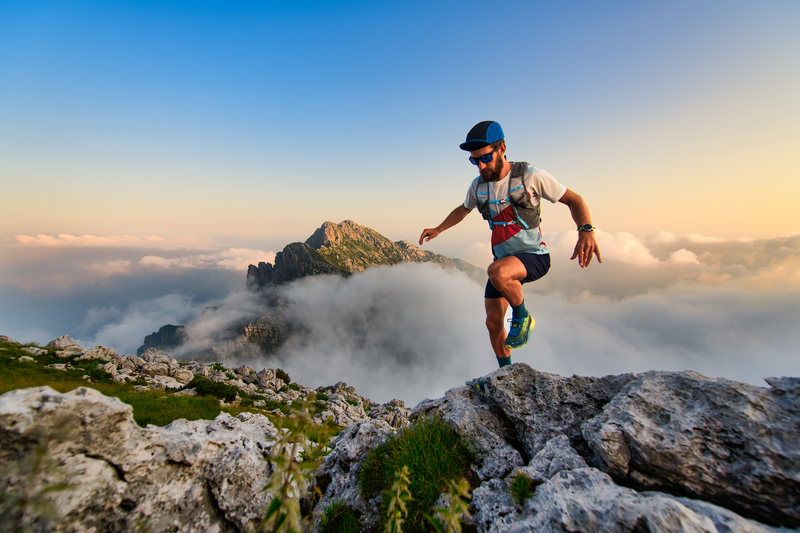
95% of researchers rate our articles as excellent or good
Learn more about the work of our research integrity team to safeguard the quality of each article we publish.
Find out more
EDITORIAL article
Front. Environ. Sci. , 10 May 2022
Sec. Environmental Informatics and Remote Sensing
Volume 10 - 2022 | https://doi.org/10.3389/fenvs.2022.917590
This article is part of the Research Topic Big Earth Data Intelligence for Environmental Modeling View all 15 articles
Editorial on the Research Topic
Big Earth Data Intelligence for Environmental Modeling
Environmental modeling involves the processes of analyzing the interactions between geophysical, biological, economic, and social systems (Ma et al., 2018; Xu et al., 2020; Zhang et al., 2020; Li et al., 2021). Environmental modeling can be used to understand environmental systems, or for performing interdisciplinary analysis that can support decisions and policy. Model-driven methods have remained dominant, especially for traditional and classical problems that deal with Big Earth Data that come from various data sources with different formats and scales. With recent breakthroughs in data acquisition technology, Big Earth Data can provide globally established, multi-source, multi-scale, high-dimensional, heterogeneous, high-resolution, and highly dynamic datasets. Big Earth Data, with its emerging technologies and approaches, may be an evolution in earth observation, and it also may allow us to use and apply them to various domains in new ways (Sudmanns et al., 2020; Zhang L et al., 2021). At the same time, artificial intelligence is rapidly progressing thanks to advanced algorithm architectures (He et al., 2016), powerful computing devices, and large available datasets (Deng et al., 2009; Liu et al., 2022). The convergence between Big Earth Data and artificial intelligence could open a new era for the advance of environmental modeling. Big Earth Data Intelligence provides new opportunities to understand the environmental modeling of earth systems which helps to resolve problems, such as spatiotemporal complement, data assimilation and fusion (Armstrong et al., 2019; Liu et al., 2021; Zhang L et al., 2021; Zhang Z et al., 2021), uncertainty (Zhang et al., 2014; Zhang et al., 2018), and model calibration. It also promotes development and presents new challenges for remote sensing of the environment (Yuan et al., 2020).
With this topic on Big Earth Data Intelligence in environmental modeling, we try to introduce the latest theory and methods of applying Big Earth Data to environmental science. It contains 14 papers that demonstrate the latest research to advance the science in research areas such as forest, ocean, ecosystem, coast, water, and weather information.
Forest mapping and modeling is an important research area for understanding environmental modeling. The forest cover change in Africa was analyzed by a data-driven method by Xiao et al. and the driving effects of the population, economy, and cultivated land expansion, were analyzed using geographical spatial heterogeneity. Urban plantation tree detection is another very challenging problem because of the irregularity in the shape of a tree canopy. The authors make an attempt to use single shot multibox (Zheng et al.) and apply YOLOv4 (Zheng et al.) for tree detection with high-resolution remote sensing imagery. To satisfy the temporal requirement in forest active fires, Hong et al. use a novel convolutional neural network to learn and search for fire spot features in Himawari-8 satellite images.
