analyze the characteristics of a specific group.
Simultaneous, this paper compares the regression outcomes of the spatio-temporal geographically weighted regression model (GTWR) to the ordinary least squares regression model (OLS), the time-weighted regression model (TWR), and the geographically weighted regression model (GWR) to ensure precision and relevance (Table 9). The comparison revealed that the spatio-temporal geography-weighted regression model had the highest fit R2 among the four models: ordinary least squares regression model, time-weighted regression model, geography-weighted regression model, and spatio-temporal geography-weighted regression model. Simultaneously, the spatio-temporal geographically weighted regression model, which integrates both spatio-temporal and spatial dimensions, offers more advantages in visually representing data. As a result, the spatio-temporal regionally weighted regression model was ultimately chosen to examine the spatial and temporal variations in the primary factors influencing carbon emissions.
3.4.2 Spatio-temporal geographically weighted regression analyses
The study aimed to comprehensively analyze the factors and spatial variations affecting carbon emissions from land use in Zibo County. The distribution of regression coefficients in 2010, 2015, and 2020 was visually represented using the natural breakpoint method in ArcGIS 10.6 software. This analysis was based on the findings of the spatio-temporal geographically-weighted regression model (Figure 7).
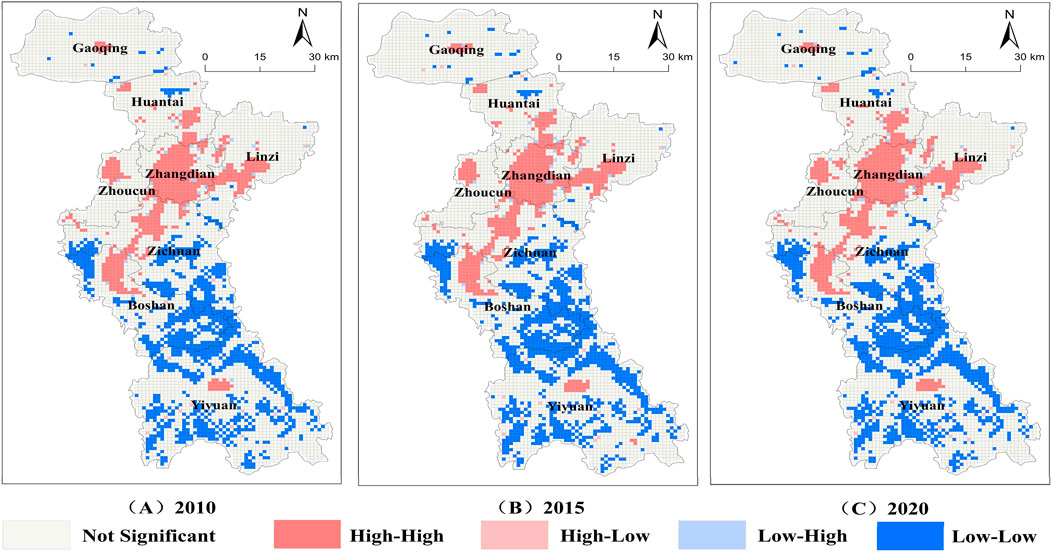
Figure 7. 1km grid scale County carbon emissions local Moran Index scatter plot of Zibo city from 2010 to 2020. (A) 2010, (B) 2015, (C) 2020.
When the regional and temporal differences in the regression coefficient of population size are taken into account, it is observed that population size has the least impact on net carbon emissions in Zibo County. However, all coefficients have a positive effect, which is increasing year after year. This is a consequence of the growing population, which leads to a rise in carbon emissions caused by human activities. Specifically, there is an increase in indirect carbon emissions, as well as emissions from living and energy sources. Additionally, the expansion of urban areas and the conversion of non-construction land into construction land contribute to a net increase in carbon emissions within the region. The spatial distribution of net carbon emissions in Zibo City follows a clear pattern of “strong in the north and weak in the south”. The areas with high carbon emissions, such as Gaoqing County, Zhoucun District, Zhangdian District, and Linzi District, are primarily characterized by arable land and construction land, high population density, and significant resource consumption. These factors contribute significantly to the overall net carbon emissions from land use. On the other hand, the areas with low carbon emissions, namely, Yiyuan County and Boshan District, are mainly mountainous regions with a small population and lower levels of economic development. Consequently, their influence on carbon emissions is relatively minor.
When the regional and temporal variations in the regression coefficient of the urbanization rate are taken into account, the impact of the urbanization rate on the county of Zibo City can be observed as initially increasing and then gradually diminishing. The cause for this is Zibo City’s fast urbanization, which increased urban population density and accelerated the growth of secondary and tertiary industries. This resulted in an improvement in economic activity and an abundance of mineral resources, oil, and natural gas. Zibo City is an important petrochemical industry base. However, in the pursuit of industrialization and urbanization, the region neglected to prioritize the protection of the ecology and the improvement of habits, and a rise in urbanization rates correlates with a rise in carbon emissions. However, in the later period, the urbanization rate of Zibo City slowed down and shifted its focus towards high-quality, environmentally friendly, and low-carbon development. As a result, the urbanization rate has a diminished impact on promoting carbon emissions. The spatial distribution exhibits a pattern of high values in the northern and southern regions and low values in the central region. The places with high values each year are primarily concentrated in Gaoqing County and Yiyuan County, among others. Gaoqing County is predominantly characterized by agricultural land, whereas Yiyuan County and Boshan District are primarily covered by forests. As a result, the existing land usage in these areas contributes to a lower level of urbanization compared to other regions. As the rate of urbanization increases, the economy and industrialization also develop. This leads to a shift in investment towards secondary and tertiary industries. In certain situations, this can encroach on carbon sinks like forest land and grassland, reducing the amount of carbon sinks in the county and increasing carbon emissions. Although the urbanization level in these areas is low, the rate of increase is fast, which ultimately promotes higher carbon emissions in areas with low urbanization rates.
