- 1School of Business Administration, Zhongnan University of Economics and Law, Wuhan, China
- 2School of Politics and International Studies, Central China Normal University, Wuhan, China
Using data from 30 provincial-level in China during 2005–2019, this paper investigates the impact of foreign direct investment (FDI) quality characteristics on carbon emission intensity. Based on the Copeland-Taylor model, the mechanism of the impact of FDI quality characteristics on carbon emission intensity is also investigated. The key findings demonstrate that FDI quality traits considerably lower regional carbon intensity, and the result is still robust after considering the spatial correlation and using IV-2sls in consideration of endogeneity. The FDI quality characteristics mainly reduce carbon emission intensity through green technology improvement, industrial structure, and factor endowment structure optimization. Moreover, further evidence shows that there are heterogeneous effects with regard to regional and FDI quality characteristics. From the standpoint of FDI quality characteristics, this study adds to the literature on FDI and environmental pollution. Policy recommendations for China are also discussed in this paper, which can serve as a guide for other emerging nations.
1 Introduction
Atmospheric problems will not only lead to a decline in labor productivity, but they will also threaten human health (Graff Zivin and Neidell, 2013, Graff Zivin and Neidell, 2012; Chang et al., 2019; Fu et al., 2021) and bring about regional migration of population (Chen et al., 2022), which has attracted significant attention from governments and academia (Chao and Feng, 2018). As the global average temperature rises, greenhouse gas emissions have risen to the forefront of global air governance (Soutter and Mttus, 2020; Nejati and Taleghani, 2022). Carbon dioxide and other greenhouse gases are the primary cause of climate change and temperature rise (Schmalensee et al., 1998). According to the International Energy Agency’s (IEA) report titled “Global Energy Review: Carbon Dioxide Emissions in 2021,” global carbon dioxide emissions reached a record high of 36.3 billion tons in 2021, an increase of 6 percent year-over-year (IEA, 2021). The international community has made concerted efforts to combat climate change on a global scale. The 26th United Nations Climate Change Conference reached an agreement on the implementation rules for the Paris Agreement on 13 November 2021, clarifying the global climate governance model with nationally determined contributions as the foundation after 2020 (UNFCCC, 2021). Currently, over 120 nations and regions have placed carbon neutrality on their agendas, and China, a major carbon emitter, has pledged to achieve “carbon neutrality” by 2060. (Ye et al., 2022).
Since China’s accession to the WTO, however, FDI has poured into China and played an indispensable role in fostering China’s economic development (Wei et al., 2009; Yao et al., 2016). Numerous studies have demonstrated that FDI promotes the economic development of the host country through the technology spillover effect (Anwar and Sun, 2014; Sultana and Turkina, 2020) and the effect of FDI on enterprise productivity (Javorcik, 2004; Lin and Kwan, 2016). However, as China’s opening-up continues to expand, the influx of FDI is accompanied by increasingly severe environmental issues (Tang and Tan, 2015; Wang et al., 2022). As seen in Figure 1, there is a positive correlation between foreign direct investment and China’s carbon dioxide emission. Academic circles are now divided regarding the positive, negative, and non-linear relationship between FDI and China’s environment (Jun et al., 2018; Li and Ramanathan, 2020; Xu et al., 2021). China’s economy is currently advancing to the high-quality stage of sustainable green development, and the utilization of FDI is also shifting from the initial extensive quantitative expansion to the high-quality stage of sustainable green development. Therefore, the impact of FDI on China’s environment should be reexamined from the perspective of FDI quality, we hope, on the one hand, to provide a new interpretation perspective for the controversy of “Pollution Heaven” and “Pollution Halo,” and on the other, to serve as a guide for the modification of investment attraction strategies in China and other emerging nations.
The “Pollution Heaven Hypothesis” and the “Pollution Halo Hypothesis” have always been diametrically opposed perspectives in academia regarding the impact of FDI on the environment. Copeland and Taylor (1994) proposed the “Pollution Haven Hypothesis,” which states that developed countries transferred some industries with high pollution and low added value to developing countries due to their high environmental protection requirements and the need for industrial transformation and upgrading. To close the economic development gap with developed countries, foreign capital recipient countries have adopted a tacit and conniving attitude towards polluting foreign investment, and the “race to the bottom” in environmental regulation has created a “Pollution Haven” for the transfer of polluting foreign capital from developed countries (Cheng et al., 2020). Since the “Pollution Haven Hypothesis” was proposed, a plethora of literatures have examined the impact of international capital flows on environmental issues such as carbon emissions from the perspective of foreign direct investment (FDI). Singhania and Saini (2021), Baek (2016), and Sapkota and Bastola (2017) use data from developing countries in Africa, Southeast Asia, and Latin America, respectively, to establish the existence of “Pollution Haven.” Using China’s empirical data, Ren et al. (2014), Jun et al. (2018), and Wang et al. (2022) reach the same conclusion. Contrary to the “Pollution Haven Hypothesis,” also known as the “Pollution Halo Hypothesis” (Birdsall and Wheeler, 1993), the influx of FDI does not exacerbate the environmental degradation in the host country. In contrast, the advanced technology and management experience brought by FDI are partially advantageous to the improvement of production mode and resource utilization efficiency of the host country, which is advantageous for the reduction of environmental pollution in host countries (Reppelin-Hill, 1999; Zeng and Eastin, 2012; Huang et al., 2019). FDI enterprises have a higher technical level and management experience than local enterprises in the host country, so they are more capable of improving energy utilization efficiency for cleaner production, which in turn helps reduce the emission of pollutants in the host country (Hille et al., 2019). Moreover, the green production technology of FDI can effectively stimulate local firms to engage in technological innovation through the spillover effect and competition effect, thereby enhancing the environment of the host country (Dong et al., 2019; Li and Ramanathan, 2020). Demena and Afesorgbor (2020) combed through and analyzed 65 relevant research literatures, and used the Meta-analysis method to analyze the relationship between FDI and carbon dioxide emissions, thereby proving the “Pollution Halo Hypothesis” despite the heterogeneity of the research. Another study asserts that FDI and the environment of host countries have a non-linear relationship (Xie and Sun, 2020), such as a U-shaped (Xu et al., 2021) or N-curve (Liu et al., 2019) relationship. Alternatively, whether FDI reduces carbon emissions depends on whether it has reached the corresponding threshold, such as human capital (Lan et al., 2012; Jahanger et al., 2022), income (Shahbaz et al., 2015), and environmental regulation (Chang and Li, 2019; Mahmood et al., 2020).
