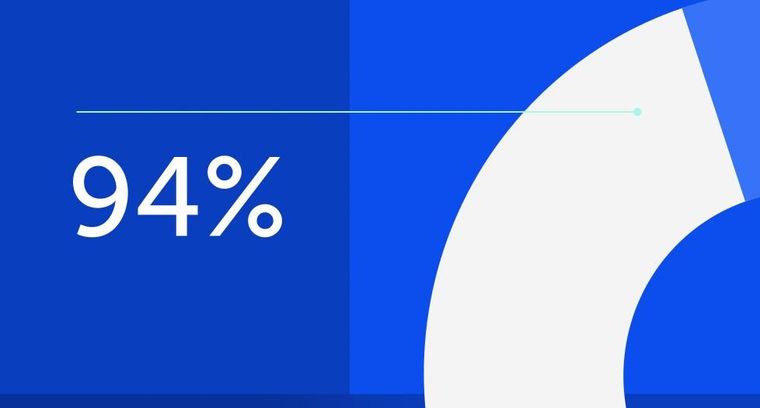
94% of researchers rate our articles as excellent or good
Learn more about the work of our research integrity team to safeguard the quality of each article we publish.
Find out more
METHODS article
Front. Environ. Sci., 06 February 2023
Sec. Interdisciplinary Climate Studies
Volume 11 - 2023 | https://doi.org/10.3389/fenvs.2023.1069613
This article is part of the Research TopicFood-Energy-Water Systems: Achieving Climate Resilience and Sustainable Development in the 21st CenturyView all 21 articles
Climate change continues to challenge food, energy, and water systems (FEWS) across the globe and will figure prominently in shaping future decisions on how best to manage this nexus. In turn, traditionally engineered and natural infrastructures jointly support and hence determine FEWS performance, their vulnerabilities, and their resilience in light of extreme climate events. We present here a research framework to advance the modeling, data integration, and assessment capabilities that support hypothesis-driven research on FEWS dynamics cast at the macro-regional scale. The framework was developed to support studies on climate-induced extremes on food, energy, and water systems (C-FEWS) and designed to identify and evaluate response options to extreme climate events in the context of managing traditionally engineered (TEI) and nature-based infrastructures (NBI). This paper presents our strategy for a first stage of research using the framework to analyze contemporary FEWS and their sensitivity to climate drivers shaped by historical conditions (1980–2019). We offer a description of the computational framework, working definitions of the climate extremes analyzed, and example configurations of numerical experiments aimed at evaluating the importance of individual and combined driving variables. Single and multiple factor experiments involving the historical time series enable two categories of outputs to be analyzed: the first involving biogeophysical entities (e.g., crop production, carbon sequestered, nutrient and thermal pollution loads) and the second reflecting a portfolio of services provided by the region’s TEI and NBI, evaluated in economic terms. The framework is exercised in a series of companion papers in this special issue that focus on the Northeast and Midwest regions of the United States. Use of the C-FEWS framework to simulate historical conditions facilitates research to better identify existing FEWS linkages and how they function. The framework also enables a next stage of analysis to be pursued using future scenario pathways that will vary land use, technology deployments, regulatory objectives, and climate trends and extremes. It also supports a stakeholder engagement effort to co-design scenarios of interest beyond the research domain.
Sufficient and secure supplies of food, energy, and water are fundamental to human wellbeing and a sustainable society across the globe (UN General Assembly, 2015). In the United States, the agriculture and energy sectors together account for 78% of all freshwater withdrawals and 65% of all consumption, making these sectors collectively the largest user of water in the nation (Dieter et al., 2018). At the same time, growing evidence suggests that human-induced climate change is increasing the frequency and severity of extreme weather events such as heatwaves, droughts, intense precipitation, and heavy flooding (IPCC, 2021), precisely those climate stressors shown to compromise these important provisioning resource systems (Brown et al., 2015; USGCRP, 2018; Weiskopf et al., 2020). Understanding how climate-related shocks move through the FEWS will greatly impact the management of supporting infrastructures—traditionally engineered (e.g., dams, irrigation, water treatment plants) (McKinsey & Company, 2006; ASCE, 2016), nature-based (e.g., landscapes, aquatic systems) (EPA, 2015; Green et al., 2015; European Commission, 2016), and their combination (Young, 2000; McDonald et al., 2016; Vörösmarty et al., 2021).
We report here on a framework to study Climate-induced Extremes on Food, Energy, Water Systems (C-FEWS), a system designed to identify and evaluate policy response options to extreme climate events that engage traditionally engineered (TEI) and nature-based infrastructures (NBI). We describe technical elements of the framework and how it can be used to explore FEWS behaviors in the context of historical (1980–2019) system dynamics, where we consider the individual and conjunctive roles of climate, land management, technology and regulation.
We begin with a presentation of the key facets of FEWS dynamics that were considered as design criteria for the framework. We then present a methods section, detailing the overall framework and describing the component models and key data sets, starting with the nature of the climate extremes and the set of quantitative metrics used to identify them. We continue with summary descriptions of the models, their input data requirements, and key output variables, followed by our approach to single and multi-factor scenario experiments. We also provide a comment on framework-supported stakeholder engagement. A section on potential applications follows and demonstrates how outputs from the C-FEWS framework can be used identify which elements of the FEWS could be most/least resilient over the coming decades. More detailed descriptions of the models and data sets and the interpretation of results are given in an accompanying series of papers in this Frontiers Special Topic (Bokhari et al., 2022; Chang et al., 2022; Fekete et al., 2022; Kicklighter et al., 2022; Lin et al., 2022; Maxfield et al., 2022; Tuler et al., 2022; Zhang et al., 2022). An early synthesis of five emblematic studies using the framework is given in Vörösmarty et al. (this issue).
An important motivation for this study is a key finding of the 4th National Climate Assessment (NCA), namely, that climate change and its extremes are increasing (USCGRP, 2017) and simultaneously reducing the capacity of the environment to withstand additional stresses. This, in turn, produces collateral losses of ecosystem goods and services that otherwise yield valuable benefits to society (USGCRP, 2018).
The capacity to anticipate the impacts of climate change and its variability on the nation’s FEWS is a national and global imperative (Newmark et al., 2012; Miara et al., 2013; Warner et al., 2013; Arent et al., 2014; Wuebbles et al., 2014; Challinor et al., 2015; Kotamarthi et al., 2016; Martinich and Crimmins, 2019). For example, shifting patterns of drought and other severe weather in the U.S. are anticipated to lower crop yields and raise food prices (USDA, 2012; USGCRP, 2017), with economic impacts extending well beyond the U.S. to countries importing our goods (FAO, 2012). In the electric power sector, changes in seasonal water shortage and elevated river temperatures are tandem concerns, reducing generation efficiencies and constraining power production during periods of peak demand (van Vliet et al., 2012; Miara et al., 2013). The management of agricultural impacts on water pollution extends from local up to regional to even continental scales, with land-to-ocean fluxes extending fully to the coastal zone (National Academies of Sciences, Engineering, and Medicine, 2022). The climate-dependent security of water supplies is also of concern as headline-dominating droughts persistently plague a large part of the nation (USDA, 2012; Wilhite et al., 2014; NY Times, 2022). Extremes in precipitation and concomitant flooding lead to damage in the built environment totaling tens of billions of dollars each year in direct and commercial trade-related impacts (Willner et al., 2018). Depending on its timing and severity, extreme rainfall during planting season can eliminate an entire year’s crop harvest (Li et al., 2019). Complicating such tradeoffs are environmental regulations, like the Clean Water Act, with its thermal effluent limitations crafted well before climate concerns entered the domain of citizen awareness or public policy (Kraft and Vig, 2006), but take on renewed importance as we develop strategies aimed at climate adaptation and mitigation.
Both TEI and NBI infrastructures underpin the nation’s FEWS. There are countless TEI components defining a full food-energy-water system, which itself interacts within a broader context of climate and other environmental conditions as well as specific investments in TEI made in the context of local to national-level economies, environmental and social safeguards, commitments to system maintenance, and the traditions of hydraulic engineering deployments (Vörösmarty et al., 2021). For the water sector, TEI supports water supply and sanitation, irrigation, hydropower, navigation, and flood/drought protection (ASCE, 2021) and there is growing interest in designing sustainable infrastructure in light of climate change and their related hazards (Röttgers et al., 2018; ISI Institute for Sustainable Infrastructure, 2022). Nature-based solutions for climate resilience have gained currency (UNEP-IUCN United Nations Environment Programme and International Union for Conservation of Nature, 2021). These recognize the value of ecosystem services (IPBES, 2018) and are central to achieving water security arising from climate change and other, more direct human-induced threats to water systems (e.g., pollution, poor land management) (WWAP/UN-Water, 2018; USACE-EWN, 2020). For this study, we define NBI broadly as landscapes (i.e., terrestrial ecosystems) connected to their aquatic counterparts (i.e., rivers, natural lakes, reservoirs, wetlands), with their functionality assessed collectively at the regional scale (Vörösmarty et al., 2021). Well-managed NBI assets support the production of food and biomass energy crops; provide clean water supply and pollution abatement through intact uplands and wetlands; and produce the water necessary to operate TEI (e.g., cooling water for thermoelectric power production), with clear, positive contributions that are regionally significant in economic terms (Costanza et al., 2014; Vörösmarty et al., 2021). NBI also plays a critical role in climate mitigation through the substantial carbon (C) sequestration potential of vegetation and soils but, if landscapes are mismanaged, as an additional source of emissions for CO2 and other radiatively-important gases (Lu et al., 2015; Sha et al., 2022).
In practice, TEI and NBI seldom operate in isolation to produce such services, and must be sensibly co-managed to sustain their societal benefits. The New York City water supply system is a quintessential example of a blended TEI-NBI, with the capital and maintenance costs of its massive engineered infrastructure determined in large measure by the integrity of its three provisioning water supply landscapes—two located across the Hudson River in the Catskill mountains and upper Delaware River and one in Westchester county north of the city. Suburbanization and the associated pollution runoff from the northern watershed necessitated a $3.2 B investment in 2015 for an advanced water filtration system, while the western watersheds can still rely on investments in landscape protection that maintains a high level of existing water quality and avoids the need for similar costly treatment (Hu, 2018). Another good example of the conjunctive use of engineered and natural systems involves electric power production, clearly produced directly by TEI, but a service that could not otherwise be realized without collaborating elements of NBI—the water provided by nature to cool turbines at power stations, the potential energy represented by river water stored behind dams to generate hydropower, or landscapes dedicated to commercial solar energy and wind production.
The condition of TEI and NBI also determines how these assets contribute to regional FEWS. Engineered infrastructure in the United States has routinely earned poor grades, creating the impetus for massive government spending on new systems (ASCE, 2021). For the water sector specifically, these poor grades have been used to justify the infusion of approximately $50B in new investments dedicated to clean drinking water and wastewater treatment (DeFazio, 2021). Similarly, poor management of NBI in upland watersheds diminishes the quantity, quality, and economic value of water provisioning services, placing downstream populations, the built environment, and ecosystems at risk (Vörösmarty et al., 2018). By some estimates (Costanza et al., 2014; Vörösmarty et al., 2021) current global losses of water security-related ecosystem services are disappearing at a rate of 2%–3% annually. Furthermore, the functional NBI contribution to global water security, if lost to poor environmental management, would require by 2030 $2.3 Tr annually to replace it with engineering, a figure more than twice the yearly expenditures for TEI (Vörösmarty et al., 2021).
The condition of one type of infrastructure yields reciprocal impacts on the other. The operation of water storage reservoirs can be severely compromised if erosion from uplands is left unchecked, resulting in major capacity losses (∼1% year−1 globally) (Zarfl and Lucía, 2018) and rendering much of the original TEI investment lost (George et al., 2017; Randle et al., 2021). Indoor urban water systems coupled to sewer networks yield well-recognized benefits to human health, but if accompanied by incomplete levels of wastewater treatment—the norm throughout much of the world—overtax the self-purification potential of receiving waters, substantially elevating downstream drinking water treatment costs (McDonald et al., 2016) and degrades aquatic habitat and biota (UN-Habitat and WHO, 2021). NBI-based instream self-purification can attenuate the problem to some degree, but may require a significant length of functional river course (Wollheim et al., 2008), which may or may not exist before downstream ecosystems or human populations are negatively impacted.
The kinds of interactions discussed above imply that the overall efficiency of FEWS will reflect the settings in which particular TEI and NBI investments exist. These infrastructures are distributed locally over space and time and combined in ways that make them unique: i) when viewed over broader more heterogeneous spatial domains; ii) through their spatial hydrologic connectivity; iii) placed into a regulatory or management context; and, iv) over longer time horizons that yield legacy effects. A good example of how these contexts interact is the Mississippi River drainage basin, where policy objectives of the Clean Water Act incurred substantial investments to upgrade wastewater treatment facilities. While these helped to control point source pollution (U.S. EPA, 2016) they these were not matched by corresponding reductions in diffuse agricultural pollution distributed over many thousands of stream and river lengths, thus obscuring much the benefit of the costly advanced systems and creating a chronic oxygen dead zone offshore of the delta (Secchi and McDonald, 2019). Another example is when surface waters generated by NBI are used to cool multiple thermoelectric power plants distributed sequentially across downstream river reaches, accumulating sufficient heat to then interfere with the performance of individual plants located downstream and their aggregate power production, while also exceeding Clean Water Act thermal tolerance limits for fish and other aquatic life forms (Miara et al., 2013). Tracking the carbon balance and sequestration potential of terrestrial ecosystems requires the careful spatial tracking of cohorts of landscapes, each with a unique carbon content and flux potential determined by history of human action. Here particular decisions regarding land clearance or abandonment as well as the incursion of urban and suburban landscapes produce long-term legacy impacts, detectable over multiple decades to centuries (Kicklighter et al., 2022). A macro-scale staging is also supported by recommendations made in the sequence of National Climate Assessments (NCA) [e.g., (USGCRP, 2018)], namely, that understanding climate impacts and crafting adaptation responses must be focused on the regional multi-decadal scale, owing to environmental and economic impacts that are uniquely sub-national and long-term in their evolution.
