- 1Department of Civil, Environmental, and Geospatial Engineering, Michigan Technological University, Houghton, MI, United States
- 2Department of Computer Science, Michigan Technological University, Houghton, MI, United States
- 3Key Laboratory of the Three Gorges Reservoir Region’s Eco-Environment, Ministry of Education, Chongqing University, Chongqing, China
- 4Department of Human Ecology, School of Environmental and Biological Sciences, Rutgers University, New Brunswick, NJ, United States
The household is an important locus of decision-making regarding food, energy, and water (FEW) consumption. Changes in household FEW consumption behaviors can lead to significant reductions in environmental impacts, but it can be difficult for consumers to compare the relative impacts of their consumption quantitatively, or to recognize the indirect impacts of their household consumption patterns. We describe two novel tools designed to address this problem: A hybrid life cycle assessment (LCA) framework to translate household consumption of food, energy, and water into key environmental impacts including greenhouse gas emissions, energy use, and water use; and a novel software application called HomeTracker that implements the framework by collecting household FEW data and providing environmental impact feedback to households. We explore the question: How can a life cycle assessment-based software application facilitate collection and translation of household consumption data to meaningful environmental impact metrics? A case study in Lake County, Illinois is presented to illustrate use of the HomeTracker application. Output data describing environmental impacts attributable to household FEW consumption in the study area are shown in order to illustrate key features and trends observed in the case study population. The framework and its associated output data can be used to support experimental research at the household scale, allowing for examination of what users purchase and consume over an extended period of time as well as increased understanding of household behavior trends and environmental impacts, and as future work.
1 Introduction
The interdependencies of food, energy, and water (FEW) resources, known collectively as the FEW nexus, require careful planning and management of each resource to avoid unintended consequences in the other sectors. Pressure on these resources is rising due to global population growth, increases in per capita consumption, changes in dietary preferences to include more animal products, and a changing climate (Flammini et al., 2014; Scanlon et al., 2017). Globally, household consumption accounts for more than 70% of total greenhouse gas (GHG) emissions (Hertwich and Peters 2009; Wilson et al., 2013) and approximately 80% of total freshwater use (Ivanova et al., 2016). In the United States, over 80% of GHG emissions have been attributed to consumption at the household level (Jones and Kammen 2011). Thus, there is an opportunity to reduce water use and greenhouse gas impacts globally and domestically through changes in household consumption behavior, and an understanding of current behavior trends, motivations, and barriers to change can help identify effective interventions.
Everyday household consumption of food, energy, and water has both direct and indirect resource use and environmental impacts that need to be accounted for. For example, the average water footprint of an individual person’s diet varies between approximately 158,500 and 475,000 gallons (600 and 1,800 m3) per year, depending on what type of food is consumed (Hoekstra and Chapagain 2008; D’Odorico et al., 2018). This water footprint includes both “green water” (rainwater used by crops) and “blue water” (water withdrawals for irrigation) (Falkenmark and Rockström 2006). The average GHG emissions from a person’s diet is estimated at 4.7 kg CO2 eq. per day (Heller et al., 2018). Similarly, electricity generation requires significant volumes of freshwater use and emits greenhouse gasses into the atmosphere. Over 40% of United States energy is consumed for household and commercial purposes (Chini et al., 2016). While the industry average water use for electricity generation has been cited as 25 gallons per kWh, the water use intensity of electricity generation varies by orders of magnitude depending on fuel mix, prime mover of generation, cooling technology, and emissions controls (Sovacool and Sovacool 2009; Grubert and Sanders 2018).
Multiple studies have investigated the environmental impacts, including GHG emissions and water use, of dietary choices and maintaining a healthy diet (e.g., Heller and Keoleian 2015; Tom et al., 2016; Hallström et al., 2017). Agricultural activities have negative impacts on the environment through emission of GHGs (Chandio et al., 2020; Chandio et al., 2021; Alavijeh et al., 2022), intensive use of fertilizers and pesticides (e.g., Kross et al., 2022), withdrawal and consumption of freshwater resources (D’Odorico et al., 2018), land use change (e.g., Hunter et al., 2017), and degradation of biodiversity (Yang et al., 2018). The agricultural sector accounts for approximately 70% of global water withdrawals (Marston et al., 2018). In addition, the environmental impact of food consumption at the household level has been quantified and related to sociodemographic characteristics such as race, income, and education level (Boehm et al., 2018). Other studies have related food consumption, and in particular the obesity epidemic, with energy use and environmental degradation (Koengkan and Fuinhas 2021; Koengkan and Fuinhas, 2022). Kanyama et al. (2021) assessed the GHG emission impacts of shifting expenditures on food and holidays, along with furnishings.
Several studies have quantified the environmental impacts of household consumption at global, national, or regional levels. For example, Ivanova et al. (2016) used the EXIOBASE 2.2 multiregional input-output (MRIO) database (Wood et al., 2013) to analyze global supply chains and trace the origin of products consumed by households, quantifying the impacts of consumption in terms of global GHG emissions and total land, material, and water use. Steen-Olsen et al. (2016) combined use of the EXIOBASE 2 MRIO database with a national consumer expenditure survey to assess the GHG footprint of Norwegian household consumption. Kok et al. (2006) compared different methods for quantifying the energy requirements of household consumption, including input-output analysis based on national accounts, input-output analysis using household expenditure data, and a hybrid analysis combining input-output modeling with process modeling. They obtained similar results from the three methods for a case study of households in the Netherlands but noted that the hybrid analysis enables identifying options for more sustainable consumption. Jones and Kammen (2011) quantified carbon footprints of typical U.S. households in 28 cities for a range of household sizes, as well as GHG and financial savings from a set of potential mitigation actions across household types. Chini et al. (2016) evaluated the relationship between water and energy in U.S. household appliances and fixtures and identified opportunities for reducing direct and indirect water and energy use through a cost abatement analysis.
In addition to these large-scale studies, a number of studies have developed tools for tracking consumption and measuring the impacts of individual households. For example, Benders et al. (2006) describe a web-based tool that provides participants with feedback on their energy use and personalized options for energy conservation. Indirect energy use was estimated using a hybrid approach combining input-output analysis with process analysis. The tool was tested with a sample of 300 households in the Netherlands, resulting in a reduction of about 8.5% in direct energy use compared to a control group, though the reduction in indirect energy use was not statistically significant. Using the same analysis program, Abrahamse et al. (2007) developed other web-based tools to encourage households to reduce direct and indirect energy use. Using a combination of tailored information, goal setting, and feedback, they examined whether this combination of interventions would result in changes in direct and indirect energy use, energy-related behaviors, and knowledge. Households exposed to the combination of interventions reduced their direct energy use by about 5%, but as in Benders et al. (2006), changes in indirect energy use were not significant. Jones and Kammen (2011) incorporated their carbon footprint model in an open-access online tool that can be used to inform behavior change at the household level. Another online tool, called EcoRunner, was developed by Frostell et al. (2015) to calculate the direct and indirect environmental loads of purchase decisions by households in Sweden. Use of EcoRunner was demonstrated using average expenditure values to analyze energy use, global warming potential, and nitrogen oxide emissions. Related to these studies are a number of “living lab” experiments, i.e., collaborative work with households to change practices and behaviors, particularly focusing on energy conservation (e.g., Korsnes et al., 2018; Sovacool et al., 2020; Sahakian et al., 2021).
Our work builds on these previous studies to focus on the FEW nexus at the household scale. Specifically, we have developed a web-based software application called HomeTracker for measuring the direct and indirect energy use, water use, and GHG emissions of household food purchases, water use, and energy consumption. While many of the existing studies use datasets that represent either an average level of consumption or a snapshot of consumption behavior, HomeTracker captures FEW consumption in participating households over an extended period of time to allow for trends to be assessed. HomeTracker provides timely feedback on the life-cycle impacts of FEW consumption, potentially affecting conservation behavior and short-term decision making.
