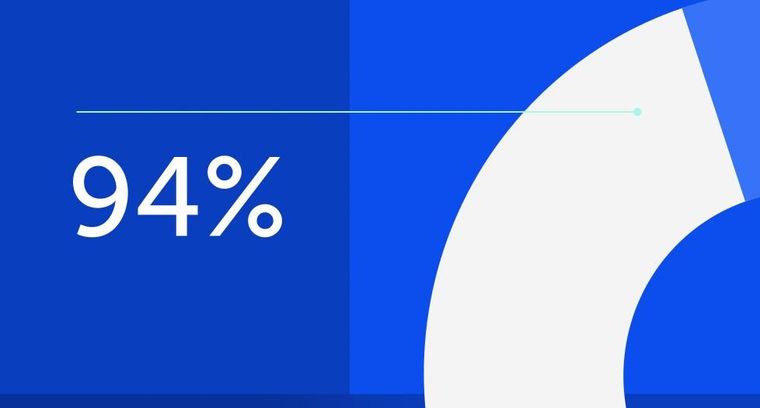
94% of researchers rate our articles as excellent or good
Learn more about the work of our research integrity team to safeguard the quality of each article we publish.
Find out more
REVIEW article
Front. Environ. Sci., 19 May 2022
Sec. Biogeochemical Dynamics
Volume 10 - 2022 | https://doi.org/10.3389/fenvs.2022.866082
This article is part of the Research TopicPhysical and Biogeochemical Processes Driving Methane Sources, Sinks and Emissions in Aquatic Systems: The Past, Present and Future under Global ChangeView all 24 articles
Key challenges to regionalization of methane fluxes in the Amazon basin are the large seasonal variation in inundated areas and habitats, the wide variety of aquatic ecosystems throughout the Amazon basin, and the variability in methane fluxes in time and space. Based on available measurements of methane emission and areal extent, seven types of aquatic systems are considered: streams and rivers, lakes, seasonally flooded forests, seasonally flooded savannas and other interfluvial wetlands, herbaceous plants on riverine floodplains, peatlands, and hydroelectric reservoirs. We evaluate the adequacy of sampling and of field methods plus atmospheric measurements, as applied to the Amazon basin, summarize published fluxes and regional estimates using bottom-up and top-down approaches, and discuss current understanding of biogeochemical and physical processes in Amazon aquatic environments and their incorporation into mechanistic and statistical models. Recommendations for further study in the Amazon basin and elsewhere include application of new remote sensing techniques, increased sampling frequency and duration, experimental studies to improve understanding of biogeochemical and physical processes, and development of models appropriate for hydrological and ecological conditions.
Emissions of methane from inland aquatic ecosystems are large and highly variable (Saunois et al., 2020; Rosentreter et al., 2021). Hence, estimating regional and global emissions is important and challenging. Bottom-up extrapolations often lack sufficient measurements for robust estimates. Simulation models of fluxes and aircraft or satellite analyses of atmospheric concentrations and emissions have other limitations and uncertainties (Ma et al., 2021). Tropical wetlands, in particular, are large, natural sources of methane and their interannual variations in area contribute to varying atmospheric concentrations (Nisbet et al., 2016; Pandey et al., 2017). As the climate warms, the role of tropical wetlands is likely to be enhanced (Zhang et al., 2017). With aquatic ecosystems extending over about 20% of its area (Junk et al., 2011), the Amazon basin represents a major proportion of tropical methane emissions. Hence, we use the Amazon basin as a data-rich, tropical region, and take advantage of its extensive and varied aquatic environments to illustrate and evaluate regionalization approaches, data requirements and results. The challenges considered for the Amazon basin are general to regionalization efforts elsewhere, and lessons learned can be applied to other regions, such as the warming arctic (Wik et al., 2016) or African wetlands (Lunt et al., 2021).
Aquatic ecosystems contribute to large fluxes of carbon in the Amazon basin. High rates of primary production, respiration and methanogenesis lead to fluxes of carbon dioxide and methane to the atmosphere from rivers, lakes, floodplains and other wetlands (Richey et al., 2002; Melack et al., 2004; Melack et al., 2009; Forsberg et al., 2017; Pangala et al., 2017). Though remote sensing of inundation (e.g., Hamilton et al., 2002; Parrens et al., 2019; Prigent et al., 2020) and aquatic habitats (Hess et al., 2003, 2015), inundation modeling (e.g., Coe et al., 2007; Paiva et al., 2013), and measurements of gas concentrations and fluxes in rivers, reservoirs, lakes and wetlands are available (Melack, 2016; Barbosa et al., 2020), considerable uncertainty and information gaps remain. Key challenges to regionalization of methane fluxes in the Amazon basin are the large seasonal variation in inundated areas and habitats, the wide variety of aquatic ecosystems throughout the Amazon basin, and the variability in methane fluxes in time and space. Further issues stem from the various types of methods used and difficulties estimating ebullitive fluxes.
Regionalization can be done at several scales from that of floodplain lakes and wetland types to large regions to the whole Amazon basin. Habitat-specific fluxes can be combined with estimates of habitat areas and their seasonal variations. Mechanistic models can provide an alternative way to estimate regional fluxes. Results from aircraft and satellite measurements of gas concentrations combined with atmospheric transport models can offer integrated regional estimates.
To examine challenges regionalizing methane emissions in the Amazon basin we first consider the hydrological variability and the variety of aquatic ecosystems and their spatial extent. Next the adequacy of sampling and of field methods plus atmospheric measurements, as applied to the Amazon basin, are discussed, followed by a summary of published fluxes. Then, understanding of relevant biogeochemical and physical processes in Amazon aquatic environments and their incorporation into mechanistic and statistical models are examined. Prior and current regional estimates using bottom-up and top-down approaches are reviewed and critiqued. Lastly, recommendations for further study are made.
The hydrological Amazon basin extends over ∼6 million km2 with major rivers including the Solimões, Madeira, Negro and Japurá joining to form the mainstem Amazon River with annual discharge up to about 20% of global fluvial inputs to oceans. Recent reviews and analyses offer valuable perspectives on hydrological conditions in the Amazon basin of relevance to regionalization of methane fluxes. Fassoni-Andrade et al. (2021) provide a comprehensive review of the water cycle, associated hydrological processes and relevant remote sensing advances in the Amazon basin with its high rates of precipitation, extensive floodplains, diverse tropical forests, complex topography, and large variations in freshwater storage and discharge. Melack and Coe (2021) focus on hydrological aspects of Amazon floodplains in relation to ecological processes. Fleischmann et al. (2021) present an intercomparison of 29 inundation datasets for the Amazon basin derived from remote sensing-based products, hydrological models and multi-source products, and illustrate the variety and divergences among the datasets currently available (Figures 1, 2).
FIGURE 1. Maximum and minimum inundated areas for Amazon basin <500 m asl based on different remote sensing and analysis techniques. GIEMS: Prigent et al. (2020), GIEMS-D15: Fluet-Chouinard et al. (2015), Chapman: Chapman et al. (2015), SWAF: Parrens et al. (2019), Rosenqvist: Rosenqvist et al. (2020), Hess: Hess et al. (2015).