As we document in a companion paper applying the C-FEWS to the U.S. Northeast and Midwest (Vörösmarty et al., this issue), high quality inventories of many of the components of gray-green infrastructures are available as time series to support regional-scale studies, which in turn enable their derivative services to be quantified and linked to sensitivities produced by climate and non-climate forcings. Identifying these sensitivities helps to uncover cumulative impacts and tradeoffs involving key TEI and NBI-based policy “levers,” which can then be tested in scenario experiments aimed at optimizing FEWS performance over the long term.
Following early generalized conceptualizations (Hoff, 2011; World Economic Forum, 2011) several more articulated FEWS approaches and framings have emerged, with recent reviews (Albrecht et al., 2018; McGrane et al., 2018; Simpson and Jewitt, 2019) documenting a wide spectrum of themes (from the physical to the socioeconomic), scale (from local to basin to national, if not global), and degree of quantification of individual FEWS elements and their interactions. Lawford (2019) advocated the use of essential FEWS variables, based on monitoring data from ground-based networks as well as satellite remote sensing. Ingesting such information into data-rich accounting systems can then convert such inputs into indicators of FEWS performance (e.g., Giampetro et al., 2013; Daher and Mohtar, 2015; Sadegh et al., 2020), without necessarily formulating complex fully interacting models. McGrane et al. (2018) also discuss the role of input-output approaches as well as life cycle analysis as nexus-relevant quantitative tools. A theory-based framing of FEWS extending to social welfare considerations through a lumped water-energy-food consumption index has also been demonstrated (Teitelbaum et al., 2020). Decision-support systems can be used to frame FEWS research (Wolfe et al., 2016) and generate scenarios and affiliated tradeoffs (Daher and Mohtar, 2015; Daher et al., 2017).
Dargin et al. (2018) review a spectrum of FEWS approaches and report on a disarray in existing techniques, which are moving toward more complex simulation systems but narrowing the sectoral linkages and failing to capture some critical interactions. Nevertheless, dynamical configurations have been constructed, specifically for FEWS applied over national, sub-national and basin scales (Howells et al., 2013; Kling et al., 2017). CLEWS (Howells et al., 2013) represents a series of “soft-linked” models that maintain coordinated assumptions, input data sets, and treatments of essential interactions. The presence of strong FEWS linkages non-etheless argues, at least implicitly, for fully coupled or as completely coupled models as practicable. Integrated assessment models have been configured and used broadly to analyze policy and financial tradeoffs in the climate mitigation space (van Beek et al., 2020). Several include interactive Earth system components that link water cycle, land dynamics, and energy sector dynamics, so in principle these could be used productively to analyze FEWS interactions per se (Kling et al., 2017). However, integrated assessment models typically have prodigious computational overhead, require a large team to execute the algorithms, and extend well beyond FEWS itself and well beyond the spatial domain of regionally focused efforts like ours.
It is clear that the choice of existing frameworks is expansive, with tools and approaches often matched to a particular research question or geographic area of interest, but not ideally suited conceptually or practically to the study at hand. Nonetheless, the linked nature of these issues summarized in Sections 2.1-2.4 convinces us of the need for a systematic framing with a sufficient level of integration (Weaver et al., 2012; Leck et al., 2015) and this requirement guides our approach. In particular, societal needs revolve around detecting climate trends and extremes, diagnosing impacts on biogeophysical and human systems, and identifying regional management tradeoffs, all in the context of evolving environmental regulations and economic incentives. The framework we use must also enable a sufficient degree of contextualized (i.e., region-specific) modeling (Daher et al., 2017) but without seeking to capture idiosyncratic dynamics at the local scale. FEWS climate resilience is essentially a geographical question and the framework needs to accommodate models that are organized over space (i.e., regions depicted in pixel space, administrative units, river networks, drainage basins). In the context of a loosely coupled confederation of models, use of carefully monitored workflows, sufficiently mature and peer-reviewed algorithms, and shared performance metrics will be essential, particularly to systematically test hypotheses and answer fundamental questions. Further, we have the specific objective of exploring the roles of TEI and NBI on FEWS performance. Working with stakeholders adds an additional design requirement, that is, the capacity to distill otherwise complex modeling results into formats that facilitate dialogue between data providers and users. While the basic FEWS research needs to be executed using fully articulated, dynamic spatial models, we can also take advantage of reduced complexity approaches that convey to the stakeholders simplified depictions of otherwise complex dynamics (Bokhari et al., 2022).
The C-FEWS framework comprises a semi-coupled confederation of models, similar to the approach in CLEWS (Howells et al., 2013). This decision enables the use of existing, peer-reviewed algorithms and data sets, as well as efficient model set-up, execution, and post-processing. As explained below, this coupling arises from the standardization of time horizons, reporting units, and shared protocols for model execution and synthesis. We present models at two levels of organization. The first comprises six models operating in full (pixelated) and partially aggregated (e.g., country, state-level) geospatial mode, with a broad set of temporal dynamics (from minutes to days). The second level represents reduced complexity models (RCM) using lumped spatial parameters and a variety of timesteps (from daily to annual) and spatial aggregations to harmonize with other components of the project. We also develop a regional FEWS services portfolio and valuation model using state-level accounting units over an annual timestep. This section describes the overall framing, the component models and their chief data requirements.
The overall architecture for our research approach is given in (Figure 1), showing how we exercise the C-FEWS core models through digital data exchanges in a soft-linked configuration. An overview is given immediately below with additional elaboration and acronyms defined in Sections 3.3. Complete descriptions can be found in (Bokhari et al., 2022; Chang et al., 2022; Fekete et al., 2022; Kicklighter et al., 2022; Lin et al., 2022; Maxfield et al., 2022; Tuler et al., 2022; Zhang et al., 2022). Section 3.4 describes more specific computational exchanges invoked for the set of single and multi-factor experiments used in hypothesis testing and scenario analysis.
FIGURE 1. Key elements of the C-FEWS model suite, their connections, and the overall organizing framework.
Climate Forcings driving the C-FEWS Models are prescribed from the North American Land Data Assimilation Phase 2 (NLDAS-2) (Xia et al., 2012a; Xia et al., 2012b). These are combined with FEWS Specifications for exogenous water/land resource demand, technologies, operations, and management to test Hypotheses. WATER SYSTEMS are simulated for water supply, multi-sectoral use and pollution impacts. FOOD is modeled with ISAM (food crop biomass, resource demands, adaptation, agricultural emissions, nutrient leaching). ENERGY components in ISAM and TEM (for terrestrial C, resource demands greenhouse gas emissions, nutrient cycling) compute biofuel feedstock potential. ENERGY models also simulate thermo/hydroelectricity production. For the latter, power plant performance and thermal pollution are computed by TP2M, limited by climate and modified through technology/innovation/policy targets and deployments. C-FEWS MODELS also estimate levels of competition between the electric energy sector and food and biofuels production, when water becomes limiting. We also developed RCMs, simplifying the complex geospatial models to enable rapid conceptual testing of key FEWS dynamics. C-FEWS Performance Metrics summarize core model biogeophysical outputs and are used to evaluate the state of engineered and nature-based capital, which together generate a Regional Services Portfolio. This Portfolio guides Stakeholders in a workshop Charrette Process, including Scenario Co-Design with researchers and stakeholders jointly developing policy or technology targets. The Services Portfolio provides inputs to an Economic Valuation model to estimate, in dollar terms, the FEWS scenario outcomes. We then exercise an Optimization scheme to maximize positive outcomes while minimizing externalities. As model outputs reveal tradeoffs across the nexus, new targets can evolve and FEWS Specifications can be appropriately revised. This information feedback is activated through interaction with stakeholders. The C-FEWS MODELS in Figure 1 are referred to in this paper as assessment models.
Changing climate affects the frequency and intensity of many types of extreme weather events (Wuebbles et al., 2014; Wuebbles, 2018). The ongoing and unprecedented change in intensity and frequency of these events historically generate large and often negative socio-economic impacts expressed through the FEWS nexus. In this study, we analyze changes in the spatiotemporal patterns of four categories of extreme events: droughts, heat waves, extreme precipitation, and cold waves. These are analyzed in the first phase of our study over four recent decades (1980–2019) but are also relevant in the future (to 2100). The initial C-FEWS focus is on the 20 states constituting the U.S. Northeast (NE) and Midwest (MW) and uses the NLDAS-2 dataset. We developed a ranking method for each of the event types, integrating their duration, spatial extent and intensity across different timescales.
• Drought—We use the newly developed Drought Intensity Score (DIS) to define drought conditions across the CFEWS region (Sanyal and Wuebbles, 2022a). The metric is defined using the Standard Precipitation Index (SPI) (Svoboda et al., 2012), which can be defined as the number of standard deviations by which the observed anomaly deviates from the long-term mean over 1–36 months duration (Guttman, 1999; World Meteorological Organization, 2012). In this study, SPI-3 (3 months SPI), and the area affected by this condition, through a rank-based identification is used to define DIS. SPI-3 gives medium term moisture conditions and is very effective in agricultural regions to determine drought conditions. SPI-3 also has a better response rate compared to Palmer Drought Index (Svoboda et al., 2012). While SPI by itself informs the general wet/dry condition of a region, it is not as effective in identifying the degree of severity of drought. A DIS score greater than 4 indicates a severe drought condition over the region and less than 2 indicates very mild to no drought condition. Intermediate values intuitively represent more moderate drought conditions.
• Heat Wave—Heat waves can be defined as an extended period of extremely hot weather, most often accompanied by high humidity that has adverse effects on human health, agriculture, food services and the energy sector. The definition of a heat wave varies according to regions across the country and is measured compared to the local weather, where their impacts are most often assessed over smaller geographical domains, like city or county scale. If any pixel used in our analysis (1/8th degree long/lat) shows the following criteria for 3 consecutive days (Kew et al., 2019; Fisher et al., 2022), between April to September, we define it as a heat wave event (Sanyal and Wuebbles, 2022a): i) maximum temperature >95th percentile value; and, ii) Heat Index greater than 35 C. Heat Index is the apparent temperature felt by the human body when relative humidity is combined with temperature and is calculated using the Rothfusz equation described in a 1990 National Weather Service (NWS) Technical Attachment (SR 90-23) (Rothfusz and NWS Southern Region Headquarters, 1990). The time duration spans late spring to early fall. Since this study analyzes the impact of climate extremes over the broad C-FEWS region, area-weighted counts of 3-day events are calculated for the NE, MW and their combination. Total number of events in each region are then reported at an annual scale. These data are then used to identify and rank the years with the most impactful heat waves.
• Extreme Precipitation—Each grid box is identified to have an extreme precipitation event when its value exceeds 5 cm per day. Extreme precipitation events have become more frequent and intense in both the NE and MW (Sanyal and Wuebbles, 2022b), resulting in more instances of saturated soils and flash floods (Erlingis et al., 2019; Khajehei et al., 2020). Similar to the procedure for heat waves and cold waves, we calculate area-weighted threshold event values across the states, sub-regions, and the entire C-FEWS region at an annual scale.
• Cold Wave—We define a cold wave as a rapid decrease of temperature within a span of 24-h and low temperature spanning over a 3-day period. In this study, we define a cold wave spell in each pixel when three consecutive days experience a temperature less than or equal to −6.7°C (Sanyal and Wuebbles, 2022b). Like heat waves, cold waves are localized events but here identified over the larger NE, MW or combined macro-region. We further evaluate the number of 3-day area-weighted cold wave events at an annual scale and rank the aggregate results.
Existing C-FEWS models and their refinements are used to evaluate the effects of climate change and extremes, non-climate environmental drivers, and management actions on regional FEWS. The components are outlined immediately below. We begin by describing the individual models, detailing their characteristics and functions within the overall analysis scheme (Figure 1), as well as their data requirements. We also present our strategy for integrating the modeling results within the overall study and our approach to stakeholder engagement.
Owing to their complex regional-scale dynamics, we see that the best characterization of climate trends and extremes is achieved through multi-model techniques with project-specific, systematic analysis of results (Wuebbles et al., 2014; Zobel et al., 2017; Zobel et al., 2018). We used prescribed forcings from the NLDAS-2 (Xia et al., 2012a; Xia et al., 2012b), part of a multi-institutional project, aimed at producing spatially and temporally consistent, quality-controlled land surface model datasets, drawn from observed and reanalysis time series. NLDAS-2 was specifically created to reduce errors in soil moisture and energy, sometimes observed in numerical models. The model is run on a 1/8th degree grid with its geographical domain extending from 124.93oW to 67.06 oW and 25.06 oN to 52.93 oN. The forcings are mostly derived from North American Regional Reanalysis data (NARR) (Mesinger et al., 2006), along with monthly Parameter-elevation Regressions on Independent Slopes Model (PRISM) data (Daly et al., 1994). The NLDAS-2 dataset has been extensively analyzed with respect to the quality of its output variables (Xia et al., 2012a; Xia et al., 2012b), which for our baseline climate scenario uses 3-hourly surface temperature, precipitation, specific humidity, wind speed and long and shortwave radiation for the period 1980–2019. Table 1 shows the timing of extreme events recorded for the two macro-regions of the Northeast and Midwest. The table gives a synoptic picture that reflects the variability of each type of climate extreme, which is superimposed over longer-term trends spanning the 40 years of our historical baseline. Each of the entries are used to temporally bound the analysis of impacts that a particular climate extreme yields on different C-FEWS assessment models, as explained in Supplementary Appendices S1–S3 and explored in the last section of this paper.
TABLE 1. Target years and associated 5-year analysis periods (2 years pre/post) in the historical record for the US Midwest and Northeast during the early, middle, and late stages of the historical time period. Individual years representing specific extreme events* are identified using the methods summarized in the narrative. Individual years can be associated with multiple categories of events recorded (e.g., extreme precipitation and cold-waves across the MW in 2015; cold-wave and heat-wave in the NE in 2016). Adapted from (Sanyal and Wuebbles, 2022a; Sanyal and Wuebbles, 2022b).