Design and development of the HomeTracker application, participant FEW data collection procedures, and environmental impact factors and norming used in the model are summarized in the methods section. (Additional details are provided in Supplementary Information.) Data is then presented to demonstrate how HomeTracker is used in a multidisciplinary study. Finally, limitations of the tool and future work are discussed.
2 Materials and methods
2.1 HomeTracker software development
The Food-Energy-Water Conscious (FEWCON) project focuses on the environmental impacts of household food, energy, and water consumption and potential interventions for reducing those impacts (Watkins et al., 2019). A major component of the project is a study conducted from February 2020 to August 2021 among 174 household participants in Lake County, Illinois, an area selected to be representative of United States suburban populations. The HomeTracker application was developed to provide a common portal for participants in the study.
Through HomeTracker, study participants enter their grocery and restaurant receipt purchases, monthly water bills, monthly natural gas bills, and monthly electricity bills. Environmental impacts, including greenhouse gas emissions and water use, are calculated from this consumption, and feedback is provided to participants in a visual interface highlighting the environmental impact of their household consumption. A key component of HomeTracker is a novel food, energy, and water consumption-based life cycle assessment (LCA) model quantifying direct and indirect environmental impacts from households. The hybrid LCA model, based on input-output and processed-based methods, is implemented in the software to compute environmental impact metrics from consumption input. In addition to collecting utility and food data via HomeTracker, the study also included a series of surveys to capture socio-economic and demographic information, as well as beliefs, attitudes and self-reported behaviors related to food, energy, and water consumption.
As the central communication medium for participants in the FEW consumption study, the HomeTracker system has a number of key system requirements. The primary behavioral (functional) requirements are continuous collection of consumption data and accurate reporting of household consumption via intervention messages. Equally important are the following non-behavioral requirements: minimization of participant burden, maintenance of privacy, and clarity of the consumption input interface and the intervention messaging.
The foundation for the HomeTracker application is Grails, an open-source Java-based framework that uses the Apache Groovy programming language. An Apache Tomcat server hosted at Michigan Technological University provides Java Database Connectivity between the application and the MariaDB relational database management system. Implementing HomeTracker as a web application allows household participants to access the service through any device that supports a standard web browser. Since Grails is the application framework used in Michigan Technological University’s User Interface Design and Implementation course, students can easily transition from that course into a HomeTracker development role. The W3.CSS (cascading style sheet) framework provides a responsive interface layout that adapts to the type of device being used (e.g., laptop, tablet, smartphone).
Development of HomeTracker began in summer 2018; student developers at Michigan Technological University worked iteratively with members of the project team in designing and implementing the app, according to the needs and expectations of the project scientists. In spring 2019, students at Michigan Technological University and Rutgers University provided initial user testing, followed by a pilot test with household volunteers from the Rutgers community. User feedback from this testing drove changes to the interface design, along with development of the HomeTracker User Guide, in summer 2019. A second round of user testing was conducted among FEWCON project staff and a small group of volunteers in Lake County, evaluating the revised interface and checking that the HomeTracker application and the User Guide were compatible. HomeTracker was deployed and made available to study participants in February 2020.
2.2 Data collection
Consumption data collected by HomeTracker include electricity use, natural gas use, water use, and food purchases. Food data collection is the most onerous from the household perspective and was thus limited to three two-week periods during the study period, as opposed to continuous monthly collection of energy and water data. HomeTracker collects consumption data through a variety of automated and manual methods. In addition to these quantitative data, households were asked to respond to a series of surveys and invited to provide reflective statements on their consumption behavior through open-ended survey questions and a journaling feature in HomeTracker.
In designing the data acquisition processes, automation was favored for easing the burden on household participants, but only if the underlying technology was robust and came at minimal risk to accuracy of the data. We initially explored the use of in-house sensor devices (e.g., Smappee, Sense) that household owners can install on the metering equipment in their houses. Many of these devices offer application programming interfaces (APIs) that allow third parties to access data collected by the devices, but they also require some non-trivial work attaching physical sensors to metering equipment. After some experience installing a few such devices in local houses, it became clear that the risk of faulty installation and the cost of installing and maintaining hundreds of individual monitors made this option infeasible.
An alternative automated means of collecting electricity consumption data that avoids the costs and inaccuracies of in-house installation was identified. The study area’s local service provider, Commonwealth Edison (ComEd), partners with a company called UtilityAPI to provide electricity billing data, for consumers who authorize it, to third-party applications. UtilityAPI stores up-to-date versions of these data on its own secure servers so that apps like HomeTracker can access them as needed. In addition, subscribing to the UtilityAPI service provides access to a rich set of additional historical billing data for authorizing consumers. Household participants must authorize UtilityAPI to access their ComEd billing data. Completing the authorization form creates secure credentials that HomeTracker then uses to access data through UtilityAPI.
Unlike electricity, the utilities supplying natural gas and water vary within the study area. The smaller-scale authorities providing these utilities, particularly the local municipalities in charge of water supply, do not have the resources to provide third-party data access. While there are home-installed sensors (e.g., Sense, Pecan Street) that provide monitoring, most of these products were not available during the development of HomeTracker, and risk and cost concerns over installation and maintenance made this option infeasible. Participants instead entered their gas and water billing data manually in HomeTracker using the standard billing statements they receive at regular intervals.
Food data collection occurred during several specified two-week periods in the study. During these periods, household participants were asked to upload all purchases, both food at home (i.e., food purchased with the intent of preparing it at home) and food away from home (i.e., food prepared and purchased outside the home). Participants distinguished between full service restaurants, defined as food establishments that provide not only preparation but also service of the food and limited service restaurants, defined as establishments like delicatessens or cafeterias that prepare but do not serve the food. For food at home, the itemized breakdown of the purchases allows for greater detail and more nuanced analysis. In entering these purchases into HomeTracker, participants were asked to provide details for each item purchased. If the purchase included a receipt, the household participant uploaded images of the receipt and provided an item-by-item description of the purchase. Later, student workers consulted the receipt images and participant descriptions of the line items to determine the food category and confirm price information. For a purchase without receipt images (e.g., farmer’s market, forgotten or lost receipt), household participants provided descriptions and prices of the line items.
2.3 Environmental impact factors
HomeTracker uses a LCA approach to quantify direct and indirect environmental impacts of household consumption of food, energy, and water resources. Life cycle assessment is used to assess the potential environmental impact of a product, process or service using four key steps: i) Goal definition and scoping, ii) Inventory analysis, iii) Impact assessment, and iv) Interpretation of results (Curran 2008). The framework for this LCA-based environmental impact model starts with input of direct household resource consumption values. These resources include water use in gallons (gal), electricity use in kilowatt hours (kWh), natural gas use in therms (therm), and food purchases in U.S. dollars (USD). Environmental impact factors are applied to determine the direct and indirect environmental impact due to the use or consumption of each resource. The direct and indirect environmental impacts are then summed to output total water withdrawal in gallons and total greenhouse gas emissions in kilograms of carbon dioxide equivalents (kg CO2 eq). Figure 1 summarizes the steps in calculating direct and indirect environmental impacts. The environmental impact factors used in these calculations are summarized in Table 1. Sample calculations are shown in Supplementary Information.