When regional and temporal changes in the regression coefficient of land use efficiency are taken into account, it is discovered that land use efficiency has a positive impact on net carbon emissions in Zibo County. However, this positive impact fades over time, and in some areas of the county, it even has a negative effect. This is because the growth of construction land has resulted in a rise in carbon emissions caused by human activities. These indirect carbon emissions far outweigh the carbon emissions caused by changes in land use, resulting in an overall improvement in the efficiency of carbon emissions from land use. However, by altering and optimizing the arrangement of land usage, enhancing pollution and carbon reduction technology, and adopting new energy sources extensively, the impact of land use efficiency on carbon emissions is diminished. The spatial distribution of land use efficiency in Zibo City follows the pattern of “high in the north and low in the south”. The high-value areas are concentrated in Zhangdian District, Linzi District, and others. This distribution is primarily attributed to the dominance of arable land, construction land, and other land with carbon sources in Linzi District, Gaoqing County, Zhoucun District, and similar areas. Due to the rapid socio-economic growth, the construction land in all districts and counties of Zibo City has expanded, leading to the encroachment of forest land, grassland, and other carbon sinks. As a result, there has been an increase in indirect carbon emissions caused by land construction. It is evident that improving land use efficiency can assist in reducing carbon emissions.
When considering changes in the regression coefficient of unit GDP energy consumption, it is clear that unit GDP energy consumption has the greatest impact on Zibo City’s net carbon emissions. Overall, it contributes positively to reducing carbon emissions. However, in 2010, certain countries faced an inhibiting influence. From 2010 to 2020, Zibo City’s industrial development was rapid, consistent with its stance as a modern industrial city and a significant petrochemical base. This development is closely related to energy consumption. Zibo City’s entire industrial output value has steadily increased, leading to an overall improvement in the economic status of its districts and counties. In 2020, the industrial output value over a certain threshold in Zibo City reached 468,495 million, surpassing other industries by a significant margin. The rapid industrialization of Zibo City has caused a substantial increase in the utilization of mineral resources, oil, and natural gas energy in the area. However, low conversion efficiency has resulted in a significant amount of carbon emissions. Consequently, the net carbon emissions per unit of GDP energy consumption in Zibo City play a crucial role in driving the overall carbon emissions. The spatial distribution of energy consumption per unit of GDP in Zibo City follows a pattern of being “high in the east and low in the west”. The areas with high values are primarily located in Linzi and Zhangdian Districts, which are characterized by high economic development and substantial energy consumption. This distribution plays a significant role in influencing net carbon emissions. The areas with high values are primarily located in Linzi and Zhangdian Districts, which are characterized by high economic development and substantial energy consumption (Figure 8).
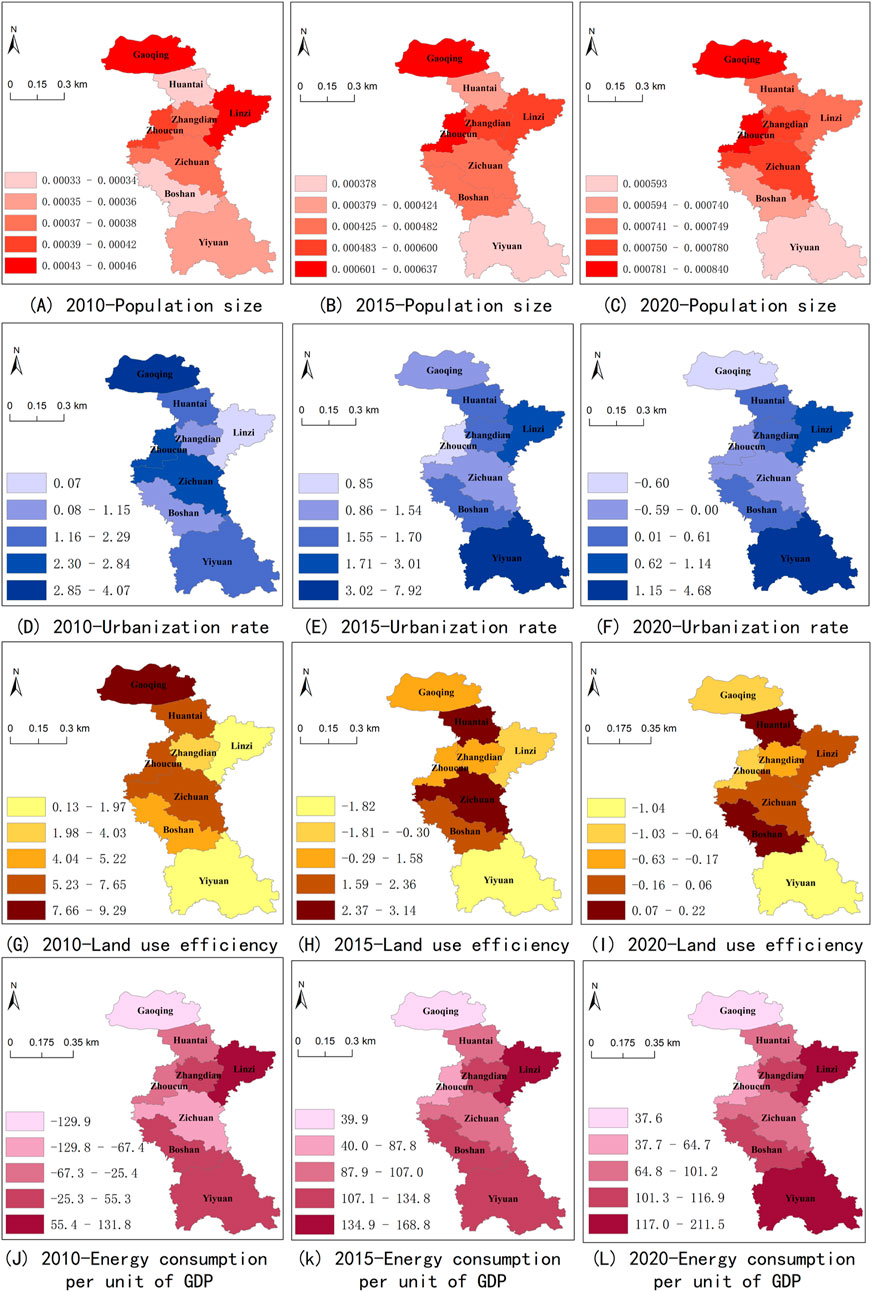
Figure 8. The change of influence factor coefficient of county carbon emissions in Zibo City from 2010 to 2020. (A) 2010-Population size, (B) 2015-Population size, (C) 2020-Population size, (D) 2010-Urbanization rate, (E) 2015-Urbanization rate, (F) 2020-Urbanization rate, (G) 2010-Land use efficiency, (H) 2015-Land use efficiency, (I) 2020-Land use efficiency, (J) 2010-Energy consumption per unit of GDP, (K) 2015-Energy consumption per unit of GDP, (L) 2020-Energy consumption per unit of GDP.