Present-day carbon, water, and biogeochemical dynamics in terrestrial ecosystems reflect a century-scale legacy of NBI management, most importantly, land-use change across the NE-MW (Lu et al., 2015) but modified by nitrogen and water availability on landscapes, climate forcings, and atmospheric constituents that include CO2 and pollutants. These must be modeled insofar as land surface conditions in 2022 are ultimately a product of prior land cover and management decisions, some dating back to 1700. We use TEM’s dynamic cohort approach to represent land-cover change, abandonment, and regrowth (Reilly et al., 2012). In this approach, a grid cell is initially assumed to be entirely covered by a mosaic of undisturbed natural vegetation. When a disturbance occurs, such as timber harvest or conversion to agricultural or urbanized land, over a part of the grid cell, a new cohort is created and the area associated with the disturbance is subtracted from the affected natural cohort and is assigned to the new disturbed cohort. A new cohort may be created when part of an existing disturbed cohort changes land use (e.g., cropland to pasture, suburban to urban), experiences a new disturbance (e.g., timber harvest), or may be abandoned back to natural vegetation. For each of these cases, the new cohort is created with the area associated with the change subtracted from the existing disturbed cohort and assigned to the new cohort. As time progresses and more disturbances occur, more cohorts are added to the grid cell to track the entire land-use history of the grid cell in a time-series data set. A historical cohort time-series data set (1700–2019) has been developed for C-FEWS at 0.1o pixel (L/L) resolution by combining extant land-cover time-series data developed for the NE (Lu et al., 2013) and MW (Meiyappan and Jain, 2012) as described in (Kicklighter et al., 2022). We also consider future scenarios of regional population growth (U.S. EPA, 2017), land-use/cover consistent with the most recent IPCC Shared Socioeconomic Pathways (SSPs) (Riahi et al., 2017), plus land development pathways created with our Stakeholders.
The ISAM (Song et al., 2013; Niyogi et al., 2015) is used in C-FEWS to simulate food and bioenergy crops. ISAM calculates crop productivity, carbon, nitrogen, energy, and water fluxes at spatial resolutions ranging from 0.1o to 0.5o (L/L) and at multiple temporal resolutions ranging from one-half hour to yearly time scales. Thus, ISAM can capture diurnal and seasonal dynamics associated with individual crops at site, regional, and national scales. Some of the important features include: i) crop-specific phenology and dynamic carbon allocation schemes (Song et al., 2013; Song et al., 2014), accounting for the sensitivity of different crops to extreme cold, hot dry, and wet environmental conditions (e.g., frost, drought, waterlogging, etc.) and nutrient stress while allocating assimilated C to leaf, root, stem, and grain pools (Song et al., 2013); ii) dynamic vegetation structure that captures seasonal variability in LAI, canopy height, and root depth (Song et al., 2013); iii) dynamic root distribution processes at depth, to better simulate root-mediated soil water uptake and transpiration (Song et al., 2013); iv) heat stress impact during the reproductive periods simulated using canopy temperature (Gahlot et al., 2020; Lin et al., 2021); and, v) vertically-resolved C-N dynamics associated with soil organic carbon (SOC) profiles (across 10 layers) and their spatial heterogeneity (Shu et al., 2020). Recent model extensions include: vertical transport of SOC, discretized soil decomposition and abiotic modifiers for topsoil/subsoil, and a gas diffusion module for estimating oxygen availability and microbial control on SOC decomposition (Shu et al., 2020). Pastures and manure cycles are also simulated (Xu et al., 2021). These features are unique to ISAM and generally not included in other such models. We apply ISAM at 0.1o spatial resolution when simulating NE-MW’s major crops—maize, soy, spring/winter wheat, sorghum, and bioenergy crops including corn for ethanol, Miscanthus, and switchgrass.
The TEM is used to evaluate land-based natural infrastructure services, consistent with process-level insights from ISAM. TEM uses spatially-referenced information on climate, atmospheric chemistry, elevation, soils, land cover, and land use to estimate fluxes and pool sizes of C, N, and water in vegetation and soils. It is well-documented and has been used to examine patterns of land C dynamics over regional up to global scales, assessing impacts from multiple factors such as CO2 fertilization, climate change and variability, vegetation shifts, land-use change, and ozone pollution (McGuire et al., 2001; Tian et al., 2003; Felzer et al., 2005; Melillo et al., 2009; Reilly et al., 2012; Kicklighter et al., 2014; Melillo et al., 2016). TEM computes land C dynamics that strongly depend on the interactions between nutrients and water including: i) mineralization of soil organic N associated with litter and soil organic decomposition; ii) N inputs from fertilizer applications; and, iii) soil moisture which can limit decomposition, N mineralization and the capacity of plants to acquire inorganic N under drier conditions. TEM also simulates changes in C, N and water associated with ecosystem recovery after human and natural disturbance (McGuire et al., 2001; Balshi et al., 2007; Melillo et al., 2009; Reilly et al., 2012; Kicklighter et al., 2014). TEM (and ISAM) can determine bioenergy potential, focusing on 1st–3rd generation biofuels, emerging bioenergy technologies, and impacts of biofuels on land, energy, water, and climate (Heath et al., 2009; Warner et al., 2013; NREL, 2014).
We use the USGS SPARROW model to simulate land-to-waterway nitrogen (N) fluxes (Alexander et al., 2008; Shih et al., 2022). The original model has been used in a wide variety of contaminant sources and transport studies, including process investigations of stream denitrification (e.g., Alexander et al., 2000; Alexander et al., 2007), management-related studies of nutrient sources and their delivery to sensitive receiving waters (Alexander et al., 2008; Robertson et al., 2009; Preston et al., 2011), and forecasts of the effects of future climate and land-use change on nutrient and sediment fluxes (Bergamashci et al., 2014). Earlier studies include watershed assessments of nutrients (Alexander et al., 2008; Ator et al., 2011; Preston et al., 2011), total organic C (Shih et al., 2010), sediment (Brakebill et al., 2010), and streamflow (Alexander, 2015). The model is spatially explicit with separate source generation, landscape, instream and reservoir non-linear, and mechanistic process components that simulate engineered and natural (terrestrial and aquatic) nutrient processing infrastructures. More recent versions have been applied regionally in the NE-MW and Mid-Atlantic (Moore et al., 2004; Ator et al., 2011; Hoos et al., 2013). SPARROW has commonly been used to predict long-term means but can also handle seasonal nutrient flux over decadal periods, based on a dynamic formulation with transient storage components including historical nutrient source input legacies (Smith et al., 2014). SPARROW has been modified to account for the frequency of extreme climate conditions, and data inputs were altered to specifically accommodate C-FEWS single and multi-factor experiments. The SPARROW N model was statistically calibrated to account for dry/wet/hot/cold month frequency (Maxfield et al., 2021). Historical temperature and precipitation records (NLDAS, 2022) were used to generate mean frequency of occurrence per month of each of the four climate conditions over a 4-decade period to be used as predictor variables in the SPARROW calibration. This was done so that short-duration extreme climatic conditions could be reflected in the steady-state SPARROW model. We analyze both non-point source nitrogen pollution from cropland and atmospheric deposition plus point sources from wastewater treatment facilities (several 1,000s of plants), the latter using an EPA database digitized by our team (Rychtecka et al., 2010) and USGS analysis (Skinner and Maupin, 2019) plus livestock-based loading (Zering et al., 2012).
A suite of sub-models implemented within an earlier framework [FrAMES; Framework for Aquatic Modeling of the Earth System (Wollheim et al., 2008)] has been modified and used to simulate water supply and use. A water balance/transport model (WBM/WTM) (Vörösmarty et al., 1989; Vörösmarty et al., 1998) has been upgraded with several new capabilities relevant to FEWS: sectoral water use and management infrastructure with irrigation water use (including small reservoir effects) (Wisser et al., 2008; Wisser et al., 2010b) and reservoir operations for hydroelectricity (Wisser et al., 2010a; Fekete et al., 2010; Ehsani et al., 2015). We simulate these reservoir operations (WBMplus) with recent improvements based on neural network optimization for dam operation (Ehsani and Afshar, 2011) and spatial distributions from the National Inventory of Dams, combining this with extension to the MW of a NE interbasin transfer database (Buckley, 2013; Shikhmacheva, 2017), to compute regional reallocations of water. Data sets to drive FrAMES include climate (from ARRM2/CESM), water demands (including livestock, Zering et al., 2012), geolocated infrastructure, reservoir/lake location and dimensions, land cover and soils. Runoff is routed downstream using Muskingum-Cunge, accounting for both flowing streams and reservoir storage to predict spatially distributed discharge at daily time steps (Wisser et al., 2010a; Wisser et al., 2010b; Ehsani et al., 2015). We find that for regional applications using WBM/WTM a daily time step using 3’ (L/L) spatial resolution river networks (USGS, 2016) provides an adequate balance between accuracy and computational tractability (Fekete et al., 2002; Lehner et al., 2008).
Electric energy technology mixes are from the U.S. Energy Information Administration (EIA, 2022). Technology deployments (fossil, nuclear, renewables) reflect the suitability of facilities in the context of climate trends, their inherent space and time variability, and uncertainties in technology, economy, and policy drivers. The Thermoelectric Power & Thermal Pollution Model (TP2M) (Miara and Vörösmarty, 2013a; Miara and Vörösmarty, 2013b) couples power plant engineering, hydrology, and riverine thermal transport submodels. Its regional application to the NE (Miara et al., 2013; Stewart et al., 2013) with 384 power stations quantified the importance of the region’s hydrologic systems in providing an essential NBI-based ecosystem service, that is, the transport and dissipation of power plant heat. As in Miara et al. (2013) for current climate, we simulate the impacts of greenhouse warming on regional power plant operating capacity and temperature-dependent efficiencies, and assess these with/without adherence to Clean Water Act (CWA) regulations mandating shutdowns during times of excessive heat. We have geo-located and characterized 87% of thermoelectric stations nationwide (n = 1080) (Miara et al., 2017), from which we draw our C-FEWS NE and MW subsets, n = 266 and 228 power plants, respectively.
The societal impact of TEI and NBI investments and policies are estimated using an economic valuation model, VM. This model provides value estimates using a social surplus valuation methodology (Letourneau et al., 2015; Sanders and Barreca, 2022) for outcomes such as food and energy that are sold in monetary transactions. Such values are the difference between the benefit of such goods to consumers and the costs of producing them. Social surplus is the area between the supply curve (capturing unit costs of production) and the demand curve (capturing the benefit of each unit to society) integrated over the units sold in the market. We calculate the change in social surplus for a good between a baseline and alternative scenario by using data on baseline price and quantity sold in the two scenarios, information about the slopes of supply and demand curves from previous research, and information from the outputs of the C-FEWS models on how much weather conditions shift the supply curve up or down. VM also uses a benefit transfer methodology (Richardson et al., 2015; Hungate et al., 2017) to estimate values of non-market goods in our scenarios (e.g., for C sequestration and water pollution abatement) based on previous research. Methodological details are in Chang et al. (2021 and this issue).
A suite of stand-alone and coupled Reduced Complexity Models has been developed as a diagnostic tool to more understand linkages across the FEWS and diagnose its systemic behaviors that otherwise would be limited by the higher computational burdens of the original C-FEWS high resolution models. The RCMs are also cast to explore scenario and parameter sensitivities and to engage with stakeholders. Three mass and energy balanced RCMs at the basin scale (for hydrology, thermal pollution and energy, and N mobilization and transport) were adapted in part from three complex, fully spatially distributed counterpart models from the C-FEWS framework: WBM/WTM (Vörösmarty et al., 1989; Vörösmarty et al., 1998), TP2M (Miara et al., 2013; Stewart et al., 2013), and SPARROW (Moore et al., 2004; Ator et al., 2011; Hoos et al., 2013; Saleh and Domagalski, 2015). The RCMs aggregate climate, infrastructural, and hydrological input data of varied spatial resolution (12 km grid cells to county-level reports) to the basin scale in order to capture: i) major fluxes and stocks of the terrestrial water cycle, including snowmelt and rainfall runoff, evapotranspiration, and river discharge at the daily time scale; ii) the impacts of power plant operation on downstream river temperature, water consumption, and power generation at the daily time step; and, iii) nitrogen mobilization and transport from atmospheric and landmass sources (e.g., deposition, industrial fertilizer, livestock, and human waste) to riverine receiving waters at an annual time scale. The RCMs are calibrated and validated using observed stream gauge data and explored through single factor climate and infrastructure experiments as for the fully resolved models (Supplementary Appendices S1–S3) as part of our historical simulations. An initial a test case is on the Delaware River Basin (Bokhari et al., this issue). With the icon-based programming language STELLA Architect (isee systems, inc., Lebanon NH, United States), the RCM framework allows for rapid reconfiguration of a simulation (e.g., creating new state variables, changing links across variables; assigning different parameter values) and multi-objective optimization to study tradeoffs among FEWS linkages, all with computation times of under a minute, and representing a capability of enormous value in engaging with stakeholders.
A preliminary version of the optimization module is being linked to the reduced complexity models (RCMs) and will be exercised in analysis of management scenarios operating under future climate and other environmental change. The focus of these optimization studies is to explore simulated tradeoffs between thermal pollution and thermoelectric power generation in single river basins. As demonstrated for the NE (Miara et al., 2013; Stewart et al., 2013), riverine power plant efficiencies rely on the withdrawal and consumption of water for cooling, which can result in both power generation losses for downstream plants and the impairment of ecosystem services during periods of excessive heat discharge. Two basin scale RCMs – corresponding to hydrology, electricity production, and thermal pollution–which operate at the daily time scale are coupled via hydrologic linkages (i.e., river discharge, velocity, and depth) as modules of a single aggregate model (Bokhari et al., this issue). The coupled model simulates: thermal pollution in the form of river temperature increase from power plants; impacts of river temperature on downstream power generation efficiency; and, the interdependent feedbacks that these outputs create for downstream power plants. The ‘Multiobjective Optimization’ tool in Stella Architect (isee systems, inc.; Lebanon NH, United States) is used to facilitate and design scenario experiments for freshwater utilization, infrastructures, technologies, and policies. These experiments seek to evaluate tradeoffs for multi-factor impacts on the thermal regime of a river basin by computing a set of optimal solutions (i.e., optimized Pareto front using a differential evolution algorithm) that minimizes thermal pollution while maximizing power generation for a given constraint. The coupled model can be used to study, for example, the multi-objective optimizations of daily power plant operation and capacity, plant cooling technologies, upstream reservoir operation and capacity, in the context of the Clean Water Act’s regulation of water temperature limits (Copeland, 2016). Economic valuations are also incorporated in this analysis (see Economic Valuation, above), thus enabling a comparison of the damages to downstream aquatic habitats and commercial fisheries versus losses in electricity generation when CWA regulations are otherwise enforced. The same overall approach can be applied to synthesized distillations of the more complete C-FEWS geospatial assessment models.