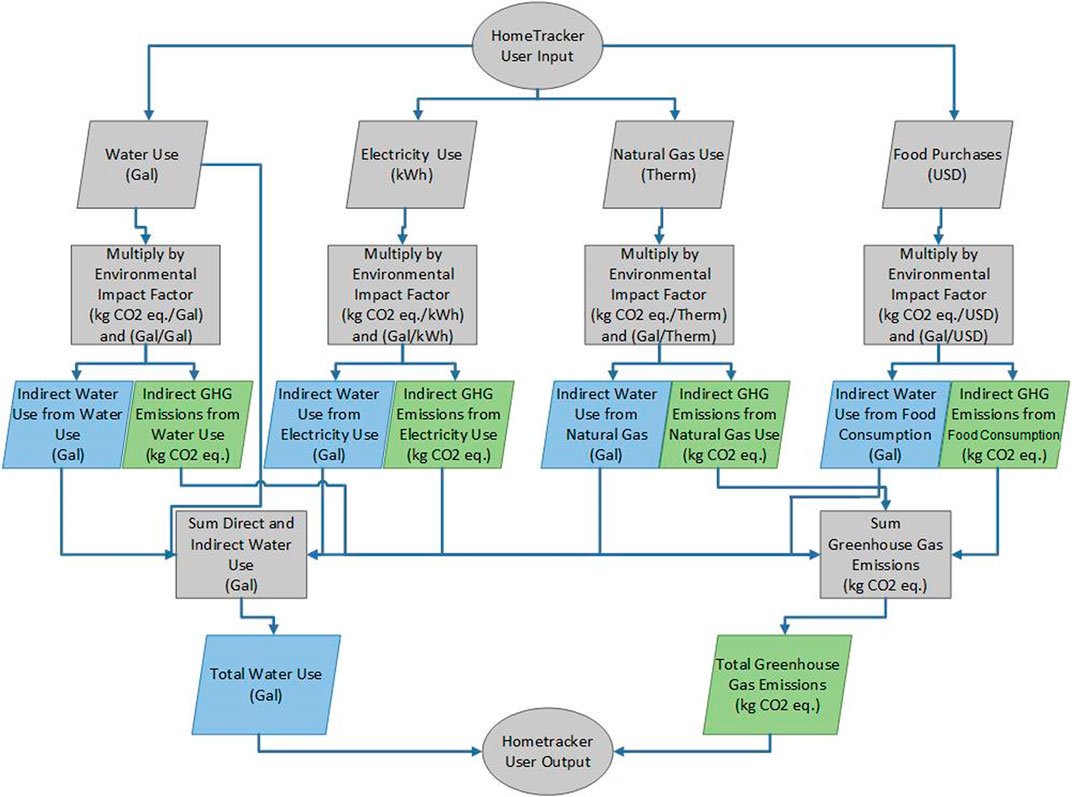
FIGURE 1. Model steps to convert consumption-based direct and indirect inputs to environmental impacts: water use in gallons and greenhouse gas emissions in kg CO2-eq.
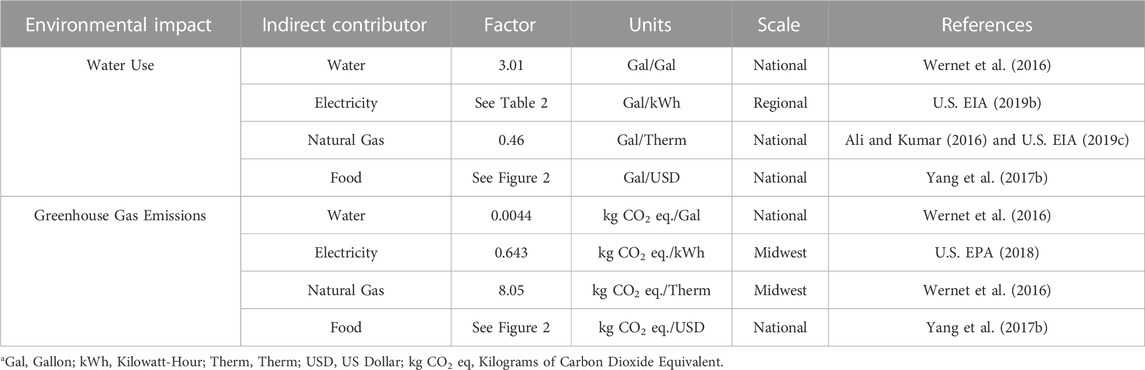
TABLE 1. Summary of environmental impact factors for indirect resource consumption (1 Gal = 0.003785 m3).
2.3.1 Water use factors
It is important to clearly define water use when quantifying it to avoid ambiguity and inconsistency (Grubert, Rogers, and Sanders 2020). Water use in this study refers to water withdrawn from its original source (i.e., blue water). Water use per therm of natural gas was estimated from a study which developed life cycle water use factors for different stages of conventional and shale gas life cycles, combined with Energy Information Administration data on the current proportion of each gas source currently in use in the U.S. (Ali and Kumar 2016; U.S. EIA, 2019c). Water use per gallon of water used at the household is a cumulative estimate that includes both direct water use and indirect water use embedded in all of the materials and energy required to treat and deliver water to the home, as well as all of the unit operations involved in treating water after it leaves the household in a standard municipal wastewater treatment system. Life cycle inventory data for upstream water treatment and delivery, as well as downstream wastewater treatment, comes from the Ecoinvent database (Wernet et al., 2016). Water use factors for food purchases are described in Section 2.3.3, and water use factors for electricity are described in Section 2.3.4.
2.3.2 Greenhouse gas emissions factors
Greenhouse gas emissions per gallon of water used in the household are also estimated from Ecoinvent, and are analyzed with the Intergovernmental Panel on Climate Change 2013 GWP 100a method, which is an impact assessment method that expresses emissions impacts of climate-active greenhouse gas emissions in kg CO2-eq. (Wernet et al., 2016). Greenhouse gas emissions per kilowatt hour (kWh) of electricity generated were estimated by combining U.S. EPA eGRID data on average emissions per kWh for power plant emissions in the RFC West subregion, combined with the average grid composition in the region and the upstream emissions impacts for fuel production for each relevant fuel type from Ecoinvent (U.S. EPA 2018). Greenhouse gas emissions per therm of natural gas were estimated by combining combustion emissions per therm of natural gas with Ecoinvent data on upstream natural gas processing and transmission. Greenhouse gas emissions factors for food purchases are described in Section 2.3.4.
2.3.3 Water use intensity for electricity generation
The Pennsylvania-New Jersey-Maryland Interconnection (PJM) is a Regional Transmission Organization (RTO) that administers the grid for the region in which our study area lies. The water use intensity of electricity generation for the entire fuel mix in PJM was calculated at a monthly resolution for 2019 to best represent the FEWCON study area. The United States Energy Information reports monthly thermoelectric cooling water data at the generator level for power plants in the United States in Form EIA-923 (U.S. EIA 2019a). This form was cross-indexed with Form EIA-860 (U.S. EIA 2019b) to determine which plants are connected to the PJM grid. Since Form EIA-923 only reports on thermoelectric generators, electricity generation data from PJM was used to determine how much electricity generation was attributed to hydroelectric, solar, and wind generation (PJM 2019). Total water withdrawal and total generation were aggregated by month. The total water withdrawal (gallons) in month
Monthly water withdrawal intensity values (AWFi) for PJM are shown in Table 2.