To sum up, there are controversies regarding the influence of FDI on carbon emissions, including promotion, inhibition, and non-linear characteristics. The majority of existing research views FDI as homogeneous capital and examines its impact on carbon emissions. Rarely has research been conducted on the effect of FDI quality characteristics on carbon emissions. The absence of a unified standard for measuring the quality characteristics of FDI is one possible explanation. Moreover, FDI quality characteristics are more challenging to quantify than FDI flow and stock. Currently, research on the quality characteristics of FDI focuses primarily on the impact of FDI with different characteristics on economic growth (Alfaro and Charlton, 2007; Javorcik and Spatareanu, 2011; Jahanger, 2021) and energy efficiency (Pan et al., 2020) and other aspects, but studies on the intensity of carbon emissions are scarce.
This paper’s marginal contribution relative to previous research may be threefold: This paper attempts to provide empirical evidence that the quality characteristics of FDI impact carbon emissions intensity. As described by Kumar (2002) and Buckley et al. (2004), we comprehensively evaluated FDI quality characteristics from the perspectives of technological level, investment intensity, export level, profitability, and management ability by using the global entropy method. Second, according to Grossman and Krueger (1995), previous research has broken down the impact mechanism of FDI on the environment of host nations into scale, composition, and technique effects (Nathaniel et al., 2020; Pazienza,2019; Shahbaz et al., 2019). We extend the Copeland-Taylor model by incorporating FDI quality characteristics and decomposing the influence mechanism of FDI quality characteristics on carbon emission intensity into technique and composition effects. Third, we examine in detail the mechanism of the impact of FDI quality characteristics on carbon emission intensity, using the industrial structure and factor structure adjustment of green technological progress as mediating variables and taking into account the regional and FDI quality characteristics’ heterogeneity.
The remaining sections of this paper are structured as follows. Section 2 examines the theoretical mechanism by which the quality of FDI affects the intensity of carbon emissions. The third section presents the data and establishes the empirical model. The fourth section presents and analyzes the empirical findings. The concluding section outlines the paper’s key findings and policy implications.
2 Theoretical model
Following Copeland and Taylor’s paradigm for analysis and quoting Wang et al. (2019), we introduce the quality characteristics of FDI to analyze its effect on carbon emission intensity, laying the theoretical foundation for the ensuing empirical analysis.
2.1 Basic settings
It is assumed that the entire production system produces two types of products, clean product
Where,
2.2 Reduction decision for enterprise
According to the production function of
obtaining the first-order reciprocal of Eq. 6:
2.3 Emission decision for enterprise
Assuming the market is perfectly competitive and the enterprise’s net profit is zero, then:
Where,
A further deformation of Eq. 9:
Where,
Therefore, the emission of pollutants is mainly affected by output scale, output structure and technical level. Extensive literatures have confirmed that international capital flows (FDI) will affect pollutant emissions through these three effects (Pazienza,2019; Shahbaz et al.,2019). Based on this, we define pollution emission intensity as the emission level of pollution per unit of output. Therefore, divide both sides of Eq. 10 by the total output S and take the logarithm to obtain Eq. 12:
Where,
High-quality FDI is more capable of upgrading green technology and adjusting production structure to reduce pollution emission level: on the one hand, high-quality FDI frequently brings advanced green production technology and management experience to host countries, which facilitates the development of local enterprises toward cleaner production via the spillover effect (Sun et al., 2019; Chen et al., 2021). On the other hand, high-quality FDI facilitates the transition from a labor-intensive to a capital-intensive factor endowment structure. In conjunction with research and development of clean technology, pollution emissions can be reduced (De Vita et al., 2021). If capital accumulation increases energy consumption and emission demand, it is not conducive to reducing pollution emissions (Li and Lin, 2017). Additionally, high-quality FDI, particularly those that tend to flow into service and high-tech industries, can assist in optimizing industry and product structure, enhancing energy efficiency, and so reducing pollutant emission levels (Bakhsh et al., 2017; Hossain et al., 2022).
To sum up, the quality characteristics of FDI will influence the intensity of pollution emissions via the composition effect and the technique effect. In order to verify the impact of the quality characteristics of FDI on pollution emission intensity, the composition effect function and technique effect function are revised as follows, with reference to Wang et al. (2019):
Where,
3 Methodology and data
3.1 Empirical model
To investigates the impact of FDI quality characteristics on carbon emission, we follow the theoretical mechanism analysis in Section 2, the benchmark regression model is:
Where, the subscripts
Due to geographical proximity, carbon emissions may have spatial correlation or local carbon emission levels may be affected by neighboring FDI. Disregarding the spatial correlation may lead to inaccurate conclusions. Therefore, we constructed the following Spatial Durbin Model (SDM) based on the baseline regression in order to examine the impact of quality characteristics of FDI on regional carbon emissions in a more complete manner (The test and selection of spatial econometric model are explained in detail in Section 4.4 of this paper).
Where,
Common space weight matrices include the 0–1 adjacency space matrix, the inverse distance space matrix, and the economic distance space matrix, among others. Due to the fact that the radius of CO2 diffusion is primarily dependent on distance, this paper uses the distance between provinces to create an inverse distance space matrix. Using the county-level longitude and latitude coordinate data published by the National Platform for Common Geospatial Information Services, and relying on the average longitude and latitude of the provincial capital cities, we construct the inverse distance space matrix
3.2 Description of variables
3.2.1 Core explained variables
Indicators for measuring the level of regional carbon emissions generally include the total emission of CO2 and the intensity of CO2. As China’s economic structure is in the adjustment phase, industrialization and urbanization are still accelerating, and energy consumption for economic construction remains high. China will not be able to reduce its carbon emissions overnight. China’s pledge to peak carbon emissions by 2030 demonstrates that China’s current emission reduction challenge entails a progressive reduction in the intensity of carbon emissions to give priority to the objective of “carbon peaking,” followed by a transition to “carbon neutrality.” Therefore, measuring the level of carbon emissions by the intensity of carbon emissions is more consistent with China’s national reality. Carbon emission per unit of GDP is employed in this paper to measure CO2 emission intensity, which is recorded as CEI.
3.2.2 Core explanatory variables
There is no standardized approach for measuring the quality characteristics of FDI. Based on the concept of FDI quality proposed by Kumar (2002) and Buckley et al. (2004), we define high-quality FDI as possessing a high degree of technical expertise, a wealth of export experience, strong managerial skills, and profitability. Therefore, we measure the quality characteristics of FDI from five aspects: technical characteristics
The technical characteristics of FDI are measured by the total factor productivity (TFP) of foreign-invested industrial enterprises. We measure the relative technical level of FDI using the ratio of the per capita unit output of the above-scale FDI industrial companies to the per capita unit output of the above-scale Industrial Enterprises. This index also shows the technical difference between local industrial firms and industrial enterprises with foreign investment.
The average investment amount of a single foreign direct investment business measures the investment intensity of FDI. The greater the investment intensity, the stronger the capital strength of the foreign investment enterprise and the larger the scale of the single investment.
The export of FDI enterprises has traditionally accounted for a significant percentage of China’s regional export, thus export capability has become an indispensable aspect of FDI. We use the ratio of the export volume of FDI enterprises to the total regional export volume to measure the export capacity of FDI.