We use off-the-shelf estimates of past and future trends in air quality based on our own and other studies (e.g., Lin et al., 2008; Weaver et al., 2009; Lei et al., 2013; He et al., 2016; He et al., 2018). Air quality in the C-FEWS framework is represented by atmospheric CO2 concentrations, atmospheric deposition of reduced (NHx) and oxidized (NOy) nitrogen forms, and ozone pollution as represented by accumulated ozone over a 40 ppbv threshold (AOT40) (Felzer et al., 2004). In the current study, annual mean global atmospheric CO2 concentrations are prescribed from 1700 to 2019 based on two studies: 1765 to 2015 (Meinshausen et al., 2011) and 2016 to 2019 (Dlugoclenky and Tans, 2021). Mean atmospheric CO2 concentrations before 1765 are assumed to equal those in 1765 (278 ppmv). Both spatial and temporal variability in atmospheric nitrogen deposition and AOT40 across the region are represented with gridded time-series data. Gridded time series data for monthly atmospheric nitrogen deposition are based on NADP (National Atmospheric Deposition Program, 2022) for 2000 to 2018 and extend backward and forward for the rest of the years following the trend from the CMIP6 Chemistry-Climate Model Initiative (CCMI) (Hegglin et al., 2022). Gridded time series data for monthly AOT40 are based on ozone estimates from simulations by the MIT Integrated Global System Model linked to the NCAR Community Atmospheric Model (IGSM-CAM) (Monier et al., 2013).
An appropriate staging of the C-FEWS models (Figure 1) and their harmonization (i.e., via driving variables, time and spatial resolutions) become essential parts of the framework exercise, especially given the sizable number and range of climate and sectoral scenarios and the large volumes of outputs generated. Some of the C-FEWS models are formally coupled (e.g., WBM-TEM, WBM-TP2M) while others lack such integration. From our prior experience using multi-model approaches analyzing energy-water interactions, we find that rigidly seeking a formal coupling of models: i) can consume inordinate computing and personnel resources; ii) impedes rapid turnaround for model calibration/validation and scenario testing; and, iii) may ultimately prove unnecessary (e.g., inconsequential feedbacks between SPARROW-based N fluxes and WBM/TP2M energy production). Following a partial coupling approach (Howells et al., 2013), we therefore developed a computational framework that uses fast backbone transfer protocols coupled with specific data staging/conversion routines to ensure that data flows from one model to the next are as simple and efficient as possible.
There are several reliable protocols available for the necessary data transfers, and we developed a multi-tier approach. Larger data sets that required distribution to all of the C-FEWS research teams were managed using a Globus endpoint (Foster, 2011; Allen et al., 2012), while for more selective distribution and atomized access we used a GeoServer backend (GeoServer.org, 2022), which allows for data streaming and direct integration into analytical platforms such as GIS software or any programming framework. Simpler file exchange protocols, such as a NextCloud (NextCloud.com, 2022), are used for a documents repository and project administration. These platforms, integrated with our existing FrAMES, organize the overall C-FEWS data handling and workflows (model execution in space and time, I/O management for forcing data, state variables and diagnostics, final data outputs). A component of the model integration involves the creation of C-FEWS Performance Metrics (Supplementary Appendix S4), which are used to summarize the biogeophysical and economic modeling outputs for model calibration and validation, constructing a portfolio of regional C-FEWS services and further distilled into quantitative information used to support the stakeholder workshops.
We describe here the experimental set-up of our diagnostic and prognostic tests, which we use to explore how climate extremes produce vulnerabilities and/or resilience across the regional FEWS. We assess these emergent properties by first creating a historical benchmark, comprising the observed climate from 1980–2019 plus the recorded exogenous, non-climate determinants that drive each of the assessment models. The climate and non-climate forcings are then reconfigured to create single and multi-factor experiments.
Single Factor Experiments (SFEs) are divided into two sub-groups (Table 2). First, we construct single factor climate experiments (cSFEs), representing each of the four categories of climate extremes (drought, heat-wave, extreme precipitation, cold-wave). We use three approaches to simulate the climate impacts: i) the verbatim observational record (Supplementary Approach A); ii) a case with exacerbated climate extremes (B); and, iii) a de-extremed scenario (C) (Supplementary Appendix S1). By comparing these results to Baseline, we can evaluate whether repeated climate events yield a cumulative impact when superimposed onto longer-term climate trends. Table 1 gives the most prominent extreme event years adopted for Supplementary Approach A.
TABLE 2. The four main categories of FEWS elements manipulated using the Single Factor Experiments, expressed for climate (cSFEs) and for non-climate entities (ncSFEs), and dedicated to uncovering FEWS sensitivities. For the ncSFEs, each subordinate entry defines a particular variable that is fixed at 1980 levels while the baseline otherwise progresses as in the historical time series (i.e., with all other elements varying as observed). The resulting time series of assessment model output variables under the scenario can then be compared to baseline. The sensitivity of any assessment model variable or collection of variables to a single or multiple perturbation can be computed using Δ statistics as described, using specific examples, in Supplementary Appendices S1–S3. Relevancy to engineered or nature-based infrastructure is indicated.
Second, we stage non-climate single factor experiments (ncSFEs) to explore the impact of evolving technology, land use, management and regulations (Supplementary Appendix S2). The ncSFEs are generated by fixing key variables in the assessment models at their initial 1980 values, running the models with these variables inactivated, and then comparing results to Baseline in the last decade of the time series (2010–19). Using simple normalized differences relative to Baseline (specified below) or more complex signal-to-noise approaches and optimal fingerprinting we can detect signatures of single and multiple factor effects (Stein and Alpert, 1993; Hegerl et al., 2006; Hegerl et al., 2007; Santer et al., 2009) in these counterfactual experiments. By selecting key variables from our assessment models and isolating them individually or in tandem, we can discover the degree of control each exercises on sector-specific as well as overall FEWS performance. Multi-factor experiments (“MFEs”) (Supplementary Appendix S3) combine individual climate and non-climate factors to assess their interactions within FEWS and to identify and evaluate potential feedbacks. These outputs are then quantified with respect to the support or refutation of hypotheses, measures of system sensitivity, and full system impact. Information on calibration and validation of the C-FEWS assessment models is given in Supplementary Appendix S4.
The manipulations that form the experiments in Table 2 are also designed to elucidate the contributions of TEI or NBI (and their combination) to regional FEWS performance. There are two components of the analysis. The first involves manipulating the forcing factors. This action, at least in principle, can uncover some of the key targets for regional planning and FEWS management under each of the non-climate SFEs or MFEs to identify the importance of specific TEI or NBI-based factors. For each of the forcing factors listed within the three non-climate themes in Table 2, we indicate the predominant category of associated infrastructure to which manipulation of that variable can provide insight. For example, the impacts of changing technology on FEWS energy production are reflected by different fuel mixes or power plant cooling systems, TEI components. Inactivating elements of land cover change (e.g., suburbanization, reforestation) is an obvious NBI manipulation. Technology can span both TEI and NBI, for example, using cultivars from biotechnology in crop production. Environmental regulations, like regional net carbon emission targets, also arguably combine TEI (through emission technologies in fossil fuel facilities) and NBI, through land use C sequestration or biofuels.
The second component explores not the causes of but the impacts on TEI and NBI generated by the manipulations given in Table 2 as exercised through the cSFEs, ncSFEs, or MFEs (Table 3). These outputs are expressed as TEI and NBI performance metrics, emerging as essential indicators of the state and functionality of regional FEWS. These outputs therefore can be compared within and across each of the experiments. Several of the comparisons we report use a relative measure of impact sensitivity, computed as a normalized difference calculation, as given in Supplementary Appendix S1 for the cSFEs, Supplementary Appendix S2 for the ncSFEs, and Supplementary Appendix S3 for the MFEs.
TABLE 3. Core variables computed by the suite of C-FEWS models, organized by sector and by assignment as representative of the performance of different infrastructures. These variables are used to evaluate system impacts from the climate and non-climate factors manipulated to produce the contrasting scenarios given in Table 2.
C-FEWS model biogeophysical outputs are also summarized into quantitative metrics that comprise a regional FEWS Services Portfolio (Figure 1) together with its economic valuation. Interactions with stakeholders center around this portfolio and, as a consequence of our consultations, may require a reconstitution of the chosen forcings, SFE and/or MFE design, and reported model outputs. In our companion paper (Vörösmarty et al., 2022), we present representative findings from our initial study on SFEs and MFEs over the historical period across the NE and MW, which lays the groundwork for analysis of future conditions.
Regional planners increasingly recognize the importance of a ‘whole-landscape’ approach to decision-making (DeFries and Rosenzweig, 2010) that includes land-use planning, environmental legislation, and global change impacts. This transformation benefits from high quality regional-scale climate and weather projections embedded within land, water, and energy management scenarios (Allen et al., 2013; Rosenzweig et al., 2014). Our stakeholder dialogue—organized as Charrettes—has been designed to acknowledge those planning variables deemed meaningful by users, but it also attempts to understand their logic in constructing options, for example, particular landscape and water use scenarios or choice of power sector technologies. Unforeseen byproducts emerge from such outreach, as in our dialogue with lawyers challenging EPA decisions on Clean Water Act Section 316(b) thermal loading requirements and threatened aquatic biota under the Endangered Species Act (Super Law Group, 2013), who recognized the capacity of WBM/TP2M (Miara and Vörösmarty, 2013a; Miara et al., 2013; Stewart et al., 2013) to map the collective thermal impact of power sector emissions by TEI but also attenuation by aquatic NBI over entire regions. Further, the stakeholder dialogue facilitates an articulation of otherwise extreme, but potentially plausible scenarios, as with possible future migration north to escape extreme heat outbreaks and drought in other parts of the country (Black et al., 2011; USGCRP, 2017).
To ensure relevancy beyond pure research, we created a C-FEWS Stakeholder Working Group comprising participants actively involved in land-use and energy sector planning, climate mitigation and adaptation, and civil sector investment strategies for infrastructure at both local and regional scales. In partnership with the Group, we i) co-design regionally-focused socioeconomic scenarios to reflect stakeholders’ information needs; ii) work stepwise through storyline development; iii) convert conceptual inputs into numerical data assignments; iv) iteratively present and interpret results; and, v) re-cast data for model parameterization and driving variables in response this iterative process (Rosenzweig et al., 2014). To achieve such engagement, we execute short 1-day hybrid virtual workshops and interim meetings to gather information on the design of additional single-factor and multi-factor experiments and share in the interpretation of results. We have sought stakeholders who are active in regional planning across the spectrum of climate and FEWS, and who can discuss the engineering and nature-based “policy levers” necessary for improved climate resiliency.
The single and multi-factor experiments produces a large matrix of possible interactions among climate, technology deployments, land use strategies, and management/regulation. Here we provide a sampling of how some of the potential, major categories of FEWS-climate issues, posed as questions, can be addressed using the C-FEWS framework. Results from a first suite of such experiments are summarized in (Vörösmarty et al., 2022), based on a more in-depth collection of C-FEWS experiments carried out over the same historical time frame of 1980–2019 (Bokhari et al., 2022; Chang et al., 2022; Fekete et al., 2022; Kicklighter et al., 2022; Lin et al., 2022; Maxfield et al., 2022; Tuler et al., 2022; Zhang et al., 2022).
What is the capacity of a regional FEWS to endure or benefit from the four types of climate extremes (drought, heatwaves, extreme precipitation, cold waves) during the early, middle, and late periods of our historical record?
This question can be addressed by straightforward application of Approach A among the single factor climate experiments (cSFEs). It explores the immediate impact of a climate extreme identified from within the recorded historical period. The designated event-year is analyzed with respect to any pre-conditioning (2 years prior) as well as its immediate legacy effects (2 years post event). Given the evolution of the non-climate themes (technology, land use, management/regulations), which could substantially influence event response, three time periods are studied—the early, middle, and late phases of the 40-year time series. This enables examination of the impacts of the extreme event within the broader context of history, that is, with all variables (climate and non-climate) evolving within a multi-decade time horizon. We thus can explore how critical either precursor or post-event legacy effects are to FEWS performance. For example, we can pose and answer a subsidiary question: To what degree is crop production across the three recurring, 5-year extreme climate event sequences made more or less resilient by planting new cultivars? For the food sector, the question could be addressed by the counterfactual experiment removing historical biotechnology improvements from the ISAM model, examining how the crop production would have evolved during each 5-year sequence over the early, middle and late periods of the 40-year record, and comparing these results to Baseline.
Supplementary Approach B climate attempts to address a similar question, through a hypothetical scenario, which attempts to capture the anticipated potential for more frequent and/or sustained extremes associated with climate change (Sanyal and Wuebbles, 2022a; Sanyal and Wuebbles, 2022b). It focuses on the last decade and creates a synthetic time series with an increased frequency of extreme years. We identify the year of maximum (or minimum) extreme in the Baseline for 2010–19 and identify the two subsequent years (e.g., heatwave year 2012, with 2013 and 2014). Using this 3-year period, we then triple its frequency of occurrence commencing in 2010 (i.e., three 3-year duration events versus one in the baseline time series over the last decade of the analysis). Supplementary Approach B assesses responses over the last decade of the historical time period, from which the potential readiness of near-contemporary land use, technology and management/regulations to meet imminent climate challenges can be evaluated (i.e., prior to formally analyzing forecasts of the future). Supplementary Approach B is analogous to Supplementary Approach C, but focuses on the opposite effect, i.e., the removal of extremes.
What were the roles of each major category of non-climate factors across a region that enabled FEWS to remain productive (or not) over the 40-year period of 1980–2019, with its mixture of recorded climate extremes?