2.3.4 USEEIO v.1.1
The United States Environmentally Extended Input-Output Model (USEEIO v.1.1) is a United States-specific environmentally extended input-output model that can be used to quantify environmental impacts of production and consumption in 389 industry sectors. Environmental data allows for quantification of impacts related to land cover, water, energy use, mineral use, greenhouse gas emissions, air pollutants, nutrients, and toxics. This model was selected for use in this research task as it is useful in performing streamlined life cycle assessment. Environmental impact is quantified per U.S. Dollar (USD) spent, allowing for simple calculation of environmental impact based on purchase data submitted by participants through the HomeTracker interface. The environmental impacts, specifically water use and greenhouse gas emissions, can be calculated for 29 detailed categories of food-related spending. Greenhouse gas emissions are estimated using the 2013 greenhouse gas inventory as compiled by the U.S. Environmental Protection Agency while water withdrawals were determined for irrigation of crops, watering of livestock, cooling water in thermoelectric power generation, mining operations, and other commercial and industrial purposes using multiple data sources as outlined in the USEEIO Model Details (Yang et al., 2017a).
Figure 2 represents the environmental impact factors for calculation of GHG emissions and water withdrawal resulting from food consumption. Packaged meat and dairy have the highest greenhouse gas emissions per dollar spent, while fresh fruits, breakfast cereals, and seafood have notably lower greenhouse gas emissions per dollar spent. Fresh vegetables, melons, and potatoes require the most water per dollar spent. Other water-intensive categories include fresh fruits; sugar, candy and chocolate; snack foods; coffee and tea; and seasonings and dressings. Less water-intensive categories include mushrooms, breakfast cereal, and seafood. Full-service and limited-service restaurant impacts are relatively low for both GHG emissions and water withdrawal per dollar spent compared to other food categories, due to the increase in price of goods purchased at a restaurant rather than at a market, effectively increasing the denominator in the “impact per dollar spent” factor.
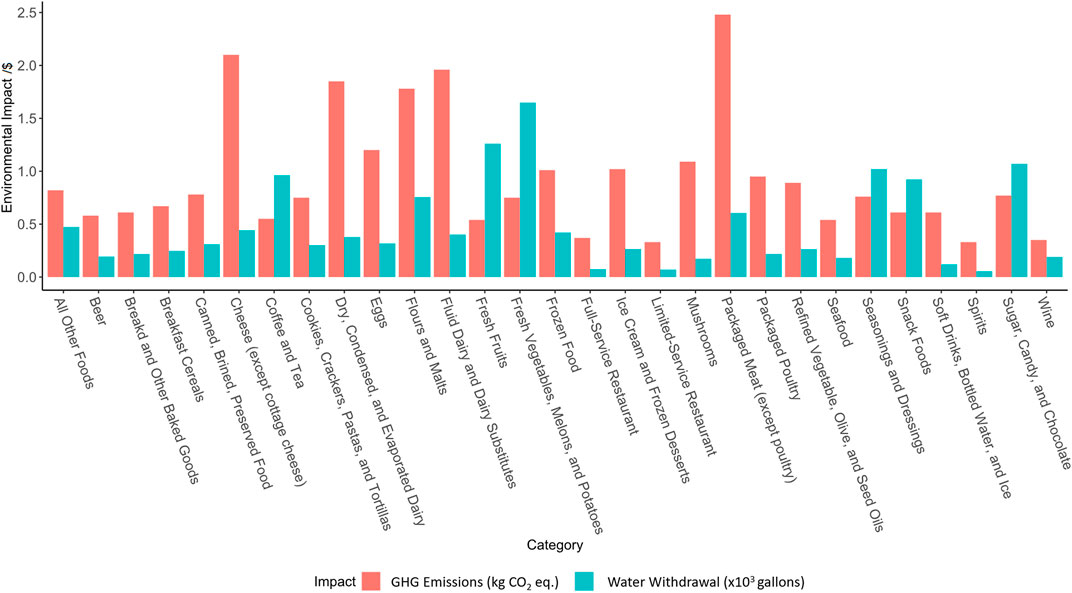
FIGURE 2. Environmental impact factors for calculation of greenhouse gas emissions (kg CO2 eq.) and water withdrawal (gallons) resulting from food purchases (USD) (Yang et al., 2017a).
2.4 Norming values
Table 3 shows the average household consumption values that are displayed as norming feedback to participants in the FEWCON study. According to Steg and Vlek (2009), descriptive norms “refer to the extent to which behaviour is supposed to be commonly approved or disapproved of.” These values were selected to be as representative as possible of Lake County, IL. The average volume of water for domestic water use in Lake County is 6,254 gallons per household per month. This data comes from the United States Geological Survey (USGS) Water Resources National Water Information System for Lake County, IL (USGS 2018). The average monthly household electricity use and natural gas use is 796 kWh and 64 therms, respectively. These values are from the 2018 Residential Energy Consumption Survey (RECS) Report and are based on annual averages for the Midwest United States. This data is representative of the year 2015. The average dollar amount spent on food at home and food away from home is 658 USD and comes from the U.S. Bureau of Labor Statistics 2018 Consumer Expenditure Survey: Table 1,400 (BLS 2018). The average water withdrawal and GHG emissions footprints for food are calculated based on the average dollar amount spent using the United States Environmentally Extended Input Output model (Yang et al., 2017b).
3 Results and discussion
This section presents consumption data and associated environmental impact data for 130 households in Lake County, IL using the HomeTracker application. We show how household consumption and environmental impact data can be analyzed and summarized to identify opportunities for behavior change and reduced environmental impact. Data is analyzed by evaluating temporal trends, trends by household size and income range, and general averages for the entire study group. These trends and observations are summarized in Figures 3–10.
Figure 3 shows average household consumption data for all participating households collected via HomeTracker in 2020 compared with the norming values (Table 3). On average, households participating in the study consumed less electricity and natural gas, but used more water and spent more on food than the norming values. These results may be indicative of the study sample, which on average had household incomes above the median income for the county. Additionally, 79 households reported having children, while 51 households reported having no children. This consumption data can be assessed for individual households, and paired with survey responses to identify specific causes of above or below average household consumption behavior as future work.
HomeTracker food data entry distinguishes between food consumed at home and food consumed away from home. Figure 4 shows average monthly household food consumption both at the home and out of the home for 2020. Notable differences in the style of food consumption can be observed in the households enrolled within the study. While most households spent more in the “Food at Home” category compared to “Food Away From Home,” this proportion varied considerably across households. Total household spending on food was also quite variable, which can be attributed in part to the short duration of our food data collection, as well as the variability between households in size, income, and consumption patterns. Disruptions due to the COVID-19 pandemic may also have contributed to high variability in food purchasing patterns, such as households stocking large quantities of food.
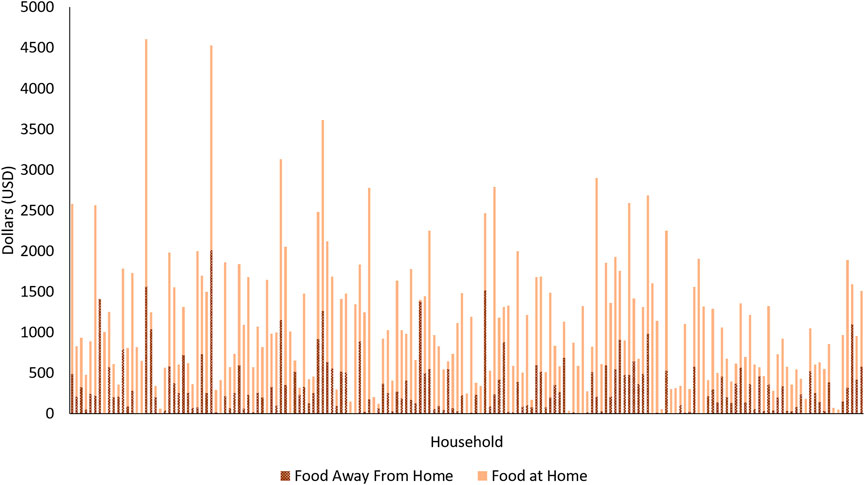
FIGURE 4. Average monthly total food spending for 130 sample households categorized as amount (USD) spent on food consumed away from home (FAFH) and amount spent on food consumed at the home (FAH) in 2020.