The ocean attracts much attention from researchers for its unique role in global change. As an example of the breadth of subjects covered, manuscripts included the attempt by Xiao et al. to generate sea surface temperature products by fusion of microwave and infrared data. By procuring global concentrations of particulate carbon and nitrogen in different depths, and by analyzing particulate organic carbon-to-nitrogen ratio variations, Xiao et al.pointed out that ocean currents will have a more extensive and profound impact on the ocean carbon and nitrogen cycle. By investigating the physiological responses to different light and nitrogen levels of sargassum muticum, Yan et al. showed that higher light and/or nitrogen levels generally promoted the photosynthesis and growth of the algae. In the study on the coast, Yang et al. use the new deep architecture of vision transformers to extract the waterline for an artificial coast, and Xiao et al. construct a comprehensive platform based on remote sensing images to monitor coastal tidal mudflat ecological development. Different from the ocean, groundwater plays a role in the ecosystem in its own way. Liang et al. carry out research on water table depth prediction in Baoding city, North China Plain by combining wavelet transform with a long short-term memory neural network.
Big Earth Data are guiding us to take an interest in environmental modeling in a new way. The grassland ecosystem was modeled by an optimality-based spatial explicit ecohydrological model at watershed scale by Chen et al., and urban ecology was evaluated by high-resolution remote-sensing data (Huang et al.). Furthermore, remote sensing data such as temporal-spatial data (Ma et al.) and weather radar data (Lu et al.) are good application scenarios for using new artificial intelligence methods and give more support for the study of environmental modeling.
With these issues in mind, we try to present the current state-of-the-art theoretical, methodological, and application research on environmental modeling using Big Earth Data Intelligence. Most of the manuscripts are related to the topic of environmental modeling and geospatial big data analytics. We hope that these high-quality contributions will inspire the readers in their research.
PL wrote the first draft. All authors revised the article and approved the submitted version.
This work was supported by NSFC (Nos. 61731022, 41971397).
The authors declare that the research was conducted in the absence of any commercial or financial relationships that could be construed as a potential conflict of interest.
All claims expressed in this article are solely those of the authors and do not necessarily represent those of their affiliated organizations, or those of the publisher, the editors, and the reviewers. Any product that may be evaluated in this article, or claim that may be made by its manufacturer, is not guaranteed or endorsed by the publisher.
We are very grateful to all our colleagues who submitted, reviewed, and edited manuscripts for this Research Topic.
Armstrong, M. P., Wang, S., and Zhang, Z. (2019). The Internet of Things and Fast Data Streams: Prospects for Geospatial Data Science in Emerging Information Ecosystems. Cartogr. Geogr. Inf. Sci. 46 (1), 39–56. doi:10.1080/15230406.2018.1503973
Deng, J., Dong, W., Socher, R., Li, L. J., Li, K., and Fei-Fei, L. (2009). “June. ImageNet: A Large-Scale Hierarchical Image Database,” in 2009 IEEE conference on computer vision and pattern recognition (IEEE), 248–255.
He, K., Zhang, X., Ren, S., and Sun, J. (2016). “Deep Residual Learning for Image Recognition,” in Proceedings of the IEEE conference on computer vision and pattern recognition, 770–778. doi:10.1109/cvpr.2016.90
Li, Y., Gao, H., Allen, G. H., and Zhang, Z. (2021). Constructing Reservoir Area-Volume-Elevation Curve from TanDEM-X DEM Data. IEEE J. Sel. Top. Appl. Earth Obs. Remote Sens. 14, 2249–2257. doi:10.1109/jstars.2021.3051103
Liu, P., Li, J., Wang, L., and He, G. (2022). “A Review on Remote Sensing Data Fusion with Generative Adversarial Networks (GAN),” in IEEE Geoscience and Remote Sensing Magazine, Just Accepted (March 2022) (IEEE).