FIGURE 2. Hydrological Amazon basin with inundated areas shown for basin <500 m asl based on different remote sensing and analysis techniques. Each 1 km pixel shows the number of basin-scale inundation datasets (total of 14 products were assessed) that agree that the pixel is floodable, according to Fleischmann et al. (2021). Rivers and wetlands mentioned in the text: Solimões—S, Madeira—Md, Negro—N, Japurá—Jp, Juruá—Ju, Purus—P, Amazonas—A, Tapajós—T, Xingu—X, Ji-Paraná—JiP, Uatumã—U, Llanos de Moxos - Mo, Pacaya-Samiria - PS, Negro interfluvial wetlands - Nif, Roraima—R, Amazon delta wetlands—Ad.
Variations in rainfall and large changes in river stage and discharge combined with backwater effects and flood propagation result in seasonal and interannual variations in extent of inundation of thousands of lakes, floodplains and other wetlands (Meade et al., 1991; Espinoza et al., 2009; Paiva et al., 2013). Annual amplitude variation in river water levels can be as high as 15 m (Fassoni-Andrade et al., 2021). Particularly high or low rainfall is linked to ENSO events and strong warming of surface waters in the tropical North Atlantic (Marengo and Espinoza 2016). Moreover, the hydrology of the Amazon is not stationary, and positive trends in maximum river water levels across the central basin are evident (Gloor et al., 2013; Barichivich et al., 2018), with several record-breaking floods in the last decade registered in cities such as Manaus (Espinoza et al., 2021). The hydrology of floodplains and other wetlands combines inputs from local catchments with regional-scale fluxes and is characterized by variations in the amplitude, duration, frequency, and predictability of inundation and a seasonally flooded ecotone, called the aquatic-terrestrial transition zone, that often contains woody and herbaceous vegetation (Melack and Coe 2021).
Inundation extent can be simulated with process-based models, and models have been applied at the scale of specific lakes (Ji et al., 2019), floodplain reaches (Wilson et al., 2007; Rudorff et al., 2014 a,b; Pinel et al., 2019) and the whole basin (e.g., Coe et al., 2007; Yamazaki et al., 2011; Miguez-Macho and Fan 2012; Paiva et al., 2013). Apers et al. (2022) used literature-based parameters for natural peatlands to develop and integrate a tropical peatland hydrology module into a global land surface model; global meteorological reanalysis data were used as inputs. In the Amazon basin both lowland and Andean peatland hydrology were simulated; both need further validation.
Temporal (from static to monthly intervals, up to a few decades) and spatial (at resolutions from 12.5 m to 25 km) changes in water level and inundation can be detected with remote sensing techniques, as summarized in Fleischmann et al. (2021). Long-term, maximum inundated area for the basin <500 m asl is estimated as ∼600000 ± ∼82,000 km2 using synthetic aperture radar (SAR)-based products, though subregional products suggest a basin-wide underestimation of ∼10%. Minimum inundation extent using SAR-based products is estimated as 139,300 ± 127,800 km2. Differences among products arise from differing characteristics of sensors, periods of acquisitions, spatial resolution, and data processing algorithms. Especially large uncertainties exist for interfluvial wetlands (Llanos de Moxos, Pacaya-Samiria, campinas and campinaranas in the Negro basin, Roraima), where inundation tends to be shallower and more variable in time than along riverine floodplains.
While quite useful, remote sensing and modeling results do have limitations. Gauges of river stage are widely spaced, and floodplains are ungauged with a few exceptions; satellite-borne altimeters have wide spacing along tracks, though work fairly well for rivers. Gravity anomaly sensors based on the GRACE missions (Tapley et al., 2004) have been used to monitor changes in floodplain water storage at the basin scale (Alsdorf et al., 2010). For monitoring inundation dynamics, passive microwave has coarse spatial resolution, and SAR data have limited temporal or spatial coverage, though new sensors offer repeated regional coverage. Forthcoming missions, such as the Surface Water and Ocean Topography (SWOT) and the NASA-ISRO Synthetic Aperture Radar (NISAR) missions, will provide useful data to monitor the extent and water levels of Amazonian wetlands. Topographic and bathymetric data at high vertical resolution are fundamental to understand the dynamics of floodplain water storage, but they are rare, though improved digital elevation models are now becoming available (see https://eop-cfi.esa.int/index.php/docs-and-mission-data/dem for list; including the Copernicus global 30 m product; O’Loughlin et al., 2016; Nardi et al., 2019; Fassoni-Andrade et al., 2020).
Aquatic ecosystems in the basin range from small streams to large rivers fringed by floodplains, lakes and seasonally flooded forests and savannas. Topographic, climatic, and landscape features range from Andean highlands in the west to lowlands across the central and eastern basin. Based on information available for climate, hydrology, water, sediments and plants, Junk et al. (2011) classified 14 major types of natural wetlands in the lowland Amazon. The amplitude, duration, frequency and predictability of inundation are key criteria in their classification, though these hydrological aspects have insufficient spatial and temporal data for many parts of the basin. Furthermore, a functional classification of these aquatic ecosystems in terms of their relevance to methane biogeochemistry and flux, as encouraged by Sahagian and Melack (1998), is lacking. Hence, our first challenge to regionalizing methane emissions concerns the appropriate characterization of the varied systems, their spatial extent and temporal variability.
Based on available measurements of methane emission and areal extent, seven types of aquatic systems will be considered: streams and rivers, lakes, seasonally flooded forests, seasonally flooded savannas and other interfluvial wetlands, herbaceous plants on riverine floodplains, peatlands, and hydroelectric reservoirs. Aquatic habitats with insufficient information about methane fluxes include coastal freshwater wetlands, such as the wetlands on Marajós Island in the Amazon delta, agricultural ponds (Macedo et al., 2013), road-blocked streams (Leitão et al., 2018), tank bromeliads and cultivated rice. More broadly, retarded drainage can lead to saturated soils without standing water throughout the basin with unknown extent or duration. Estimates of areal extent, judged as the most reliable with caveats, are provided. General issues are the regional boundaries represented by different datasets, as well as inherent limitations of the methods and their validation.
Given the Amazon basin’s immense scale, variability and difficult access, remote sensing approaches are essential to characterize the aquatic systems. Aquatic habitats range in dimension from headwater streams (<1 m across) to ponds and large lakes to floodplains tens of kilometers wide to wetlands covering tens of thousands of km2, adding further challenges. Passive and active microwave, laser, visible and near-infrared and gravity anomaly detection systems have been applied in the Amazon basin (Melack 2004; Fassoni-Andrade et al., 2021). SAR techniques are of particular utility because inundation under vegetation and relevant types of aquatic vegetation can be detected, and data can be acquired during day or night and under clouds (e.g., Kasischke et al., 1997; Silva et al., 2015). Melack and Hess (2010) and Hess et al. (2015) used the methodology described in Hess et al. (2003) to determine floodable area, inundated area, and areal extent of major habitats permanently or periodically inundated in the lowland Amazon basin based on mosaics of SAR data obtained during low and high river stages in 1995 and 1996. Open water and herbaceous and woody plants within floodable regions were distinguished at a spatial resolution of about 100 m. High-resolution airborne videography and laser altimetry were used to validate the classifications (Hess et al., 2002). Other remotely sensed products that characterize aquatic habitats are available for specific locations (e.g., Silva et al., 2010; Renó et al., 2011; Hawes et al., 2012; Arnesen et al., 2013; Ferreira-Ferreira et al., 2015).