Figure 5 shows a summary of direct water and energy use in 130 households over the course of the study. Impacts of the COVID-19 crisis are not apparent in this aggregated data, and variability is consistent with typical seasonal patterns. For example, natural gas use occurs primarily in the winter for heating, while electricity use increases in the summer due to the use of air conditioning, consistent with heating and cooling degree-day data for the county (Figure 6, NOAA, 2022). Another expected pattern is the increase in water use during the growing season, when outdoor water use occurs. Outdoor water use is expected to be inversely proportional to rainfall, and this relationship is observed in the water use data. Specifically, the spring of 2020 was wetter than average (16.7 inches of rainfall in March-May), followed by near-average rainfall in the summer; whereas the spring of 2021 was very dry (just 3.4 inches of rain in March-May), followed by a wet June (4.7 inches of rain) (NOAA, 2022).
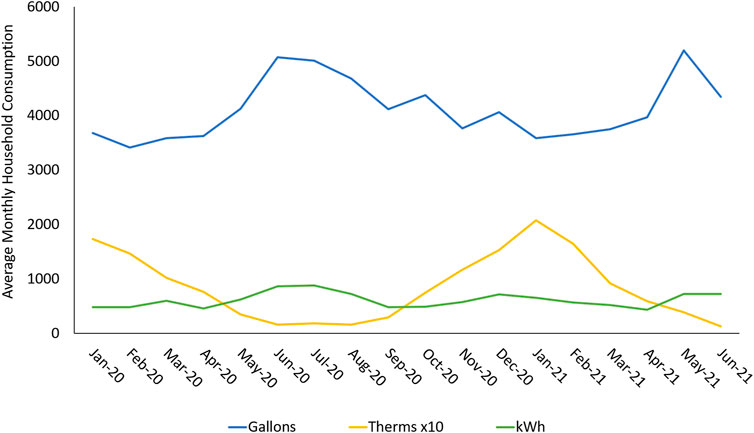
FIGURE 5. Monthly time series data showing average household direct water use (gallons), electricity use (kWh), and natural gas use (therms) over the study period for 130 households. Average household size is 3.2 people.
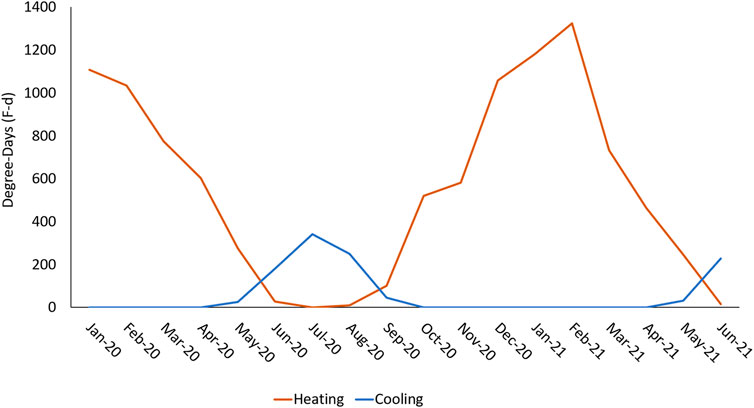
FIGURE 6. Monthly heating and cooling degree-days in Lake County, IL during the study period (NOAA, 2022).
Figures 7, 8 show the variation in contributions to environmental impacts from FEW consumption categories, along with decreases in per capita impacts associated with larger household sizes. Food purchases represent by far the largest contribution to total (blue) water use, and they are also the largest contributor to GHG emissions, with natural gas use and electricity contributing significantly to GHG emissions as well. Direct water use in the home is small compared to indirect water use, and water use makes an insignificant contribution to GHG emissions. The average person in the United States uses 82 gallons per day (Dieter et al., 2018), and in our study the average direct water use was just 42 gallons per person per day. However, the average indirect water use was 755 gallons per person per day. Thirty-seven households did not report household size and were excluded from this analysis.
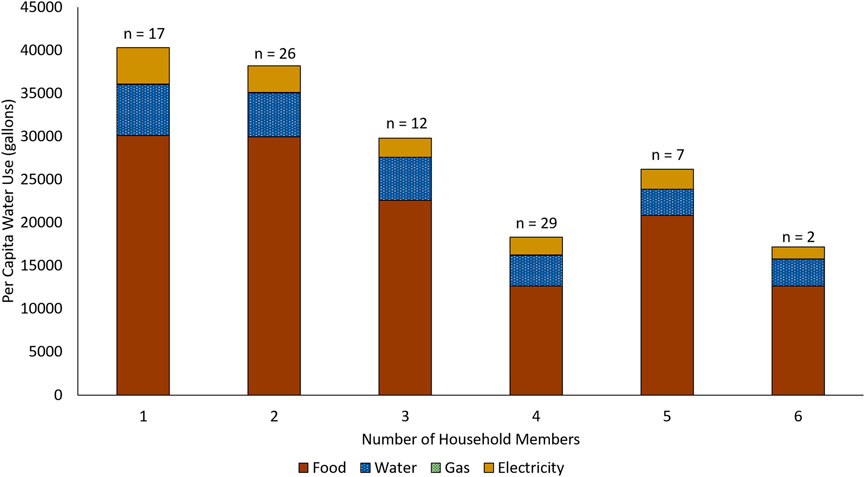
FIGURE 7. Average monthly total (direct + indirect) water use by FEW consumption category and household size in 2020.
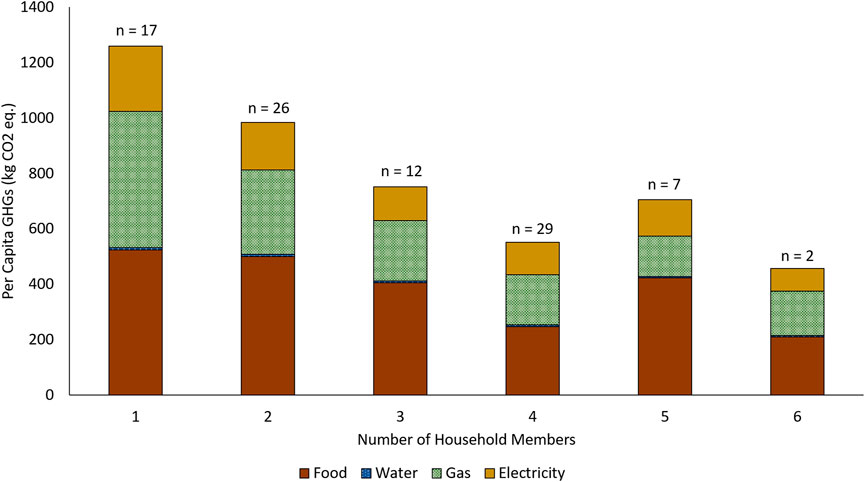
FIGURE 8. Average monthly greenhouse gas emissions by FEW consumption category and household size in 2020.
Figures 9, 10 show how consumption and environmental impacts vary with household income. There are increasing trends in all FEW consumption categories as household income rises, with the greatest increase seen in food purchases. Households in the highest income category spend more than five times the amount on food than do households in the lowest category. This results in sharply rising trends in environmental impacts with increasing income, as the highest-income households have approximately four times the total water use and GHG emissions attributable to the household FEW consumption. Eighteen households did not report their household income and were excluded from this analysis.