Liu, P., Wang, L., Ranjan, R., He, G., and Zhao, L. (2021). A Survey on Active Deep Learning: from Model-Driven to Data-Driven. ACM Comput. Surv. 1, 1. doi:10.1145/3510414
Ma, C., Yang, J., Chen, F., Ma, Y., Liu, J., Li, X., et al. (2018). Assessing Heavy Industrial Heat Source Distribution in China Using Real-Time VIIRS Active Fire/hotspot Data. Sustainability 10 (12), 4419. doi:10.3390/su10124419
Sudmanns, M., Tiede, D., Lang, S., Bergstedt, H., Trost, G., Augustin, H., et al. (2020). Big Earth Data: Disruptive Changes in Earth Observation Data Management and Analysis? Int. J. Digital Earth 13 (7), 832–850. doi:10.1080/17538947.2019.1585976
Xu, B., Li, Y., Han, F., Zheng, Y., Ding, W., Zhang, C., et al. (2020). The Transborder Flux of Phosphorus in the Lancang-Mekong River Basin: Magnitude, Patterns and Impacts from the Cascade Hydropower Dams in China. J. Hydrology 590, 125201. doi:10.1016/j.jhydrol.2020.125201
Yuan, Q., Shen, H., Li, T., Li, Z., Li, S., Jiang, Y., et al. (2020). Deep Learning in Environmental Remote Sensing: Achievements and Challenges. Remote Sens. Environ. 241, 111716. doi:10.1016/j.rse.2020.111716
Zhang, L., Liu, P., Zhao, L., Wang, G., Zhang, W., and Liu, J. (2021). Air Quality Predictions with a Semi-supervised Bidirectional LSTM Neural Network. Atmos. Pollut. Res. 12 (1), 328–339. doi:10.1016/j.apr.2020.09.003
Zhang, Z., Demšar, U., Rantala, J., and Virrantaus, K. (2014). A Fuzzy Multiple-Attribute Decision-Making Modelling for Vulnerability Analysis on the Basis of Population Information for Disaster Management. Int. J. Geogr. Inf. Sci. 28 (9), 1922–1939. doi:10.1080/13658816.2014.908472
Zhang, Z., Demšar, U., Wang, S., and Virrantaus, K. (2018). A Spatial Fuzzy Influence Diagram for Modelling Spatial Objects' Dependencies: a Case Study on Tree-Related Electric Outages. Int. J. Geogr. Inf. Sci. 32 (2), 349–366. doi:10.1080/13658816.2017.1385789
Zhang, Z., Laakso, T., Wang, Z., Pulkkinen, S., Ahopelto, S., Virrantaus, K., et al. (2020). Comparative Study of AI-Based Methods-Application of Analyzing Inflow and Infiltration in Sanitary Sewer Subcatchments. Sustainability 12 (15), 6254. doi:10.3390/su12156254
Zhang, Z., Wang, Z., Li, A., Ye, X., Usery, E. L., and Li, D. (2021). “November). An Al-Based Spatial Knowledge Graph for Enhancing Spatial Data and Knowledge Search and Discovery,” in Proceedings of the 1st ACM SIGSPATIAL International Workshop on Searching and Mining Large Collections of Geospatial Data, 13–17.
Keywords: Big Earth Data, artificial intelligence, environmental modeling, earth system, remote sensing of environment
Citation: Liu P, Haq MA and Zhan Y (2022) Editorial: Big Earth Data Intelligence for Environmental Modeling. Front. Environ. Sci. 10:917590. doi: 10.3389/fenvs.2022.917590
Received: 11 April 2022; Accepted: 20 April 2022;
Published: 10 May 2022.
Edited and reviewed by:
Alexander Kokhanovsky, Telespazio Belgium, GermanyCopyright © 2022 Liu, Haq and Zhan. This is an open-access article distributed under the terms of the Creative Commons Attribution License (CC BY). The use, distribution or reproduction in other forums is permitted, provided the original author(s) and the copyright owner(s) are credited and that the original publication in this journal is cited, in accordance with accepted academic practice. No use, distribution or reproduction is permitted which does not comply with these terms.
*Correspondence: Peng Liu, liupeng@radi.ac.cn
Disclaimer: All claims expressed in this article are solely those of the authors and do not necessarily represent those of their affiliated organizations, or those of the publisher, the editors and the reviewers. Any product that may be evaluated in this article or claim that may be made by its manufacturer is not guaranteed or endorsed by the publisher.
Research integrity at Frontiers
Learn more about the work of our research integrity team to safeguard the quality of each article we publish.