4 Discussion
4.1 Applications
With China’s economy expanding rapidly and urbanization on the rise, the amount of land required for urban development is gradually increasing. Consequently, carbon emissions from land use changes have emerged as a significant contributor to global carbon sources (Yu et al., 2022; Kang et al., 2023; Chuai et al., 2024). In light of this context, China has set a “dual-carbon” goal and is rapidly adopting efforts to meet it. Zibo City, being an established industrial city and a significant petrochemical hub, is primarily driven by petrochemicals and construction materials. It heavily relies on fossil fuels such as petroleum and coal, resulting in significant carbon dioxide emissions and other pollutants. Hence, this study focuses on Zibo City to evaluate the regional and temporal patterns of carbon emissions from land use, as well as the factors that influence them.
Regarding the regional and temporal changes in the emissions characteristics of carbon from land use, Han et al. investigated the geographical and temporal characteristics of carbon emissions in resource-based regions using the carbon emissions coefficient approach and spatial autocorrelation model (Han et al., 2021). Gui et al. (2023) studied the agglomeration of carbon emissions in counties in Guangdong Province using the same methods. Yang et al. (2023) focused on the counties in Shanxi Province and used the spatial autocorrelation model to analyze the spatial autocorrelation of the carbon ecological pressure index based on measuring the carbon emissions from construction land. To quantify carbon emissions caused by land use, the research cited above uses the carbon emissions coefficient technique. Additionally, the spatial autocorrelation model is used to analyze the spatial and temporal changes in carbon emissions. Consequently, the findings of this paper are highly scientifically valid and generalizable. Previous research analyzed carbon emissions at the county level without considering clustering tendencies within each county. Therefore, this paper aims to investigate the spatial characteristics of carbon emissions from land use in Zibo City by conducting global spatial autocorrelation analyses at the county level and local spatial autocorrelation analyses at a more detailed 1 km grid scale unit.
Within the field of research on the determinants of carbon emissions resulting from land use, Fan et al. investigated the factors that influence carbon emissions from land use in Hubei Province. They used geo-detectors and discovered that the amount of construction land, regional gross national product, urbanization rate, and resident population size all have a significant impact on regional carbon emissions (Fan et al., 2024). Tian et al. (2023) analyzed the factors influencing carbon emissions in the Beijing-Tianjin-Hebei region using a geographically weighted regression model. Their study revealed that population size, urbanization rate, and energy intensity are all major factors influencing carbon emissions in the region (Tian et al., 2023). Fang et al. (2023) used geographic probes and regionally weighted regression modeling to identify significant influencing factors and explore spatial heterogeneity. They discovered that regional gross national product had the greatest influence on carbon emissions (Fang et al., 2023). This study’s findings show that carbon emissions in Zibo City have been steadily growing over time. Zibo City’s economy is mostly driven by secondary industries. There is a noticeable spatial disparity among counties, particularly in districts and counties with a lot of construction land, high energy consumption, and large populations, all of which contribute significantly to carbon emissions. These findings are consistent with previous studies and provide credence to the scientific validity of this paper’s research results. This study focuses solely on analyzing the factors that influence carbon emissions from land use from a spatial standpoint. It does not take into account the combined spatial and temporal perspective. Therefore, this paper utilizes a spatio-temporal geographically weighted regression model to examine the influencing factors from both temporal and spatial angles. Additionally, it analyzes the spatial heterogeneity of the counties in Zibo City.
Zibo City recognized as a regional hub by the State Council, is a contemporary industrial city and a significant petrochemical center. It has large deposits of oil, natural gas, and other resources. Conducting a study on carbon emissions from land use in Zibo City holds immense practical importance for the region’s industrial transformation and the advancement of high-tech enterprises.
4.1.1 Optimisation of land use structure
The conclusions of this research’s land use change study provide suggestions for optimizing and modifying Zibo City’s land use composition. The analysis of land use change reveals that satisfying regional carbon emissions demand through carbon sink absorption in Zibo City is challenging. Rapid development and urbanization in Zibo City have resulted in an expansion of construction land and a corresponding increase in carbon emissions. The average annual growth rate of carbon emissions has reached 900,000 tonnes, far exceeding the capacity of carbon sink absorption during the same period. In the future, Zibo City should prioritize adjusting the blue-green space pattern, specifically by transforming farms back into forests and grasslands, restoring ecological balance, and comprehensively improving the national territory. This would ultimately lead to an increase in urban vegetation coverage.