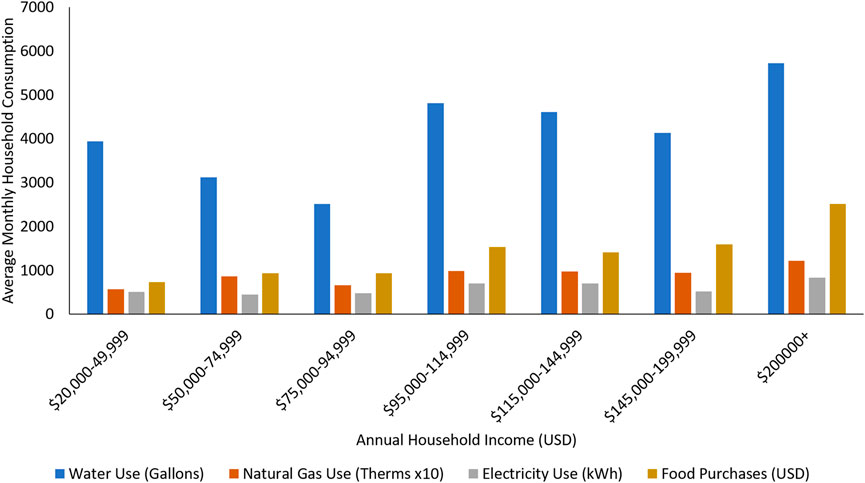
FIGURE 9. Direct FEW consumption (water use, natural gas use, electricity use, and food purchases) by household income range.
4 Future work
While the analyses herein focus on the aggregated data for all participating households, future work will investigate how demographics, attitudes, and behaviors affect consumption patterns of individual households. The impacts of intervention messages, sent periodically by HomeTracker in the closing months of the study, will also be analyzed. The messages were both graphical and textual in form, reporting household consumption and the associated environmental impacts and comparing them to the norming values. Example textual messages are shown in Table 4. This feedback from HomeTracker can help identify targeted messages that will inform households of their consumption patterns and identify mitigation strategies to reduce environmental loads.
There are also a number of methodological and data limitations in our study that could be addressed in future work. First, data collection and analysis focused on food, water, and energy consumption within the home and did not include transportation or other expenditures such as clothing, appliances, furniture, and other goods, as in other household metabolism studies (e.g., Frostell et al., 2015; Vita et al., 2021). This was mainly due to a desire to focus on the FEW nexus and limit the administrative burden placed on study participants. HomeTracker could be extended to account for other activities and household expenditures in the future using various life cycle inventory databases, including USEEIO, Ecoinvent, and the U.S. Life Cycle Inventory Database.
Second, USEEIO v1.1 (Yang et al., 2017a) has some important limitations for ecological footprint analysis. One limitation is that USEEIO considers only water withdrawals and consumptive use of withdrawals (i.e., blue water use) and does not account for rainwater used by crops (i.e., green water use). This can lead to an underestimation of the terrestrial and aquatic ecosystem impacts of food production, as well as an inability to compare results directly with those of other water footprint studies. Another limitation of USEEIO v1.1 is that it is based on the value of commodities at the point of manufacture (producer’s price) rather than at the point of sale (purchaser’s price). Since distribution and retail margins may be considerable, this leads to a systematic overestimation of environmental impacts in our study based on food purchase data. While this may have some effect on household behavior changes (e.g., participants overestimate the relative impact of changing their diet compared to adjusting their thermostat), this is not expected to have a significant impact on observed household behavior since norming values were derived consistently. In future work, the most recent version of USEEIO will be used, and USEEIO v.2 accounts for the value added per commodity between point of manufacture and point of sale (Ingwersen et al., 2022).
5 Conclusion
This paper explores how a life cycle assessment-based software application can facilitate collection and translation of household food, energy, and water consumption data to meaningful environmental impacts. Specifically, the HomeTracker software tool supports quantification of the environmental impact values for household consumption in a typical U.S. suburban area, allowing for examination of what consumers actually purchase and consume over an extended period of time. The HomeTracker was implemented in an intervention study with a data collection period running from February 2020 through June 2021. The study included three two-week food collection periods, continuous electricity monitoring, bi-monthly water data input, and monthly natural gas input. Households also received messaging and took surveys throughout the study. Across all households in the study, seasonal and weather-dependent trends in water, electricity, and natural gas use are apparent, as are significant trends in per capita food, energy, and water consumption with household size and income. Impacts of the COVID-19 pandemic are not apparent in the aggregated data.
Future research using HomeTracker consumption and environmental impact data will investigate the specific attitudes and behaviors that affect consumption levels, as well as attempt to better understand consumption impacts of the COVID-19 pandemic, in individual households. Future work may also include expansion of the environmental impact factors to improve spatial and temporal resolution, in areas with sufficient data availability. It may also include expansion of the geographic coverage of environmental impact factors to allow users across the United States, or globe, to benefit from use of the HomeTracker without modifying the source code. In addition, as sensor and automation technology become more robust and affordable, HomeTracker can be modified to reduce the burden of data entry on users.
Ultimately, we think it is valuable to provide more detailed information to stakeholders, on both the consumption side and the production side of FEWS, regarding the direct and indirect consequences of our current FEW system metabolism on multiple scales. On the consumption side, a more informed set of choices at the household scale might lead people to make different choices about the amount and type of FEW services to consume in order to lower their household impacts. At a policy level, the results of this study could inform policy analyses of environmental labeling and information schemes, as well as the setting of nationally determined contributions to emissions reduction targets. While it is hard to predict the ultimate effects household behavior change may have on the production of FEW services, the impacts could lead to, for instance, a shifting of power production to a different grid mix when baseload power needs are lower, postponement of energy and water infrastructure investments, as conservation offsets projected increases in demand; or a shift in the market share of comparable FEW products with markedly different environmental impacts, such as the rise in popularity of plant-based dairy and meat alternatives.
Data availability statement
The raw data supporting the conclusion of this article will be made available by the authors, without undue reservation.
Ethics statement
The studies involving human participants were reviewed and approved by the Institutional Review Board of Michigan Technological University. The patients/participants provided their written informed consent to participate in this study.
Author contributions
JD: Methodology, data generation, data curation, visualization, investigation, writing—original draft, writing—review and editing. CW: Conceptualization, methodology, software, data generation, writing–original draft, writing—review and editing. DW: Conceptualization, investigation, writing—review and editing, funding. RH: Methodology, data generation, visualization, writing—review and editing. YY: Methodology, data generation. DH: Methodology, data curation, writing—review and editing. SA: Data generation, data curation, investigation, writing—review and editing.
Funding
This work was supported by NSF Grant CBET-1639342, “INFEWS/T3: Climate Change Mitigation via Reducing Household Food, Energy and Water Consumption: A Quantitative Analysis of Interventions and Impacts of Conservation.”
Acknowledgments
This work was made possible with contributions from software developers and maintainers, including Nichole Mackey, Prathyusha Sreedhara, Elijah Cobb, and Zong Deng. We also thank Evan Lanese, Grayson Wagner, Katie Schmidt, Kirk Thelen, Lydia Savatsky, Sarah Larkin, Emma Ryden, Jenna Byron, Jessie Ramsey, Lena Stenvig, Sidney Mechling, and Zach Heimer for their work to categorize food purchase data. Parker Herrera, Holly Berman Caggiano, Cara Cuite, and Rachael Shwom at Rutgers University were instrumental in testing the food data collection protocol and developing data cleaning procedures. Finally, we acknowledge the entire FEWCON team for their input on the development of this software, including other collaborators from Rutgers University, The Pennsylvania State University, University of Minnesota, Arizona State University, Michigan Technological University and the U.S. Forest Service.
