- 1School of Economics, Hainan University, Haikou, China
- 2Institute of Open Economy, Hainan province, Haikou, China
- 3School of Financial and Business Management Studies Federal Polytechnic Ohodo, Enugu, Nigeria
- 4Department of Economics and Development studies, Alex Ekwume Federal University Ndufu Alike, Abakaliki, Nigeria
- 5Department of Economics, Cyprus International University, Nicosia, Turkey
- 6Department of Agricultural Finance and Banking, Bangladesh Agricultural University, Mymensingh, Bangladesh
- 7Department of Agribusiness, Bangabandhu Sheikh Mujibur Rahman Agricultural University, Gazipur, Bangladesh
- 8Department of Economics, University of Nigeria, Nsukka, Nigeria
First and foremost, the present study seeks to traverse the informal sector characterized by a shadow economy in the presence of financial development, economic growth, and stock market performance on environmental pollution in Nigeria from 1981 to 2019. The dynamic autoregressive distributed lag (DARDL) approach was used to measure the short- and long-run elasticities, while spectral causality is applied to categorize the causal directions. Findings from the study revealed that the structural break unit root test revealed that all variables are stationary at first difference. The ARDL bound test confirmed the existence of long-run association among the used variables. The ARDL long-run results reveal that economic growth, financial development, and stock market performance are significantly responsible for carbon emission in Nigeria, while the shadow economy significantly improves environmental quality in Nigeria. Findings from the spectral causality results show a unidirectional causal relationship between financial development, economic growth, trade, stock market performance, and shadow economy to carbon emission in Nigeria. The empirical findings of this study provide some perceptive policy recommendations to overcome the adverse effect of carbon emissions in the environment.
1 Introduction
Motivated by the prevalence of informal economy and the role it plays in the economy in Nigeria, it has become imperative to examine its impact on the environment. Nigeria has the second-largest shadow economy (56.8% of GDP) among the sub-Saharan African nations, after only Zimbabwe (60.6% of GDP) (Medina and Schneider, 2018). In addition, Nigeria is the fourth-largest shadow economy in the world, after Georgia (64.87% of GDP), Bolivia (62.28% of GDP), and Zimbabwe (60.6% of GDP) (Medina and Schneider, 2018). Thus, Nigeria is a country of concern in the context of the shadow economy and its effects on the economy and environment, although the shadow economy size is declining with an annualized rate of −10.63% between 1991 and 2017, and this fluctuation is not significant compared to that of other African countries (Camara, 2022; Qiang et al., 2022). As a result, Nigeria's enormous shadow economy may have a considerable impact on both environmental stewardship and industrial progress, two of the biggest problems the nation is now experiencing. However, a boom in “dirty growth” has coincided with worrisome pollution levels in Nigeria (Agboola and Bekun, 2019). According to the World Bank (2021), even though Nigeria and six other nations generate 40% of global oil supply, they are also responsible for roughly two-thirds (65%) of the gas flared globally over the previous 9 years. According to Maduka et al. (2022), this ranks Nigeria as one of the top emitters in the world. As a result, pollution research is still essential for fostering green growth in Nigeria.
However, it is still questionable whether a bigger shadow economy improves or degrades the quality of the environment, especially for Nigeria, which has one of the biggest shadow economies globally. In order to meet the COP26 target of net-zero emission by 2060 and the sustainable development goals within the allotted timeframe, it is crucial to investigate the relationship between the shadow economy and environment in the context of Nigeria, and thereby policymakers can react accordingly.
Recent studies suggest a variety of macroeconomic indicators, including aggregated energy usage, economic growth, trade, financial development, and resource depletion, used to describe shadow economy and environmental sustainability (Camara 2022; dada et al., 2021; Chen et al., 2018; Bekun et al., 2019; Jahanger et al., 2022a; Yang et al., 2021b). It is impossible to overstate the impact of economic growth on environmental damage since increasing production in most nations results in more pollution; Nigeria, one of the biggest economies in Africa, is no exception (Whiting, 2019). The stock market is another significant aspect that may be tied to environmental progress (Younis et al., 2021). According to Sadorsky (2010), the stock market is very appealing to businesses since it enables entrepreneurs to raise extra capital and equity finance for business expansion. It is projected that this increased economic activity would result in a high energy demand, which might have an impact on the environment (Younis et al., 2021; Yang et al., 2020a; Usman et al., 2022a, Usman et al., 2022b, Usman et al., 2022c; Yang et al., 2021a; Qayyum et al., 2021; Bilal et al., 2021; Jahanger et al., 2022c). Another element that harms the environment is financial development. Financial development spurs economic expansion, which in turn draws more investments and necessitates greater energy use, which leads to CO2 emissions (Tamazian et al., 2009; Kamal et al., 2021). Additionally, there is debate on the relationship between trade and the environment. International trade, according to some studies, has a significant impact on pollution since it increases countries’ energy usage and CO2 emissions when they exchange technology, goods, and resources (Khaskheli et al., 2021; Jiang et al., 2022a, 2022b). Some people have proposed the fact that trade operations initially harm the environment because of lax environmental standards. However, trade operations are more likely to decrease pollution in later phases of growth with robust environmental legislation (Khaskheli et al., 2021; Ahmad et al., 2022; Jiang et al., 2022c, Jiang et al., 2022d; Wan et al., 2022; Ke et al., 2022; Yu et al., 2020, Yu et al., 2021, Yu et al., 2022).
However, the relationship between the shadow economy, stock market, and emission nexus is still unexplored, emphasizing Nigeria as a developing nation and a substantial contributor to global warming (Wang et al., 2022a; Wang et al., 2022b; Long et al., 2015, Long et al., 2017, Long et al., 2018). Considering the aforementioned circumstances, this study seeks to investigate the relationship between CO2 emission, the shadow economy, economic growth, and stock market performance in Nigeria while controlling for trade and financial depth for the first time. To that aim, the extent of the research and econometric approach used in this work makes a significant contribution to the body of knowledge. First, to the state of the art, this research is among the first to concentrate on the effects of Nigeria’s shadow economy on the environment. However, considering the magnitude of the shadow economy in this nation, research of this kind can assist policymakers in understanding the function of the shadow economy in the transition to greener development. Second, a valuable contribution to the body of extant literature is related to the focus on Nigeria’s stock market development in terms of environmental degradation, which was not taken into account in earlier studies. Third, dynamic Autoregressive Distributed Lag (DARDL) simulations, a novel method developed by Jordan and Philips (2018), are used to supplement the flexible ARDL modeling strategy, which only generates complex in-sample parameters. In essence, this approach depicts how an environmental indicator reacts to potential shocks from a certain regressor over a defined time frame.
The next sections of the study are framed in the following way: the next part reviews pertinent literature and Section 3 shows the data source, an empirical model, and an econometric procedure. The results of the econometric investigation are illustrated in Section 4 before being discussed in Section 5. The conclusion of the research with some policies and future research directions is covered in Section 6.
2 Literature review
2.1 Nexus between shadow economy and CO2 emission
The corpus of research on the relationship between the shadow economy and environmental quality is expanding. However, various nations have diverse findings on this link. Imamoglu (2018) examined the correlation between the extent of the shadow economy and CO2 emissions in Turkey from 1970 to 2014. According to the report, increased production-related environmental pollution is being slowed down by the rise of the informal economy. The informality–environmental quality nexus for 22 sub-Saharan African nations between 1991 and 2005 was revisited by Nkengfack et al. (2021) using the ARDL technique. Higher shares of the shadow economy are observed to affect CO2 emissions in both short and long terms, especially in lower-middle-income nations, in accordance with the scale impact of the shadow economy.
On the contrary, several research studies have confirmed the deregulation impact of informality on the environment. According to Abid (2015), the shadow economy in Tunisia expands at the expense of the environment, as measured by carbon dioxide emissions, similar to the official sector’s work. Based on a co-integrated VECM model design, this monotonically positive connection has persisted from the years 1980 through 2009. Similarly, Chen et al. (2018) investigate how environmental laws and the scale of the shadow economy affected environmental quality in 30 Chinese regions between 1998 and 2012. The effectiveness of environmental controls is reduced by the shadow economy, which increases the environmental costs of production activities. Similar to this, Baloch et al. (2021) assessed the environmental hazards brought on by the expansion of the shadow economy. The analysis confirms that the principal sources of CO2 emissions are greatly increased by subterranean economic activity.
According to Shao et al. (2021), the shadow economy might be used as a tool to control production-related environmental concerns. The panel data threshold regressions and co-integration methods show that the shadow economy exhibits unfavorable short- and long-term relationships with gas emissions. On the other hand, Mazhar and Elgin (2013) used data from more than 100 nations between 2007 and 2010 to demonstrate the veracity of the deregulation impact at the global level. The study explains how environmental contamination occurs when there is unregulated commercial activity. In particular, the implementation of strict environmental regulations in the official sector would cause the shadow economy to grow, which in turn will increase carbon dioxide emissions. Canh et al. (2019) found the link between the shadow economy and the emission of greenhouse gases, such as N2O, CH4, and CO2, for 106 nations. The effect of the shadow economy on economic growth and CO2 emissions in ECOWAS nations was empirically explored by Camara (2022). The results show that the shadow economy reduces economic growth and CO2 emissions. The effect of the shadow economy on economic development, however, is greater and more substantial than the effect on CO2 emissions. Contrary to the findings mentioned above, there is variability in the informality–environmental quality connection even in nations with comparable levels of institutional quality, development, and income. Recent research on South Asian nations by Sahail et al. (2021) showed that the rise of the subterranean economy only lowers CO2 emissions in India.