The C-FEWS framework can be used to explore the long-term system-wide performance associated with individual variables drawn from the three themes that represent non-climate factors (land use, technology, management/regulations). Single factor non-climate scenarios (ncSFEs) can be constructed by inactivating change to the inputs representing a single, specific non-climate factor within each of the assessment models (Table 2). For the retrospective time period, this inactivation of a particular non-climate variable fixes its value at the 1980 level, whereas for future forecasts they are benchmarked to 2020. Our standard approach is to impose the historical time series of the unmodified climate (through Supplementary Approach A) and non-climate drivers and to then record differences between this Baseline and that of the scenario with the inactivated variable. This yields a measure of FEWS sensitivity to the particular non-climate input varied in the scenario, with summary statistics enabling comparisons, rankings, and statements regarding its overall importance (Supplementary Appendices S1–S3). These experiments can also be used to explore the presence of progressive system stress, from which we can draw inferences on how the changing state of land, technology, and management/regulations, decade by decade, amplify or attenuate the impact of the observed climate stresses. A similar analysis can be formulated using the assumed climates associated with Supplementary Approaches B,C over the last decade of the historical period (2010–2019). By running a full suite of such ncSFEs, we can assemble a picture of the individual importance that green and gray infrastructures play in determining FEWS outcomes, decade by decade. We also can assess TEI and NBI sensitivities on variables that are not manipulated (Table 3).
What were the roles of climate and different combinations of the main non-climate factor variables (technology, land use, management/regulations) across a region that enable the FEWS to remain productive (or not) in response to climate over the historical period?
Here the C-FEWS frame can be used to explore the short and longer-term impact of specific combinations of non-climate driving variables acting in concert with different climatic conditions to jointly determine FEWS performance. These different combinations of cSFE and ncSFEs comprise multi-factor experiments (MFEs). Under Supplementary Approach A for the historical time series, we inactivate two or more non-climate factor inputs controlling the assessment models. For the retrospective time period, this inactivation of particular non-climate variables fixes their values at 1980 levels, while for the future the values are fixed at 2020 and a climate time series produced by an atmospheric forecast model commences. Our standard approach is to record differences between the baseline performance metrics (both historical and future) and that of the scenario with the inactivated combination of non-climate variables. Under Supplementary Approaches SA, SB, multiple input factors can be modified, but a climate event itself (e.g., intensified drought) can become one of the multi-factors to be tested. To do so we combine the climate scenario with inactivated non-climate variables, evaluate differences from the baseline and thus create a climate/non-climate multi-factor experiment. MFEs enable inferences to be made about how the changing state of land, technology, and management/regulations, decade by decade, amplify or attenuate the impact of climate stresses. In this way we can help determine how the conjunctive manipulation of particular themes and collections of variables from Table 2 can build resilience into FEWS in light of different climate stresses. As for the first two questions, an appropriate choice of input variable enables an identification of the roles of engineered and nature-based infrastructure in determining overall system sensitivity and resilience patterns.
In a companion paper (Vörösmarty et al., 2022), we exercise the analytical strategies discussed above over the historical time frame, highlighting the use of several of the C-FEWS component models to gain essential insight into the behavior of regional food-energy-water systems in the U.S. Northeast and Midwest. In that work, we demonstrate the clear impacts that climate stresses have already have had on FEWS, but also show how the other strategic forces have been at work—technology, land use, management/regulation. These factors have combined to create additional vulnerabilities as well as opportunities for ongoing adaptation to climate change, whether purposeful or inadvertent. We see important roles for both engineered and nature-based infrastructures, separate and in combination, in defining the contemporary state of FEWS and positioning these important resource systems to encounter future challenges.
The raw data supporting the conclusion of this article will be made available by the authors, without undue reservation.
Conceptualization: CV, JM, DW, AJ, AA, and MC; method development: CV, MC, and SS; formal analysis: MC, DK, BF, HB, JC, T-S L, NM, SS, JZ, and ST; writing/review/editing: CV, JM, DW, AJ, AA, ST, RS, DK, and AM; framework data and IT support: FC and DV; project administration: CV and MC; student/post-doctoral mentoring: CV, DW, AJ, AA, ST, RS, and FC. All authors have read and agreed to the published version of the manuscript.
Financial support for this work was provided by the U.S. National Science Foundation’s Innovations at the Nexus of Food, Energy and Water Systems Program (INFEWS/T1 Grant #1856012). We also acknowledge V. Puricelli for assistance in formatting the final manuscript.
The authors declare that the research was conducted in the absence of any commercial or financial relationships that could be construed as a potential conflict of interest.
All claims expressed in this article are solely those of the authors and do not necessarily represent those of their affiliated organizations, or those of the publisher, the editors and the reviewers. Any product that may be evaluated in this article, or claim that may be made by its manufacturer, is not guaranteed or endorsed by the publisher.
The Supplementary Material for this article can be found online at: https://www.frontiersin.org/articles/10.3389/fenvs.2023.1069613/full#supplementary-material
Albrecht, T. R., Crootof, A., and Scott, C. A. (2018). The water-energy-food nexus: A systematic review of methods for nexus assessment. Environ. Res. Lett. 13 (4), 043002. doi:10.1088/1748-9326/aaa9c6
Alexander, R. B. (2015). Advances in quantifying streamflow variability using hierarchical Bayesian methods with SPARROW. PhD Dissertation. The Pennsylvania State University, College of Agricultural Sciences, 281pp.
Alexander, R. B., Boyer, E. W., Smith, R. A., Schwarz, G. E., and Moore, R. B. (2007). The role of headwater streams in downstream water quality. JAWRA 43 (1), 41–59. doi:10.1111/j.1752-1688.2007.00005.x
Alexander, R. B., Smith, R. A., Schwarz, G. E., Boyer, E. W., Nolan, J. V., and Brakebill, J. W. (2008). Differences in phosphorus and nitrogen delivery to the gulf of Mexico from the Mississippi River Basin. Environ. Sci. Tech. 42 (3), 822–830. doi:10.1021/es0716103
Alexander, R. B., Smith, R. A., and Schwarz, G. E. (2000). Effect of stream channel size on the delivery of nitrogen to the Gulf of Mexico. Nature 403, 758–761. doi:10.1038/35001562
Allen, B., Bresnahan, J., Childers, L., Foster, I., Kandaswamy, G., Kettimuthu, R., et al. (2012). Software as a service for data scientists. Comm. ACM 55 (2), 81–88. doi:10.1145/2076450.2076468
Allen, E., Kruger, C., Leung, F.-Y., and Stephens, J. C. (2013). Diverse perceptions of stakeholder engagement within an environmental modeling research team. J. Environ. Stud. Sci. 3 (3), 343–356. doi:10.1007/s13412-013-0136-x
Arent, D., Pless, J., Mai, T., Wiser, R., Hand, M., Baldwin, S., et al. (2014). Implications of high renewable electricity penetration in the U.S. for water use, greenhouse gas emissions, land-use, and materials supply. Appl. Energy. 123, 368–377. doi:10.1016/j.apenergy.2013.12.022
ASCE (2016). Policy statement 360-impact of climate change. Energy, environment and water policy committee, public policy committee. Reston, VA: Board of DirectionASCE.
ASCE (2021). Report card for America’s infrastructure. American Society of Civil Engineers. Available at: https://infrastructurereportcard.org.
Ator, S. W., Brakebill, J. W., and Blomquist, J. D. (2011). Sources, fate, and transport of nitrogen and phosphorus in the Chesapeake Bay Watershed: An empirical model. Reston, VA: U.S. Geological Survey Scientific Investigations Report, 2011–5167.
Balshi, M. S., McGuire, A. D., Zhuang, Q., Melillo, J., Kicklighter, D. W., Kasischke, E., et al. (2007). The role of historical fire disturbance in the carbon dynamics of the pan-boreal region: A process-based analysis. J. Geophys. Res. 112 (G2), G02029. doi:10.1029/2006jg000380
Bergamaschi, B. A., Smith, R. A., Sauer, M. J., Shih, J.-S., and Ji, L. (2014). “Terrestrial fluxes of nutrients and sediment to coastal waters and their effects on coastal carbon storage in the eastern United States,” in Baseline and projected future carbon storage and greenhouse-gas fluxes in ecosystems of the eastern United States. Editors Z. Zhu,, and B. C. Reed (Reston, VA: U.S. Geological Survey). SV-1804.
Black, R., Adger, W. N., Arnell, N. W., Dercon, S., Geddes, A., and Thomas, D. (2011). The effect of environmental change on human migration. Glob. Environ. Change 21, S3–S11. S3–S11. doi:10.1016/j.gloenvcha.2011.10.001
Bokhari, H. H., Najafi, E., Dawidowicz, J., Wuchen, L., Maxfield, N., and Vörösmarty, C. J. (2022). Simulating basin-scale linkages of the food-energy-water nexus with reduced complexity modeling. Front. Environ. Sci. (Special Issue FEWS).
Brakebill, J. W., Ator, S. W., and Schwarz, G. E. (2010). Sources of suspended-sediment flux in streams of the chesapeake bay watershed: A regional application of the SPARROW model. JAWRA 46 (4), 757–776. doi:10.1111/j.1752-1688.2010.00450.x
Brown, M. E., Antle, J. M., Backlund, P., Carr, E. R., Easterling, W. E., Walsh, M. K., et al. (2015). Climate change, global food security, and the U.S. Food system. Washington, DC: U.S. Global Change Research Program, 146. doi:10.7930/j0862dc7
Buckley, J. (2013). Quantifying the impacts of inter basin transfers on water balances in the conterminous United States. Raleigh, NC: North Carolina State University. Available at: http://repository.lib.ncsu.edu/dr/handle/1840.4/8150.
Challinor, A., Elliott, J., Kent, C., Lewis, K., and Wuebbles, D. (2015). “Climate and global crop production shocks,” in UK-US taskforce on extreme weather impact on food resilience (UK: Science and Innovation Network). Available at: http://www.foodsecurity.ac.uk/assets/pdfs/climate-and-global-crop-production-shocks.pdf.
Chang, J. W., Ando, A. W., and Chen, M. (2022). (this issue) Valuing changes in the portfolio of service flows from climate-induced extremes on a linked Food, Energy, Water System (C-FEWS). Front. Environ. Sci. (Special Issue FEWS).
Chang, J. W. (2021). Valuation tool for food-energy-water portfolio outcomes. Urbana-Champaign: MSc Thesis, University of Illinois. https://www.ideals.illinois.edu/items/120926.
Copeland, C. (2016). Clean water Act: A summary of the Law. Washington, DC: Congressional Research Service. Available at: https://sgp.fas.org/crs/misc/RL30030.pdf.
Costanza, R., de Groot, R., Sutton, P., van der Ploeg, S., Anderson, S. J., Kubiszewski, I., et al. (2014). Changes in the global value of ecosystem services. Glob. Environ. Change 26 (26), 152–158. doi:10.1016/j.gloenvcha.2014.04.002
Daher, B., Mohtar, R. H., Lee, H., and Assi, A. (2017). “Modeling the water-energy-food nexus,” in Water-energy-food nexus: Principles and practices. Editors P. Abdul Salam, S. Shrestha, V. Prasad Pandey, and A. K. Anal (Washington, DC: John Wiley & Sons), 55–66.
Daher, B., and Mohtar, R. H. (2015). Water–energy–food (WEF) nexus tool 2.0: Guiding integrative resource planning and decision-making. Water Int. 40 (5-6), 748–771. doi:10.1080/02508060.2015.1074148
Daly, C., Neilson, R. P., and Phillips, D. L. (1994). A statistical-topographic model for mapping climatological precipitation over mountainous terrain. J. Appl. Meteorol. 33 (2), 140–158. doi:10.1175/1520-0450(1994)033<0140:astmfm>2.0.co;2
Day, J. W., Moerschbaecher, M., Pimentel, D., Hall, C., and Yáñez-Arancibia, A. (2014). Sustainability and place: How emerging mega-trends of the 21st century will affect humans and nature at the landscape level. Ecol. Eng. 65, 33–48. (special issue: Sustainable Restoration). doi:10.1016/j.ecoleng.2013.08.003
DeFazio, P. A. (2021). Text - H.R.3684 - 117th congress (2021-2022): Infrastructure investment and jobs Act. Available at: https://www.congress.gov/bill/117th-congress/house-bill/3684/text.
DeFries, R., and Rosenzweig, C. (2010). Toward a whole-landscape approach for sustainable land use in the tropics. P. Natl. Acad. Sci. 107 (46), 19627–19632. doi:10.1073/pnas.1011163107
Dieter, C. A., Maupin, M. A., Caldwell, R. R., Harris, M. A., Ivahnenko, T. I., Lovelace, J. K., et al. (2018). Estimated use of water in the United States in 2015. U.S. Geol. Surv. Circ. 1441, 65. doi:10.3133/cir1441
Dlugoclenky, E., and Tans, P. (2021). Trends in atmospheric carbon dioxide. National Oceanic and Atmospheric Administration. Earth System Research Laboratory (NOAA/ESRL). Available at: http://www.esrl.noaa.gov/gmd/ccgg/trends/global.html (Accessed May 21, 2021).
Ehsani, N., and Afshar, A. (2011). “Application of NA-ACO in multi objective contaminant sensor network design for water distribution systems,” in Water distribution systems analysis 2010 (American Society of Civil Engineers), 237–337. doi:10.1061/41203(425)31
Ehsani, N., Fekete, B. M., Vörösmarty, C. J., and Tessler, Z. D. (2015). A neural network based general reservoir operation scheme. Stoch. Environ. Res. Risk Assess. 30 (4), 1151–1166. doi:10.1007/s00477-015-1147-9
EIA (U.S. Energy Information Administration) (2022). Eia - annual energy outlook 2022. Available at: https://www.eia.gov/outlooks/aeo/.
EPA (2015). EPA science matters newsletter: From gray to green - helping communities adopt green infrastructure. Environmental Protection Agency. Available at: https://www.epa.gov/water-research/epa-science-matters-newsletter-gray-green-helping-communities-adopt-green.
Erlingis, J. M., Gourley, J. J., and Basara, J. B. (2019). Diagnosing moisture sources for flash floods in the United States. Part I: Kinematic trajectories. J. Hydromet. 20 (8), 1495–1509. doi:10.1175/jhm-d-18-0119.1
European Commission (2016). Background on green infrastructure. Available at: http://ec.europa.eu/environment/nature/ecosystems/background.htm.