4.1.2 Transformation and upgrading of highly polluting industries
Analyze the carbon emissions patterns caused by land use in Zibo City to provide recommendations for reducing energy consumption and pollution in the city’s high-polluting industries. According to research, land use carbon emissions in Zibo City have different agglomeration characteristics. The principal source of these emissions is construction land, with human social activities on the surface, particularly energy consumption, accounting for the majority of carbon emissions from construction land. The aforementioned analysis revealed that energy-related carbon emissions constitute about 95% of the total carbon emissions in Zibo City. Hence, the primary emphasis for industrial development in Zibo City should be on upgrading low-carbon technologies, improving energy use, and enhancing the industry’s green intelligence level, among other factors. It is crucial to update and transform Zibo City’s traditional industries, which emit significant levels of pollution and consume a lot of energy.
4.1.3 Differentiated low-carbon development policy formulation
This study uses a spatio-temporal regionally weighted regression model to examine the factors that influence Zibo City’s districts and counties. The results of this analysis provide a solid platform for developing carbon reduction policies suited to the specific districts and counties in Zibo City. There is clear variation in space within the region of Zibo City, and certain causes have distinct impacts in different districts and counties. Hence, implementing specialized low-carbon development strategies for various regions is crucial for Zibo City’s low-carbon development goal.
4.2 Limitations and prospects
This study focuses on Zibo City and looks at the spatial and temporal trends of carbon emissions from land use. It also examines the clustering characteristics and factors that influence land use changes. The goal is to establish a scientific foundation for designing specific carbon emissions reduction strategies in Zibo City. Nevertheless, the following limitations persist: (1) In terms of carbon emissions agglomeration characteristics, this paper is based on the spatial autocorrelation model for the agglomeration characteristics analysis of the county unit and 1 km grid unit in Zibo City, and there is a lack of focus on the research of carbon emissions agglomeration characteristics on the scale of townships and the scale of refinement; (2) In terms of carbon emissions accounting, this paper adopts the traditional carbon emissions coefficient method, which is widely used and easy to calculate, but the accuracy of the carbon emissions coefficients of different land use types still needs to be considered, and the carbon emissions coefficients need to be studied accurately by geographical areas, taking into account the impact of factors such as different regions, different altitudes, different ecological environments, the degree of vegetation cover and socio-economic conditions on the carbon emissions coefficients, and improving the accuracy of carbon emissions accounting; (3) In the selection of influencing factors, land use carbon emissions of many factors, this paper in reference to existing research, the selection of factors is relatively limited, only the main factors of the degree of influence of the analysis, the future can be constructed through a more complete system of indicators of influencing factors to determine the degree of influence of various types of factors on the land use of carbon emissions. Additionally, this article analyzes Zibo City as an isolated entity, ignoring the impact of natural and social environmental elements in neighboring cities on carbon emissions. Therefore, future research should take into account the interplay between different cities and include neighboring regions in the study area.
Therefore, in future research, more attention should be paid to the development of the following contents: (1) Focusing on more detailed carbon emission accounting methods: scholars generally adopt the carbon emission coefficient method in carbon emission accounting at this stage, but the carbon emission coefficients apply to different regions to different degrees. In the future, scholars should revise the carbon emission coefficients applicable to different regions in terms of their socio-economic environment, natural conditions, etc.; (2) Focus on the spatial expression of carbon emissions at the micro-scale: in terms of spatial expression of carbon emissions, scholars have mainly adopted methods such as the GIS spatial analysis and the “point-line-plane” model. Whether there is a more accurate spatial expression of carbon emissions in the future is still a key point in the field of carbon emission research.
4.3 Recommendations
Despite its limitations, this article effectively depicts the spatial and temporal distribution patterns of carbon emissions in Zibo County and identifies the key drivers. Based on these findings, the paper proposes the following recommendations:
In future urban development, Zibo should be guided by the strategic goal of “reducing carbon intensity and increasing carbon sink capacity”.
(1) Diminish the carbon emissions
Zibo City, as a resource-based city and a historic industrial base in China, has a strong industrial base and infrastructure. However, the city’s industry has suffered from the “three high” phenomena of high pollution, high energy consumption, and high carbon emissions as a result of years of “rough” development marked by outmoded technology, low manufacturing standards, and excessive resource consumption. To overcome these challenges posed by industrial transformation and increasing environmental pressure, Zibo City should focus on transforming production and lifestyle. It should prioritize the development of ecological and environmental protection industries as well as high-tech industries. Additionally, efforts should be made to enhance green, low-carbon technology and improve energy efficiency. Take advantage of the opportunity for transformation and development in the “double carbon” era by bringing new energy to the city. Exploit established businesses’ potential while encouraging the development of new materials, intelligent equipment, new medicine, and electronic information sectors. Push the industry towards high-end, intelligent, and environmentally friendly practices.
(2) Enhance carbon sequestration
Due to Zibo City’s rapid growth, a rise in construction land has resulted in the loss of forest land, grassland, and other carbon sinks. Forestland and grassland are crucial for absorbing carbon dioxide, and their loss lowers the city’s capacity to absorb carbon. Consequently, the city’s carbon emissions have increased. Zibo City must ensure a set amount of land dedicated to carbon absorption, enhance the capacity of urban areas to absorb carbon, improve the arrangement of ecological spaces and land use structure in urban areas, and fully utilize underutilized land. Enhance the efforts to restore the urban ecological system and promote the reforestation and regrowth of grasslands. Green plants play a crucial role in absorbing carbon dioxide in urban settings, making them valuable carbon sinks. To enhance the amount of green space in cities, one can optimize the organization of public green areas and adopt three-dimensional greening techniques, among other ways for urban greening.