The profitability of FDI refers to the profitability of enterprises after deducting corresponding costs and expenses, which is generally measured by the cost expense profit margin. In this paper, the ratio of the cost expense profit margin of the above-scale FDI industrial enterprises to the cost expense profit margin of the above-scale industrial enterprises is used to express the profitability of FDI.
Enterprises with superior management skills are able to efficiently coordinate and manage internal resources and increase the asset operation’s efficiency. The contribution rate of assets reflects the enterprise management level to some extent. To measure FDI management capability, we use the ratio of the asset contribution rate of the above-scale FDI industrial firms to the asset contribution rate of the above-scale industrial enterprises.
3.2.3 Control variables
Referring to Jiang and Zhao (2019), we first select a set of control variables that are expected to influence both the level of regional carbon emissions and the quality of FDI inflows, including regional energy consumption structure, industrial structure, regional R&D level, urbanization, and openness level: 1) The energy consumption structure
In addition, we introduce the level of regional environmental regulation, comprising both formal and informal environmental regulation, as a control variable. Formal environmental regulation generally relies on government directives to affect the environment and economic system. Earlier, Pargal and Wheeler (1996) defined informal environmental regulation as follows: when formal environmental regulation cannot play a full role, informal environmental regulation such as public supervision and social demand can supplement the upper position. According to North’s (1991) institutional theory, formal institutions can only function effectively if they are compatible with informal institutions. Considering only one level of environmental regulation may be biased, so we use both formal and informal environmental regulation as control variables to reduce the bias of omitted variables.
Formal environmental regulation is usually measured by the proportion of industrial pollution control investment in GDP. However, due to the differences in regional industrial structure, in order to reduce the deviation in the evaluation of environmental regulation indicators caused by the differences in industrial structure, the formal environmental regulation indicators in this paper are treated as follows:
The subscripts
Drawing on Pargal and Wheeler (1996), we select income level, population density and education level to construct an informal environmental regulation index system, and use the entropy method to obtain the informal environmental regulation level, which is recorded as
3.2.4 Mediating variables
According to the preceding theoretical study, the quality characteristics of FDI would influence the intensity of carbon emissions via the technique effect and the composition effect. However, if technological progress develops in the direction of scale expansion rather than cleaner production, it will enhance the intensity of carbon emissions. Therefore, in order to better measure whether the quality characteristics of FDI would affect the regional carbon emission intensity through green technology, technological progress
Where, the subscripts
3.3 Data source
In this paper, the data of 30 provinces, municipalities and autonomous regions in China from 2005 to 2019 are selected as the research samples (Tibet, Hong Kong, Macao, and Taiwan are more missing, which are excluded from the sample). Institute of Public & Environmental Affairs (IPE) has produced monitoring data of carbon dioxide emissions since 2005, which more accurately reflects the regional carbon emission level in terms of CO2 emission intensity. This study uses IPE’s public carbon emission statistics to manually compile carbon emission intensity data for 30 provinces, municipalities, and autonomous regions in China from 2005 to 2019 (The remaining variables and data sources are shown in Supplementary Table S1 in Appendix A).
To reduce the quantity difference between variables and the problem of multiple collinearities, the absolute number is expressed in logarithmic form. For the variables measured in US dollar, the annual average exchange rate is used to convert into RMB, and the data of per capita capital stock and other currency types are respectively adjusted by using the fixed assets investment index and CPI based on 2005 to eliminate the impact of inflation. Since there are no missing data for explained variables and explanatory variables, the forward-backward filling method is adopted for very few missing data to fill in the missing data. Finally, we obtain the balanced panel data of 30 provinces, municipalities and autonomous regions in China (The descriptive statistical analysis of variables is shown in Supplementary Table S2 in Appendix A).
4 Empirical results and discussion
4.1 Baseline results and discussion
The White test indicates that the baseline model (model 16) rejects the null hypothesis of homoscedasticity [chi2 (54) = 220.05]. Therefore, we adopt “panel fixed effect + clustering standard error” and more comprehensive FGLS (FGLS) for regression in an effort to mitigate statistical bias induced by heteroscedasticity. For regression, we additionally employ OLS and “panel random effect + cluster standard error” to enable comparison and verify the robustness of the results. The benchmark regression results of the impact of FDI quality characteristics on carbon emission intensity are shown in Table 1. Considering the heteroscedasticity and intra-group autocorrelation, the FGLS regression findings serve as the analytical criterion. The quality characteristics of FDI have a considerable inhibitory influence on regional carbon emission intensity, as shown in Table 3. From the perspective of the quality characteristics of FDI, the “pollution Hevan Hypothesis” has not been verified. Improving regional FDI quality is an effective strategy for reducing regional carbon intensity and achieving carbon emission reduction.
Table 1 displays the test results for robustness of several regression methods on model 16. Economic activities frequently result in a delayed response, and the model itself may be susceptible to reverse causality issues. Therefore, we conducted robustness test for all explanatory variables lagging behind one period. At the same time, considering that the dual environmental regulation and the level of marketization will have an impact both on the carbon emission intensity and the quality characteristics of FDI. We also add the interaction terms of formal environmental regulation and informal environmental regulation (recorded as crossER) and the level of marketization (the proportion of general public financial expenditure to GDP, recorded as market) into the control variables to further test the robustness of Model 16. Table 2 contains the results of the robustness test. It can be observed that the symbol and significance of the core explanatory variable (quality) have not changed, indicating the robustness of the benchmark regression results.
Areas with high pollution will affect the production efficiency of enterprises and the concentration of human capital, which will make it difficult for the areas to attract high-quality FDI. From this perspective, the baseline model may have potential reverse causality issues that may lead to biased regression results. Although we have tried to take a lag period for all explanatory variables to alleviate the potential reverse causality problem, and from the control of appropriate variables, time and individual fixed effect to alleviate the estimation bias caused by omitted variables. Nonetheless, it is evident that these techniques are still insufficient to eliminate the endogeneity issue. In order to further examine the problem of causality identification in basic regression, we employ the instrumental variable for the quality characteristics of FDI.
The distance from the city center of each province to the nearest port is used as an instrumental variable (IV) for the quality characteristics of FDI. The distance between each province’s core location and the port is strictly exogenous to economic development, confirming that the instrumental variable is completely exogenous. Secondly, based on China’s actual development, it is simpler to attract high-quality FDI the closer a port is to a greater degree of economic development. Consequently, employing port proximity as an IV of FDI quality attributes can successfully mitigate endogenous difficulties caused by reverse causality.