2.2 Nexus between the stock market and CO2 emissions
Shobande and Ogbeifun (2022) examined whether stock market investments increase GHG emissions in Organization for Economic Co-operation and Development (OECD) nations using yearly data from the World Bank from 1980 to 2019. The study uses panel-standard fixed effects, as well as the Arellano–Bover and Blundell–Bond dynamic techniques, to demonstrate that stock–investor confidence is crucial for emission reduction in OECD nations. Furthermore, the findings point to a possible method through which the stock market might impact emissions in OECD nations.
Jaggi et al. (2018) conducted an empirical research study to determine the value of carbon information for investors in the Italian economy. They found that when companies disclose their carbon footprint, the market reacts favorably. In the same way, research in the United States and the United Kingdom found a link between stock investments, carbon disclosure, and emissions (Plumlee et al., 2015; Matsumura et al., 2014; Middleton, 2015; Jahanger et al., 2021a; Usman and Jahanger, 2021; Usman et al., 2021a; Jahanger et al., 2022a, Jahanger et al., 2022b; Li et al., 2022; Wang et al., 2022b). According to Lee at al., (2015), investors are skeptical of carbon disclosure since it impugns investment decisions and stock prices. Institutional investors, according to Jahanger, (2021a); Bolton and Kacperczyk, (2021); Yu and Wang, (2021); Yu and Liu, (2022), apply exclusionary screening in a few major industries based on the direct emission rate. Byrd & Cooperman, (2018); Zeng, et al., (2020), on the other hand, believe that if carbon emissions are not included in investing choices, investors and assets may be exposed to hazards. Zafar et al. (2019) investigated the influence of the stock market, banking sector development, and renewable energy on carbon emissions in the G-7 and N-11 countries using the panel-bootstrap-cointegration technique. They found that the stock market development index has a favorable impact on carbon emissions in the G-7 nations but has a negative impact in the N-11 countries.
Furthermore, Yue et al. (2019) show that the development of stock markets leads to lower energy consumption, particularly in developed stock markets, due to lower financing costs for public and private sectors, allowing for the introduction of advanced energy-saving technologies and improved energy efficiency. Razmi et al. (2019) used the ARDL method to investigate the relationship between two types of renewable energy consumption, stock market development, and economic growth in Iran. The findings show that stock market value influences renewable energy consumption in the long run.
Chnag et al. (2020) investigated whether good stock returns affect changes in CO2 emissions, or vice versa, using a financial market-based technique based on the Granger causality test to assess cause and effect or leader and follower. The empirical data clearly reveal that all statistically significant causation findings from stock market returns to CO2 emissions from coal, oil, and gas are unidirectional, but not the other way around. More crucially, the regression findings show that when stock returns increase by 1%, CO2 emissions from coal burning reduce by 9% across the nations. Furthermore, when stock returns increase by 1%, CO2 emissions from oil combustion increase by 2%. Stock market capitalization and foreign direct investment, according to Nguyen et al. (2021), may contribute to carbon emissions in G6 nations.
2.3 Nexus between financial development and CO2 emissions
Since the global economic crisis, scholars and policymakers have been paying special attention to the link between carbon emissions and financial development, and some believe that financial development may help reduce carbon emissions. Thus, Adebayo et al. (2022) used historical data from 1969 to 2019 to examine the effects of financial development on CO2 emissions in the MINT nations. These findings suggest that in the MINT countries, there exist strong feedback causal relationships between financial development and CO2 emissions in sub-sampled periods. According to the author, the Chinese economy’s financial expansion is a major generator of carbon emissions. Zaidi et al. (2019) investigated the dynamic relationship between globalization, financial development, and carbon emissions in Asian Pacific Economic Cooperation nations, and their findings revealed that financial development decreased carbon emissions in the short and long run. Tsaurai (2019) observed that financial development had a favorable impact on carbon emissions in Africa. Based on their analysis of the influence of financial development on carbon emissions in 155 established, emerging, and developing countries, Jiang and Ma (2019) concluded that financial development had a beneficial impact on carbon emissions.
Using an extended approach of moments, Acheampong et al. (2020) evaluated financial-market trends and carbon-emission intensity in 83 nations. They found that the influence of financial market growth on carbon emission intensity varied depending on the stage of financial development in each country. Shobande and Asongu (2021) investigated the causal relationship between financial development and climate change in Eastern and Southern Africa, concluding that financial growth had a negative influence on carbon emissions. Using regional panel data from 1997 to 2011, Xiong et al. (2017) demonstrated that financial development might enhance the environment. Gök, (2020) used a meta-analysis to show that financial development leads to environmental degradation. Financial development, according to Acheampong (2019), enables businesses to acquire the lower-cost financing required to deliver environmentally friendly technologies. Khan et al. (2021) revealed that financial development might assist in reducing carbon emissions for a panel of 184 nations. Using a cross-sectionally augmented, autoregressive-distributed-lag model, Shen et al. (2021) found that financial development had a favorable influence on carbon emissions in the Chinese economy. In China, Li and Wei (2021) repeatedly found a link between financial development and carbon emissions. Xu et al. (2018) investigated the role of financial development on environmental degradation in Saudi Arabia between 1971 and 2016, using a globalization and power consumption model. Financial development, according to empirical evidence, leads to CO2 emissions and lowers environmental quality.
2.4 Nexus between economic growth and CO2 emissions
In empirical studies, the link between economic growth and environmental pollution has been well-documented. A variety of studies were evaluated, encompassing many nations, variables, and methodology. Zhang et al. (2021) found that economic growth had a beneficial influence on CO2 emissions. Using data from 1971 to 2014, Adebayo and Kalmaz (2021) used ARDL, FMOLS, and DOLS methodologies to find a positive interaction of economic growth on CO2 emissions in Egypt. Adebayo (2020) discovered that economic development had a beneficial impact on CO2 emissions in Mexico. Prastiyo et al. (2020) used the ARDL approach to find favorable effects of economic growth on CO2 emissions for Indonesia from 1970 to 2015. Using annual data from 1981 to 2016, Odugbesan and Adebayo (2020) discovered the beneficial effects of economic growth on CO2 emissions in Nigeria. Nondo and Kahsai (2020) used the ARDL technique to show that economic expansion had a favorable impact on CO2 emissions in South Africa from 1970 to 2016. Kirikkaleli and Kalmaz (2020) discovered the favorable effects of economic development on CO2 emissions in Turkey from 1960 to 2016.
A number of studies have also shown that economic expansion has a favorable impact on CO2 emissions in a group of countries. Between 1980 and 2019, the study by Maâlej and Cabagnols (2020) showed the influence of economic growth on carbon emissions in a number of West African nations. Vo et al. (2019) discovered that CO2 emissions are positively related to economic growth in ASEAN. Using the data for MINT nations with temporal coverage from 1980 to 2018, Adebayo et al., (2020) discovered a positive relationship between economic growth and CO2 emissions. Wang et al. (2019) also found that economic expansion increases CO2 emissions using the DSUR approach and data from APEC nations from 1990 to 2014. Using the STIRPAT and ARDL techniques, Zmami and Ben-Salha (2020) investigated the beneficial effects of economic development on CO2 emissions in GCC nations between 1980 and 2017. According to Teng et al. (2020), economic expansion has a favorable impact on CO2 emissions in OECD nations.
3 Data and Methods
3.1 Data and model
The present study tends to assess the drivers of carbon emissions in Nigeria. In doing so, we utilized a yearly dataset spanning from 1981 to 2019. The dependent variable is carbon emission CO2, which is gathered from the British Petroleum database and measured as metric tons per capita. The independent variables are GDP which is obtained from the World Bank database is and measured as GDP per capita constant 2010 USD; trade is also gathered from (World Bank 2020) database and is measured as Total export plus import (% GDP); financial depth is obtained from both the IMF and IFS databases, and it is measured as Liquid liabilities (M3 and M1) to GDP (%); stock market capitalization is obtained from the Central Bank of Nigeria database, and it is calculated as stock market capitalization; and shadow economy is obtained through author computation and is measured as the currency demand approach. Table 1 presents a summary of the variables of investigation.
For the aforementioned selected variables, this research trailed prior empirical works by Dada, et al. (2021) by incorporating stock Market capitalization and financial depth into the model. The effect of stock market capitalization, shadow economy, economic growth, trade, and financial depth on CO2 emissions is presented in Eq. 1.
where the intercept is depicted by
3.2 Methodology
3.2.1 ARDL bounds testing method
The ARDL bounds testing technique was created by Pesaran et al., (2001) to examine long-run connections among variables with a mixed integration order [I(1) or I(0)] but not I(2). The dependent variable in this approach must be I(1). The preceding unconstrained error correction model is utilized to assess the cointegration of the variables after these requirements are met.
where the difference operator is denoted by
The null hypothesis of no cointegration can be refuted if the estimated F-statistic is larger than the critical values of upper bounds determined by Pesaran et al. (2001). Otherwise, the variables do not have a long-term connection.