5 Conclusion
This paper uses Zibo City as the research object, investigates the land use changes in Zibo City from 2010 to 2020 based on the attitude of land use dynamics, analyzes the spatial and temporal evolution characteristics of Zibo county by measuring its net carbon emissions, and then employs exploratory spatial analysis methods to analyze the spatial correlation of Zibo county. Finally, a spatio-temporal regionally weighted regression model was used to analyze the various factors influencing net carbon emissions in the county.
(1) From 2010 to 2020, the rate of change in land use in Zibo City decreased. The overall quality of land use in Zibo City declined from 0.14% in the period of 2010–2015 to 0.09% in the period of 2015–2020, indicating major shifts in land use structure. Within the designated category, the area of arable land, forest land, and grassland decreased. Conversely, there was a rise in the area of water, construction land, and unused land. The largest increase was observed in the area of transferred-in construction land, which expanded by 65.168 km2. On the other hand, the largest decrease was observed in the area of transferred-out arable land, which contracted by 61.449 km2. Additionally, land was converted from arable to construction land.
(2) From 2010 to 2020, Zibo City’s carbon source rapidly expanded from 3.017 × 107t to 3.918 × 107t, with an average annual growth rate of 9 × 105t. In contrast, the carbon sink in Zibo City grew more slowly, from 63,636.9t to 64,381.8t, with an average annual growth rate of 74.49t. The study reveals that the land in Zibo City has a limited capacity to absorb carbon and is unable to meet the demands of carbon emissions. Net carbon emissions have quickly increased, from 3.011 × 107t to 3.911 × 107t. In terms of spatial distribution, the southern portion of Zibo contains the majority of carbon sinks, which are mostly forest land. Conversely, the central part of the economically developed area serves as the main source of carbon emissions. The spatial distribution pattern of net carbon emissions in Zibo City has consistently followed a trend of being “high in the east and low in the west, high in the south and low in the north”. High-value areas are concentrated in Linzi District, Zhangdian District, and other locations.
(3) The spatial autocorrelation study, which was carried out using ArcGIS10.6 software, demonstrated that the net carbon emissions of the counties in Zibo City clustered positively. This means that areas with high (low) values were located next to other areas with high (low) values. Overall, the clustering effect was weakening, as indicated by the decline in Moran’s I from 0.219 to 0.212. This suggests that the clustering effect of the district and county units was diminishing. The local spatial autocorrelation analysis revealed that the agglomeration effect of areas with high-net carbon emissions and areas with low agglomeration within Zibo City has been strengthened. The high-high agglomeration area is primarily concentrated in Zhangdian District, Linzi District, and other central areas of Zibo, exhibiting a gradual expansion pattern. Conversely, the low-low agglomeration area is mainly found in the southern part of Zibo, such as Boshan District and Yiyuan County, which also display a slow expansion trend. This indicates an increasing concentration of carbon emissions within the 1 km scale unit.
(4) The spatiotemporal spatially weighted regression model (GTWR) study revealed that the same influencing factors differed significantly amongst Zibo districts and counties due to variances in socioeconomic and natural surroundings. Population size has the smallest impact on Zibo counties’ net carbon emissions, whereas energy consumption per unit of GDP has the greatest impact. The regression coefficients for population size are all positive, indicating a growing trend; however, the urbanization rate, land use efficiency, and unit GDP energy consumption are all negative values, and overall performance is initially improved and later weakened.
This study presents a detailed examination of the regional and temporal patterns of land use carbon emissions in Zibo City, with a focus on the influence of land use change. The findings of this study can be used to inform future research on land use carbon emissions at various scales. Furthermore, the results can inform decisions regarding land use planning, energy adjustments, and the development of a low-carbon strategy in Zibo City, ultimately contributing to the city’s low-carbon construction initiatives.
Data availability statement
The raw data supporting the conclusions of this article will be made available by the authors, without undue reservation.
Author contributions
LL: Conceptualization, Methodology, Validation, Writing–original draft. XZ: Conceptualization, Data curation, Formal Analysis, Investigation, Methodology, Project administration, Software, Supervision, Validation, Writing–review and editing. LY: Investigation, Methodology, Software, Writing–review and editing. HX: Data curation, Project administration, Resources, Validation, Writing–original draft.
Funding
The author(s) declare that financial support was received for the research, authorship, and/or publication of this article. This research was funded by the Humanities and Social Sciences Research Youth Fund Project of the Ministry of Education, grant no. 23YJCZH306, the Youth fund of Shandong Natural Science Foundation, grant no. ZR2022QE151, and the Jinan Philosophy Social Science Project, grant no. JNSK23C54.
Acknowledgments
The authors would like to acknowledge all colleagues and friends who have voluntarily reviewed the translation of the survey and the manuscript of this study.
Conflict of interest
The authors declare that the research was conducted in the absence of any commercial or financial relationships that could be construed as a potential conflict of interest.
Publisher’s note
All claims expressed in this article are solely those of the authors and do not necessarily represent those of their affiliated organizations, or those of the publisher, the editors and the reviewers. Any product that may be evaluated in this article, or claim that may be made by its manufacturer, is not guaranteed or endorsed by the publisher.