Iv-2sls is utilized for estimation in this paper, and the results are shown in Table 3. The 2SLS technique is predicated on the existence of endogenous explanatory variables and the validity of instrumental variables. The DWH and unidentified tests are done, rejecting the null hypothesis that the core explanatory variable is exogenous (p = 0.0000) and that the instrumental variable is unidentified (p = 0.0000, demonstrating the validity of instrumental variables). Table 3 reveals that the F-statistic of the first stage regression is 119.16, which is significantly greater than the empirical value of 10, demonstrating that there is no weak instrumental variable problem. Since the instrumental variable is time-invariant, it is not suitable for controlling the panel fixed effect, and the instrumental variable satisfies strict exogeneity and is not related to the error and the explained variable. Therefore, only controlling the time fixed effect can identify the causal relationship between the quality characteristics of FDI and the intensity of carbon emissions. It can be seen from the results in Table 3 that the potential endogenous problems underestimate the carbon emission reduction effect of the quality characteristics of FDI, Nonetheless, the impact of the quality characteristics of FDI on the carbon emission intensity remains negative, and the significance and sign have not changed, demonstrating the robustness of the benchmark regression results.
4.2 Heterogeneity analysis
4.2.1 Regional heterogeneity analysis
Based on factors such as policy, economy, and geographical environment, FDI is concentrated in the eastern coastal areas of China, and the saturation of FDI in coastal areas is significantly higher than in China’s interior. To determine if the impact of FDI quality characteristics on carbon emission intensity is regionally heterogeneous, we divide the entire region into eastern coastal China and central and western inland China. The eastern coastal China include 12 provinces and autonomous regions such as Hainan, Guangxi, Guangdong, Fujian, and Shandong. While the rest are divided into central and western inland China. Table 4 displays the regional heterogeneity regression results.
The quality characteristics of FDI in eastern coastal China exacerbate the carbon emission intensity, while the quality characteristics of FDI in central and western inland China reduce the carbon emission intensity. The reasons may be as follows: 1) the high degree of FDI saturation has caused a large number of resources to accumulate in eastern coastal China. With the sustainable development of local enterprises in eastern coastal China, the spillover impact of FDI on local enterprises has diminished, progressively manifesting as crowding out and competition effects. In order to reduce costs and maintain market share, high-quality FDI in the eastern region tends to expand in scale and profits, thus crowding out R&D costs for clean and emissive-reducing technologies and increasing carbon emission levels. 2) FDI in eastern China’s coastal region mainly flows into the high-yield and high-profit real estate industry, as well as the high-emission transportation, electric power and gas industry, which leads to the improvement of carbon emission level. 3) The inland areas are far behind the eastern coastal areas in terms of FDI saturation, industrial base or economic development level in China. Through spillover effects and competitive incentive effects, high-quality FDI in inland regions can effectively increase the quality level of firms in the central and western regions of China. Moreover, the introduction of high-quality FDI in the central and western regions of China not only promotes GDP growth, but also aids in the elimination of excess capacity in the central and western regions and promotes the development of clean technology, thereby contributing to the reduction of carbon emission intensity.
4.2.2 Heterogeneity of FDI quality characteristics
From the perspective of the quality characteristics of FDI, FDI is an aggregate of resources such as technology capital management ability. In order to further explore whether the quality characteristics of FDI have a heterogeneous impact on carbon emission intensity, it is necessary to investigate the impact on carbon emission intensity from the five quality characteristics of FDI. Table 5 demonstrates that, among the five quality characteristics of FDI, the export capacity of FDI significantly reduces the intensity of carbon emissions, whereas the profitability and management abilities of FDI increase the intensity of carbon emissions. Positive but insignificant impact of technical qualities and investment intensity on carbon emission intensity.
First, the technical characteristics of FDI have not played a significant role in local energy conservation and emission reduction. The reason may be that the technological progress of FDI is biased towards production expansion rather than energy saving and emission reduction, or that the carbon reduction effect of clean technology is not yet obvious. Second, the impact of investment intensity on carbon emission intensity is not significant, which is also explained that there is no significant evidence of “pollution paradise” effect in China during the sample period. Thirdly, the impact of FDI’s export capacity on carbon emissions intensity significantly reduces indigenous carbon emissions intensity at the 1% level. The export capacity of FDI enterprises represents, to some extent, the competitiveness of FDI enterprises on the international market, which not only opens up new international markets for local enterprises but also forces local enterprises to innovate their production technology and adopt clean technology production, thereby contributing to the reduction of carbon emission levels. In addition, the spillover effect of clean technology from FDI exporters also accelerates the pace of clean technology innovation in local enterprises. Fourth, FDI with higher profitability and management significantly increases the level of carbon intensity, which contradicts the previously held belief that enterprises with higher profitability and capital operation capabilities have sufficient funds for clean technology development and lower carbon intensity. This may be related to the industry sector of FDI inflows, external constraints and the social responsibility of firms. From 2005 to 2019, the majority of foreign direct investment (FDI) in China flowed into the real estate and manufacturing sectors with high profit margins and high emissions, both of which captured more profits for FDI enterprises while bringing higher carbon emission levels; the inflow of FDI into high-end manufacturing, service, and information technology sectors is the key to the carbon emission reduction effect, but as can be seen in Figure 2, the proportion of FDI flowing into these sectors is relatively small. Furthermore, when firms take the initiative to reduce emissions, with the limited capital constraints of the firm, capital is allocated efficiently within the firm while the firm tilts some of its resources towards low carbon emissions reduction. Therefore, the reason why profitability and management capabilities do not reduce carbon emissions of FDI firms is not only related to the industries into which they have flowed, but also because the capital operations of FDI firms still seek to maximize capital gains, so they expand production to obtain revenue, which in turn increases the intensity of carbon emissions.
We also investigate the heterogeneous effects of FDI quality characteristics in eastern coastal China and central and western inland China on carbon emission intensity. From the regression results in Table 6, it can be seen that the positive effect of FDI quality on carbon emission intensity in eastern coastal China is primarily attributable to the investment intensity and exports of FDI companies. The export capability of FDI is primarily responsible for the carbon emission reduction effect of qualitative characteristics of FDI in central and western inland China, but profitability and management level of FDI enhance the carbon emission intensity of the central and western areas. As discussed in the preceding section, FDI in the eastern region primarily flows into high-emission real estate, electricity, and gas industries, and the effect of the expansion of production scale brought about by the increase in FDI investment intensity is insufficient to offset the resultant increase in carbon emissions, thereby increasing the carbon emission intensity in the eastern region of China. In addition, the eastern coast of China is still dominated by exports with poor added value. Even if exports of high-tech goods are on the upswing, their share of total exports remains limited. On the one hand, the export of low-value-added items, primarily from the manufacturing industry, has boosted carbon emissions. On the other hand, it has constrained resources in the East and lowered the global output rate, resulting in a rise in the intensity of carbon emissions. Due to the gap in economic development, the export of FDI in central and western inland China not only helps to reduce excess capacity, but also opens up new markets for enterprises in the central and western regions, introduces new technologies, and increases regional output, thereby contributing to the reduction of carbon emissions in central and western China. The reasons why profitability and management capabilities do not reduce carbon intensity in central and western inland China may be consistent with the previous analysis and relate to the industries into which they flow and the social responsibility of the firms.