Allen and Pavelsky (2018) used Landsat and river stage data to determine river widths at mean annual discharge and judged their estimates accurate for widths wider than 90 m. Since the HydroBASINS dataset used for the river network is derived from a single flow direction algorithm, it cannot represent braided channels, such as occur in some reaches of Amazonian rivers. Their river channel, surface area estimate within the Amazon basin is ∼58,000 km2. Mouthbays of rivers, such as the Tapajós, Xingu, Tefé and Coari, were considered lakes, and the analysis did not include the whole delta. Combining their river channel area with estimates for the delta (9,500 km2, Castello et al., 2013 plus L.L. Hess, personal communication) and mouthbays of the Tapajós and Xingu (3,800 km2, Sawakuchi et al., 2014) results in a river channel area of 77,500 km2. An analysis for drainage areas from 1 to 431,000 km2 and channels >2 m in width used hydraulic geometry and the drainage network to estimate the Amazon basin (excluding the delta and mouthbays) to have a combined area of about 60,000 km2 (Beighley and Gummadi 2011). Given that this procedure included smaller rivers than Allen and Pavelsky’s analysis and used independent data, the two estimates are quite similar. As another example, Rasera et al. (2008) developed empirical relationships between drainage area and channel widths combined with river lengths derived from a digital river network to determine the area of streams and rivers in the Ji-Paraná River basin. Assuming their relationship applied to the whole lowland Amazon basin, an area of 23,000 km2 would result for rivers from third to sixth order, similar to that estimated by Beighley and Gummadi (2011) for rivers in that size range. These estimates do not include headwater streams (zero or first orders) that require high resolution topography quite difficult to obtain under forest canopies or labor-intensive surveying. Riparian corridors, often with saturated soils, periodically flooded (Chambers et al., 2004), are also not included.
Large Amazon rivers are typically considered “white waters” with near-neutral pH and relatively high suspended sediment and nutrient concentrations (e.g., Amazon, Madeira, Purus, Juruá and Japurá), “black waters” with low pH, nutrients and suspended sediments and high dissolved organic carbon (e.g., Negro, Uatumã), and “clear waters” with low to neutral pH and low nutrients, suspended sediments and dissolved organic carbon (e.g., Tapajós and Xingu) (Mayorga and Aufdenkampe 2002; Junk et al., 2011). These three types of river water were classified for 6th to 11th order rivers based on field observations and visual inspection of optical images by Venticinque et al. (2016). Smaller rivers and streams are likely to vary considerably in chemical composition, but regional characterization is lacking.
If calculated as the difference between SAR analysis of open water areas at ∼100 m resolution for a period with near-maximum inundation in the central lowland Amazon basin (Hess et al., 2015) minus the river channel areas for rivers wider than 90 m (Allen and Pavelsky 2018), an area of ∼20,000 km2 results. Since the river channel areas were derived for the whole basin and the SAR analysis applies only to the basin <500 asl, the lake area is probably under estimated. The HydroLAKES database (Messager et al., 2016) subsetted for the Amazon basin has a lake area of ∼23,000 km2. This data set for lakes >0.1 km2 used several data sources, including the SRTM waterbody data (Slater et al., 2006), and underwent manual removal of river and wetland polygons and other corrections.
Using side-looking airborne radar imagery acquired mostly during periods of low to moderate inundation by Projecto RadamBrasil (e.g., Departmento Nacional da Produção Mineral), Sippel et al. (1992) measured lake areas on the mainstem floodplain from 51o to 70o W and along the lower 400 km of the Japurá, Purus, Negro and Madeira rivers totaling ∼11,800 km2.
Radar mosaics and maps generated from these images, both at 1:250,000 scale, allowed areas ≥∼0.05 km2 to be estimated. Hamilton et al. (1992) found that the areas of lakes on the Amazon floodplains appear to follow the Pareto distribution, with censorship and truncation on both ends, which indicates that the lakes are statistically self-similar and that descriptive statistics for the lakes will vary with the scale of observation.
Seasonal and interannual variations in inundation lead to a range of lake areas and associated depths that are not represented by the available regional estimates. Results for well-studied lakes provide an indication of variations: Calado, 2–8 km2 (Lesack and Melack 1995), Janauacá, 23–390 km2 (Pinel et al., 2015), Curuai, 850–2,250 km2 (Rudorff et al., 2014a); these values include some flooded vegetation at high stages. Limnological and ecological conditions in Amazon floodplain lakes are reviewed in Melack and Forsberg (2001), Melack et al. (2009), Melack et al. (2021), and Junk (1997).
The SAR-based analysis used by Hess et al. (2015) is well validated for detection of inundated forests. By combining the proportion of woody vegetation (forest, woodland and shrubs) compared to total inundated areas at the time of the high (70%) and low (62%) water levels analyzed by Hess et al. with the maximum (631,000 km2) and minimum (53,000 km2) inundated areas from Parrens et al. (2019), maximum (442,000 km2) and minimum (∼33,000 km2) flooded forest areas can be estimated. Seasonally flooded forests vary considerably in species diversity and composition, biomass and productivity depending on fertility of the sediments, duration of inundation and ecological factors not fully understood (Junk et al., 2010). Most flooded forests are associated with white-water rivers, and called várzea forests. Those along black-water rivers are called igapó forests, cover up to ∼84,000 km2, and those near clear-water rivers that include várzea and igapó forests, are estimated to cover up to ∼50,000 km2, based on the maximum flooded forest area calculated above and proportional areas from Melack and Hess (2010).
Seasonally inundated savannas with a variety of herbaceous plants and palms and other trees occur in Roraima (Brazil) and Llanos de Moxos (Bolivia) (Melack and Hess 2010; Junk et al., 2011). Interannual maxima, minima and mean inundation over an 8-year period in these two regions are provided by Hamilton et al. (2002): Roraima (16,500, 250 and 3,000 km2) and Llanos de Moxos (83,000, 6,100 and 34,000 km2). The Rupununi savannas, near Roraima are similar, and may reach a maximum area of ∼15,000 km2 (Junk et al., 2011).
Other interfluvial wetlands occur in several regions within the Amazon basin and are not well studied (Junk et al., 2011). In the middle Negro basin ∼30,000 km2 of wetlands with sedges, other aquatic plants, patches of palm, mainly Mauritia flexuosa, and open water occur; Frappart et al. (2005) estimated a similar inundated area for this region. A time series of SAR data was used to determine inundation duration, extent and vegetation in the Cuini (minimum, 784 km2; maximum, 964 km2), and Itu (minimum, 550 km2; maximum, 762 km2) wetlands, both located near the middle Negro River (Melack et al., 2009; Belger et al., 2011). Fleischmann et al. (2020) compared several inundation datasets for the Negro interfluvial wetlands associated with campinas and campinaranas (Rossetti et al., 2017), with maximum inundation extent reaching up to 20,000 km2. The muted variation in area of these wetlands is likely owed to the limited hydrological connection to the rivers. Between the Purus and Madeira rivers wetland patches a few hectares to ∼150 km2, totaling approximately 5,000 km2 occur (Junk et al., 2011).