Conflict of interest
The authors declare that the research was conducted in the absence of any commercial or financial relationships that could be construed as a potential conflict of interest.
Publisher’s note
All claims expressed in this article are solely those of the authors and do not necessarily represent those of their affiliated organizations, or those of the publisher, the editors and the reviewers. Any product that may be evaluated in this article, or claim that may be made by its manufacturer, is not guaranteed or endorsed by the publisher.
Supplementary material
The Supplementary Material for this article can be found online at: https://www.frontiersin.org/articles/10.3389/fenvs.2023.1059301/full#supplementary-material
References
Abrahamse, W., Steg, L., Vlek, C., and Rothengatter, T. (2007). The effect of tailored information, goal setting, and tailored feedback on household energy use, energy-related behaviors, and behavioral antecedents. J. Environ. Psychol. 27 (4), 265–276. doi:10.1016/j.jenvp.2007.08.002
Alavijeh, K. N., Salehnia, N., Salehnia, N., and Koengkan, M. (2022). ‘The effects of agricultural development on CO2 emissions: Empirical evidence from the most populous developing countries.’. Environ. Dev. Sustain. doi:10.1007/s10668-022-02567-1
Ali, B., and Kumar, A. (2016). 'Development of life cycle water footprints for gas-fired power generation technologies'. Energy Convers. Manag. 110, 386–396. doi:10.1016/j.enconman.2015.12.048
Benders, R. M. J., Kok, R., Moll, H. C., Wiersma, G., and Noorman, K. J. (2006). New approaches for household energy conservation—in search of personal household energy budgets and energy reduction options. Energy Policy 34 (18), 3612–3622. doi:10.1016/j.enpol.2005.08.005
Boehm, R., Wilde, P. E., Ver Ploeg, M., Costello, C., and Cash, S. B. (2018). 'A comprehensive life cycle assessment of greenhouse gas emissions from US household food choices'. Food Policy 79, 67–76. doi:10.1016/j.foodpol.2018.05.004
Chandio, A. A., Akram, W., Ahmad, F., and Ahmad, M. (2020). Dynamic relationship among agriculture-energy-forestry and carbon dioxide (CO2) emissions: Empirical evidence from China. Environ. Sci. Pollut. Res. 27, 34078–34089. doi:10.1007/s11356-020-09560-z
Chandio, A. A., Akram, W., Ozturk, I., Ahmad, M., and Ahmad, F. (2021). Towards long-term sustainable environment: Does agriculture and renewable energy consumption matter? Environ. Sci. Pollut. Res. 28, 53141–53160. doi:10.1007/s11356-021-14540-y
Chini, C. M., Schreiber, K. L., Barker, Z. A., and Stillwell, A. S. (2016). Quantifying energy and water savings in the US residential sector. Environ. Sci. Technol. 50, 9003–9012. doi:10.1021/acs.est.6b01559
Curran, M. A. (2008). “Life-cycle assessment” in Encyclopedia of Ecology, Editor S. E. Jørgensen, and B. D. Fath (New York: Academic), 2168–2174.
Dieter, C. A., Maupin, M. A., Caldwell, R. R., Harris, M. A., Ivahnenko, T. I., Lovelace, J. K., et al. (2018). Estimated use of water in the United States in 2015: U.S. Geological Survey Circular. Rev. Geophys. 1441, 65. doi:10.3133/cir1441
D'Odorico, P., Dell'Angelo, J., Davis, K. F., Rosa, L., Carr, J. A., Suweis, S., et al. (2018). 'The global food-energy-water nexus'. Rev. Geophys. 56, 456–531. doi:10.1029/2017rg000591
Falkenmark, M., and Rockström, J. (2006). The new blue and green water paradigm: Breaking new ground for water resources planning and management. J. Water Resour. Plan. Manag. 132 (3), 129–132. doi:10.1061/(asce)0733-9496(2006)132:3(129)
Flammini, A., Puri, M., Pluschke, L., and Dubois, O. (2014). Walking the nexus talk: Assessing the water-energy-food nexus in the context of the sustainable energy for all initiative. Rome: Food and Agricultural Organization of the United Nations.
Frostell, B. M., Sinha, R., Assefa, G., and Olsson, L. E. (2015). 'Modeling both direct and indirect environmental load of purchase decisions: A web-based tool addressing household metabolism',. Environ. Model. Softw. 71, 138–147. doi:10.1016/j.envsoft.2015.05.014
Grubert, E., Rogers, E., and Sanders, K. (2020). ‘Consistent Terminology and reporting are needed to describe water quantity use’. J. Water Resour. Plan. Manag. 146, 04020064. doi:10.1061/(ASCE)WR.1943-5452.0001241
Grubert, E., and Sanders, K. T. (2018). 'Water use in the United States energy system: A national assessment and unit process inventory of water consumption and withdrawals',. Environ. Sci. Technol. 52, 6695–6703. doi:10.1021/acs.est.8b00139
Hallström, E., Gee, Q., Scarborough, P., and Cleveland, D. A. (2017). 'A healthier US diet could reduce greenhouse gas emissions from both the food and health care systems'. Clim. Change 142, 199–212. doi:10.1007/s10584-017-1912-5
Heller, M. C., Amelia, W. S., Robert, M., Keoleian, G. A., and Rose, D. (2018). 'Greenhouse gas emissions and energy use associated with production of individual self-selected US diets'. Environ. Res. Lett. 13, 044004. doi:10.1088/1748-9326/aab0ac
Heller, M. C., and Keoleian, G. A. (2015). 'Greenhouse gas emission estimates of US dietary choices and food loss'. J. Industrial Ecol. 19, 391–401. doi:10.1111/jiec.12174
Hertwich, E. G., and Peters, G. P. (2009). Carbon footprint of nations: A global, trade-linked analysis. Environ. Sci. Technol. 43 (16), 6414–6420. doi:10.1021/es803496a
Hunter, M. C., Smith, R. G., Schipanski, M. E., Atwood, L. W., and Mortensen, D. A. (2017). Agriculture in 2050: Recalibrating targets for sustainable intensification. BioScience 67 (4), 386–391. doi:10.1093/biosci/bix010
Ingwersen, W. W., Li, M., Young, B., Vendries, J., and Birney, C. (2022). USEEIO v2.0, The US environmentally-extended input-output model v2.0. Sci. Data 9, 194. doi:10.1038/s41597-022-01293-7
Ivanova, D., Stadler, K., Steen-Olsen, K., Wood, R., Vita, G., Tukker, A., et al. (2016). 'Environmental impact assessment of household consumption'. J. Industrial Ecol. 20, 526–536. doi:10.1111/jiec.12371
Jones, C. M., and Kammen, D. M. (2011). 'Quantifying carbon footprint reduction opportunities for US households and communities'. Environ. Sci. Technol. 45, 4088–4095. doi:10.1021/es102221h
Kanyama, C., Annika, J. N., and Benders, R. (2021). 'Shifting expenditure on food, holidays, and furnishings could lower greenhouse gas emissions by almost 40%'. J. Industrial Ecol. 25, 1602–1616. doi:10.1111/jiec.13176
Koengkan, M., and Fuinhas, J. A. (2021). Does the overweight epidemic cause energy consumption? A piece of empirical evidence from the European region. Energy 216, 1–19. doi:10.1016/j.energy.2020.119297
Koengkan, M., and Fuinhas, J. A. (2022). ‘Does the obesity problem increase environmental degradation? Macroecon. Soc. Evid. Eur. countries.’ Econ. 10 (6), 131. doi:10.3390/economies10060131
Kok, R., Benders, R. M. J., and Moll, H. C. (2006). Measuring the environmental load of household consumption using some methods based on input–output energy analysis: A comparison of methods and a discussion of results. Energy Policy 34 (17), 2744–2761. doi:10.1016/j.enpol.2005.04.006
Korsnes, M., Berker, T., and Woods, R. (2018). Domestication, acceptance and zero emission ambitions: Insights from a mixed method, experimental research design in a Norwegian Living Lab. Energy Res. Soc. Sci. 39, 226–233. doi:10.1016/j.erss.2017.11.004
Kross, A., Kaur, G., and Jaeger, J. A. (2022). A geospatial framework for the assessment and monitoring of environmental impacts of agriculture. Environ. Impact Assess. Rev. 97, 106851. doi:10.1016/j.eiar.2022.106851
Marston, L., Ao, Y., Konar, M., Mekonnen, M. M., and Hoekstra, A. Y. (2018). 'High-resolution water footprints of production of the United States'. Water Resour. Res. 54, 2288–2316. doi:10.1002/2017wr021923
NOAA (2022). Climate data online. Available at: https://www.ncdc.noaa.gov/cdo-web/datatools.