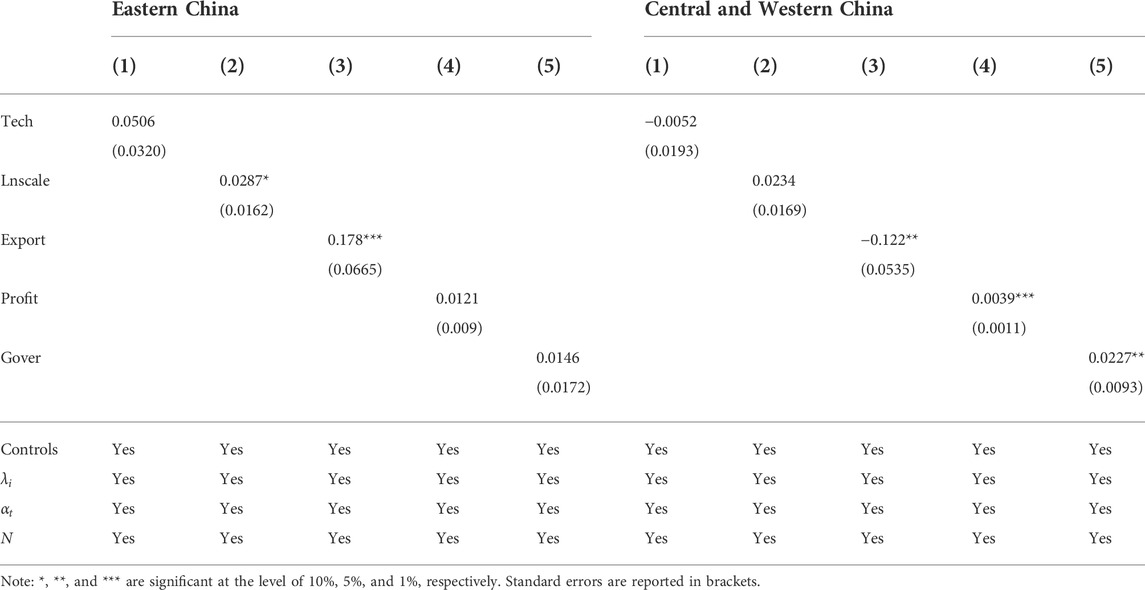
TABLE 6. Regional differences of FDI heterogeneity quality characteristics on carbon emission intensity.
4.3 Mechanism test
The aforementioned findings demonstrate that the quality characteristics of FDI contribute to the reduction of carbon emissions, with a decreasing effect in central and western inland China and a rising effect in eastern coastal China. Therefore, what causes this phenomenon? Based on the previous theoretical mechanism analysis, FDI quality characteristics have an impact on carbon emission intensity by influencing regional green technology progress and production restructuring. Therefore, we focus on the transmission mechanism of carbon emission reduction by FDI quality characteristics and the differences between the eastern coastal China and central-western inland China.
4.3.1 Technique effect
The quality characteristics of FDI affect the level of carbon emission intensity through technique effect. Through technology spillover, high-quality FDI enhances the level of regional green technological innovation. On the other side, high-quality FDI encourages local businesses to engage in green innovation through the rivalry mechanism and high-quality FDI demand for clean products, thereby reducing the intensity of regional carbon emissions through these two channels. To validate this transmission mechanism, we use the proportion of regional green patent applications as a proxy variable for green technology. The specific empirical results are reported in Table 7, where columns (1)–(3) demonstrate that FDI quality characteristics significantly increase the amount of green technology innovation in both the eastern and mid-western regions. And column (4) shows that regional green technology innovation significantly reduces carbon emissions intensity. Therefore, it is suggested that the influence mechanism of FDI quality characteristics to reduce regional carbon intensity by increasing the level of regional green innovation is valid.
4.3.2 Composition effect
According to the above theoretical mechanism analysis, the quality characteristics of FDI will also affect the carbon emission intensity through the optimization of industrial structure and factor endowment structure. The secondary sector, mainly industry, is still the main source of carbon emissions. On the one hand, high-quality FDI can reduce carbon emissions by improving the level of green technology in the secondary industry, On the other hand, it optimizes the current industrial structure by flowing into high-tech industries and clean industries, such as service industries, to achieve the goal of carbon emission reduction. The shift in resource factor endowments from labor-intensive to capital-intensive may enhance the demand for pollution emissions, but it also completes the capital accumulation required for clean technology innovation increasing labor productivity and increasing output, which in turn reduces carbon emissions intensity. The green technology effect of FDI quality characteristics has been verified in the previous section, and the next section focuses on verifying the composition effect of FDI quality characteristics.
According to Table 8, the quality characteristics of FDI optimize the industrial structure and increase the capital stock per capita. However, only the central and western inland China are significant, while the eastern coastal China are not. As previously analyzed, this is due to the increased saturation of FDI in eastern China and the industries it flows into. The higher saturation of FDI and the capture of FDI by low-end manufacturing makes the spillover effect of FDI in the eastern China weaker as well as not releasing quality resources of eastern China. As can be observed from columns (4) and (8) of Table 8, the industrial structure dominated by the secondary sector enhances the regional carbon intensity, and the increase in the capital stock per capita helps to reduce the carbon intensity. The regression results in Table 8 show that the quality characteristics of FDI reduce the level of carbon emission intensity by optimizing the structural effect of industrial structure and factor endowment.
4.4 Consider spatial correlation
4.4.1 Spatial correlation test
Due to the proximity of geographical locations, environmental pollution issues are frequently spatially correlated, and disregarding the spatial correlation of carbon emissions may bias the regression results. As depicted in Figure 3, the spatial correlation of Moran I for CO2 emission intensity of 30 provinces from 2005 to 2019 is tested. The index ranges between 0.08 and 0.11, and all values surpass the 1% significance threshold, indicating that spatial correlation should be considered.
Further, data from the years 2005, 2008, 2013, and 2018 were chosen to generate Moran I scatter plots illustrating the regional association of carbon emissions intensity. As seen in Figure 4, the majority of provinces, with the exception of a few, are in quadrants one and three, exhibiting the typical characteristics of high value aggregation and low value aggregation, indicating that the 30 provinces have a high positive correlation in carbon emission intensity, and further demonstrating the necessity to consider the spatial correlation of carbon emission intensity.
4.4.2 Estimation results of SDM
In Section 3, the spatial panel is set up as a spatial Durbin model (SDM). To justify the setting of the baseline econometric model, here, the LM, LR and Wald tests are conducted on the spatial model, and the results all prove the reasonableness of SDM setting (The test results are shown in Supplementary Table S3 in the Appendix A).