3.2.2 Dynamic ARDL Model
Intricate specifications, including first differences, lagged differences of variables, and various lag structures, are common in ARDL modeling. To put it in another way, determining the long- and short-run effects of regressors on the dependent variable using an ARDL model with first differences and multiple lag lengths is difficult. Jordan and Philips (2018) created dynamic ARDL (DARDL) produce, which incorporates a dynamic ECM, to reduce this burden. Predicated on the ceteris paribus idea, this approach enables the impacts of negative or positive shifts in an independent variable on the dependent variable to be quantified and visually examined (Hossain et al., 2022a, Hossain et al., 2022b, Hossain et al., 2022c; Agboola et al., 2022; Zhang et al., 2022). As a result, the DARDL framework gives a one-to-one assessment of the connection between dependent and independent variables. The following two requirements must be satisfied in order for the DARDL model to be used: the variables’ integration order must be I(1) (Jordan and Philips, 2018; Islam et al., 2022; Khan et al., 2022). Cointegration of the variables is required (Abbasi et al., 2021). The dependent variable must be I(1) in the first criterion, whereas the regressors can be I(1) and I(0) (Jordan and Philips, 2018). The DARDL model’s error correction equation is illustrated below:
where the constant term is denoted by
3.2.3 Spectral causality
The study also used spectral causality to investigate the causal relationships among variables. Instead of just observing that they are consistent when integrated across all frequencies and the time-domain Granger causality measure, we specifically try to interpret them at each frequency in terms of causal interactions between the observed processes. The lack of a spectral representation of Granger causality using simple variables from the empirical methods suggests that the autoregressive model should be explicitly considered when interpreting spectral measurements linked to Granger causality. The innovation variables in this model are inherent and have no physical significance. This makes it impossible to solve the result at each frequency by measuring how strongly the causal interactions occur within a specific frequency band. This is not to say that the spectral measures do not help understand the dynamics resulting from the causal interactions, but one should exercise caution when utilizing them to link the causative connections to particular functionally significant rhythms.
4 Empirical findings
4.1 Results of descriptive statistics
It has become necessary to analyze the econometric and descriptive attributes of all variables used in the study before ascertaining the role played by the shadow economy, financial development, and stock market performance on environmental pollution in Nigeria. Table 1 reveals that the average content of CO2 generated yearly is 0.62 metric tons, while the minimum metric ton is 0.30, the maximum value of financial depth is 15.02, and the maximum value is 8.46. The average value of the shadow economy in Nigeria is 59.80, which represents a high value, indicating that shadow-environmental pollution represents over 59.8% on the average yearly and on a minimum 42.54% yearly. Furthermore, the mean value of GDP per capita is over $1765.416, with the median as $1573.278; this shows that the GDP per capita in Nigeria is skewed to the right. Trade in Nigeria is relatively low to GDP by 32.30% on the average yearly, while on the maximum representing 53.27% of GDP in Nigeria. The mean value of stock market performance is $5584.306, while the median value represents $472.300. This implies that stock market performance in Nigeria is skewed to the right. Additionally, the standard deviation for the various variables shows that CO2 is the most stable variable within the sample period, while GDP per capita is the most widely dispersed variable from the mean. Again, CO2 and trade from the study have a negative skewness, while others have a positive skewness; all the variables in the study have a platykurtic kurtosis, given that the value of their kurtosis is below 3. The correlation matrix in Table 2 also shows that there is no case of multicollinearity that exists among the variables.
4.2 Results of unit root tests
Given that the choice estimation for the study is the ARDL approach, where it is required to ascertain the level of stationarity of all the used variables in the study and check if the variables are integrated at level or at first difference or integrated of a mixed order, while ARDL estimation breaks at the second difference. The summary of the unit root test is presented in Table 3. The Zivot and Andrews (1992) structural break unit root is presented in Table 4 where all the variables are stationary at the first difference and with structural break identified in 2000, 2002, and 1993 for CO2, economic growth, and shadow, respectively. Against the backdrop Pesaran et al., (2001) agree that the ARDL approach is unbiased, consistent, and the most preferred technique for empirical investigations.
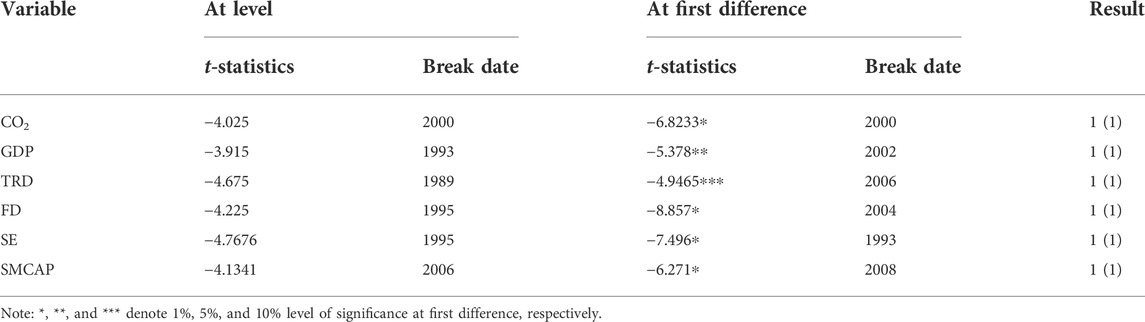
TABLE 4. Zivot and Andrews (1992) unit root test.
4.3 Results of ARDL bounds test
It has become imperative to examine the existence of a long-run relationship; the cointegrating relationship among variables should be tested. The outcome of the ARDL bound test is presented in Table 5, which revealed that there exists a long-run relationship among the variables in the study. The H0 of No Cointegration is rejected among the variables at a 5% significant level.
4.4 Results of DARDL long-run estimates
As mentioned, we proceed with the analysis to inspect the dynamic effect of FD, GDP, TRD, SE, and SMCAP on CO2 emission in Nigeria. The long-run results as presented in Table 6 unearthed that economic growth has a substantial significant and positive influence on CO2 emissions. Specifically, the findings suggest that a 1% influence on economic growth will cause carbon emissions to reduce by 0.8697%. Thus, environmental pollution increases as the activities in the economy increase in Nigeria. The results validate those of previous studies (Al-Mulali et al., 2015; Meza et al., 2021; Jahanger, 2022a). This further implies the activities in various sectors of the economy do not encourage environmental quality within the economy.
Going forward, a 1% influence in financial development could control environmental quality in Nigeria by 0.0487%. The findings agree with those of previous literature (Kamal, et al., 2021; Usman and Makhdum, 2021) and differ from those of Nasreen et al., (2017); Usman et al., (2021); Yang et al., (2022a). The findings also reveal that trade significantly hastens environmental pollution in Nigeria such that a 1% rise in trade will lead to a proportional increase in environmental pollution by 0.044% significantly. Our findings conform to those of previous studies by Destek et al., (2018); Hundie, (2018) and differ with those of Essandoh et al., (2020). The findings show that Nigeria's main imports reside in energy-related products such as gas, oil, petrol chemical, and other pollutant products, which tend to increase pollution in the environment.
Moving toward the shadow economy, the outcome indicates that the shadow economy has a statistically significant and negative correspondence with CO2 emission. More specifically, the outcome suggests that a possible change in the shadow economy will cause CO2 emissions to reduce by 0.0084% in the economy. This outcome coincides with that of Nkengfack et al. (2021). This implies that the activities of the informal sectors in the production of services and goods do not encourage environmental pollution in Nigeria. However, stock market performance in Nigeria in the long run does not significantly impact environmental pollution at all levels of significant. However, exhibiting a positive influence on CO2 implies that a 1% enhancement in stock market performance will accelerate environmental pollution by 0.0372%. This finding supports that of previous studies (Apergis et al., 2018; Meza, et al., 2021). This shows that activities in the stock market by investors encourage environmental pollution.
4.5 DARDL short-run outcomes
In order to analyze the error correction model (ECM), the ARDL technique provides the prevalence of having an order of series of 1(0) and 1(0) and a mixture of 1(0) and 1(0) where ARDL breaks in 1(2). The DARDL short-run results are presented in Table 7 where the results explore the short-run changes of carbon emission on other aforementioned variables. The ARDL short-run outcomes confirm that financial development has a positive effect on carbon emission in Nigeria such that, a 1% increase in financial development will cause carbon emissions to increase by 0.699%. In contrast, trade has a significantly negative effect on carbon emission; the outcome further revealed that a 1% influence in trade could cause carbon emission to decrease by 0.096%, which helps control the environmental pollution level in Nigeria. Moreover, the role of economic growth, shadow economy, and stock market performance is found to have an insignificant impact on carbon emission in the short run. The value of the ECM confines to theory (indicating a negative sign), with a convergence of 33.37% from the short-run to the long-run equilibrium yearly for all used variables.
4.6 Counterfactual graphs of the DARDL model
The DARDL model’s ability to simulate and predict counterfactual changes in the regressor and as a result of a shock to a regressor is one of its key characteristics. While holding all other factors constant, each figure reflects a 10% increase or reduction in the regressor and its effect on CO2 emission. Green dots indicate the anticipated value, while orange-red lines indicate a confidence interval at 75%, orange lines indicate a confidence interval at 90%, and maroon lines indicate a confidence interval at 95%. The graph’s first trend line highlights the short-term effects, while the horizontal line shows the long-term effects with time. The DARDL model’s counterfactual simulations are depicted in the figures below.
Figure 1 demonstrates that a 10% change in GDP per capita has a significant short-term impact on CO2 emissions. However, over time, a 10% increase in GDP per capita positively increases CO2 emissions, and a 10% decrease in GDP per capita negatively reduces CO2 emissions. The impact is greater over the long term than it is in the short term due to the marginal rate of rise from the baseline being larger. Similar to Figure 1, Figure 2 shows that a 10% upsurge or decline in financial development over time has little to no effect on CO2 emissions in the long term, while changes in financial development have an influence on CO2 emissions in the short term. However, because of the dotted line’s tendency to flatten out over time and remain close to the baseline, changes in financial development will not significantly affect CO2 emissions in the long run.