Herbaceous plants are abundant throughout the white-water floodplains of the Amazon basin. These plants grow profusely on sediments during low water and as rooted emergent and as free-floating plants, as inundation and water depths increase seasonally (Junk and Piedade 1997). Common C4 grasses are Echinochloa polystachya, Paspalum repens and Paspalum fasciculatum; other plants include the C3 grass, Hymenachne amplexicaulis, rice (Oryza perennis), and the genera Eichhornia, Ludwigia, Neptunia, Salvinia, and Pistia (Engle et al., 2008; Silva et al., 2013). SAR and other remotely sensed imagery can detect these plants, though their seasonal succession, growth and decline require time-series data. Also, narrow bands of floating plants fringing lakes and rivers and intermingled with woodlands can limit their accurate measurement. For the lowland basin, the combination of the proportion of inundated area represented by herbaceous vegetation in white-water river catchments and areas inundated permit an estimated area during high water of approximately 25,000 km2 (Melack and Hess 2010; Hess et al., 2015). As an example of areal variations, in floodplain lakes totaling ∼9,400 km2 along the eastern Amazon River, herbaceous plant coverage ranged from 770 to 2,900 km2, based an analysis of Radarsat and MODIS images over 2 years (Silva et al., 2013). Herbaceous plants are rarely abundant in black-water river systems, and tend to be only moderately common in clear-water rivers (Junk et al., 2011).
Insufficient information is available to determine basin-wide variations and differences.
Among the floodplain and other wetlands delineated in sections 3.3–3.5, some are likely to be peatlands, though the classification of organic-rich soils in the region as peats is not standardized, and sampling is insufficient to determine their extent. Gumbricht et al. (2017) defined peat as a soil having at least 50% organic matter in the upper 0.3 m, while others have used different criteria, for example to identify minerotrophic and ombrotrophic sites (Lähteenoja et al., 2009). Among the possible peatlands based on the analysis by Gumbricht et al. (2017), the Pacaya-Samiria wetlands in the Peruvian lowlands, composed of seasonally flooded forests, palm swamps and peatlands, have had their inundated area and peat fairly well characterized (Lähteenoja et al., 2012; Jensen et al., 2018). By combing multi-sensor remote sensing with forest censuses and cores of peat thickness Draper et al. (2014) estimated that peatlands cover 35,600 ± 2,130 km2 in the Pastaza-Marañon foreland basin (located in the Pacaya-Samiria region). A portion of this area is included within wetland areas described earlier, though during the period of the high water represented in Hess et al. (2015) fluvial flooding had receded in the Peruvian lowlands. Evidence for peat accumulation is also provided by sampling in parts of the Negro and Madre de Dios river basins, and suggested by model results (summarized in Gumbricht et al., 2017). However, the hybrid expert system used by Gumbricht et al. to estimate the regional distribution of wetlands and peatlands is not consistent with other estimates of inundation (Fleischmann et al., 2021), perhaps because of overestimation of soil moisture by the topographic index used or large rainfall in 2011, the year used. Their designation of floodplains and other wetlands as peatlands requires considerably more evidence, though recent studies are detecting peats scattered through the basin (e.g., Winton et al., 2021)
Hydroelectric reservoirs currently in the Amazon basin cover approximately 4,575 km2 (Almeida et al., 2019; Kemenes et al., 2007 for Balbina). In Bolivia (50 km2), Ecuador (35 km2) and Peru (103 km2) almost all are above 1,000 m asl while in Brazil all are <500 m asl. The area of reservoirs (∼2,600 km2) estimated by Messager et al. (2016) is too low. Plans for more hydroelectric systems, especially in the Andes, could considerably expand their area (Almeida et al., 2019) and have substantial ecological consequences (Forsberg et al., 2017).
The majority of the studies in Amazonian aquatic environments, albeit sampled infrequently and usually in open waters of lakes or rivers, represent considerable effort given the immense area and remoteness of the basin. Few studies covered a complete hydrological cycle, and rarely included diel variations or multiple years. Related limnological, hydrological and meteorological measurements were seldom done concurrently, limiting the ability to evaluate the role of ecological factors, thermal structure and other processes on CH4 fluxes and concentrations. Regions or habitats with few or no data include the Llanos de Moxos, coastal freshwater wetlands, riparian zones along streams, small reservoirs associated with agriculture, cultivated rice in Roraima and elsewhere, and habitats above 500 m. The recent findings of significant fluxes from trees, especially when inundated, included measurements in several types of forests (Pangala et al., 2017) and seasonal variations (Gauci et al., 2021), and point to the need for considerably more data on fluxes from trees given the large diversity of trees. Among hydroelectric reservoirs, measurements are available for a few (summarized in Melack et al., 2004; Guerin et al., 2006; Barros et al., 2011; Kemenes et al., 2016), but only Balbina reservoir has data collected from multiple reservoir and downstream stations as well as measurements of fluxes associated with turbines over a year (Kemenes et al., 2007, 2011). Abril et al. (2005) provide comparable, long-term data for Petit Saut reservoir in French Guyana.
Floating chambers of various designs have been used to measure diffusive fluxes based on deployments of often less than 30 min on regional surveys and at specific locations (e.g., Barlett et al., 1988; Devol et al., 1990; Kemenes et al., 2007; Barbosa et al., 2016, 2020). As CH4 accumulated in the chambers, sequential samples are removed or the gas is circulated from the chamber to a portable instrument measuring CH4 continuously; analytical methods are described below. An alternative approach is to calculate the diffusive flux (F) based on measurements of concentration of methane in the water and estimation of gas exchange velocity (k) using the following equation:
where Cw is the observed dissolved CH4 concentration and Ceq is the CH4 concentration in equilibrium with the atmosphere. To estimate k, MacIntyre et al. (2019) and MacIntyre et al. (2021) provide theoretical and empirical evidence for the validity of the surface renewal model, though most studies in Amazon lakes used wind-based equations (Engle and Melack 2000; Barbosa et al., 2016), which are likely to underestimate fluxes by a factor of two or more. For large rivers, equations for k often incorporate current velocity and/or wind speed (Alin et al., 2011), while equations applied to streams and small rivers use hydraulic parameters, such as slope and velocity (Raymond et al., 2012). In small rivers, the dominant driver for near-surface turbulence driving gas exchange has thresholds which vary with current speed and wind speed (Guseva et al., 2021). Using data from montane streams, Ulseth et al. (2019) found that turbulent diffusion is important in low-energy streams and that entrainment of air bubbles in high-energy streams enhanced gas exchange.
Pangala et al. (2017) and Gauci et al. (2021) attached chambers to tree trunks to estimate methane fluxes from the trees at several locations during a 1-month period of rising water and in seasonal deployments at several locations, respectively. Given the considerable variations in fluxes from the trees, large diversity of trees, and difficulty of extrapolating to whole trees and then forests, many more measurements of both fluxes and forest characteristics are needed to characterize fluxes from flooded and upland trees.