Columns (1)–(6) in Table 9 detail the SDM regression results for the effects of FDI quality characteristics, technology level, investment intensity, export capacity, profitability, and management capacity on carbon emission intensity. The spatially lagged terms are all significant at the 1% level, demonstrating that carbon emission intensity is very spatially dependent and that an increase in carbon emission intensity in nearby regions will lead to an increase in local carbon emission levels. Therefore, it is difficult to achieve the goal of regional emission reduction by relying on separate governance. Regionally coordinated methods of joint prevention and control should be implemented to prevent the transmission of carbon emissions in close proximity. The impact of FDI quality characteristics on carbon emission intensity is consistent with the previous regression results, demonstrating once again the robustness of baseline regression findings.
The spatial Durbin models above all use fixed effects models to mitigate omitted variable bias. The robustness tests of the spatial Durbin model also incorporate a one-period lag for all independent variables to mitigate the reverse causality issue. The direction and significance level of the effects on carbon intensity are unaltered for both the core explanatory variables and the control variables. In addition, we test the robustness of the spatial Durbin model in terms of replacing the spatial weight matrix and the main control variables. In the regression of the spatial Durbin model described above, the spatial weight matrix
5 Conclusion and policy implications
We select the balanced panel data of 30 provinces, municipalities and autonomous regions in China from 2005 to 2019 to empirically test the impact of FDI quality characteristics on regional carbon emission intensity. Regressions, whether using a panel model or a spatial Durbin model that takes spatial correlation into account, show that FDI quality characteristics contribute to a reduction in regional carbon emissions intensity. This result remains robust after using instrumental variables to mitigate potential endogeneity issues. The five qualitative characteristics of FDI have a heterogeneous impact on carbon intensity, and the “pollution heaven hypothesis” and “pollution halo hypothesis” do not show an either/or relationship. The export capability of FDI adds significantly to the carbon reduction effect, whereas the profitability and management capacity of FDI raise the carbon intensity. The regional heterogeneity analysis reveals that the quality characteristics of FDI in eastern China increased the carbon emission intensity, whereas in central and western China the converse is true. Further study revealed that the export capacity and investment intensity of FDI in eastern China increased the intensity of carbon emissions, which was mostly attributable to the saturation of FDI, the export structure of firms, and the industries into which FDI flows. The influence of FDI quality characteristics on carbon emission reduction in central and western China is primarily attributable to FDI export capacity. FDI’s profitability and management level boost carbon emission intensity. Further verify the impact mechanism of FDI quality characteristics on carbon emission intensity, and find that FDI quality characteristics mainly reduce the level of regional carbon emission intensity by enhancing green technological progress and optimizing industrial structure and factor endowment structure, but the composition effect of FDI quality characteristics on carbon emission reduction is only significant in central and western China.
In response to these findings, this study has some policy implications for the area of carbon emission reduction. On the one hand, high-quality FDI has obvious carbon emission reduction characteristics, so local governments should prioritize the introduction of high-quality FDI while avoiding blind pursuit of FDI’s high profitability and capital operation capability. On the other hand, the Chinese government should continue to improve the level of openness in central and western China and reasonably guide FDI, particularly export-oriented FDI, to central and western China in order to effectively solve overcapacity in central and western regions and release high-quality resources in eastern regions. In conclusion, the principal routes for high-quality FDI to reduce carbon emissions are the development of clean technologies and structural optimization. Therefore, FDI should be directed toward industries with lower pollution emission intensity, such as the service industry, the information technology industry, and the high-end manufacturing industry, to optimize the industrial layout of FDI and promote clean technology advancement.
Despite these promising results, the limitations of present study still need to be considered. First, the sample for this paper consists of provincial-level data, which is a relatively small sample size. Second, the evaluation of FDI quality focuses mostly on industrial industries, ignoring the quality of FDI in service industries and other sectors. Future studies should enlarge the sample size and examine the quality of FDI in more aspects.
Data availability statement
The datasets presented in this study can be found in online repositories. The names of the repository/repositories and accession number(s) can be found in the article/Supplementary Material.
Author contributions
WM: conceptualization, methodology, data curation, formal analysis, writing-original draft, and project administration. KL: methodology, formal analysis, writing-original draft, and funding acquisition. YL: conceptualization, supervision, funding acquisition, and editing. HZ: methodology, writing-review, and editing.
Funding
This work is supported by the Major Project of Philosophy and Social Sciences Research in Colleges and Universities of Hubei Province (No. 21ZD013), Fundamental Research Funds for the Central Universities (No. 2722022BY013), and The National Social Science Fund of China (No. 21BGJ002).
Conflict of interest
The authors declare that the research was conducted in the absence of any commercial or financial relationships that could be construed as a potential conflict of interest.
Publisher’s note
All claims expressed in this article are solely those of the authors and do not necessarily represent those of their affiliated organizations, or those of the publisher, the editors and the reviewers. Any product that may be evaluated in this article, or claim that may be made by its manufacturer, is not guaranteed or endorsed by the publisher.
Supplementary material
The Supplementary Material for this article can be found online at: https://www.frontiersin.org/articles/10.3389/fenvs.2022.998915/full#supplementary-material
References
Alfaro, L., and Charlton, A. (2007). Growth and the quality of foreign direct investment: Is all FDI equal. Alexandria: HBS Finance Working, Paper No. 07-072.