Following that, it can be deduced from Figure 3 that a 10% increment and decline in the shadow economy index has a considerable short- and long-term impact on CO2 emission. Despite the fact that both scenarios have a significant impact on CO2 emission, the environment can gain more from the increase in the shadow economy index by 10%, while the reduction of the shadow economy index would hamper the environmental quality. Figure 4 further shows that a 10% positive or negative shock in trade openness does not cause substantial changes in CO2 emission in the short and long run since the dotted line remains same over the time. Furthermore, Figure 5 illustrates the 10% positive and negative change in the stock market capitalization and its impact on CO2 emission in Nigeria. It is evident from the Figure that a 10% positive change in the stock market capitalization reduces the CO2 emissions and thereby improves the environmental quality in the long run. On the other hand, the reduction in the stock market capitalization is harmful for the environment since it increases emissions in the environment.
4.7 Robustness check
Table 7 also shows the summary of the ARDL diagnostic check conducted to explore the model`s efficiency, reliability, and validity. To check for the stability of the model, the cumulative sum (CUSUM) and cumulative sum of square (CUSUMsq.) test were performed to distinguish the difference in coefficients both in the short run and long run. Thus, Figure 6 sheds light on the fact that the model is well-specified as the blue line lies between the upper and lower critical bounds at a 5% level of significance. This further implies that the variables in the model are relatively stable.
4.7 Spectral causality
The findings from the spectral causality test reveal that in the long run, evidence of causality surfaced from economic growth to CO2 emissions, suggesting that the null hypothesis of “no causality” is refuted at a 10% level of significance (see Figure 7A). Furthermore, at 5% and 10% levels of significance, the null hypothesis of “no causality” is dismissed, which implies that financial development can predict CO2 emissions in the long term (see Figure 7B). On the contrary, in the short and medium term, we found support for the causality running from a shadow economy to CO2 emissions in both the short and medium term at a significance level of 10% (see Figure 7C). Moreover, in the short, medium, and long term, stock market Granger causes CO2 emissions at 5% and 10% levels of significance, suggesting that any policy in the short, medium, and long term directed toward the stock market will impact CO2 emissions (see Figure 7D). Last, evidence of causality surfaced from trade openness to CO2 emissions, suggesting that the null hypothesis of “no causality” is refuted at a 10% level of significance in all frequencies (see Figure 7E). Based on these findings, policymakers in this country should consider these variables when drafting policies regarding CO2 emissions as any shift in these variables will impact CO2 emissions.
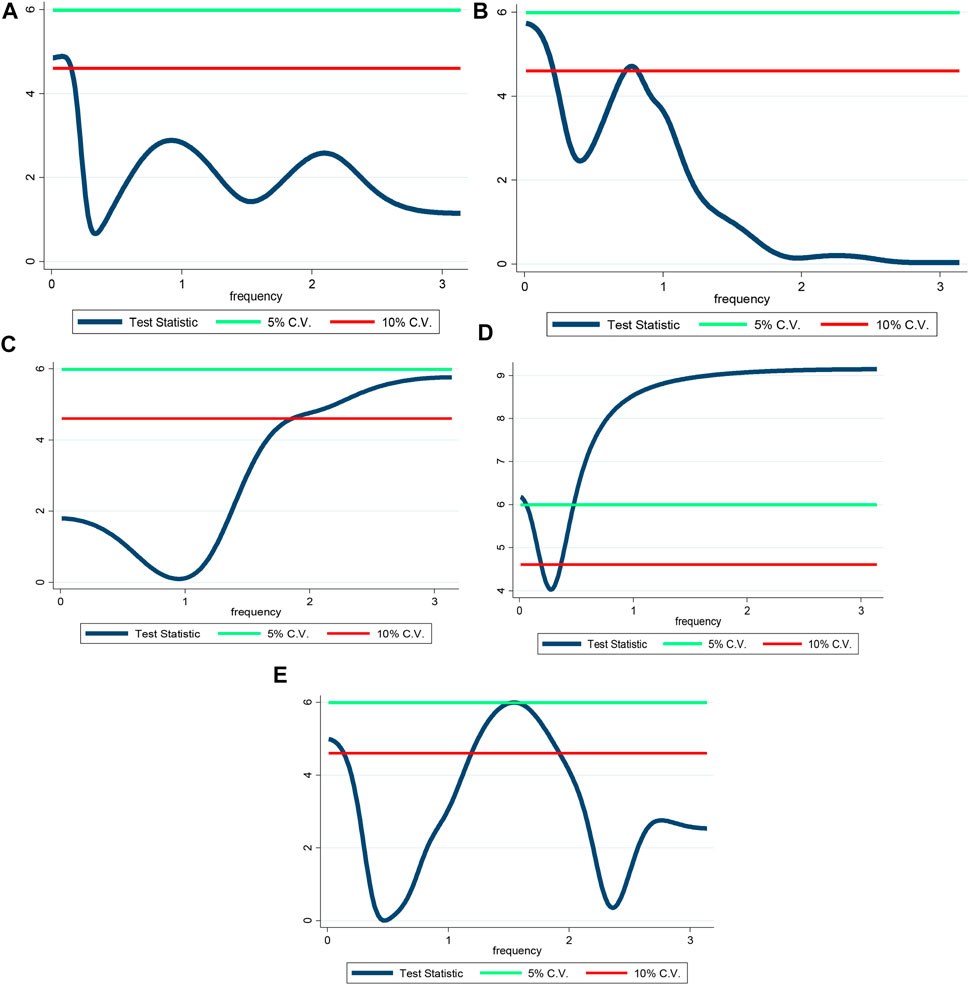
FIGURE 7. (A) Spectral causality from economic growth to carbon emission. (B) Spectral causality from financial development to carbon emission. (C). Spectral causality from shadow economy to carbon emission. (D) Spectral causality from stock market performance to carbon emission. (E) Spectral causality from trade to carbon emission.
5 Discussion of findings
The empirical outcomes of the study are elucidated in this section, with a more in-depth and detailed discussion based on the practical repercussion of the findings. The study adopted the use of CO2 emission as an indicator for environmental pollution. Exploring the empirical findings in the context of economic growth, they found that there is a substantial significant and positive relationship of economic growth on environmental pollution in Nigeria. This finding is consistent with the recent studies by Abbasi and Shahbaz, (2021); Jahanger et al., (2021); Khalid et al., (2021); Usman et al., (2021). This finding further implies that the economy of Nigeria is fast growing at the cost of the environment. Again, being an oil producing country, it is expected that there will be a high level of energy consumption and large importation of fossil fuels which is used to increase economic activities within the various productive sectors, and this perceived growth in the GDP growth rate through increased economic activities tends to affect the environment upon which the resultant effect is increase in carbon emission and gross environmental pollution. Again, the effect of the shadow economy which represents the activities of the informal sectors of the economy revealed that the shadow economy has a negative and significant impact on the environment. This finding is in line with that of new studies Nkengfack et al. (2021) that based on the unrecognized activities of the informal sector by the government where this sector has exempted itself from high taxes of government, social security contributions, and heavy regulation, and it is based on this new norms that the influence of this sector to the economy with respect to environmental pollution is relatively low and negative.
Establishing the environmental influence of financial development, and stock market performance on carbon emission in Nigeria based on empirical finding is revealed to have a positive influence on environmental pollution over the long-run period. This established findings conforms to those of new studies by Ahmad et al., (2021) and differs with those of Assi et al., (2020; Usman et al., (2021). Unarguably, the Nigeria financial sector does not have a well-organized financial institution and a stock market that can motivate environmental sustainability through the employment of stringent regulations that will increase funding to the productive sectors of the economy to adopt the installation of renewable energy and green technologies in Nigeria, where this gesture will steel up advocacy through the financial system and stock market in reducing the environmental pollution in Nigeria. Moving toward the impact of trade on environmental pollution is both positive and statistically significant in Nigeria. This finding agrees with that of previous studies (Al-Mulali et al., 2015; Le, et al., 2016; Twerefou et al., 2017) where trade openness facilitates environmental damages to the economy. The Nigerian economy holds oil and fossil fuel electrical, electronic, and fuel-powered vehicles as its main imports, and the trade tends to elevate environmental pollution in Nigeria.
6 Conclusion, policy implication, and further scope
This study examines the impression of economic growth, financial development, trade, stock market performance, and shadow economy on environmental pollution from 1981 to 2019 in Nigeria. The unit root outcomes signified that all variables follow the same integration order. The study, therefore, used the ARDL method to estimate both the long–run and dynamic short-run among all used variables in the study. The ARDL bound test confirms the existence of a long-run relationship among the concerned variables. The dynamic short-run empirical outcomes revealed that financial development and trade significantly impact environmental pollution in Nigeria, with financial development having a positive influence on carbon emission, while trade improves environmental sustainability in Nigeria in the short run. Other variables such as economic growth, shadow economy, and stock market performance do not significantly impact environmental pollution in Nigeria in the short term. The error correction model suggests the convergence of 33.3% from the short-run to the long-run equilibrium yearly. The ARDL long-run empirical results revealed that economic growth, trade, financial development, and stock market performance significantly increase environmental pollution, with a 1% impact of economic growth, trade, financial development, and stock market performance causing a substantial increase in environmental pollution by 0.867%, 0.044%, 0.048%, and 0.0372%, respectively.
Furthermore, the long-run outcomes revealed that the shadow economy significantly improves environmental sustainability and quality in Nigeria. Specifically, a 1% increase in the shadow economy will cause carbon emissions to reduce by 0.0084%. Moreover, the spectral Granger causality test revealed that there is a unidirectional causality that exists from economic growth to carbon emission, from trade to carbon emission, from trade to carbon emission, from shadow economy to carbon emission, from financial development to carbon emission, and from stock market performance to carbon emission in Nigeria.