References
Cai, Y. X., and Li, K. Q. (2024). Spatiotemporal dynamic evolution and influencing factors of land use carbon emissions: evidence from Jiangsu Province, China. Front. Environ. Sci. 12. doi:10.3389/fenvs.2024.1368205
Cao, W., and Yuan, X. (2019). Region-county characteristic of spatial-temporal evolution and influencing factor on land use-related CO2 emissions in Chongqing of China, 1997-2015. J. Clean. Prod. 231, 619–632. doi:10.1016/j.jclepro.2019.05.248
Chen, H., Meng, C. Y., and Cao, Q. L. (2022). Measurement and influencing factors of low carbon urban land use efficiency-based on non-radial directional distance function. Land 11 (7), 1052. doi:10.3390/land11071052
Chen, L. H., Hang, Y. H., and Li, Q. F. (2023). Spatial-temporal characteristics and influencing factors of carbon emissions from land use and land cover in Black Soil region of northeast China based on LMDI simulation. Sustainability 15 (12), 9334. doi:10.3390/su15129334
Cheng, Y. X., Tang, Y. Q., Zhou, B., and Feng, H. H. (2024). Spatiotemporal analysis of national carbon emission and regional carbon simulation in China. Environ. Sci. Pollut. Res. 31 (7), 10702–10716. doi:10.1007/s11356-023-31817-6
Chuai, X., Xu, H. B., Liu, Z. M., Xiang, A., Luo, Y. T., Mao, W. L., et al. (2024). Promoting low-carbon land use: from theory to practical application through exploring new methods. Humanit. and Soc. Sci. Commun. 11 (1), 727. doi:10.1057/s41599-024-03192-1
Chuai, X. W., Huang, X. J., Wang, W. J., Zhao, R. Q., Zhang, M., and Wu, C. Y. (2015). Land use, total carbon emission's change and low carbon land management in Coastal Jiangsu, China. J. Clean. Prod. 103, 77–86. doi:10.1016/j.jclepro.2014.03.046
Cui, Y. F., Li, L., Chen, L. Q., Zhang, Y., Cheng, L., Zhou, X. S., et al. (2018). Land-use carbon emissions estimation for the Yangtze River Delta urban agglomeration using 1994-2016 Landsat image data. Remote Sens. 10 (9), 1334. doi:10.3390/rs10091334
Dong, J., and Yun, K. Q.Ieee (2015). Land use carbon emissions efficiency analysis in Hubei province, 1, 168, 172. doi:10.1109/agro-geoinformatics.2015.7248110
Fan, M. J., Wang, Z. Q., and Xue, Z. B. (2024). Spatiotemporal evolution characteristics, influencing factors of land use carbon emissions, and low-carbon development in Hubei Province, China. Ecol. Inf. 81, 102567. doi:10.1016/j.ecoinf.2024.102567
Fang, W., Luo, P. P., Luo, L. T., Zha, X. B., and Nover, D. (2023). Spatiotemporal characteristics and influencing factors of carbon emissions from land-use change in Shaanxi Province, China. Environ. Sci. Pollut. Res. 30 (59), 123480–123496. doi:10.1007/s11356-023-30606-5
Gui, D. W., He, H. G., Liu, C. M., and Han, S. S. (2023). Spatio-temporal dynamic evolution of carbon emissions from land use change in Guangdong Province, China, 2000-2020. Ecol. Indic., 156. doi:10.1016/j.ecolind.2023.111131
Guo, R., Wu, X. C., Wu, T., and Dai, C. (2023). Spatial-temporal pattern characteristics and impact factors of carbon emissions in production-living-ecological spaces in Heilongjiang province, China. Land 12 (6), 1153. doi:10.3390/land12061153
Han, X. J., Yu, J. L., Xia, Y., and Wang, J. J. (2021). Spatiotemporal characteristics of carbon emissions in energy-enriched areas and the evolution of regional types. Energy Rep. 7, 7224–7237. doi:10.1016/j.egyr.2021.10.097
Han, Y., and Ge, X. S. (2023). Spatial-temporal characteristics and influencing factors on carbon emissions from land use in suzhou, the world's largest industrial city in China. Sustainability 15 (18), 13306. doi:10.3390/su151813306
He, J., and Yang, J. (2023). Spatial-temporal characteristics and influencing factors of land-use carbon emissions: an empirical analysis based on the GTWR model. Land 12 (8), 1506. doi:10.3390/land12081506
Huang, H. Q., and Zhou, J. (2022). Study on the spatial and temporal differentiation pattern of carbon emission and carbon compensation in China's provincial areas. Sustainability 14 (13), 7627. doi:10.3390/su14137627
Huang, H. Z., Jia, J. S., Chen, D. L., and Liu, S. T. (2024). Evolution of spatial network structure for land-use carbon emissions and carbon balance zoning in Jiangxi Province: a social network analysis perspective. Ecol. Indic. 158, 111508. doi:10.1016/j.ecolind.2023.111508
Huang, S. Q., Xi, F. R., Chen, Y. M., Gao, M., Pan, X., and Ren, C. (2021). Land use optimization and simulation of low-carbon-oriented-A case study of jinhua, China. Land 10 (10), 1020. doi:10.3390/land10101020
Jia, J. S., Xin, L. L., Lu, C. F., Wu, B., and Zhong, Y. X. (2023). China's CO2 emissions: a systematical decomposition concurrently from multi-sectors and multi-stages since 1980 by an extended logarithmic mean divisia index. Energy Strategy Rev. 49, 101141. doi:10.1016/j.esr.2023.101141
Kang, T. T., Wang, H., He, Z. Y., Liu, Z. Y., Ren, Y., and Zhao, P. J. (2023). The effects of urban land use on energy-related CO2 emissions in China. Sci. Total Environ., 870. doi:10.1016/j.scitotenv.2023.161873