Anwar, S., and Sun, S. (2014). Heterogeneity and curvilinearity of FDI-related productivity spillovers in China’s manufacturing sector. Econ. Model. 41, 23–32. doi:10.1016/j.econmod.2014.03.021
Baek, J. (2016). A new look at the FDI–income–energy–environment nexus: Dynamic panel data analysis of ASEAN. Energy Policy 91, 22–27. doi:10.1016/j.enpol.2015.12.045
Bakhsh, K., Rose, S., Ali, M. F., Ahmad, N., and Shahbaz, M. (2017). Economic growth, CO2 emissions, renewable waste and FDI relation in Pakistan: New evidences from 3SLS. J. Environ. Manag. 196, 627–632. doi:10.1016/j.jenvman.2017.03.029
Birdsall, N., and Wheeler, D. (1993). Trade policy and industrial pollution in Latin America: Where are the pollution havens? J. Environ. Dev. 2 (1), 137–149. doi:10.1177/107049659300200107
Buckley, P. J., Clegg, J., and Wang, C. (2004). The relationship between inward foreign direct investment and the performance of domestically‐owned Chinese manufacturing industry. Multinatl. Bus. Rev. 12, 23–40. doi:10.1108/1525383X200400014
Chang, S. C., and Li, M. H. (2019). Impacts of foreign direct investment and economic development on carbon dioxide emissions across different population regimes. Environ. Resour. Econ. (Dordr). 72 (2), 583–607. doi:10.1007/s10640-018-0216-1
Chang, T. Y., Graff Zivin, J., Gross, T., and Neidell, M. (2019). The effect of pollution on worker productivity: Evidence from call center workers in China. Am. Econ. J. Appl. Econ. 11 (1), 151–172. doi:10.1257/app.20160436
Chao, Q., and Feng, A. (2018). Scientific basis of climate change and its response. Glob. Energy Interconnect. 1 (4), 420–427. doi:10.14171/j.2096-5117.gei.2018.04.002
Chen, M., Sinha, A., Hu, K., and Shah, M. I. (2021). Impact of technological innovation on energy efficiency in industry 4.0 era: Moderation of shadow economy in sustainable development. Technol. Forecast. Soc. Change 164, 120521. doi:10.1016/j.techfore.2020.120521
Chen, S., Oliva, P., and Zhang, P. (2022). The effect of air pollution on migration: Evidence from China. J. Dev. Econ. 156, 102833. doi:10.1016/j.jdeveco.2022.102833
Chen, Z., Yu, B., Yang, C., Zhou, Y., Yao, S., Qian, X., et al. (2021). An extended time series (2000–2018) of global NPP-VIIRS-like nighttime light data from a cross-sensor calibration. Earth Syst. Sci. Data 13 (3), 889–906. doi:10.5194/essd-13-889-2021
Cheng, Z., Li, L., and Liu, J. (2020). The impact of foreign direct investment on urban PM2. 5 pollution in China. J. Environ. Manag. 265, 110532. doi:10.1016/j.jenvman.2020.110532
Copeland, B. R., and Taylor, M. S. (1994). North-South trade and the environment. Q. J. Econ. 109 (3), 755–787. doi:10.2307/2118421
De Vita, G., Li, C., and Luo, Y. (2021). The inward FDI-energy intensity nexus in oecd countries: A sectoral R&D threshold analysis. J. Environ. Manag. 287, 112290. doi:10.1016/j.jenvman.2021.112290
Demena, B. A., and Afesorgbor, S. K. (2020). The effect of FDI on environmental emissions: Evidence from a meta-analysis. Energy Policy 138, 111192. doi:10.1016/j.enpol.2019.111192
Dong, Y., Shao, S., and Zhang, Y. (2019). Does FDI have energy-saving spillover effect in China? A perspective of energy-biased technical change. J. Clean. Prod. 234, 436–450. doi:10.1016/j.jclepro.2019.06.133
Fu, S., Viard, V. B., and Zhang, P. (2021). Air pollution and manufacturing firm productivity: Nationwide estimates for China. Econ. J. 131 (640), 3241–3273. doi:10.1093/ej/ueab033
Graff Zivin, J., and Neidell, M. (2013). Environment, health, and human capital. J. Econ. Literature 51 (3), 689–730. doi:10.1257/jel.51.3.689
Graff Zivin, J., and Neidell, M. (2012). The impact of pollution on worker productivity. Am. Econ. Rev. 102 (7), 3652–3673. doi:10.1257/aer.102.7.3652
Grossman, G. M., and Krueger, A. B. (1995). Economic growth and the environment. Q. J. Econ. 110 (2), 353–377. doi:10.2307/2118443
Hille, E., Shahbaz, M., and Moosa, I. (2019). The impact of FDI on regional air pollution in the republic of Korea: A way ahead to achieve the green growth strategy? Energy Econ. 81, 308–326. doi:10.1016/j.eneco.2019.04.004
Hossain, M., Rej, S., Saha, S. M., Onwe, J. C., Nwulu, N., Bekun, F. V., et al. (2022). Can energy efficiency help in achieving carbon-neutrality pledges? A developing country perspective using dynamic ARDL Simulations. Sustainability 14 (13), 7537. doi:10.3390/su14137537
Huang, Y., Chen, X., Zhu, H., Huang, C., and Tian, Z. (2019). The heterogeneous effects of FDI and foreign trade on CO2 emissions: Evidence from China. Math. Problems Eng. 2019, 9612492. doi:10.1155/2019/9612492
Jahanger, A. (2021). Influence of FDI characteristics on high-quality development of China’s economy. Environ. Sci. Pollut. Res. 28 (15), 18977–18988. doi:10.1007/s11356-020-09187-0
Jahanger, A., Usman, M., and Ahmad, P. (2022). A step towards sustainable path: The effect of globalization on China’s carbon productivity from panel threshold approach. Environ. Sci. Pollut. Res. 29 (6), 8353–8368. doi:10.1007/s11356-021-16317-9
Javorcik, B. S. (2004). Does foreign direct investment increase the productivity of domestic firms? In search of spillovers through backward linkages. Am. Econ. Rev. 94 (3), 605–627. doi:10.1257/0002828041464605
Javorcik, B. S., and Spatareanu, M. (2011). Does it matter where you come from? Vertical spillovers from foreign direct investment and the origin of investors. J. Dev. Econ. 96 (1), 126–138. doi:10.1016/j.jdeveco.2010.05.008
Jiang, X., and Zhao, S. (2019). Does FDI inhibit carbon emissions from the perspective of dual environmental regulation—an empirical study based on dynamic system GMM estimation and threshold model. J. Int. Trade 03, 115–130. doi:10.13510/j.cnki.jit.2019.03.009
Jun, W., Zakaria, M., Shahzad, S. J. H., and Mahmood, H. (2018). Effect of FDI on pollution in China: New insights based on wavelet approach. Sustainability 10 (11), 3859. doi:10.3390/su10113859
Kumar, N. (2002). Globalization and the quality of foreign direct investment. New Delhi: Oxford University Press.