The empirical findings of this study and its policy implications are as follows: the study found that financial development and stock market performance have a positive impact on environmental pollution issues in Nigeria. This further reveals that the activities of the financial institution and stock market in Nigeria are aimed at and worsen environmental sustainability in Nigeria. This implies that policy makers within the financial climate and stock market should bring out stringent measures that will cause a financial institution to invest in renewable and green energy. This can be carried out through loan and credit support to the productive sectors of the economy, thereby encouraging them to cultivate the use of green technologies that will curb environmental pollution in Nigeria.
Economic growth has a positive and significant effect on environmental pollution in Nigeria both in the long-run and short-run period. This implies that growth in GDP is detrimental to the environment. This implies that the government of Nigeria employs an efficient-energy scheme that can promote a green economy; this can also be carried out by encouraging renewable energy installations for energy use while encouraging local investors and manufacturers to adopt eco-friendly energy means in production as this will curb further pollution to the environment in Nigeria. Shadow economy is negative but significant to carbon emissions in Nigeria. This outcome suggests that shadow economy accelerates environmental quality in Nigeria; this finding implies that the shadow economy in Nigeria is not yet familiarized with the formal sector; this could be due to high taxes and social security contributions, among others. However, to sustain the environmental quality of the informal sector, the government should formalize green-based economy where the size of the shadow economy will be included while the government enforces environmental laws that will be effective for the informal sector, thereby promoting a sustained economy. Again, the study discovered a unidirectional causality from trade to carbon emission in Nigeria. This implies that trade is useful to environmental quality in Nigeria. This study recommends that government should promote the trade of eco-friendly products into the economy and also increase tariffs on goods that will adversely affect environmental quality in Nigeria.
Further research can supplement this study in the following ways by considering the role of energy use and utilization, shadow economy, financial development, and stock market performance on carbon emission. Again, considering the role of institutional quality and another normative antecedent of environmental pollution, future studies can adopt the ARDL quantile regression or the nonlinear autoregressive distributed lag (NARDL) technique for analysis.
Data availability statement
The original contributions presented in the study are included in the article/Supplementary Material; further inquiries can be directed to the corresponding authors.
Author contributions
YY and JC: Conceptualization, formal writing, investigation and software writing; AJ and YY: formal writing, revised and reviewed; TA: formal writing, Supervision, software writing and investigation. MH: conceptualization, formal writing, software writing and editing; AD: data curation, formal writing; YY: Project Administration; All authors have read and agreed to the published version of the manuscript.
Funding
This work is supported by the fundamental research funds for the Project supported by the Education Department of Hainan Province, project number: Hnky 2022-11; Hainan Provincial Philosophy and Social Science 2021 Planning Project, project number: HNSK (JD)21-16; the Research Startup Fund of Hainan University, project number: kyqd (sk) 2022008 and Research Startup Fund of Hainan University, project number: kyqd (sk) 2022011.
Conflict of interest
The authors declare that the research was conducted in the absence of any commercial or financial relationships that could be construed as a potential conflict of interest.
Publisher’s note
All claims expressed in this article are solely those of the authors and do not necessarily represent those of their affiliated organizations, or those of the publisher, the editors, and the reviewers. Any product that may be evaluated in this article, or claim that may be made by its manufacturer, is not guaranteed or endorsed by the publisher.
References
Abbasi, K. R., Hussain, K., Redulescu, M., and Ozturk, I. (2021). Does natural resources depletion and economic growth achieve the carbon neutrality target of the UK? A way forward towards sustainable development. Resour. Policy 74, 102341. doi:10.1016/j.resourpol.2021.102341
Abbasi, K., Shahbaz, M. J., Jiao, Z., and Tufail, M. (2021). How energy consumption, industrial growth, urbanization and CO2 emissions affect economic growth in Pakistan? A novel dynamic ARDL simulations approach. Energy 221, 119793. doi:10.1016/j.energy.2021.119793
Abid, M. (2015). The close relationship between informal economic growth and carbon emissions in Tunisia since 1980: The (ir)relevance of structural breaks. Sustain. Cities Soc. 15, 11–21. doi:10.1016/j.scs.2014.11.001
Acheampong, A., Amponsah, M., and Elliot, B. (2020). Does financial development mitigate carbon emissions? Evidence from heterogeneous financial economies. Energy Econ. 88, 104768. doi:10.1016/j.eneco.2020.104768
Acheampong, A. (2019). Modelling for insight: Does financial development improve environmental quality? Energy Econ. 83, 156–179. doi:10.1016/j.eneco.2019.06.025
Adebayo, T., Awosusi, A., and Adeshola, I. (2020). Determinants of CO2 emissions in emerging markets: An empirical evidence from MINT economies. Int. J. Renew. Energy Dev. 9, 411–422. doi:10.14710/ijred.2020.31321
Adebayo, T., Haouas, I., Akadiri, S., and Rjoub, H. (2022). A time-varying analysis between financial development and carbon emissions: Evidence from the MINT countries. Energy Environ. doi:10.1177/0958305X221082092
Adebayo, T., and Kalmaz, D. (2021). Determinants of CO2 emissions: Empirical evidence from Egypt. Environ. Ecol. Stat. 28, 239–262. doi:10.1007/s10651-020-00482-0
Adebayo, T. (2020). Revisiting the EKC hypothesis in an emerging market: An application of ARDL-based bounds and wavelet coherence approaches. SN Appl. Sci. 2, 1945–2015. doi:10.1007/s42452-020-03705-y
Adebayo, T. S., Oladipupo, S. D., Adeshola, I., and Rjoub, H. (2021). Wavelet analysis ofimpact of renewable energy consumption and technological innovation on CO2 emissions: Evidence from Portugal. Environ. Sci. Pollut. Res. 29, 23887–23904. doi:10.1007/s11356-021-17708-8
Agboola, M. O., and Bekun, F. V. (2019). Does agricultural value added induce environmental degradation? Empirical evidence from an agrarian country. Environ. Sci. Pollut. Res. 26 (27), 27660–27676. doi:10.1007/s11356-019-05943-z
Agboola, P. O., Hossain, M., Gyamfi, B. A., and Bekun, F. V. (2022). Environmental consequences of foreign direct investment influx and conventional energy consumption: Evidence from dynamic ARDL simulation for Turkey. Environ. Sci. Pollut. Res. 29, 53584–53597. doi:10.1007/s11356-022-19656-3
Ahmad, M., Ahmed, Z., Yang, X., Hussain, N., and Sinha, S. (2021). Financial development and environmental degradation: Do human capital and institutional quality make a difference? Gondwana Res. 105, 299–310. doi:10.1016/j.gr.2021.09.012
Ahmad, U. S., Usman, M., Hussain, S., Jahanger, A., and Abrar, M. (2022). Determinants of renewable energy sources in Pakistan: An overview. Environ. Sci. Pollut. Res. 29, 29183–29201. doi:10.1007/s11356-022-18502-w
Al-Mulali, U., Ozturk, I., and Lean, H. (2015). The influence of economic growth, Urbanization, trade openness, financial development and renewable energy on pollution in Europe. Nat. Hazards (Dordr). 79, 621–644. doi:10.1007/s11069-015-1865-9
Apergis, N., Paramati, S., and Alam, M. (2018). The role of stock marketson environmental degradation; A comparative study of developed and emerging market economies across the globe. Emerg. Mark. Rev. 35, 19–30. doi:10.1016/j.ememar.2017.12.004
Assi, A., Isiksal, A., and Tursoy, T. (2020). Highlighting the connection between financial development and consumption of energy in countries with the highest economic freedom. Energy Policy 147, 111897. doi:10.1016/j.enpol.2020.111897
Baloch, A., Shah, S., Rasheed, S., and Rasheed, S. (2021). The impact of shadow economy on environmental degradation: Empirical evidence from Pakistan. GeoJournal 87, 1887–1912. doi:10.1007/s10708-020-10354-6
Bekun, F. V., Alola, A. A., and Sarkodie, S. A. (2019). Toward a sustainable environment: Nexus between CO2 emissions, resource rent, renewable and nonrenewable energy in 16-EU countries. Sci. Total Environ. 657, 1023–1029. doi:10.1016/j.scitotenv.2018.12.104
Bilal, A., Li, X., Zhu, N., Sharma, R., and Jahanger, A. (2021). Green technology innovation, globalization, and CO2 emissions: Recent insights from the OBOR economies. Sustainability 14 (1), 236. doi:10.3390/su14010236
Bolton, P., and Kacperczyk, M. (2021). Do investors care about carbon risk? J. Financial Econ. 142, 517–549. doi:10.1016/j.jfineco.2021.05.008
Byrd, J., and Cooperman, E. (2018). Investors and stranded asset risk: Evidence from shareholder responses to carbon capture and sequestration (CCS) events. J. Sustain. Finance Invest. 8, 185–202. doi:10.1080/20430795.2017.1418063
Camara, M. (2022). The impact of the shadow economy on economic growth and CO2 emissions: Evidence from ECOWAS countries. Environ. Sci. Pollut. Res. Int. 1, 16. doi:10.1007/s11356-022-20360-5
Canh, N. T., Schinckus, C., Bensemann, J., and Thanh, L. (2019). Global emissions: A new contribution from the shadow economy. Int. J. Energy Econ. Policy 9 (3), 320–337. doi:10.32479/ijeep.7244