PJM (2019). Generation by fuel type. Available at: https://dataminer2.pjm.com/feed/gen_by_fuel.
Sahakian, M., Rau, H., Grealis, E., Godin, L., Wallenborn, G., Backhaus, J., et al. (2021). ‘Challenging social norms to recraft practices: A living lab approach to reducing household energy use in eight European countries. Energy Res. Soc. Sci. 72, 101881. doi:10.1016/j.erss.2020.101881
Scanlon, B. R., Ruddell, B. L., Reed, P. M., Hook, R. I., Zheng, C., Tidwell, V. C., et al. (2017). 'The food-energy-water nexus: Transforming science for society'. Water Resour. Res. 53, 3550–3556. doi:10.1002/2017wr020889
Sovacool, B. K., and Sovacool, K. E. (2009). 'Identifying future electricity–water tradeoffs in the United States'. Energy Policy 37, 2763–2773. doi:10.1016/j.enpol.2009.03.012
Sovacool, B. K., Martiskainen, M., Osborn, J., Anaam, A., and Lipson, M. (2020). From thermal comfort to conflict: The contested control and usage of domestic smart heating in the United Kingdom. Energy Res. Soc. Sci. 69, 101566. doi:10.1016/j.erss.2020.101566
Steen-Olsen, K., Wood, R., and Hertwich, E. G. (2016). ‘The carbon footprint of Norwegian household consumption 1999–2012.’. J. Industrial Ecol. 20 (3), 582–592. doi:10.1111/jiec.12405
Steg, L., and Vlek, C. (2009). 'Encouraging pro-environmental behaviour: An integrative review and research agenda'. J. Environ. Psychol. 29, 309–317. doi:10.1016/j.jenvp.2008.10.004
Tom, M. S., Fischbeck, P. S., and Hendrickson, C. T. (2016). 'Energy use, blue water footprint, and greenhouse gas emissions for current food consumption patterns and dietary recommendations in the US'. Environ. Syst. Decis. 36, 92–103. doi:10.1007/s10669-015-9577-y
U.E. EIA (2019c). Natural Gas Explained: Where our natural gas comes from U.S. Energy Information Administration. Available at: https://www.eia.go/energyexplained/naturalgas/where-our-natural-gas-comes-from.php.
U.S. BLS (2018). U.S. Bureau of labor Statistics 2018 consumer expenditure survey: Table 1400. Washington, DC, United States: U.S. Bureau of Labor Statistics.
U.S. EIA (2018). US. Residential Energy Consumption Survey (RECS). Available at: https://www.eia.gov/consumption/residential/data/2015/.
U.S. EIA (2019c). Natural Gas Explained: Where our natural gas comes. Washington, DC, United States: U.S. Energy Information Administration. Available at: https://www.eia.gov/energyexplained/natural-gas/where-our-natural-gas-comes-from.php.
U.S. EPA (2018). Emissions and generation resource integrated database (eGRID). Available at: https://www.epa.gov/egrid.
USGS (2018). Water use data for Illinois. Reston, VA, United States: U.S. Geological Survey. Available at: https://waterdata.usgs.gov/il/nwis/water_use.
U.S. EIA (2019a). Annual cooling summary data - EIA-923 data file. Available at: https://www.eia.gov/electricity/data/eia923/.
U.S. EIA (2019b). Annual Electric Generator data - EIA-860 data file. Available at: https://www.eia.gov/electricity/data/eia860/.
Vita, G., Rao, N. D., Usubiaga-Liaño, A., Min, J., and Wood, R. (2021). Durable goods drive two-thirds of global households’ final energy footprints. Environ. Sci. Technol. 55 (5), 3175–3187. doi:10.1021/acs.est.0c03890
Watkins, D., Shwom, R., Schelly, C., Agusdinata, B., Floress, K., and Halvorsen, K. E. (2019). “Understanding household conservation, climate change and the food–energy–water nexus from a transdisciplinary perspective,” in A research agenda for environmental management (Cheltenham, United Kingdom: Edward Elgar Publishing).
Wernet, G., Bauer, C., Steubing, B., Reinhard, J., Moreno-Ruiz, E., and Weidema, B. (2016). 'The ecoinvent database version 3 (part I): Overview and methodology'. Int. J. Life Cycle Assess. 21, 1218–1230. doi:10.1007/s11367-016-1087-8
Wilson, J., Tyedmers, P., and Spinney, J. E. L. (2013). An exploration of the relationship between socioeconomic and well-being variables and household greenhouse gas emissions. J. Industrial Ecol. 17, 880–891. doi:10.1111/jiec.12057
Wood, R., Bulavskaya, T., Ivanova, O., Stadler, K., Simas, M., Tukker, A., et al. (2013). CREEA report D7.2: Update EXIOBASE with WP3-6 input.
Yang, Y., Ingwersen, W. W., Hawkins, T. R., Srocka, M., and Meyer, D. E. (2017a). USEEIO model details supporting information for USEEIO: A new and transparent United States environmental extended input-output model. New York: Elsevier Science Ltd.
Yang, Y., Hawkins, T. R., Srocka, M., Meyer, D. E., and Ingwersen, W. W. (2017b). 'USEEIO: A new and transparent United States environmentally-extended input-output model',. J. Clean. Prod. 158, 308–318. doi:10.1016/j.jclepro.2017.04.150
Keywords: environmental life cycle assessment, food-energy-water (FEW) nexus, resource management, household consumption, web application development, household monitoring
Citation: Daignault J, Wallace C, Watkins D, Handler R, Yang Y, Heaney D and Ahamed S (2023) A household-scale life cycle assessment model for understanding the food-energy-water nexus. Front. Environ. Sci. 11:1059301. doi: 10.3389/fenvs.2023.1059301
Received: 01 October 2022; Accepted: 30 January 2023;
Published: 13 February 2023.
Edited by:
Charles Vörösmarty, CUNY Advanced Science Research Center, United StatesReviewed by:
Abbas Ali Chandio, Sichuan Agricultural University, ChinaMatheus Koengkan, University of Aveiro, Portugal
Hong Yang, Swiss Federal Institute of Aquatic Science and Technology, Switzerland
Copyright © 2023 Daignault, Wallace, Watkins, Handler, Yang, Heaney and Ahamed. This is an open-access article distributed under the terms of the Creative Commons Attribution License (CC BY). The use, distribution or reproduction in other forums is permitted, provided the original author(s) and the copyright owner(s) are credited and that the original publication in this journal is cited, in accordance with accepted academic practice. No use, distribution or reproduction is permitted which does not comply with these terms.
*Correspondence: Jessica Daignault, jkdaigna@mtu.edu