Li, H. P., and Lin, T. (2022). Do land use structure changes impact regional carbon emissions? A spatial econometric study in sichuan basin, China. Int. J. Environ. Res. Public Health 19 (20), 13329. doi:10.3390/ijerph192013329
Li, L., Chen, Z. C., and Wang, S. D. (2022). Optimization of spatial land use patterns with low carbon target: a case study of sanmenxia, China. Int. J. Environ. Res. Public Health 19 (21), 14178. doi:10.3390/ijerph192114178
Li, L., Li, J. F., Peng, L., Wang, X. L., and Sun, S. J. (2023). Spatiotemporal evolution and influencing factors of land-use emissions in the Guangdong-Hong Kong-Macao Greater Bay Area using integrated nighttime light datasets. Sci. Total Environ. 893, 164723. doi:10.1016/j.scitotenv.2023.164723
Li, X., Liu, Z. S., Li, S. J., Li, Y. X., and Wang, W. Y. (2023). Urban land carbon emission and carbon emission intensity prediction based on patch-generating land use simulation model and grid with multiple scenarios in tianjin. Land 12 (12), 2160. doi:10.3390/land12122160
Li, X. P., Hu, S., Jiang, L. F., Han, B., Li, J., and Wei, X. (2023). Spatiotemporal patterns and the development path of land-use carbon emissions from a low-carbon perspective: a case study of guizhou province. Land 12 (10), 1875. doi:10.3390/land12101875
Lin, Q. W., Zhang, L., Qiu, B. K., Zhao, Y., and Wei, C. (2021). Spatiotemporal analysis of land use patterns on carbon emissions in China. Land 10 (2), 141. doi:10.3390/land10020141
Liu, C. X., Xu, R., Xu, K. J., Lin, Y. W., and Cao, Y. G. (2023). Carbon emission effects of land use in chaobai river region of beijing-tianjin-hebei, China. Land 12 (6), 1168. doi:10.3390/land12061168
Liu, S. T., Jia, J. S., Huang, H. Z., Chen, D. L., Zhong, Y. X., and Zhou, Y. M. (2023). China’s CO2 emissions: a thorough analysis of spatiotemporal characteristics and sustainable policy from the agricultural land-use perspective during 1995-2020. Land 12 (6), 1220. doi:10.3390/land12061220
Meng, Q. X., Zheng, Y. N., Liu, Q., Li, B. L., and Wei, H. J. (2023). Analysis of spatiotemporal variation and influencing factors of land-use carbon emissions in nine provinces of the Yellow River basin based on the LMDI model. Land 12 (2), 437. doi:10.3390/land12020437
Rong, T. Q., Zhang, P. Y., Li, G. H., Wang, Q. X., Zheng, H. T., Chang, Y. H., et al. (2023). Spatial correlation evolution and prediction scenario of land use carbon emissions in the Yellow River Basin. Ecol. Indic. 154, 110701. doi:10.1016/j.ecolind.2023.110701
Shi, F. C., Liao, X., Shen, L. Y., Meng, C. H., and Lai, Y. Y. (2022). Exploring the spatiotemporal impacts of urban form on CO2 emissions: evidence and implications from 256 Chinese cities. Environ. Impact Assess. Rev. 96, 106850. doi:10.1016/j.eiar.2022.106850
Song, C. R., Yang, J., Wu, F., Xiao, X. M., Cecilia, J. X., and Li, X. M. (2022). Response characteristics and influencing factors of carbon emissions and land surface temperature in Guangdong Province, China. Urban Clim. 46, 101330. doi:10.1016/j.uclim.2022.101330
Tan, Y. C., Liu, Y. Z., Chen, Y., Zhang, Z., Wu, D., Chen, H. Y., et al. (2023). The impact of urban construction land change on carbon emissions-A case study of wuhan city. Int. J. Environ. Res. Public Health 20 (2), 922. doi:10.3390/ijerph20020922
Tang, W. W., Cui, L. H., Zheng, S., and Hu, W. (2022). Multi-Scenario simulation of land use carbon emissions from energy consumption in shenzhen, China. Land 11 (10), 1673. doi:10.3390/land11101673
Tian, C., Cheng, L. L., and Yin, T. T. (2023). Spatial heterogeneity of driving forces in response to carbon emissions from land use at county-level in beijing-tianjin-hebei region. Pol. J. Environ. Stud. 32 (1), 267–279. doi:10.15244/pjoes/153982
Tian, M. J., Chen, Z., Wang, W., Chen, T. Z., and Cui, H. Y. (2022). Land-use carbon emissions in the Yellow River basin from 2000 to 2020: spatio-temporal patterns and driving mechanisms. Int. J. Environ. Res. Public Health 19 (24), 16507. doi:10.3390/ijerph192416507
Wang, H., Jin, Y. J., Hong, X. M., Tian, F., Wu, J. X., and Nie, X. (2022). Integrating IPAT and CLUMondo models to assess the impact of carbon peak on land use. Land 11 (4), 573. doi:10.3390/land11040573
Wang, M. M., Kong, D. Z., Mao, J. H., Ma, W. J., and Ayyamperumal, R. (2023). The impacts of land use spatial form changes on carbon emissions in qinghai-tibet plateau from 2000 to 2020: a case study of the lhasa metropolitan area. Land 12 (1), 122. doi:10.3390/land12010122
Wei, B. H., Kasimu, A., Reheman, R., Zhang, X. L., Zhao, Y. Y., Aizizi, Y., et al. (2023). Spatiotemporal characteristics and prediction of carbon emissions/absorption from land use change in the urban agglomeration on the northern slope of the Tianshan Mountains. Ecol. Indic. 151, 110329. doi:10.1016/j.ecolind.2023.110329
Wu, H., Fang, S. M., Zhang, C., Hu, S. W., Nan, D., and Yang, Y. Y. (2022). Exploring the impact of urban form on urban land use efficiency under low-carbon emission constraints: a case study in China's Yellow River Basin. J. Environ. Manag. 311, 114866. doi:10.1016/j.jenvman.2022.114866