Chen, H., Hao, Y., Li, J., and Song, X. (2018). The impact of environmental regulation, shadow economy, and corruption on environmental quality: Theory and empirical evidence from China. J. Clean. Prod. 195, 200–214. doi:10.1016/j.jclepro.2018.05.206
Chnag, C., Ilomäki, J., Laurila, H., and McAleer, M. (2020). Causality between CO2 emissions and stock markets. Energies 13, 2893. doi:10.3390/en13112893
Dada, J. T., Ajide, F. M., and Sharimakin, A. (2021). Shadow economy, institutions and environmental pollution: Insights from Africa. World J. Sci. Technol. Sustain. Dev. 18, 153–171. doi:10.1108/WJSTSD-12-2020-0105
Destek, M., Ulucak, R., and Dogan, E. (2018). Analyzing the environmental Kuznets curve for the E.U. countries: The role of ecological footprint. Environ. Sci. Pollut. Res. 25 (29), 29387–29396. doi:10.1007/s11356-018-2911-4
Essandoh, O., Islam, M., and Kakinaka, M. (2020). Linking international trade and foreign direct investment to CO2 emissions: Any diferences between developed and developing countries? Sci. Total Environ. 712, 136437. doi:10.1016/j.scitotenv.2019.136437
Gök, A. (2020). The role of financial development on carbon emissions: A meta regression analysis. Environ. Sci. Pollut. Res. 22, 11618–11636. doi:10.1007/s11356-020-07641-7
Hossain, M. E., Islam, M. S., Bandyopadhyay, A., Awan, A., Hossain, M. R., and Rej, S. (2022b). Mexico at the crossroads of natural resource dependence and COP26 pledge: Does technological innovation help? Resour. Policy 77, 102710. doi:10.1016/j.resourpol.2022.102710
Hossain, M., Islam, M., Sujan, M., Khan, H., Tuhin, M., and Bekun, F. V. (2022c). Towards a clean production by exploring the nexus between agricultural ecosystem and environmental degradation using novel dynamic ARDL simulations approach. Environ. Sci. Pollut. Res. 29, 53768–53784. doi:10.1007/s11356-022-19565-5
Hossain, M., Rej, S., Saha, S. M., Onwe, J. C., Nwulu, N., Bekun, F. V., et al. (2022a). Can energy efficiency help in achieving carbon-neutrality pledges? A developing country perspective using dynamic ARDL simulations. Sustainability 14 (13), 7537. doi:10.3390/su14137537
Hundie, S. (2018). Modelling energy consumption, carbon dioxide emissions and economic growth nexus in Ethiopia: Evidence from cointegration and causality analysis. Turk. JAF. Sci. Tech. 699, 1720. doi:10.24925/turjaf.v6i6.699-709.1720
Imamoglu, H. (2018). Is the informal economic activity a determinant of environmental quality? Environ. Sci. Pollut. Res. 25 (29), 29078–29088. doi:10.1007/s11356-018-2925-y
Islam, M., Hossain, M., Khan, M., Rana, M., Ema, N. S., and Bekun, F. V. (2022). Heading towards sustainable environment: Exploring the dynamic linkage among selected macroeconomic variables and ecological footprint using a novel dynamic ARDL simulations approach. Environ. Sci. Pollut. Res. 29 (15), 22260–22279. doi:10.1007/s11356-021-17375-9
Jaggi, B., Allini, A., Richardo, M., and Zampella, A. (2018). Do investors find carbon information useful? Evidence from Italian firms. Rev. Quantitative Finance Account. 50, 1031–1056. doi:10.1007/s11156-017-0653-x
Jahanger, A. (2022a). Impact of globalization on CO2 emissions based on EKC hypothesis in developing world: The moderating role of human capital. Environ. Sci. Pollut. Res. 29 (14), 20731–20751. doi:10.1007/s11356-021-17062-9
Jahanger, A. (2021a). Influence of FDI characteristics on high-quality development of China’s economy. Environ. Sci. Pollut. Res. 28 (15), 18977–18988. doi:10.1007/s11356-020-09187-0
Jahanger, A., Usman, M., and Ahmed, P. (2021). A step towards sustainable path: The effect of globalization on China’s carbon productivity from panel threshold approach. Environ. Sci. Pollut. Res. 29, 8353–8368. doi:10.1007/s11356-021-16317-9
Jahanger, A., Usman, M., and Balsalobre‐Lorente, D. (2021a). Autocracy, democracy, globalization, and environmental pollution in developing world: Fresh evidence from STIRPAT model. J. Public Aff., e2753. doi:10.1002/pa.2753
Jahanger, A., Usman, M., Murshed, M., Mahmood, H., and Balsalobre-Lorente, D. (2022a). The linkages between natural resources, human capital, globalization, economic growth, financial development, and ecological footprint: The moderating role of technological innovations. Resour. Policy 76, 102569. doi:10.1016/j.resourpol.2022.102569
Jahanger, A., Yang, B., Huang, W. C., Murshed, M., Usman, M., and Radulescu, M. (2022b). Dynamic linkages between globalization, human capital, and carbon dioxide emissions: Empirical evidence from developing economies. Environ. Dev. Sustain. 1, 29. doi:10.1007/s10668-022-02437-w
Jahanger, A., Yu, Y., Awan, A., Chishti, M. Z., Radulescu, M., and Balsalobre-Lorente, D. (2022c). The impact of hydropower energy in Malaysia under the EKC hypothesis: Evidence from quantile ARDL approach. SAGE Open 12 (3), 215824402211095. doi:10.1177/21582440221109580
Jiang, C., and Ma, X. (2019). The impact of financial development on carbon emissions: A global perspective. Sustainability 11 (19), 5241. doi:10.3390/su11195241
Jiang, T., Li, S., Yu, Y., and Peng, Y. (2022c). Energy-related carbon emissions and structural emissions reduction of China’s construction industry: The perspective of input–output analysis. Environ. Sci. Pollut. Res. 29 (26), 39515–39527. doi:10.1007/s11356-021-17604-1
Jiang, T., Song, J., and Yu, Y. (2022d). The influencing factors of carbon trading companies applying blockchain technology: Evidence from eight carbon trading pilots in China. Environ. Sci. Pollut. Res. 29 (19), 28624–28636. doi:10.1007/s11356-021-18425-y
Jiang, T., Yu, Y., Jahanger, A., and Balsalobre-Lorente, D. (2022a). Structural emissions reduction of China's power and heating industry under the goal of" double carbon": A perspective from input-output analysis. Sustain. Prod. Consum. 31, 346–356. doi:10.1016/j.spc.2022.03.003
Jiang, T., Yu, Y., and Yang, B. (2022b). Understanding the carbon emissions status and emissions reduction effect of China’s transportation industry: Dual perspectives of the early and late stages of the economic “new normal”. Environ. Sci. Pollut. Res. 29 (19), 28661–28674. doi:10.1007/s11356-021-18449-4
Jordan, S., and Philips, A. Q. (2018). Cointegration testing and dynamic simulations of autoregressive distributed lag models. Stata J. 18 (4), 902–923. doi:10.1177/1536867X1801800409
Ke, J., Jahanger, A., Yang, B., Usman, M., and Ren, F. (2022). Digitalization, financial development, trade, and carbon emissions; implication of pollution haven hypothesis during globalization mode. Front. Environ. Sci. 211, 873880. doi:10.3389/fenvs.2022.873880
Khalid, K., Usman, M., and Mehdi, M. (2021). The determinants of environmental quality in the SAARC region: A spatial heterogeneous panel data approach. Environ. Sci. Pollut. Res. 28 (6), 6422–6436. doi:10.1007/s11356-020-10896-9
Khan, M. A., Hossain, M. E., Islam, M. S., Rahman, M. T., and Dey, M. M. (2022). Shrimp export competitiveness and its determinants: A novel dynamic ARDL simulations approach. Aquac. Econ. Manag. 1, 28. doi:10.1080/13657305.2022.2089772
Khan, S., Muhammad, K., and Bashir, M. (2021). Impact of financial development and energy consumption on environmental degradation in 184 countries using a dynamic panel model. Environ. Sci. Pollut. Res. 28, 9542–9557. doi:10.1007/s11356-020-11239-4
Khaskheli, A., Jiang, Y., Raza, S. A., Khan, K. A., and Qureshi, M. A. (2021). Financial development, international trade, and environmental degradation: A nonlinear threshold model based on panel smooth transition regression. Environ. Sci. Pollut. Res. 28 (21), 26449–26460. doi:10.1007/s11356-020-11912-8
Kirikkaleli, D., Güngör, H., and Adebayo, T. S. (2021). Consumption‐based carbon emissions, renewable energy consumption, financial development and economic growth in Chile. Bus. Strategy Environ. 31, 1123–1137. doi:10.1002/bse.2945
Kirikkaleli, D., and Kalmaz, D. (2020). Testing the moderating role of urbanization on the environmental kuznets curve: Empirical evidence from an emerging market. Environ. Sci. Pollut. Res. 27, 38169–38180. doi:10.1007/s11356-020-09870-2
Le, T., Chang, Y., and Park, C. (2016). Trade openness and environmental quality: International evidence. Energy Policy 92, 45–55. doi:10.1016/j.enpol.2016.01.030
Lee, S., Yun-Seon, P., and Robert, D. (2015). Market responses to firms’ voluntary climate change information disclosure and carbon communication. Corp. Soc. Responsib. Environ. Manag. 22, 1–12. doi:10.1002/csr.1321
Li, G., and Wei, W. (2021). Financial development, openness, innovation, carbon emissions, and economic growth in China. Energy Econ. 97, 105194. doi:10.1016/j.eneco.2021.105194
Li, S., Yu, Y., Jahanger, A., Usman, M., and Ning, Y. (2022). The impact of green investment, technological innovation, and globalization on CO2 emissions: Evidence from MINT countries. Front. Environ. Sci. 156, 868704. doi:10.3389/fenvs.2022.868704
Long, X., Chen, Y., Du, J., Oh, K., and Han, I. (2017). Environmental innovation and its impact on economic and environmental performance: Evidence from Korean-owned firms in China. Energy Policy 107, 131–137. doi:10.1016/j.enpol.2017.04.044
Long, X., Naminse, E. Y., Du, J., and Zhuang, J. (2015). Nonrenewable energy, renewable energy, carbon dioxide emissions and economic growth in China from 1952 to 2012. Renew. Sustain. Energy Rev. 52, 680–688. doi:10.1016/j.rser.2015.07.176
Long, X., Wu, C., Zhang, J., and Zhang, J. (2018). Environmental efficiency for 192 thermal power plants in the yangtze river delta considering heterogeneity: A metafrontier directional slacks-based measure approach. Renew. Sustain. Energy Rev. 82, 3962–3971. doi:10.1016/j.rser.2017.10.077
Maâlej, A., and Cabagnols, A. (2020). CO2 emissions and growth: Exploring the nexus between renewable energies, economic activity, and technology. J. Energy Dev. 46 (1/2), 1–24. AvaliableAt: https://www.jstor.org/stable/27107166.