Lan, J., Kakinaka, M., and Huang, X. (2012). Foreign direct investment, human capital and environmental pollution in China. Environ. Resour. Econ. (Dordr). 51 (2), 255–275. doi:10.1007/s10640-011-9498-2
Li, J., and Lin, B. (2017). Rebound effect by incorporating endogenous energy efficiency: A comparison between heavy industry and light industry. Appl. Energy 200, 347–357. doi:10.1016/j.apenergy.2017.05.087
Li, R., and Ramanathan, R. (2020). Can environmental investments benefit environmental performance? The moderating roles of institutional environment and foreign direct investment. Bus. Strategy Environ. 29 (8), 3385–3398. doi:10.1002/bse.2578
Lin, M., and Kwan, Y. K. (2016). FDI technology spillovers, geography, and spatial diffusion. Int. Rev. Econ. Finance 43, 257–274. doi:10.1016/j.iref.2016.02.014
Liu, J., Qu, J., and Zhao, K. (2019). Is China’s development conforms to the Environmental Kuznets Curve hypothesis and the pollution haven hypothesis? J. Clean. Prod. 234, 787–796. doi:10.1016/j.jclepro.2019.06.234
Mahmood, H., Alkhateeb, T. T. Y., and Furqan, M. (2020). Exports, imports, foreign direct investment and CO2 emissions in North Africa: Spatial analysis. Energy Rep. 6, 2403–2409. doi:10.1016/j.egyr.2020.08.038
Nathaniel, S., Aguegboh, E., Iheonu, C., Sharma, G., and Shah, M. (2020). Energy consumption, FDI, and urbanization linkage in coastal mediterranean countries: Re-assessing the pollution haven hypothesis. Environ. Sci. Pollut. Res. 27 (28), 35474–35487. doi:10.1007/s11356-020-09521-6
Nejati, M., and Taleghani, F. (2022). Pollution halo or pollution haven? A cge appraisal for Iran. J. Clean. Prod. 344, 131092. doi:10.1016/j.jclepro.2022.131092
Pan, X., Guo, S., Han, C., Wang, M., Song, J., and Liao, X. (2020). Influence of FDI quality on energy efficiency in China based on seemingly unrelated regression method. Energy 192, 116463. doi:10.1016/j.energy.2019.116463
Pargal, S., and Wheeler, D. (1996). Informal regulation of industrial pollution in developing countries: Evidence from Indonesia. J. political Econ. 104 (6), 1314–1327. doi:10.1086/262061
Pazienza, P. (2019). The impact of FDI in the OECD manufacturing sector on CO2 emission: Evidence and policy issues. Environ. Impact Assess. Rev. 77, 60–68. doi:10.1016/j.eiar.2019.04.002
Ren, S., Yuan, B., Ma, X., and Chen, X. (2014). International trade, FDI (foreign direct investment) and embodied CO2 emissions: A case study of chinas industrial sectors. China Econ. Rev. 28, 123–134. doi:10.1016/j.chieco.2014.01.003
Reppelin-Hill, V. (1999). Trade and environment: An empirical analysis of the technology effect in the steel industry. J. Environ. Econ. Manag. 38 (3), 283–301. doi:10.1006/jeem.1999.1085
Sapkota, P., and Bastola, U. (2017). Foreign direct investment, income, and environmental pollution in developing countries: Panel data analysis of Latin America. Energy Econ. 64, 206–212. doi:10.1016/j.eneco.2017.04.001
Schmalensee, R., Stoker, T. M., and Judson, R. A. (1998). World carbon dioxide emissions: 1950–2050. Rev. Econ. Statistics 80 (1), 15–27. doi:10.1162/003465398557294
Shahbaz, M., Gozgor, G., Adom, P. K., and Hammoudeh, S. (2019). The technical decomposition of carbon emissions and the concerns about FDI and trade openness effects in the United States. Int. Econ. 159, 56–73. doi:10.1016/j.inteco.2019.05.001
Shahbaz, M., Nasreen, S., Abbas, F., and Anis, O. (2015). Does foreign direct investment impede environmental quality in high-middle-and low-income countries? Energy Econ. 51, 275–287. doi:10.1016/j.eneco.2015.06.014
Singhania, M., and Saini, N. (2021). Demystifying pollution haven hypothesis: Role of FDI. J. Bus. Res. 123, 516–528. doi:10.1016/j.jbusres.2020.10.007
Soutter, A. R. B., and Mõttus, R. (2020). Global warming” versus “climate change”: A replication on the association between political self-identification, question wording, and environmental beliefs. J. Environ. Psychol. 69, 101413. doi:10.1016/j.jenvp.2020.101413
Sultana, N., and Turkina, E. (2020). Foreign direct investment, technological advancement, and absorptive capacity: A network analysis. Int. Bus. Rev. 29 (2), 101668. doi:10.1016/j.ibusrev.2020.101668
Sun, H., Edziah, B. K., Sun, C., and Kporsu, A. K. (2019). Institutional quality, green innovation and energy efficiency. Energy policy 135, 111002. doi:10.1016/j.enpol.2019.111002
Tang, C. F., and Tan, B. W. (2015). The impact of energy consumption, income and foreign direct investment on carbon dioxide emissions in Vietnam. Energy 79, 447–454. doi:10.1016/j.energy.2014.11.033
UNFCCC (2021). COP26 Reaches Consensus on key Actions to address climate change. Available at: https://unfccc.int/news/cop26-reaches-consensus-on-key-actions-to-address-climate-change.
Wang, F., He, J., and Niu, Y. (2022). Role of foreign direct investment and fiscal decentralization on urban haze pollution in China. J. Environ. Manag. 305, 114287. doi:10.1016/j.jenvman.2021.114287
Wang, Y., Liao, M., Wang, Y., Malik, A., and Xu, L. (2019). Carbon emission effects of the coordinated development of two-way foreign direct investment in China. Sustainability 11 (8), 2428. doi:10.3390/su11082428
Wei, K., Yao, S., and Liu, A. (2009). Foreign direct investment and regional inequality in China. Rev. Dev. Econ. 13 (4), 778–791. doi:10.1111/j.1467-9361.2009.00516.x
Xie, Q., and Sun, Q. (2020). Assessing the impact of FDI on PM2. 5 concentrations: A nonlinear panel data analysis for emerging economies. Environ. Impact Assess. Rev. 80, 106314. doi:10.1016/j.eiar.2019.106314
Xu, C., Zhao, W., Zhang, M., and Cheng, B. (2021). Pollution haven or halo? The role of the energy transition in the impact of FDI on SO2 emissions. Sci. Total Environ. 763, 143002. doi:10.1016/j.scitotenv.2020.143002
Yao, S., Wang, P., Zhang, J., and Ou, J. (2016). Dynamic relationship between China’s inward and outward foreign direct investments. China Econ. Rev. 40, 54–70. doi:10.1016/j.chieco.2016.05.005
Ye, P., Xia, S., Xiong, Y., Li, Y., and Chen, L. (2022). Can the natural resources capitalization contribute to the achievement of carbon neutrality? A pilot experiment evidence from China. Front. Environ. Sci. 48. doi:10.3389/fenvs.2022.831840
Keywords: FDI, quality characteristics, CO2 emission intensity, technique effect, composition effect
Citation: Ma W, Liu K, Li Y and Zhang H (2022) The impact of FDI quality characteristics on carbon emission intensity: Evidence from China. Front. Environ. Sci. 10:998915. doi: 10.3389/fenvs.2022.998915
Received: 20 July 2022; Accepted: 29 July 2022;
Published: 17 August 2022.
Edited by:
Atif Jahanger, Hainan University, ChinaReviewed by:
Min Wang, Shanghai Normal University, ChinaJoshua Chukwuma Onwe, Federal Polytechnic Ohodo, Nigeria
Xu Wenyun, Jiangxi University of Finance and Economics, China
Muhammad Usman, Wuhan University, China
Copyright © 2022 Ma, Liu, Li and Zhang. This is an open-access article distributed under the terms of the Creative Commons Attribution License (CC BY). The use, distribution or reproduction in other forums is permitted, provided the original author(s) and the copyright owner(s) are credited and that the original publication in this journal is cited, in accordance with accepted academic practice. No use, distribution or reproduction is permitted which does not comply with these terms.
*Correspondence: Kai Liu, bGl1a2FpQHp1ZWwuZWR1LmNu