Although plant-mediated transport is known to occur via herbaceous plants (e.g., Villa et al., 2020), it has not been consistently observed, albeit seldom sampled, in Amazon lakes or wetlands. Wassmann et al. (1992) did not detect different fluxes between chambers with or without mats of Paspalum repens, a dominant floating grass with few roots reaching the sediments. Among the few measurements by Bartlett et al. (1988), floating mats of Eichornia or Paspalum had no or slight enhancement compared to open water, while rooted Victoria regia growing in shallow water did emit more CH4 than open water, as would be expected based on other studies of water lilies (Dacey 1981). In Eichornia stands in the southern Amazon and Pantanal, Oliveira Junior et al. (2020) found that diffusive CH4 emissions were much higher when the plants were rooted, but that emissions from free-floating plant mats were lower than those from nearby open water. In interfluvial wetlands with shallow water in the Negro basin, Belger et al. (2011) reported higher fluxes in chambers covering emergent, rooted plants at one seasonally inundated site but not at another, permanently flooded site.
Most estimates of ebullitive CH4 fluxes in Amazon lakes have been made using floating chambers during a short deployment. Devol et al. (1988), Devol et al. (1990) and Sawakuchi et al. (2014) used floating chambers with discrete sampling of gas and estimated ebullition indirectly by subtracting diffusive fluxes, calculated from an estimated gas transfer velocity and CH4 concentration gradient, from combined ebullitive and diffusive fluxes. Alternatively, chambers can be coupled to a portable gas analyzer to obtain high-frequency measurements, and CH4-enriched bubbles detected from abrupt increases in gas concentration (Crill et al., 1988; Wassmann et al., 1992; Barbosa et al., 2021). Submerged inverted funnels (bubble traps) deployed for hours to days integrate episodic fluxes. Acoustic methods to detect bubbles in the water column and estimate ebullition (DelSontro et al., 2011; Linkhorst et al., 2020) have seldom been used in the Amazon. Barbosa et al. (2021) made the most complete set of measurements to date in both vegetated habitats and open water over 2 years using floating chambers with high frequency measurements and bubble traps, and during falling water, a hydroacoustic echo sounder was employed to detect bubbles.
The variability of ebullition introduces considerable uncertainty in estimates of ebullitive and total CH4 flux, and the three approaches have different problems. Floating chambers cover a small area and are typically deployed for less than 30 min; very small bubbles cannot be detected. Bubble traps also cover small areas, and bubble volumes of less than ∼1 ml are difficult to measure. Hydroacoustic surveys capture bubbles at high spatial resolution but for only a short time interval, although moored acoustic transponders could be deployed. All three approaches are likely to underestimate ebullition.
Until recently, almost all samples from the atmosphere, bubble traps, floating chambers and dissolved in water were assayed in a gas chromatograph equipped with a flame ionization detector. Gas samples for analyses of dissolved CH4 were obtained using a headspace technique by vigorous shaking of water and air in the sampling syringe (Hamilton et al., 1995). A customized system used during the 1980s employed a gas filter correlation technique (Sebacher and Harriss 1982) with the infrared detector inside the floating chamber to generate a continous record of concentration changes. Portable, off-axis integrated cavity output spectrometers (e.g., products of Los Gatos Research, Inc. and Picarro, Inc.) are now available to measure gas samples directly from floating chambers, from equilibrators receiving water pumped from rivers or lakes, or after headspace extraction in samples. However, the equilibration time between water and gas in equilibrators varies among designs and concentrations.
Factors relevant to interpretation of methane fluxes include meteorological variables, stratification and mixing, dissolved oxygen, nutrient and other solute concentrations, current velocities and water depths, underwater light attenuation, chlorophyll and dissolved and particulate carbon concentrations, and sediment characteristics. Time-series of vertical profiles of temperature and dissolved oxygen measured with moored sensors are especially important given the strong diel variations typical of these tropical locations. Seldom do such measurements accompany those of fluxes; exceptions include Barbosa et al. (2020).
Eddy covariance is now widely used to measure methane fluxes in wetlands (Dalmagro et al., 2019; Delwiche et al., 2021) and lakes (e.g., Schubert et al., 2012; Podgrajsek et al., 2016), and has been used in short campaigns to measure CO2 fluxes in an Amazon lake (Polsenaere et al., 2013) and reservoir (Souza do Vale et al., 2016). Two studies provide methane fluxes over upland forests using tower-based measurements. Carmo et al. (2006) used a profiling system based on mixing ratio measurements at three sites in the Amazon. Querino et al. (2011) provides one of the few eddy covariance estimates over upland forest at a site north of Manaus. Both studies focused on within canopy gradients and the uppermost inlet was not more than 10 m above the canopy. A recent study using a profiling system of atmospheric mixing ratio measurements at the Amazon Tall Tower Observatory (ATTO) suggest a nighttime source of methane from flooded forests along the Uatumã River (Botía et al., 2020a). An eddy covariance flux tower is now measuring CO2 and CH4 fluxes in a natural palm peatland near Iquitos, Peru (Griffis et al., 2020).
Airborne sampling of methane and other gases, followed by assays of concentrations, and in some cases, isotopic composition, in the lower atmosphere, when combined with atmospheric transport models, have provided integrated calculations of methane fluxes over subregions of the Amazon basin (Miller et al., 2007; Beck et al., 2012; Basso et al., 2016; Wilson et al., 2016; Basso et al., 2021; Wilson et al., 2021). For example, Basso et al. (2021) reported seasonal and annual CH4 fluxes based on measurements of atmospheric CH4 in vertical profiles from ∼300 m to 4.4 km collected about twice per month from 2010 through 2018 at sites located in the southeastern, northeastern, southwest-central and northwest-central Brazilian Amazon basin. The fluxes, estimated with a column budgeting technique, include all sources and sinks within the area traversed by air masses flowing from the Atlantic coast to each site, representing regional scales of ∼105–106 km2. For each of the sites’ quarter-yearly resolved air-mass trajectory, density weighted regions of influence were calculated (Cassol et al., 2020), as illustrated for site TAB in the northwest-central Amazon basin (Figure 3). These weighted trajectories show that areas closer to the flights have greater influence than more distant regions, and that the air mass trajectories vary by season. Hence, attributing the fluxes to sources requires incorporating these spatial and temporal differences.
FIGURE 3. Weighted mean quarterly regions of influence for 2010 through 2018 for the TAB site (5.9°S, 70.0°W). Adapted from Cassol et al. (2020) and Basso et al. (2021), where methods are explained.
Results reported by Basso et al. (2021) indicate that wetlands are likely the major source of methane, at least for seasons and sites with extensive inundated areas. Other sources include fires, anthropogenic emissions from enteric fermentation by cattle (Crippa et al., 2019) and urban areas, termites (van Asperen et al., 2021), and, perhaps, emissions associated with canopies of upland forests (Carmo et al., 2006; Martinson et al., 2010). Carbon monoxide measured concomitantly with CH4 was used to estimate CH4 emissions from biomass burning. Uptake by soils also occurs (Keller et al., 2005). Though each of these upland fluxes have uncertainties and regionalization challenges, we are not examining these issues here.