Maduka, A. C., Ogwu, S. O., and Ekesiobi, C. S. (2022). Assessing the moderating effect of institutional quality on economic growth—Carbon emission nexus in Nigeria. Environ. Sci. Pollut. Res. Int. 1, 15. doi:10.1007/s11356-022-20346-3
Matsumura, E., Rachna, P., and Vera-Muñoz, S. (2014). Firm-value effects of carbon emissions and carbon disclosures. Account. Rev. 89, 695–724. doi:10.2308/accr-50629
Mazhar, U., and Elgin, C. (2013). Environmental regulation, pollution and the informal economy. SBP Res. Bull. 9, 62–81.
Medina, L., and Schneider, M. F. (2018). Shadow economies around the world: What did we learn over the last 20 years?. International Monetary Fund. Available at: https://www.imf.org/en/Publications/WP/Issues/2018/01/25/Shadow-Economies-Around-the-World-What-Did-We-Learn-Over-the-Last-20-Years-45583.
Meza, C., Jain, M. K., Guerrero, J., Roopchund, R., Niedbala, G., The, C., et al. (2021). Stock markets dynamics and environmental pollution: Emerging issues and policy options in asia. Environ. Sci. Pollut. Res. 28, 61801–61810. doi:10.1007/s11356-021-15116-6
Middleton, A. (2015). Value relevance of firms’ integral environmental performance: Evidence from Russia. J. Account. Public Policy 34, 204–211. doi:10.1016/j.jaccpubpol.2014.12.001
Nasreen, S., Anwar, S., and Ozturk, I. (2017). Financial stability, energy consumption and environmental quality: Evidence from South Asian economies. Renew. Sustain. Energy Rev. 67, 1105–1122. doi:10.1016/j.rser.2016.09.021
Nguyen, D., Huynh, T., and Nasir, M. (2021). Carbon emissions determinants and forecasting: Evidence from G6 countries. J. Environ. Manag. 285, 111988. doi:10.1016/j.jenvman.2021.111988
Nkengfack, H., Kaffo Fotio, H., and Totouom, A. (2021). How does the shadow economy affect environmental quality in sub-saharan Africa? Evidence from heterogeneous panel estimations. J. Knowl. Econ. 12 (4), 1635–1651. doi:10.1007/s13132-020-00685-7
Nondo, C., and Kahsai, M. (2020). The impact of energy intensity, urbanisation, industrialisation, and income on CO<SUB align=right>2 emissions in South Africa: An ARDL bounds testing approach. Afr. J. Econ. Sustain. Dev. 7, 307–330. doi:10.1504/AJESD.2020.106826
Odugbesan, J., and Adebayo, T. (2020). The symmetrical and asymmetrical effects of foreign direct investment and financial development on carbon emission: Evidence from Nigeria. SN Appl. Sci. 2, 1982–2015. doi:10.1007/s42452-020-03817-5
Pesaran, M., Shin, Y., and Smith, R. (2001). Bounds testing approaches to the analysis of level relationships. J. Appl. Econ. Chichester. Engl. 16, 289–326. doi:10.1002/jae.616
Plumlee, M., Darrell, B., Hayes, R., and Marshall, R. (2015). Voluntary environmental disclosure quality and firm value: Further evidence. J. Account. Public Policy 34, 336–361. doi:10.1016/j.jaccpubpol.2015.04.004
Prastiyo, S., Irham, S., Hardyastuti, J., and Jamhari, I. (2020). How agriculture, manufacture, and urbanization induced carbon emission? The case of Indonesia. Environ. Sci. Pollut. Res. 27, 42092–42103. doi:10.1007/s11356-020-10148-w
Qayyum, M., Ali, M., Nizamani, M. M., Li, S., Yu, Y., and Jahanger, A. (2021). Nexus between financial development, renewable energy consumption, technological innovations and CO2 emissions: The case of India. Energies 14 (15), 4505. doi:10.3390/en14154505
Qiang, O., Tian-Tian, W., Ying, D., Zhu-Ping, L., and Jahanger, A. (2022). The impact of environmental regulations on export trade at provincial level in China: Evidence from panel quantile regression. Environ. Sci. Pollut. Res. 29 (16), 24098–24111. doi:10.1007/s11356-021-17676-z
Razmi, S., Ramezanian, B., Behname, M., salari, T., and Javad Razmi, S. (2019). The relationship of renewable energy consumption to stock market development and economic growth in Iran. Renew. Energy 145, 2019–2024. doi:10.1016/j.renene.2019.06.166
Sadorsky, P. (2010). The impact of financial development on energy consumption in emerging economies. Energy policy 38 (5), 2528–2535. doi:10.1016/j.enpol.2009.12.048
Sahail, M., Ullah, S., Majeed, M., Usman, A., and Andlib, Z. (2021). The shadow economy in South asia: Dynamic effects on clean energy consumption and environmental pollution. Environ. Sci. Pollut. Res. Int. 28 (23), 29265–29275. doi:10.1007/s11356-021-12690-7
Shao, J., Tillaguango, B., Alvarado, R., Ochoa-Moreno, S., and Alvarado-Espejo, J. (2021). Environmental impact of the shadow economy, globalisation, trade and market size: Evidence using linear and non-linear methods. Sustainability 13 (12), 6539. doi:10.3390/su13126539
Shen, Y., Zhi-wei, S., Muhammad, Y., Umar, M., Zeeshan, K., and Khan, M. (2021). Does green investment, financial development and natural resources rent limit carbon emissions? A provincial panel analysis of China. Sci. Total Environ. 755, 142538. doi:10.1016/j.scitotenv.2020.142538
Shobande, O., and Asongu, S. (2021). The rise and fall of the energy-carbon Kuznets curve: Evidence from Africa. Manag. Environ. Qual. Int. J. 33, 390–405. doi:10.1108/meq-08-2021-0185
Shobande, O., and Ogbeifun, L. (2022). Sustainable blueprint: Do stock investors increase emissions? J. Risk Financ. Manag. 15, 70, doi:10.3390/jrfm15020070
Tamazian, A., Chousa, J. P., and Vadlamannati, K. C. (2009). Does higher economic and financial development lead to environmental degradation: Evidence from BRIC countries. Energy policy 37 (1), 246–253. doi:10.1016/j.enpol.2008.08.025
Teng, J., Khan, M., Khan, M., Chishti, M., and Khan, M. (2020). Effect of foreign direct investment on CO2 emission with the role of globalization, institutional quality with pooled mean group panel ARDL. Environ. Sci. Pollut. Res. 28, 5271–5282. doi:10.1007/s11356-020-10823-y
Tsaurai, K. (2019). The impact of financial development on carbon emissions in Africa. Int. J. Energy Econ. Policy 9, 144–153. doi:10.32479/ijeep.7073
Twerefou, D. K., Danso-Mensah, K., and Bokpin, G. A. (2017). The environmental effects of economic growth and globalization in Sub-Saharan Africa: A panel general method of moments approach. Res. Int. Bus. Finance 42, 939–949. doi:10.1016/j.ribaf.2017.07.028
Usman, M., Anwar, S., Yaseen, M. R., Makhdum, M. S. A., Kousar, R., and Jahanger, A. (2021a). Unveiling the dynamic relationship between agriculture value addition, energy utilization, tourism and environmental degradation in South Asia. J. Public Aff., e2712. doi:10.1002/pa.2712
Usman, M., Balsalobre-Lorente, D., Jahanger, A., and Ahmad, P. (2022b). Pollution concern during globalization mode in financially resource-rich countries: Do financial development, natural resources, and renewable energy consumption matter? Renew. Energy 183, 90–102. doi:10.1016/j.renene.2021.10.067
Usman, M., and Jahanger, A. (2021). Heterogeneous effects of remittances and institutional quality in reducing environmental deficit in the presence of EKC hypothesis: A global study with the application of panel quantile regression. Environ. Sci. Pollut. Res. 28 (28), 37292–37310. doi:10.1007/s11356-021-13216-x
Usman, M., Jahanger, A., Makhdum, M. S. A., Balsalobre-Lorente, D., and Bashir, A. (2022a). How do financial development, energy consumption, natural resources, and globalization affect arctic countries' economic growth and environmental quality? An advanced panel data simulation. Energy 241, 122515. doi:10.1016/j.energy.2021.122515
Usman, M., Jahanger, A., Radulescu, M., and Balsalobre-Lorente, D. (2022c). Do nuclear energy, renewable energy, and environmental-related technologies asymmetrically reduce ecological footprint? Evidence from Pakistan. Energies 15 (9), 3448. doi:10.3390/en15093448
Usman, M., Kousar, R., Makhdum, M., Yaseen, M., and Nadeem, A. (2021). Do fnancial development, economic growth, energy consumption, and trade openess contribute to increase carbon emission in Pakistan? An insignt based on ARDL bound testing approach. Environ. Dev. Sustain. doi:10.1007/s10668-021-02062-z
Usman, M., and Makhdum, M. (2021). What abate ecological footprints in BRICS-T region/Exploring the influence of renewable energy, non-renewable energy, agriculture, forest and financial development. Renew. Energy 179, 12–28. doi:10.1016/j.renene.2021.07.014
Vo, A., Vo, D., and Le, Q. (2019). CO2 emissions, energy consumption, and economic growth: New evidence in the ASEAN countries. J. Risk Financ. Manag. 12, 145. doi:10.3390/jrfm12030145
Wan, X., Jahanger, A., Usman, M., Radulescu, M., Balsalobre-Lorente, D., and Yu, Y. (2022). Exploring the effects of economic complexity and the transition to a clean energy pattern on ecological footprint from the Indian perspective. Front. Environ. Sci. 9, 736. doi:10.3389/fenvs.2021.816519
Wang, S., Wang, X., and Lu, B. (2022b). Is resource abundance a curse for green economic growth? Evidence from developing countries. Resour. Policy 75, 102533. doi:10.1016/j.resourpol.2021.102533
Wang, S., Yu, Y., Jiang, T., and Nie, J. (2022a). Analysis on carbon emissions efficiency differences and optimization evolution of China’s industrial system: An input-output analysis. Plos one 17 (3), e0258147. doi:10.1371/journal.pone.0258147
Wang, Z., Rasool, Y., Zhang, B., Ahmed, B., and Wang, B. (2019). Dynamic linkage among industrialisation, urbanisation, and CO2 emissions in APEC realms: Evidence based on DSUR estimation. Struct. Change Econ. Dyn. 52, 382–389. doi:10.1016/j.strueco.2019.12.001
Whiting, K. (2019). 5 facts to know about Africa's powerhouse – Nigeria. AvaliableAt: https://www.weforum.org/agenda/2019/08/nigeria-africa-economy/.