FAO (2012). The State of Food Insecurity in the World 2012: Economic growth is necessary but not sufficient to accelerate reduction of hunger and malnutrition. Rome: Food and Agriculture Organization of the United Nations. Available at: http://fao.org/docrep/016/i3027e/i3027e.pdf.
Fekete, B. M., Cak, A. D., and Vörösmarty, C. J. (2022). The potential of hydropower to mitigate the intermittency of other renewable energy sources: Analysis for the US Northeast and Midwest. Front. Environ. Sci. (Special Issue FEWS).
Fekete, B. M., Vörösmarty, C. J., and Grabs, W. (2002). High-resolution fields of global runoff combining observed river discharge and simulated water balances. Glob. Biogeochem. Cycles 16 (3), 15-1–15-10. doi:10.1029/1999gb001254
Fekete, B. M., Wisser, D., Kroeze, C., Mayorga, E., Bouwman, L., Wollheim, W. M., et al. (2010). Millennium Ecosystem Assessment scenario drivers (1970-2050): Climate and hydrological alterations. Glob. Biogeochem. Cycles 24 (4). doi:10.1029/2009gb003593
Felzer, B., Kicklighter, D., Melillo, J., Wang, C., Zhuang, Q., and Prinn, R. (2004). Effects of ozone on net primary production and carbon sequestration in the conterminous United States using a biogeochemistry model. Tellus B 56 (3), 230–248. doi:10.1111/j.1600-0889.2004.00097.x
Felzer, B., Reilly, J., Melillo, J., Kicklighter, D., Sarofim, M., Wang, C., et al. (2005). Future effects of ozone on carbon sequestration and climate change policy using a global biogeochemical model. Clim. Change 73 (3), 345–373. doi:10.1007/s10584-005-6776-4
Felzer, B. S., Cronin, T. W., Melillo, J. M., Kicklighter, D. W., and Schlosser, C. A. (2009). Importance of carbon-nitrogen interactions and ozone on ecosystem hydrology during the 21st century. J. Geophys. Res. 114 (G1), G01020. doi:10.1029/2008jg000826
Fisher, J. T., Ciuha, U., Ioannou, L. G., Simpson, L. L., Possnig, C., Lawley, J., et al. (2022). Cardiovascular responses to orthostasis during a simulated 3-day heatwave. Sci. Rep. 12 (1), 19998–20012. doi:10.1038/s41598-022-24216-3
Foster, I. (2011). Globus online: Accelerating and democratizing science through cloud-based services. IEEE Int. Comp. 15 (3), 70–73. doi:10.1109/mic.2011.64
Gahlot, S., Lin, T.-S., Jain, A. K., Baidya Roy, S., Sehgal, V. K., and Dhakar, R. (2020). Impact of environmental changes and land management practices on wheat production in India. Earth Syst. Dyn. 11 (3), 641–652. doi:10.5194/esd-11-641-2020
George, M. W., Hotchkiss, R. H., and Huffaker, R. (2017). Reservoir sustainability and sediment management. J. Water Resour. Plann. Manag. 143 (3), 04016077. doi:10.1061/(asce)wr.1943-5452.0000720
GeoServer.org (2022). Available at: https://geoserver.org/ (Accessed Sep, 2022).
Giampietro, M., Aspinall, R. J., Bukkens, S. G. F., Benalcazar, J. C., Diaz-Maurin, F., Flammini, A., et al. (2013). An innovative accounting framework for the food-energy-water nexus: Application of the MuSIASEM approach to three case studies. Environment and Natural Resources Management Working Paper 56. Rome: FAO.
Green, P. A., Vörösmarty, C. J., Harrison, I., Farrell, T., Sáenz, L., and Fekete, B. M. (2015). Freshwater ecosystem services supporting humans: Pivoting from water crisis to water solutions. Glob. Environ. Change 34, 108–118. doi:10.1016/j.gloenvcha.2015.06.007
Guttman, N. B. (1999). Accepting the standardized precipitation index: A calculation algorithm. JAWRA 35 (2), 311–322. doi:10.1111/j.1752-1688.1999.tb03592.x
He, H., Liang, X.-Z., Lei, H., and Wuebbles, D. J. (2016). Future U.S. ozone projections dependence on regional emissions, climate change, long-range transport and differences in modeling design. Atmos. Environ. 128, 124–133. doi:10.1016/j.atmosenv.2015.12.064
He, H., Liang, X.-Z., and Wuebbles, D. J. (2018). Effects of emissions change, climate change and long-range transport on regional modeling of future U.S. particulate matter pollution and speciation. Atmos. Environ. 179, 166–176. doi:10.1016/j.atmosenv.2018.02.020
Heath, G. A., Hsu, D. D., Inman, D., Aden, A., and Mann, M. K. (2009). “Life cycle assessment of the energy independence and security Act of 2007: Ethanol - global warming potential and environmental emissions,” in American society of mechanical engineers (ASME) third international conference on sustainability (Golden, CO: National Renewable Energy Laboratory).
Hegerl, G. C., Karl, T. R., Allen, M., Bindoff, N. L., Gillett, N., Karoly, D., et al. (2006). Climate change detection and attribution: Beyond mean temperature signals. J. Clim. 19 (20), 5058–5077. doi:10.1175/jcli3900.1
Hegerl, G. C., Zwiers, F. W., Braconnot, P., Gillett, N. P., Luo, Y., Marengo Orsini, J. A., et al. (2007). “Chapter 9: Understanding and attributing climate change,” in Climate change 2007: The physical science basis. Contribution of working group I to the fourth assessment report of the intergovernmental panel on climate change. Editors S. Solomon, D. Qin, M. Manning, Z. Chen, M. Marquis, K. B. Averytet al. (Cambridge, UK; New York, NY, US: Cambridge University Press).
Hegglin, M. I., Kinnison, D., Lamarque, J.-F., and Al, E. (2022). CCMI nitrogen deposition database (1850-2100) in support of CMIP6. Geosci. Model. Dev. [in preparation].
Hirsch, R. M., Moyer, D. L., and Archfield, S. A. (2010). Weighted regressions on time, discharge, and season (WRTDS), with an application to Chesapeake Bay river inputs. JAWRA 46 (5), 857–880. doi:10.1111/j.1752-1688.2010.00482.x
Hoff, H. (2011). “Understanding the nexus,” in Background paper for the bonn 2011 conference: The water, energy and food security nexus (Stockholm, Sweden: Stockholm Environment Institute SEI).
Hoos, A. B., Moore, R. B., Garcia, A. M., Noe, G. B., Terziotti, S. E., Johnston, C. M., et al. (2013). “Simulating stream transport of nutrients in the eastern United States, 2002, using a spatially-referenced regression model and 1:100,000-scale hydrography,” in National water-quality assessment Program (Reston, VA: U.S. Geological Survey).
Howells, M., Hermann, S., Welsch, M., Bazilian, M., Segerström, R., Alfstad, T., et al. (2013). Integrated analysis of climate change, land-use, energy and water strategies. Nat. Clim. Change 3 (7), 621–626. doi:10.1038/nclimate1789
Hu, W. (2018). A billion-dollar investment in New York’s water. The New York Times. 18 Jan. Available at: https://www.nytimes.com/2018/01/18/nyregion/new-york-city-water-filtration.html.
Hungate, B. A., Barbier, E. B., Ando, A. W., Marks, S. P., Reich, P. B., van Gestel, N., et al. (2017). The economic value of grassland species for carbon storage. Sci. Adv. 3 (4), e1601880. doi:10.1126/sciadv.1601880
IPBES (2018). Summary for policymakers of the regional assessment report on biodiversity an ecosystem services for the americas of the intergovernmental science- policy platform on biodiversity and ecosystem services. Editors Rice, J., Seixas, C. S., Zaccagnini, M. E., Bedoya-Gaita´ n, M., Valderrama, N., Anderson, C. B., et al. (Bonn, Germany: IPBES Secretariat).
IPCC (2021). Climate change 2021: The physical science basis. Contribution of working group I to the sixth assessment report of the intergovernmental panel on climate change. Cambridge, England: Cambridge University Press.
Kew, S. F., Philip, S. Y., Jan van Oldenborgh, G., van der Schrier, G., Otto, F. E., and Vautard, R. (2019). The exceptional summer heat wave in southern Europe 2017. Bull. Am. Meteorological Soc. 100 (1), S49–S53. doi:10.1175/bams-d-18-0109.1
Khajehei, S., Ahmadalipour, A., Shao, W., and Moradkhani, H. (2020). A place-based assessment of flash flood hazard and vulnerability in the contiguous United States. Sci. Rep. 10 (1), 448–512. doi:10.1038/s41598-019-57349-z
Kicklighter, D. W., Cai, Y., Zhuang, Q., Parfenova, E. I., Paltsev, S., Sokolov, A. P., et al. (2014). Potential influence of climate-induced vegetation shifts on future land use and associated land carbon fluxes in Northern Eurasia. Environ. Res. Lett. 9 (3), 035004. doi:10.1088/1748-9326/9/3/035004
Kicklighter, D. W., Lin, T.-S., Zhang, J., Chen, M., Vörösmarty, C. J., Jain, A., et al. (2022). Influence of forest infrastructure on the responses of ecosystem services to climate extremes in the Midwest and Northeast United States from 1980 to 2019. Front. Environ. Sci. (Special Issue on FEWS).
Kling, C. L., Arritt, R. W., Calhoun, G., and Keiser, D. A. (2017). Integrated assessment models of the food, energy, and water nexus: A review and an outline of research needs. Ann. Rev. Resour. Econ. 9 (1), 143–163. doi:10.1146/annurev-resource-100516-033533
Kotamarthi, R. L., Mearns, K., Hayhoe, C., Castro, L., and Wuebbles, D. (2016). Use of climate information for decision-making and impacts research: State of our understanding. Department of Defense, Strategic Environmental Research and Development Program, 55.
Kraft, M. E., and Vig, N. J. (2006). “Environmental policy from the 1970s to the twenty-first century,” in Environmental policy: New directions for the twenty-first century (Washington, DC: CQ Press), 1–33.
Lawford, R. G. (2019). A design for a data and information service to address the knowledge needs of the water-energy-food (W-E-F) nexus and strategies to facilitate its implementation. Front. Environ. Sci. 7, 56. doi:10.3389/fenvs.2019.00056
Leck, H., Conway, D., Bradshaw, M., and Rees, J. (2015). Tracing the water-energy-food nexus: Description, theory and practice. Geogr. Compass 9 (8), 445–460. doi:10.1111/gec3.12222
Lehner, B., Verdin, K., and Jarvis, A. (2008). New global hydrography derived from spaceborne elevation data. Eos, Trans. AGU 89 (10), 93–94. doi:10.1029/2008eo100001
Lei, H., Wuebbles, D. J., Liang, X.-Z., and Olsen, S. (2013). Domestic versus international contributions on 2050 ozone air quality: How much is convertible by regional control? Atmos. Environ. 68, 315–325. doi:10.1016/j.atmosenv.2012.12.002
Letourneau, D. K., Ando, A. W., Jedlicka, J. A., Narwani, A., and Barbier, E. (2015). Simple-but-sound methods for estimating the value of changes in biodiversity for biological pest control in agriculture. Ecol. Econ. 120, 215–225. doi:10.1016/j.ecolecon.2015.10.015
Li, Y., Guan, K., Schnitkey, G. D., DeLucia, E., and Peng, B. (2019). Excessive rainfall leads to maize yield loss of a comparable magnitude to extreme drought in the United States. Glob. Change Biol. 25 (7), 2325–2337. doi:10.1111/gcb.14628
Lin, J.-T., Patten, K. O., Hayhoe, K., Liang, X.-Z., and Wuebbles, D. J. (2008). Effects of future climate and biogenic emissions changes on surface ozone over the United States and China. J. Appl. Meteorol. Clim. 47 (7), 1888–1909. doi:10.1175/2007jamc1681.1
Lin, T.-S., Jain, A., Kheshgi, H. S., Song, Y., and Vörösmarty, C. J. (2022). which crop has the highest bioethanol yield and water use efficiency in the United States of America?. Front. Environ. Sci. (Special Issue on FEWS).
Lin, T.-S., Song, Y., Lawrence, P., Kheshgi, H. S., and Jain, A. K. (2021). Worldwide maize and soybean yield response to environmental and management factors over the 20th and 21st centuries. J. Geophys. Res. Biogeosci. 126 (11), 6304. doi:10.1029/2021jg006304
Lu, X., Kicklighter, D. W., Melillo, J. M., Reilly, J. M., and Xu, L. (2015). Land carbon sequestration within the conterminous United States: Regional- and state-level analyses. J. Geophys. Res. Biogeo. 120 (2), 379–398. doi:10.1002/2014jg002818
Lu, X., Kicklighter, D. W., Melillo, J. M., Yang, P., Rosenzweig, B., Vörösmarty, C. J., et al. (2013). A contemporary carbon balance for the Northeast Region of the United States. Environ. Sci. Tech. 47 (23), 13230–13238. doi:10.1021/es403097z
Luo, Y., Melillo, J., Niu, S., Beier, C., Clark, J. S., Classen, A. T., et al. (2011). Coordinated approaches to quantify long-term ecosystem dynamics in response to global change. Glob. Change Biol. 17 (2), 843–854. doi:10.1111/j.1365-2486.2010.02265.x
Martinich, J., and Crimmins, A. (2019). Climate damages and adaptation potential across diverse sectors of the United States. Nat. Clim. Change 9 (5), 397–404. doi:10.1038/s41558-019-0444-6
Maxfield, N. E., Smith, R. A., Chang, J., Ando, A. W., Lin, T.-S., and Vörösmarty, C. J. (2022). Modeling the impact of technology, management, and policy-related strategies on CONUS water quality and ecosystem services. Front. Environ. Sci. (Special Issue FEWS).
Maxfield, N. E., Smith, R. A., Sanyal, S., and Vorosmarty, C. J., and Jhih-Shyang Shih (2021). Investigating the impact of climate extremes on nitrogen flux in CONUS watersheds [POSTER]. LA, December: AGU. New Orleans.