Satellite retrievals of atmospheric concentrations of CH4 are now available from several sensing systems (e.g., SCIAMACHY, Frankenbert et al., 2011; GOSAT, Webb et al., 2016; Parker et al., 2020; TROPOMI, Yu et al., 2021; AIRS onboard the NASA/AQUA satellite, Ribeiro et al., 2016). For example, Webb et al. (2016) found good agreement in the seasonal patterns from airborne vertical profiles extrapolated through the atmosphere and remote sensing data from GOSAT. When combined with inverse modeling and subsetted, these analyses permit estimates of methane emissions in the Amazon basin (e.g., Frankenberg et al., 2008; Tunnicliffe et al., 2020). Evaluations of these approaches are provided by several recent studies (e.g., Parker et al., 2018).
In parallel with section 3 on areal extent, available estimates of methane emissions are summarized for seven types of aquatic systems: streams and rivers, lakes, seasonally flooded forests, seasonally flooded savannas and other interfluvial wetlands, herbaceous plants on riverine floodplains, peatlands, and hydroelectric reservoirs. A final section identifies major gaps. Fluxes judged as representative of each habitat and that span seasonal variations are selected, if possible. Values are usually expressed in mass of CH4, not molar units, and converted to daily rates by simple multiplication, as needed.
Most of the published data have expressed averaged values as arithmetic mean fluxes, and these are used here. However, since diffusive and especially episodic fluxes are not normally distributed, arithmetic means are biased toward higher values, and standard deviations often suggest unrealistic negative fluxes. As discussed by Rosentreter et al. (2021) and others, expressing results as medians with interquartile ranges offers an alternative that represents the spread. Geometric means, while statistically sensible for these skewed data, are seldom used (e.g., Barbosa et al., 2020). Applying an approach, such as Monte Carlo uncertainty analysis, would be appropriate, except very few of the published datasets include the individual measurements required to do such analysis. For example, Melack et al. (2004) were able to calculate means and Monte Carlo-based uncertainties for habitat-specific methane emissions with individual measurements reported in Devol et al. (1990).
Methane emissions based on floating chambers deployed in the Amazon River and major tributaries during low and high water, in most cases, reported by Sawakuchi et al. (2014) ranged from ∼0.2 to 297 mg CH4 m−2 d−1 with averages (as mg CH4 m−2 d−1): Amazon (21.6), Solimões (5), Negro (8.6), Madeira (0.6), Tapajós (38), Xingu (96), Preto (1.4) and Para (5). Barbosa et al. (2016) measured diffusive CH4 fluxes using floating chambers and estimated fluxes based on the concentration of the gas in the water and calculated gas exchange coefficients along a 700-km transect including four stations in the Negro River and 21 of its tributaries at low and high water plus six stations on a 1100-km transect of the Solimões-Amazon River and one on the Maderia River occupied four times. Fluxes ranged from ∼0.2 mg CH4 m−2 d−1 in the Solimões (during early falling water) to ∼3,900 mg CH4 m−2 d−1 in the Jutaí River during low falling water with averages (as mg CH4 m−2 d−1): Amazon and Solimões (18), Negro River and tributaries (54), Madeira (6.4), Purus (6.4), Juruá (5), Japurá (9.6). While ebullition may occur in rivers, as suggested by Sawakuchi et al. (2014), the indirect method used may be compromised by the wide ranges of gas exchange velocities expected in turbulent rivers.
Measurements in tributaries were made near their confluence with the Negro, Amazon or Solimões, and no upper reaches of rivers were sampled.
Additional data for the Solimões and Amazon rivers include those by Richey et al. (1988), who combined measurements of dissolved CH4 concentrations in the main stem with estimates of air–water gas exchange to estimate a diffusive evasion of 3.2 mg CH4 m−2 d−1, which is similar to 2.7 mg CH4 m−2 d−1determined by Bartlett et al. (1990), but lower than measurements by Sawakuchi et al. (2014) and Barbosa et al. (2016). In the Uatumã River, downstream of Balbina dam, emission was 2,200 mg CH4 m−2 d−1 (Kemenes et al., 2007), a value far above other rivers, with the exception of the Jutaí River. Interactions between the fringing floodplains and river channels are not well understood, though a gradient of increasing methane toward the margins of the Solimões River suggests inputs from the floodplains (Richey et al., 1988; Bartlett et al., 1990; Devol et al., 1994).
In a perennial first-order stream in the upper Xingu catchment, average fluxes based on floating chambers deployed monthly for a year were 108 ± 25 mg CH4 m−2 h−1 or 2,600 ± 600 mg CH4 m−2 d−1 (Neu et al., 2011). These very high fluxes could reflect input of groundwater high in CH4, and may have over-estimated emissions because stationary chambers in streams can increase turbulent exchange. No other fluxes from streams are available. For comparison, average fluxes of 18 mg CH4 m−2 d−1 summarized in Stanley et al. (2016) for tropical and subtropical streams are much lower. Clearly, many more measurements are needed.
Several studies have focused on open waters of lakes, though few included a complete year, adequately measured diffusive and ebullitive fluxes and rarely sampled diel variations. Over 2 years, nearly monthly measurements in an embayment and open water area of Lake Janauacá, located in várzea of the central basin, CH4 fluxes were made with floating chambers connected to an off-axis integrated cavity output spectrometer and inverted funnels to capture bubbles (Barbosa et al., 2020; Barbosa et al., 2021). Diffusive fluxes, with measurements over diel periods combined for both sites, averaged ∼27 mg CH4 m−2 d−1, and when combined with ebullition averaged 85 mg CH4 m−2 d−1; diel variations were observed often with higher values during the day.
In várzea Lake Camaleão on Marchantaria Island in the Solimões River, Wassmann et al. (1992) deployed floating chambers connected to an automated system assaying CH4 concentrations during a range of water levels, each with at least 3 days of sequential measurements, allowing bubble detection, and reported an average flux of 29 mg CH4 m−2 d−1. Diel variations were not observed. As part of regular sampling over 18 months in two lakes on the floodplain of the Negro River and six on the floodplain of the Solimões River (Forsberg et al., 2017), Devol et al. (1990) reported average fluxes of 44 mg CH4 m−2 d−1. Average diffusive fluxes during high water in várzea Lake Calado were 8.3 mg CH4 m−2 d−1 (Crill et al., 1988), during rising water averaged 6.6 mg CH4 m−2 d−1, increased up to 220 mg CH4 m−2 d−1 during passage of a rare cold front, while during falling water averaged 54 mg CH4 m−2 d−1; total flux averaged 163 mg CH4 m−2 d−1 during falling water (Engle and Melack 2000).
As part of a transect along the Solimões and Amazon rivers, Barbosa et al. (2016) reported an overall average of 59 mg CH4 m−2 d−1, ranging below detection to 298 mg CH4 m−2 d−1 for 10 lakes, including white waters and black waters, sampled during four hydrological phases. Sawakuchi et al.’s (2014) few measurements of total fluxes from the eastern várzea Lake Curuai averaged 18 mg CH4 m−2 d−1.