World Bank (2020). Enterprise surveys: What business experience? AvaliableAt: https://www.enterprisesurveys.org/en/data/exploretopics/informality.
World Bank (2021). Seven countries account for two-thirds of global gas flaring. AvaliableAt: https://www.worldbank.org/en/news/press-release/2021/04/28/seven-countries-account-for-two-thirds-of-global-gas-flaring.
Xiong, L., Zhiyong, T., and Lan, J. (2017). Reconciling regional differences in financial development and carbon emissions: A dynamic panel data approach. Energy Procedia 105, 2989–2995. doi:10.1016/j.egypro.2017.03.716
Xu, M., Meng, D., Zhang, J., and Mahmood, Z. (2018). Nexus between financial development and CO2 emissions in Saudi Arabia: Analyzing the role of globalization. Environ. Sci. Pollut. Res. 25, 28378–28390. doi:10.1007/s11356-018-2876-3
Yang, B., Ali, M., Hashmi, S. H., and Jahanger, A. (2022a). Do income inequality and institutional quality affect CO2 emissions in developing economies? Environ. Sci. Pollut. Res. 29 (28), 42720–42741. doi:10.1007/s11356-021-18278-5
Yang, B., Jahanger, A., and Ali, M. (2021b). Remittance inflows affect the ecological footprint in BICS countries: Do technological innovation and financial development matter? Environ. Sci. Pollut. Res. 28 (18), 23482–23500. doi:10.1007/s11356-021-12400-3
Yang, B., Jahanger, A., and Khan, M. A. (2020a). Does the inflow of remittances and energy consumption increase CO2 emissions in the era of globalization? A global perspective. Air Qual. Atmos. Health 13 (11), 1313–1328. doi:10.1007/s11869-020-00885-9
Yang, B., Jahanger, A., Usman, M., and Khan, M. A. (2021a). The dynamic linkage between globalization, financial development, energy utilization, and environmental sustainability in GCC countries. Environ. Sci. Pollut. Res. 28 (13), 16568–16588. doi:10.1007/s11356-020-11576-4
Younis, I., Naz, A., Shah, S. A. A., Nadeem, M., and Longsheng, C. (2021). Impact of stock market, renewable energy consumption and urbanization on environmental degradation: New evidence from BRICS countries. Environ. Sci. Pollut. Res. Int. 28 (24), 31549–31565. doi:10.1007/s11356-021-12731-1
Yu, J., Yu, Y., and Jiang, T. (2022). Structural factors influencing energy carbon emissions in China’s service industry: An input–output perspective. Environ. Sci. Pollut. Res. 29, 49361–49372. doi:10.1007/s11356-022-19287-8
Yu, Y., Jiang, T., Li, S., Li, X., and Gao, D. (2020). Energy-related CO2 emissions and structural emissions’ reduction in China’s agriculture: An input–output perspective. J. Clean. Prod. 276, 124169. doi:10.1016/j.jclepro.2020.124169
Yu, Y., Li, S., Sun, H., and Taghizadeh-Hesary, F. (2021). Energy carbon emission reduction of China’s transportation sector: An input–output approach. Econ. Analysis Policy 69, 378–393. doi:10.1016/j.eap.2020.12.014
Yu, Y., and Liu, Q. (2022). An empirical study on correlation among poverty, inclusive finance, and CO2 emissions in China. Environ. Sci. Pollut. Res. Int. 1, 12. doi:10.1007/s11356-022-19901-9
Yu, Y., and Wang, T. (2021). New urbanization, energy-intensive industries agglomeration and analysis of nitrogen oxides emissions reduction mechanisms. Atmosphere 12 (10), 1244. doi:10.3390/atmos12101244
Yue, S., Lu, R., Shen, Y., and Chen, H. (2019). How does financial development affect energy consumption? Evidence from 21 transitional countries. Energy Policy 130, 253–262. doi:10.1016/j.enpol.2019.03.029
Zafar, M., Syed, A., Avik, S., Ayfer, G., and fujun, H. (2019). The role of stock market and banking sector development, and renewable energy consumption in carbon emissions: Insights from G-7 and N-11 countries. Resour. Policy 62, 427–436. doi:10.1016/j.resourpol.2019.05.003
Zaidi, Y., Muhammad, W., Shahbaz, M., and Fujun, H. (2019). Dynamic linkages between globalization, financial development and carbon emissions: Evidence from Asia Pacific Economic Cooperation countries. J. Clean. Prod. 228, 533–543. doi:10.1016/j.jclepro.2019.04.210
Zeng, B., Xie, J., Zhang, X., Yu, Y., and Zhu, L. (2020). The impacts of emission trading scheme on China’s thermal power industry: A pre-evaluation from the micro level. Energy & Environ. 31 (6), 1007–1030. doi:10.1177/0958305x19882388
Zhang, L., Li, Z., Kirikkaleli, D., Adebayo, T., Akisola, G. D., and Akinsola, G. D. (2021). Modeling CO2 emissions in Malaysia: An application of maki cointegration and wavelet coherence tests. Environ. Sci. Pollut. Res. 28, 26030–26044. doi:10.1007/s11356-021-12430-x
Zhang, L., Yang, B., and Jahanger, A. (2022). The role of remittance inflow and renewable and non-renewable energy consumption in the environment: Accounting ecological footprint indicator for top remittance-receiving countries. Environ. Sci. Pollut. Res. 29 (11), 15915–15930. doi:10.1007/s11356-021-16545-z
Zivot, E., and Andrews, D. (1992). Further evidence on the great crash, the oil-price shock and the unit-root hypothesis. J. Bus. Econ. Stat. 10 (3), 251–270. doi:10.2307/1391541
Keywords: shadow economy, financial development, carbon emission, stock market, ARDL, Nigeria
Citation: Yu Y, Chukwuma Onwe J, Jahanger A, Adebayo TS, Hossain ME and David A (2022) Linking shadow economy and CO2 emissions in Nigeria: Exploring the role of financial development and stock market performance. Fresh insight from the novel dynamic ARDL simulation and spectral causality approach. Front. Environ. Sci. 10:983729. doi: 10.3389/fenvs.2022.983729
Received: 01 July 2022; Accepted: 28 July 2022;
Published: 25 August 2022.
Edited by:
Diogo Ferraz, Universidade Federal de Ouro Preto, BrazilReviewed by:
Shuhong Wang, Shandong University of Finance and Economics, ChinaXingle Long, Jiangsu University, China
Copyright © 2022 Yu, Chukwuma Onwe, Jahanger, Adebayo, Hossain and David. This is an open-access article distributed under the terms of the Creative Commons Attribution License (CC BY). The use, distribution or reproduction in other forums is permitted, provided the original author(s) and the copyright owner(s) are credited and that the original publication in this journal is cited, in accordance with accepted academic practice. No use, distribution or reproduction is permitted which does not comply with these terms.
*Correspondence: Joshua Chukwuma Onwe, onwejoshuaa@gmail.com; Atif Jahanger, atif_jahanger@hotmail.com