McDonald, R. I., Weber, K. F., Padowski, J., Boucher, T., and Shemie, D. (2016). Estimating watershed degradation over the last century and its impact on water-treatment costs for the world’s large cities. P. Natl. Acad. Sci. 113 (32), 9117–9122. doi:10.1073/pnas.1605354113
McGrane, S. J., Acuto, M., Artioli, F., Chen, P., Comber, R., Cottee, J., et al. (2018). Scaling the nexus: Towards integrated frameworks for analysing water, energy and food. Geogr. J. 185 (4), 419–431. doi:10.1111/geoj.12256
McGuire, A. D., Sitch, S., Clein, J. S., Dargaville, R., Esser, G., Foley, J., et al. (2001). Carbon balance of the terrestrial biosphere in the Twentieth Century: Analyses of CO2, climate and land use effects with four process-based ecosystem models. Glob. Biogeochem. Cycles 15 (1), 183–206. doi:10.1029/2000gb001298
McKinsey, and Company (2006). Charting our water future: Economic frameworks to inform decision-making. The 2030 Water Resources Group. McKinsey, Inc., 198.
Meinshausen, M., Smith, S. J., Calvin, K., Daniel, J. S., Kainuma, M. L. T., Lamarque, J-F., et al. (2011). The RCP greenhouse gas concentrations and their extensions from 1765 to 2300. Clim. Change 109 (1-2), 213–241. doi:10.1007/s10584-011-0156-z
Meiyappan, P., and Jain, A. K. (2012). Three distinct global estimates of historical land-cover change and land-use conversions for over 200 years. Front. Earth Sci. 6 (2), 122–139. doi:10.1007/s11707-012-0314-2
Melillo, J. M., Butler, S., Johnson, J., Mohan, J., Steudler, P., Lux, H., et al. (2011). Soil warming, carbon-nitrogen interactions, and forest carbon budgets. P. Natl. Acad. Sci. 108 (23), 9508–9512. doi:10.1073/pnas.1018189108
Melillo, J. M., Lu, X., Kicklighter, D. W., Reilly, J. M., Cai, Y., and Sokolov, A. P. (2016). Protected areas’ role in climate-change mitigation. Ambio 45 (2), 133–145. doi:10.1007/s13280-015-0693-1
Melillo, J. M., Reilly, J. M., Kicklighter, D. W., Gurgel, A. C., Cronin, T. W., Paltsev, S., et al. (2009). Indirect emissions from biofuels: How important? Science 326 (5958), 1397–1399. doi:10.1126/science.1180251
Mesinger, F., DiMego, G., Kalnay, E., Mitchell, K., Shafran, P. C., Ebisuzaki, W., et al. (2006). North American regional reanalysis. B. Am. Meteorol. Soc. 87 (3), 343–360. doi:10.1175/bams-87-3-343
Miara, A., Macknick, J. E., Vörösmarty, C. J., Tidwell, V., Newmark, R., and Fekete, B. (2017). Climate and water resource change impacts and adaptation potential for U.S. power supply. Nat. Clim. Change 7, 793–798. doi:10.1038/NCLIMATE3417
Miara, A., and Vörösmarty, C. J. (2013a). A dynamic model to assess tradeoffs in power production and riverine ecosystem protection. Environ. Sci. Process. Impacts 15 (6), 1113. doi:10.1039/c3em00196b
Miara, A., and Vörösmarty, C. J. (2013b). “Balancing thermoelectric power production and thermal pollution: Power plant cooling specification/decision-making guide,” in Thermal power plant cooling: Context and engineering. Editor C. King (New York, NY: ASME Press), 22–27.
Miara, A., Vörösmarty, C. J., Stewart, R. J., Wollheim, W. M., and Rosenzweig, B. (2013). Riverine ecosystem services and the thermoelectric sector: Strategic issues facing the northeastern United States. Environ. Res. Lett. 8 (2), 025017. doi:10.1088/1748-9326/8/2/025017
Monier, E., Scott, J. R., Sokolov, A. P., Forest, C. E., and Schlosser, C. A. (2013). An integrated assessment modeling framework for uncertainty studies in global and regional climate change: The MIT IGSM-CAM (version 1.0). Geosci. Model. Dev. 6 (6), 2063–2085. doi:10.5194/gmd-6-2063-2013
Moore, R. B., Johnston, C. M., Robinson, K. W., and Deacon, J. R. (2004). Estimation of total nitrogen and phosphorus in new england streams using spatially referenced regression models. Pembroke, NH: U.S. Geological Survey.
National Academies of Sciences, Engineering, and Medicine (2022). An approach for assessing U.S. Gulf coast ecosystem restoration: A gulf research Program environmental monitoring report. Washington, D.C.: National Academies Press. doi:10.17226/26335
National Atmospheric Deposition Program (2022). NADP Program office. Madison, WI 53706: Wisconsin State Laboratory of Hygiene, 465 Henry Mall.
Newmark, R. L., Macknick, J., Heath, G., Ong, S., Denholm, P., Margolis, R., et al. (2012). “Implications of future energy choices in the energy-water-land nexus,” in WREF Forum: Energy-water nexus: An international perspective.
NextCloud.com (2022). Available at: https://nextcloud.com/ (Accessed Sep, 2022).
Niyogi, D., Liu, X., Andresen, J., Song, Y., Jain, A. K., Kellner, O., et al. (2015). Crop models capture the impacts of climate variability on corn yield. Geophys. Res. Lett. 42 (9), 3356–3363. doi:10.1002/2015gl063841
NLDAS (2022). North American land data assimilation system (NLDAS). https://ldas.gsfc.nasa.gov/nldas/nldas-get-data (Accessed 10 13.2022).
NREL (2014). Making sustainable energy choices: Insights on the energy/water/land nexus. Golden, CO: National Renewable Energy Laboratory NREL.
NY Times, (2022). How bad is the western drought? Worst in 12 centuries, study finds. Available at: https://www.nytimes.com/2022/02/14/climate/western-drought-megadrought.html.
Preston, S. D., Alexander, R. B., and Wolock, D. M. (2011). Sparrow modeling to understand water-quality conditions in major regions of the United States: A featured collection introduction. JAWRA 47 (5), 887–890. doi:10.1111/j.1752-1688.2011.00585.x
Randle, T. J., Morris, G. L., Tullos, D. D., Weirich, F. H., Kondolf, G. M., Moriasi, D. N., et al. (2021). Sustaining United States reservoir storage capacity: Need for a new paradigm. J. Hydrol. 602, 126686. doi:10.1016/j.jhydrol.2021.126686
Raymond, P. A., Oh, N.-H., Turner, R. E., and Broussard, W. (2008). Anthropogenically enhanced fluxes of water and carbon from the Mississippi River. Nature 451 (7177), 449–452. doi:10.1038/nature06505
Reilly, J., Melillo, J., Cai, Y., Kicklighter, D., Gurgel, A., Paltsev, S., et al. (2012). Using land to mitigate climate change: Hitting the target, recognizing the trade-offs. Environ. Sci. Tech. 46 (11), 5672–5679. doi:10.1021/es2034729
Riahi, K., van Vuuren, D. P., Kriegler, E., Edmonds, J., O’Neill, B. C., Fujimori, S., et al. (2017). The Shared Socioeconomic Pathways and their energy, land use, and greenhouse gas emissions implications: An overview. Glob. Environ. Change 42, 153–168. doi:10.1016/j.gloenvcha.2016.05.009
Richardson, L., Loomis, J., Kroeger, T., and Casey, F. (2015). The role of benefit transfer in ecosystem service valuation. Ecol. Econ. 115, 51–58. doi:10.1016/j.ecolecon.2014.02.018
Robertson, D. M., Schwarz, G. E., Saad, D. A., and Alexander, R. B. (2009). Incorporating uncertainty into the ranking of SPARROW model nutrient yields from Mississippi/Atchafalaya River basin watersheds. JAWRA 45 (2), 534–549. doi:10.1111/j.1752-1688.2009.00310.x
Rosenzweig, B., Vörösmarty, C., Gutowski, W., and Steiner, A. L. (2014). Joining scientists and stakeholders in regional Earth system modeling. Eos, Trans. AGU 95 (27), 247–248. doi:10.1002/2014eo270005
Rothfusz, L. P., and NWS Southern Region Headquarters (1990). The heat index equation (or, more than you ever wanted to know about heat index). Fort Worth, TexasOffice of Meteorology: National Oceanic and Atmospheric Administration, National Weather Service.
Röttgers, D., Tandon, A., and Kaminker, C. (2018). OECD progress update on approaches to mobilising institutional investment for sustainable infrastructure. OECD Environment Working Papers. Paris: OECD Publishing. No. 138. doi:10.1787/45426991-en
Rychtecka, M., Vörösmarty, C. J., and Fekete, B. M. (2010). “Spatio-temporal impact of wastewater point sources on nitrogen pollution in U.S. river systems,” in American society of limnology and oceanography (ASLO)/North American benthological society (NABS) joint summer meeting.
Saad, D. A., Schwarz, G. E., Argue, D. M., Anning, D. W., Ator, S. W., Hoos, A. B., et al. (1991). Estimates of long-term mean daily streamflow and annual nutrient and suspended sediment loads considered for use in regional SPARROW models of the conterminous United States, 2012 base year. Washington, DC: U.S. Geological Survey Scientific Investigations. Report 2019-5069.
Saad, D. A., Schwarz, G. E., Robertson, D. M., and Booth, N. L. (2011). A multi-agency nutrient dataset used to estimate loads, improve monitoring design, and calibrate regional nutrient SPARROW models. JAWRA 47 (5), 933–949. doi:10.1111/j.1752-1688.2011.00575.x
Sadegh, M., AghaKouchak, A., Mallakpour, I., Huning, L. S., Mazdiyasni, O., Niknejad, M., et al. (2020). Data and analysis toolbox for modeling the nexus of food, energy, and water. Sustain. Cities Soc. 61, 102281. doi:10.1016/j.scs.2020.102281
Saleh, D., and Domagalski, J. (2015). SPARROW modeling of nitrogen sources and transport in rivers and streams of California and adjacent states, U.S. JAWRA 51 (6), 1487–1507. doi:10.1111/1752-1688.12325
Sanders, N., and Barreca, A. I. (2022). Adaptation to environmental change: Agriculture and the unexpected incidence of the acid rain Program. Am. Econ. J.- Econ. Pol. 14 (1), 373–341. doi:10.2139/ssrn.3809563
Santer, B. D., Taylor, K. E., Gleckler, P. J., Bonfils, C., Barnett, T. P., Pierce, D. W., et al. (2009). Incorporating model quality information in climate change detection and attribution studies. P. Natl. Acad. Sci. 106 (35), 14778–14783. doi:10.1073/pnas.0901736106
Sanyal, S., and Wuebbles, D. (2022a). Changing nature of the extremes: 1. Droughts and heat eaves in CONUS. Climate (Special Issue on Extreme Precipitation in a Changing Climate). [in preparation].
Sanyal, S., and Wuebbles, D. (2022b). Changing nature of the extremes: Extreme precipitation, cold waves and compound extremes in CONUS. Climate (Special Issue on Extreme Precipitation in a Changing Climate). [in preparation].
Secchi, S., and McDonald, M. (2019). The state of water quality strategies in the Mississippi River Basin: Is cooperative federalism working? Sci. Total Environ. 677, 241–249. doi:10.1016/j.scitotenv.2019.04.381
Sha, Z., Bai, Y., Li, R., Lan, H., Zhang, X., Li, J., et al. (2022). The global carbon sink potential of terrestrial vegetation can be increased substantially by optimal land management. Comm. Earth Environ. 3 (1), 8. doi:10.1038/s43247-021-00333-1
Shih, J.-S., Alexander, R. B., Smith, R. A., Boyer, E. W., Shwarz, G. E., and Chung, S. (2010). An initial SPARROW model of land use and in-stream controls on total organic carbon in streams of the conterminous United States. Washington, DC: U.S. Geological Survey.
Shih, J.-S., Driscoll, C. T., Burtraw, D., Shen, H., Smith, R. A., Keyes, A., et al. (2022). Energy policy and coastal water quality: An integrated energy, air and water quality modeling approach. Sci. Total Environ. 816, 151593. doi:10.1016/j.scitotenv.2021.151593
Shikhmacheva, K. (2017). The northeastern regional Earth system model (NE-RESM) inter-basin water transfer (IBWT) database. New York, NY New York: The City College of.
Shu, S., Jain, A. K., Koven, C. D., and Mishra, U. (2020). Estimation of permafrost SOC stock and turnover time using a land surface model with vertical heterogeneity of permafrost soils. Glob. Biogeochem. Cycles 34 (11). doi:10.1029/2020gb006585
Simpson, G. B., and Jewitt, G. P. W. (2019). The development of the water-energy-food nexus as a framework for achieving resource security: A review. Front. Environ. Sci. 7. doi:10.3389/fenvs.2019.00008
Skinner, K. D., and Maupin, M. A. (2019). Point-source nutrient loads to streams of the conterminous United States, 2012. U.S. Geol. Surv. Data Ser. 1101, 13. doi:10.3133/ds1101
Smith, R. A., Schwarz, G. E., Brakebill, J. W., Hoos, A., Moore, R. B., Nolin, A. W., et al. (2014). Use of MODIS data in dynamic SPARROW analysis of watershed loading reductions. Abstract Retrieved from AGU Fall Meeting 2014 (Abstract ID H33P-08).
Song, Y., Cervarich, M., Jain, A. K., Kheshgi, H. S., Landuyt, W., and Cai, X. (2016). The interplay between bioenergy grass production and water resources in the United States of America. Environ. Sci. Tech. 50 (6), 3010–3019. doi:10.1021/acs.est.5b05239
Song, Y., Jain, A. K., Landuyt, W., Kheshgi, H. S., and Khanna, M. (2014). Estimates of biomass yield for perennial bioenergy grasses in the USA. BioEnergy Res. 8 (2), 688–715. doi:10.1007/s12155-014-9546-1
Song, Y., Jain, A. K., and McIsaac, G. F. (2013). Implementation of dynamic crop growth processes into a land surface model: Evaluation of energy, water and carbon fluxes under corn and soybean rotation. Biogeosciences 10 (12), 8039–8066. doi:10.5194/bg-10-8039-2013
Stein, U., and Alpert, P. (1993). Factor separation in numerical simulations. J. Atmos. Sci. 50 (14), 2107–2115. doi:10.1175/1520-0469(1993)050<2107:fsins>2.0.co;2
Stewart, R. J., Wollheim, W. M., Miara, A., Vörösmarty, C. J., Fekete, B., Lammers, R. B., et al. (2013). Horizontal cooling towers: Riverine ecosystem services and the fate of thermoelectric heat in the contemporary Northeast US. Environ. Res. Lett. 8 (2), 025010. doi:10.1088/1748-9326/8/2/025010
Super Law Group (2013). “Comments on EPA biological evaluation,” in Letter to the national marine fisheries service, U.S. Fish and wildlife service (U.S. Environmental Protection Agency).