Methane fluxes within seasonally flooded forests occur from water surfaces, from the trunks of trees and from exposed soils during low water periods. In the flooded forests fringing Lake Janauacá, Barbosa et al. (2020) recorded an average of 110 mg CH4 m−2 d−1, based on floating chamber and bubble trap measurements. Diffusive CH4 fluxes within flooded forest measured by Wassmann et al. (1992) ranged from 1 to 12 mg CH4 m−2 d−1, and ebullition averaged 69 mg CH4 m−2 d−1. Fluxes at four water levels with floating chambers by Gauci et al. (2021) ranged as follows (expressed as mg CH4 m−2 d−1) for plots along the Solimões (43–55 except for a high water value of 450), Negro (12–19) and Tapajós (36–55) rivers. Based on measurements with floating chambers in inundated igapó forests along the Jaú River Rosenqvist et al. (2002) calculated a mean annual emission of methane 30 mg CH4 m−2 d−1.
Fluxes through trees in seasonally flooded forests can be high and quite variable when expressed per unit area of emitting surface. Based on sampling transects in twelve 0.4 ha forested plots along the Negro, Amazon, Madeira and Tapajós rivers during a period of rising water, Pangala et al. (2017) reported fluxes for mature and young trees per unit area of stem surface from 0.33 to 337 mg CH4 m−2 h−1 and 0.39–581 mg CH4 m−2 h−1, respectively. Similar measurements at four water levels in plots along the Solimões, Negro and Tapajós rivers by Gauci et al. (2021) ranged as follows (expressed as mg CH4 m−2 h−1 per unit area of stem surface): Solimões (0.013–78.9), Negro (0.005–50.5) and Tapajós (-0.004–69.7). Soil CH4 fluxes when the water table was below the surface in these plots were low and often negative, ranging from uptake of ∼1 mg CH4 m−2 d−1 to evasion of ∼1 mg CH4 m−2 d−1 (Gauci et al., 2021).
The Pantanal wetland can serve as a surrogate for the savanna wetlands in the Llanos de Moxos (Bolivia), which lack measurements of methane fluxes. Regular vertical profiles of methane and other gases obtained by aircraft from March 2017 to September 2019 in the Pantanal were combined with a planetary boundary layer budgeting technique to calculate a regional CH4 flux of 50 mg CH4 m−2 d−1 averaged over 1 year, assuming a planetary boundary layer-free troposphere exchange time of 3 days (Gloor et al., 2021). This flux integrates emissions from lakes, rivers and wetlands plus enteric fermentation by cattle and fires. Floating chambers deployed at five sites over a year in a Pantanal floodplain near the Miranda River yielded an average flux of 142 mg CH4 m−2 d−1 (Marani and Alvala 2007).
Mean emission of monthly measurements with floating chambers in numerous wetlands near Boa Vista (Roraima) of about 14 mg CH4 m−2 d−1 seem rather low compared to other similar Amazonian habitats (Jati 2014), perhaps because ebullition was not captured. Emissions from cultivated rice in Roraima are not available. In the mid-Negro basin, Belger et al. (2011) measured methane uptake on unflooded lands, evasion from flooded areas as diffusive and ebullitive fluxes with chambers and funnels, and as transport through rooted plants. Emission from wetland areas averaged 77 mg CH4 m−2 d−1.
As water levels rise on riverine floodplains, herbaceous plants form floating mats. Several studies have deployed floating chambers to measure methane fluxes from the water surface or, rarely, from plants on the surface. In mats of floating herbaceous plants, Barbosa et al. (2020) measured an average diffusive CH4 flux of 53 mg CH4 m−2 d−1 and estimated an average ebullition of 97 mg CH4 m−2 d−1, totaling 150 mg CH4 m−2 d−1. Diffusive fluxes within similar mats reported by Bartlett et al. (1988; 1990) were similar, averaging 42 and 44 mg CH4 m−2 d−1, respectively. Wassmann et al. (1992) reported diffusive fluxes from ∼2 to 28 mg CH4 m−2 d−1, average ebullition of 23 mg CH4 m−2 d−1 and did not detect plant-mediated transport via floating mats of Paspalum repens.
Fluxes in palm-dominated peatlands and nearby habitats in the western Amazon basin are variable and can be high. Eddy covariance measurements in a natural palm (Mauritia flexuosa) peatland near Iquitos (Peru) over a 2-year period averaged 22 g C m−2 y−1 (= ∼80 mg CH4 m−2 d−1) (Griffis et al., 2020). Teh et al. (2017) measured fluxes in the Pastaza–Marañón basin in forests, a Mauritia flexuosa-dominated palm swamp, and a mixed palm swamp during wet and dry seasons in four campaigns. Among all data, diffusive CH4 emissions averaged ∼48 ± 4 mg CH4 m−2 d−1; fluxes in the M. flexuosa palm swamp averaged ∼49 ± 5 mg CH4 m−2 d−1 (assuming their notation, CH4–C, means the mass of C in the CH4). As noted by the authors, their estimates of ebullition from short deployments of static chambers are probably not representative. In the same region, del Aguila-Pasquel (2017) sampled 8 times over 8 months spanning wet and dry seasons in a palm swamp and reported a mean flux of 73 ± 5.4 mg CH4 m−2 d−1 (n = 129). In peatlands in the Madre de Dios River basin (Peru) Winton et al. (2017) found that open canopy Cyperacea-dominated areas emitted 4.7 ± 0.9 mg CH4 m−2 h−1 (=113 ± 22 mg CH4 m−2 d−1), and Mauritia flexuosa palm-dominated areas emitted 14.0 ± 2.4 mg CH4 m−2 h−1 (= 336 ± 58 mg CH4 m−2 d−1) during a short period in 1 month. That CH4 can be emitted through M. flexuosa trunks suggests that emissions from palm-dominated peatlands based on soil flux chambers underestimate fluxes (van Haren et al., 2021). CH4 fluxes were significantly correlated to pneumatophore density in M. flexuosa stands (van Lent et al., 2019).
Several hydroelectric reservoirs have measurements available while most, usually smaller ones, do not. In Balbina reservoir, measurements of diffusive and ebullitive fluxes from multiple stations within the reservoir (average 63 mg CH4 m−2 d−1), degassing at the turbines and downstream were made over a year, when combined produce an annual CH4 emission of 97 Gg for the whole system, excluding methane oxidation in the river (Kemenes et al., 2007). Additional measurements at Samuel and Curua-Una reservoirs indicated the significance of degassing at the turbines and downstream (Kemenes et al., 2016). Based on measurements using drifting chambers during four periods in the first 2 years after filling of the Belo Monte hydroelectric system, Bertassoli et al. (2021) reported averages of 104 and 283 mg CH4 m−2 d−1, and whole systems annual totals of 20 and 50 Gg CH4. About half the flux was attributed to ebullition, and degassing in turbines was deemed minor.