Wu, Y. G., Pu, W. P., Dong, J. H., Dai, W. T., and Wang, Y. X. (2024). Analysis of land use gravity center change and carbon emission impact in chengdu plain of China from 2006 to 2022. Land 13 (6), 873. doi:10.3390/land13060873
Xia, C. Y., Zhang, J., Zhao, J., Xue, F., Li, Q., Fang, K., et al. (2023). Exploring potential of urban land-use management on carbon emissions-- A case of Hangzhou, China. Ecol. Indic. 146, 109902. doi:10.1016/j.ecolind.2023.109902
Yan, H. M., Guo, X., Zhao, S. Q., and Yang, H. C. (2022). Variation of net carbon emissions from land use change in the beijing-tianjin-hebei region during 1990-2020. Land 11 (7), 997. doi:10.3390/land11070997
Yang, B., Chen, X., Wang, Z. Q., Li, W. D., Zhang, C. R., and Yao, X. W. (2020). Analyzing land use structure efficiency with carbon emissions: a case study in the Middle Reaches of the Yangtze River, China. J. Clean. Prod. 274, 123076. doi:10.1016/j.jclepro.2020.123076
Yang, J. Y., Li, K. N., Liu, Y. Q., and Zhang, Y. F. (2024). Time-space evolution and drivers of CO2 emissions from land utilization in xinjiang from 2000 to 2020. Sustainability 16 (7), 2929. doi:10.3390/su16072929
Yang, X., and Liu, X. Z. (2023). Path analysis and mediating effects of influencing factors of land use carbon emissions in Chang-Zhu-Tan urban agglomeration. Technol. Forecast. Soc. Change, 188. doi:10.1016/j.techfore.2022.122268
Yang, X. J., Bai, B., and Bai, Z. K. (2023). Research on spatiotemporal changes in carbon footprint and vegetation carbon carrying capacity in Shanxi province. Forests 14 (7), 1295. doi:10.3390/f14071295
Yu, Y., Du, C., Xu, X. L., Hu, C. C., Zeng, J. Y., Li, E. L., et al. (2013). Research on land use carbon emissions empirical in Guangdong province. AMR, 734–737.
Yu, Z. Q., Chen, L. Q., Tong, H. X., Chen, L. G., Zhang, T., Li, L., et al. (2022). Spatial correlations of land-use carbon emissions in the Yangtze River Delta region: a perspective from social network analysis. Ecol. Indic. 142, 109147. doi:10.1016/j.ecolind.2022.109147
Zhang, C. Y., Zhao, L., Zhang, H. T., Chen, M. N., Fang, R. Y., Yao, Y., et al. (2022). Spatial-temporal characteristics of carbon emissions from land use change in Yellow River Delta region, China. Ecol. Indic. 136, 108623. doi:10.1016/j.ecolind.2022.108623
Zhang, D., Wang, Z. Q., Li, S. C., and Zhang, H. W. (2021). Impact of land urbanization on carbon emissions in urban agglomerations of the middle reaches of the Yangtze River. Int. J. Environ. Res. Public Health 18 (4), 1403. doi:10.3390/ijerph18041403
Zhang, W. J., and Xu, H. Z. (2017). Effects of land urbanization and land finance on carbon emissions: a panel data analysis for Chinese provinces. Land Use Policy 63, 493–500. doi:10.1016/j.landusepol.2017.02.006
Zhang, X., and Zhang, D. (2023). Urban carbon emission scenario prediction and multi-objective land use optimization strategy under carbon emission constraints. J. Clean. Prod. 430, 139684. doi:10.1016/j.jclepro.2023.139684
Zhang, Y., Wu, Q., Zhao, X. Y., Hao, Y., Liu, R. M., Yang, Z. F., et al. (2018). Study of carbon metabolic processes and their spatial distribution in the Beijing-Tianjin-Hebei urban agglomeration. Sci. Total Environ. 645, 1630–1642. doi:10.1016/j.scitotenv.2018.07.033
Zhang, Y. H., Li, J. F., Liu, S. Q., and Zhou, J. Z. (2024). Spatiotemporal effects and optimization strategies of land-use carbon emissions at the county scale: a case study of shaanxi province, China. Sustainability 16 (10), 4104. doi:10.3390/su16104104
Zhao, L., Yang, C. H., Zhao, Y. C., Wang, Q., and Zhang, Q. P. (2023). Spatial correlations of land use carbon emissions in Shandong Peninsula urban agglomeration: a perspective from city level using remote sensing data. Remote Sens. 15 (6), 1488. doi:10.3390/rs15061488
Zhao, Y. B., Ma, S. F., Fan, J. H., and Cai, Y. N. (2021). Examining the effects of land use on carbon emissions: evidence from Pearl River Delta. Int. J. Environ. Res. Public Health 18 (7), 3623. doi:10.3390/ijerph18073623
Zhou, X., and Cao, F. Y. (2022). Analysis of economic efficiency and influencing factors of urban construction land in beijing-tianjin-hebei under carbon emission constraints. Land 11 (11), 1926. doi:10.3390/land11111926
Keywords: land use, carbon emissions, spatio-temporal characteristics, influencing factor, Zibo
Citation: Li L, Zhang X, Yu L and Xiao H (2024) Spatial-temporal evolution of land use carbon emissions and influencing factors in Zibo, China. Front. Environ. Sci. 12:1472558. doi: 10.3389/fenvs.2024.1472558
Received: 31 July 2024; Accepted: 22 October 2024;
Published: 05 November 2024.
Edited by:
Merja H. Tölle, University of Kassel, GermanyCopyright © 2024 Li, Zhang, Yu and Xiao. This is an open-access article distributed under the terms of the Creative Commons Attribution License (CC BY). The use, distribution or reproduction in other forums is permitted, provided the original author(s) and the copyright owner(s) are credited and that the original publication in this journal is cited, in accordance with accepted academic practice. No use, distribution or reproduction is permitted which does not comply with these terms.
*Correspondence: Xiaoping Zhang, cmx5ODkyMUAxNjMuY29t