Teitelbaum, Y., Yakirevich, A., Gross, A., and Sorek, S. (2020). Simulations of the water food energy nexus for policy driven intervention. Heliyon 6 (8), e04767. doi:10.1016/j.heliyon.2020.e04767
Tian, H., Melillo, J., Lu, C., Kicklighter, D., Liu, M., Ren, W., et al. (2011). China’s terrestrial carbon balance: Contributions from multiple global change factors. Glob. Biogeochem. Cycles 25 (1), 1–16. doi:10.1029/2010gb003838
Tian, H., Melillo, J. M., Kicklighter, D. W., Pan, S., Liu, J., McGuire, A. D., et al. (2003). Regional carbon dynamics in monsoon Asia and its implications for the global carbon cycle. Glob. Planet. Change 37, 201–217. doi:10.1016/s0921-8181(02)00205-9
Tuler, S. P., Webler, T., Hansen, R., Vorosmarty, C. J., Melillo, J. M., Wuebbles, D., et al. (2022). Prospects and challenges of regional modeling frameworks to inform planning for food, energy, and water systems: Views of modelers and stakeholders. Front. Environ. Sci (Special Issue FEWS).
UN General Assembly (2015). Transforming our world: The 2030 Agenda for sustainable development. A/RES/70/1. Available at: https://www.refworld.org/docid/57b6e3e44.html (Accessed Sep 23, 2022).
Un-Habitat, , and WHO, (2021). Progress on wastewater treatment: Global status and acceleration needs for SDG indicator 6.3.1. Geneva: United Nations Human Settlements Programme UN-Habitat and World Health Organization WHO.
UNEP-IUCN United Nations Environment Programme and International Union for Conservation of Nature, (2021). Nature-based solutions for climate change mitigation. Nairobi and Gland.
USACE EWN (2020). Army corps of engineers, engineering with nature initiative. Available at: https://ewn.el.erdc.dren.mil/.
USDA (2012). U.S. Drought 2012: Farm and food impacts. United States Department of Agriculture, Economic Research Service. Available at: http://www.ers.usda.gov/topics/in-the-news/us-drought-2012-farm-and-food-impacts.
U.S. EPA (2016). Report on point source progress in hypoxia task force states. Washington, DC: U.S. Environmental Protection Agency. Available at: https://www.epa.gov/sites/default/files/2016-03/documents/htf_pointsource_progressreport_02-25-16_508.pdf.
U.S. EPA (2017). Updates to the demographic and spatial allocation models to produce integrated climate and land use scenarios (ICLUS) (final report, version 2). Washington, DC: U.S. Environmental Protection Agency.
USGCRP (2017). Climate science special report: Fourth national climate assessment, Vol. 1. Washington, DC: U.S. Global Change Research Program, 447pp.
USGCRP (2018). Impacts, risks, and adaptation in the United States: The fourth national climate assessment, volume II. Washington, DC: U.S. Global Change Research Program, 1515. doi:10.7930/nca4.2018
USGS (2016). HydroSHEDS. U.S. Geological survey. Available at: http://hydrosheds.cr.usgs.gov/index.php.
van Beek, L., Hajer, M., Pelzer, P., van Vuuren, D., and Cassen, C. (2020). Anticipating futures through models: The rise of integrated assessment modelling in the climate science-policy interface since 1970. Glob. Environ. Change 65, 102191. doi:10.1016/j.gloenvcha.2020.102191
van Vliet, M. T. H., Yearsley, J. R., Ludwig, F., Vögele, S., Lettenmaier, D. P., and Kabat, P. (2012). Vulnerability of US and European electricity supply to climate change. Nat. Clim. Change 2 (9), 676–681. doi:10.1038/nclimate1546
Vörösmarty, C. J., Federer, C. A., and Schloss, A. L. (1998). Potential evaporation functions compared on US watersheds: Possible implications for global-scale water balance and terrestrial ecosystem modeling. J. Hydrol. 207 (3-4), 147–169. doi:10.1016/s0022-1694(98)00109-7
Vörösmarty, C. J., Melillo, J., Wuebbles, D. J., Jain, A. K., Ando, A., Tuler, S., et al. (2022). Applying a framework to study climate-induced extremes on food, energy, and water Systems (C-FEWS): The role of engineered and natural infrastructures, technology, and environmental management in the U.S. Midwest and Northeast. Front. Environ. Sci. (Special Issue FEWS).
Vörösmarty, C. J., Moore, B., Grace, A. L., Gildea, M. P., Melillo, J. M., Peterson, B. J., et al. (1989). Continental scale models of water balance and fluvial transport: An application to South America. Glob. Biogeochem. Cycles 3 (3), 241–265. doi:10.1029/gb003i003p00241
Vörösmarty, C. J., Rodríguez-Osun, V., Cak, A. D., Badhuri, A., Bunn, S. E., Corsi, F., et al. (2018). Ecosystem-based water security and the sustainable development goals (SDGs). Ecohydrol. Hydrobiology 18 (4), 317–333. doi:10.1016/j.ecohyd.2018.07.004
Vörösmarty, C. J., Stewart-Koster, B., Green, P. A., Boone, E. L., Flörke, M., Fischer, G., et al. (2021). A green-gray path to global water security and sustainable infrastructure. Glob. Environ. Change 70, 102344. doi:10.1016/j.gloenvcha.2021.102344
Warner, E., Inman, D., Kunstman, B., Bush, B., Vimmerstedt, L., Peterson, S., et al. (2013). Modeling biofuel expansion effects on land use change dynamics. Environ. Res. Lett. 8 (1), 015003. doi:10.1088/1748-9326/8/1/015003
Weaver, C. P., Lempert, R. J., Brown, C., Hall, J. A., Revell, D., and Sarewitz, D. (2012). Improving the contribution of climate model information to decision making: The value and demands of robust decision frameworks. Wiley Interdiscip. Rev. Clim. Change 4 (1), 39–60. doi:10.1002/wcc.202
Weaver, C. P., Liang, X.-Z. ., Zhu, J., Adams, P. J., Amar, P., Avise, J., et al. (2009). A preliminary synthesis of modeled climate change impacts on U.S. regional ozone concentrations. B. Am. Meteorol. Soc. 90 (12), 1843–1864. doi:10.1175/2009bams2568.1
Weiskopf, S. R., Rubenstein, M. A., Crozier, L. G., Gaichas, S., Griffis, R., Halofsky, J. E., et al. (2020). Climate change effects on biodiversity, ecosystems, ecosystem services, and natural resource management in the United States. Sci. Total Environ. 733, 137782. doi:10.1016/j.scitotenv.2020.137782
Wilhite, D. A., Sivakumar, M. V. K., and Pulwarty, R. (2014). Managing drought risk in a changing climate: The role of national drought policy. Weather Clim. Extrem 3, 4–13. doi:10.1016/j.wace.2014.01.002
Willner, S. N., Otto, C., and Levermann, A. (2018). Global economic response to river floods. Nat. Clim. Change 8 (7), 594–598. doi:10.1038/s41558-018-0173-2
Wisser, D., Fekete, B. M., Vörösmarty, C. J., and Schumann, A. H. (2010a). Reconstructing 20th century global hydrography: A contribution to the global terrestrial network- hydrology (GTN-H). Hydrol. Earth Syst. Sci. 14 (1), 1–24. doi:10.5194/hess-14-1-2010
Wisser, D., Frolking, S., Douglas, E. M., Fekete, B. M., Schumann, A. H., and Vörösmarty, C. J. (2010b). The significance of local water resources captured in small reservoirs for crop production – a global-scale analysis. J. Hydrol. 384 (3-4), 264–275. doi:10.1016/j.jhydrol.2009.07.032
Wisser, D., Frolking, S., Douglas, E. M., Fekete, B. M., Vörösmarty, C. J., and Schumann, A. H. (2008). Global irrigation water demand: Variability and uncertainties arising from agricultural and climate data sets. Geophys. Res. Lett. 35 (24), L24408. doi:10.1029/2008gl035296
Wolfe, M. L., Ting, K. C., Scott, N., Sharpley, A., Jones, J. W., and Verma, L. (2016). Engineering solutions for food-energy-water systems: It is more than engineering. J. Environ. Stud. Sci. 6 (1), 172–182. doi:10.1007/s13412-016-0363-z
Wollheim, W. M., Vörösmarty, C. J., Bouwman, A. F., Green, P., Harrison, J. A., Meybeck, M., et al. (2008). Global N removal by freshwater aquatic systems using a spatially distributed, within-basin approach. Glob. Biogeochem. Cycles 22 (GB2026), 22. doi:10.1029/2007gb002963
World Economic Forum (2011). Water security: The water-food-energy-climate nexus. Washington, DC: Island Press.
World Meteorological Organization (2012). Standardized precipitation index user guide. Geneva, Switzerland: World Meteorological Organization.
Wuebbles, D. J. (2018). “Climate change in the 21st century: Looking beyond the paris agreement,” in Climate change and its impacts. Editors C. Murphy, P. Gardoni, and R. McKim (Cham, Switzerland: Springer). doi:10.1007/978-3-319-77544-9_2
Wuebbles, D. J., Kunkel, K., Wehner, M., and Zobel, Z. (2014). Severe weather in United States under a changing climate. Eos, Trans. AGU 95 (18), 149–150. doi:10.1002/2014eo180001
WWAP/UN-Water (2018). The united nations world water development report 2018: Nature-based solutions for water. Paris: UNESCO. United Nations World Water Assessment Programme.
Xia, Y., Mitchell, K., Ek, M., Sheffield, J., Cosgrove, B., Wood, E., et al. (2012b). Continental-scale water and energy flux analysis and validation for north American land data assimilation system project phase 2 (NLDAS-2): 2. Validation of model-simulated streamflow. J. Geophys. Res. Atmos. 117 (D3). doi:10.1029/2011jd016051
Xia, Y., Mitchell, K., Ek, M., Sheffield, J., Cosgrove, B., Wood, E., et al. (2012a). Continental-scale water and energy flux analysis and validation for the North American land data assimilation system project phase 2 (NLDAS-2): 1. Intercomparison and application of model products. J. Geophys. Res. Atmos. 117 (D3), 6048. doi:10.1029/2011jd016048
Xu, X., Sharma, P., Shu, S., Lin, T.-S., Ciais, P., Tubiello, F. N., et al. (2021). Global greenhouse gas emissions from animal-based foods are twice those of plant-based foods. Nat. Food 2 (9), 724–732. doi:10.1038/s43016-021-00358-x
Young, S. C. (2000). The emergence of ecological modernisation: Integrating the environment and the economy? London: Routledge.
Zarfl, C., and Lucía, A. (2018). The connectivity between soil erosion and sediment entrapment in reservoirs. Curr. Opin. Environ. Sci. Health 5, 53–59. doi:10.1016/j.coesh.2018.05.001
Zering, K. D., Centner, T. J., Meyer, D., Newton, G. L., Sweeten, J. M., and Woodruff, S. (2012). Water and land issues associated with animal agriculture: A U. S. Perspective, 50. CAST Issue Paper, 24.
Zhang, J., Chen, M., Gao, S., Li, Z., Vorosmarty, C. J., Fekete, B. M., et al. (2022). Examining the policy, technology, and climate impacts on thermoelectric power production and river thermal pollution in the Midwest and Northeast of the United States. Front. Environ. Sci. (Special Issue FEWS).
Zobel, Z., Wang, J., Wuebbles, D. J., and Kotamarthi, V. R. (2018). Analyses for high-resolution projections through the end of the 21st century for precipitation extremes over the United States. Earth's Future 6 (10), 1471–1490. doi:10.1029/2018ef000956
Keywords: fews, climate extremes, nature-based infrastructure, engineered infrastructure, regional assessment, C-FEWS analysis framework, interdisciplinary climate studies
Citation: Vörösmarty CJ, Melillo JM, Wuebbles DJ, Jain AK, Ando AW, Chen M, Tuler S, Smith R, Kicklighter D, Corsi F, Fekete B, Miara A, Bokhari HH, Chang J, Lin T-S, Maxfield N, Sanyal S, Zhang J and Vignoles D (2023) The C-FEWS framework: Supporting studies of climate-induced extremes on food, energy, and water systems at the regional scale. Front. Environ. Sci. 11:1069613. doi: 10.3389/fenvs.2023.1069613
Received: 14 October 2022; Accepted: 03 January 2023;
Published: 06 February 2023.
Edited by:
Xander Wang, University of Prince Edward Island, CanadaReviewed by:
Ashmita Sengupta, Commonwealth Scientific and Industrial Research Organisation (CSIRO), AustraliaCopyright © 2023 Vörösmarty, Melillo, Wuebbles, Jain, Ando, Chen, Tuler, Smith, Kicklighter, Corsi, Fekete, Miara, Bokhari, Chang, Lin, Maxfield, Sanyal, Zhang and Vignoles. This is an open-access article distributed under the terms of the Creative Commons Attribution License (CC BY). The use, distribution or reproduction in other forums is permitted, provided the original author(s) and the copyright owner(s) are credited and that the original publication in this journal is cited, in accordance with accepted academic practice. No use, distribution or reproduction is permitted which does not comply with these terms.
*Correspondence: Charles J. Vörösmarty, Y3Zvcm9zbWFydHlAZ21haWwuY29t
Disclaimer: All claims expressed in this article are solely those of the authors and do not necessarily represent those of their affiliated organizations, or those of the publisher, the editors and the reviewers. Any product that may be evaluated in this article or claim that may be made by its manufacturer is not guaranteed or endorsed by the publisher.
Research integrity at Frontiers
Learn more about the work of our research integrity team to safeguard the quality of each article we publish.