Paranaíba et al. (2021) combined measurements of diffusive methane fluxes and calculations of gas exchange velocities with floating chambers at several sites and concentrations of methane sampled and analyzed on continuous transects through the Curuá-Una reservoir. During the rainy season with rising water levels, they reported average fluxes of 9.6 mg CH4 m−2 d−1 (range from 1.4 to 112) and during the drier season with falling water levels average fluxes of 14.4 mg CH4 m−2 d−1 (range from 1.3 to 69). As part of a study of organic carbon burial in Curuá-Una reservoir, Quandra et al. (2021) found methane dissolved in pore water to be above saturation in about one quarter of their measurements, indicating a potential for ebullition. Porewater concentrations were similar during periods with rising and falling water, varied spatially, but were not related to C:N ratios or organic carbon burial rates.
Emission from other Brazilian reservoirs based on overall average diffusive and ebullitive emissions from the surfaces of ten reservoirs within southern portions of the basin, as summarized in Deemer et al. (2016) is ∼80 mg CH4 m−2d−1; this value does not include degassing through turbines or below the dam. Estimating the emissions from the reservoirs in Bolivia, Ecuador and Peru is more difficult because no measurements exist and temperatures will be less at higher elevations and the watersheds differ from conditions in Brazil; a value of half that from Deemer et al. (2016) is suggested. The extent that the reservoir emissions represent net emissions, i.e., emissions additional to those associated with the undammed rivers, are uncertain, with estimates at Belo Monte (Bertassoli et al., 2021) and Balbina (Kemenes et al., 2011); upland forest soils, before being inundated, are likely to be sinks for methane.
The seasonally flooded ecotone, called the aquatic-terrestrial transition zone, is a varying mixture of bare soil and cover by herbaceous and woody plants exposed during periods of low water. Areas occupied by the aquatic-terrestrial transition zone are especially large in regions with shallow seasonal flooding, such as in savannas. Few measurements of methane flux or related processes are available for these periods in the Amazon (Pangala et al., 2017; Gauci et al., 2021); those of Smith et al. (2000) for the Orinoco floodplain are relevant. Microbial activity in response to desiccation (Conrad et al., 2014) and CH4 oxidation in exposed sediments on Amazon floodplains (Koschorreck 2000) have also been examined.
Sediments exposed as reservoir levels decline are analogous to the aquatic-terrestrial transition zone. Experimental measurements of methane emissions from sediment cores from a tropical Brazilian reservoir exposed to drying and rewetting indicated enhanced emissions from sediments with overlying water removed and when rewetted compared to sediments that had overlying water (Kosten et al., 2018). A comparative study for several types of aquatic systems including ones in tropical climates by Paranaíba et al. (2022) found emissions from portions of inland waters exposed to the atmosphere as water levels decline were consistently higher than emissions in nearby uphill soils. Statistical analyses revealed that methane emissions were negatively related to organic matter content and positively related to moisture of the sediments.
Riparian zones along streams often have soils with high water content but without standing water and occur throughout the Amazon basin. Topographic features are illustrated in Nobre et al. (2011). These environments are likely sources of methane emission. For example, soils in upland forests can release CH4, depending on soil moisture levels (Sihi et al., 2021).
Interpretation and modeling of methane fluxes requires understanding of the key processes; hence we discuss aspects of these processes with a focus on conditions relevant to the Amazon basin. Methane emissions reflect differences between CH4 production by methanogens and consumption by methanotrophs, and physical processes. Environmental factors that influence biological rates include water temperature, dissolved oxygen, trophic status and substrate availability. Wind, diel variations in thermal structure and physical processes such as convective and shear-driven mixing alter gas distributions and transfer velocities. CH4 can reach the atmosphere by three pathways: via diffusive fluxes at the air-water interface, via bubbles that form in the sediment, rise through the water column and are emitted to the atmosphere (ebullition), and through the vascular systems of herbaceous and woody aquatic plants. Ebullitive fluxes depend on bubble formation and hydrostatic pressure over the sediment, while diffusive fluxes depend on concentration gradients and turbulence. Here, we examine understanding of relevant biogeochemical and physical processes in Amazon aquatic environments.
The biogeochemical and microbial processes involved in the production and consumption of methane are known though uncertainties remain (e.g., Segers 1998; Borrel et al., 2011; Bridgham et al., 2013; Schlesinger and Bernhardt 2013). However, the quantitative importance of these processes varies among habitats and regions, and needs further study in aquatic environments within the Amazon basin. The availability to methanogens of the varied organic compounds present in Amazonian waters has not been characterized, though studies of the use of these substances for metabolism, in general, are available (e.g., Waichman 1996; Mayorga et al., 2005; Amaral et al., 2013; Ward et al., 2013; Vihermaa et al., 2014). Using samples from sediment cores obtained in an Amazon reservoir and two other tropical reservoirs in Brazil and incubated over about 2 years, Isidorova et al. (2019) found that rates of CH4 production had a strong statistical relation to sediment total nitrogen content and age of the sediment. Given the variations of sediment characteristics in Amazon floodplains and reservoirs (Hedges et al., 1986; Martinelli et al., 2003; Smith et al., 2003; Guyot et al., 2007; Moreira-Turcq et al., 2013; Cardoso et al., 2014; Sobrinho et al., 2016), further studies will likely reveal different methane production rates associated with these variations.
CH4 oxidation has been measured in a tropical reservoir (Guérin and Abril 2007) and inferred to occur in floodplain lakes (Crill et al., 1988; Engle and Melack 2000) or exposed sediments (Koschorreck 2000). Based on stable isotopic mass balances of CH4, Sawakuchi et al. (2016) estimated substantial rates of CH4 oxidation in large Amazonian rivers, and found that genetic markers for methane oxidizing bacteria were positively correlated with CH4 oxidation. Using incubations and measurements of δ13C-CH4 in a várzea lake, Barbosa et al. (2018) found that a large fraction of dissolved CH4 was oxidized with volumetric CH4 oxidation rates ranging from ∼1 to 175 mg CH4 m−3 d−1. Heavier values of δ13C-CH4 in surface waters when compared to bottom waters and sediment bubbles corroborate these high rates. They also found that CH4 oxidation had a positive relation with CH4 concentration and the presence of dissolved oxygen.
The microbial assembles in floodplain lakes (e.g., Melo et al., 2019) including methanogens and methanotrophs have received limited investigation in the Amazon (e.g., Finn et al., 2020; Bento et al., 2021; Gontijo et al., 2021). Conrad et al. (2010, 2011) and Ji et al. (2016) examined microbial communities and measured rates of methanogenesis in sediments by incubating sediment slurries from different floodplain lakes; CH4 production was found to be mainly hydrogenotrophic based on isotopic fractionation. However, the congruence of laboratory incubations of sediment slurries with CH4 production in intact sediments is unclear. More study is needed relating microbial activity to environmental conditions, such as the examination of responses to desiccation by Conrad et al. (2014). Anaerobic oxidation of methane and microbial methane production in oxygenated water also need attention, as indicated by studies elsewhere (e.g., Caldwell et al., 2008; Grossart et al., 2011; Roland et al., 2016). For example, Gabriel et al. (2020) used slurries of flooded Amazon forest soils to demonstrate the potential for anaerobic methane oxidation by Fe(III) reduction.
In the warm waters of the Amazon basin, high latent heat fluxes lead to convective mixing while diurnal heating under strong insolation leads to periods of stable stratification (e.g., Augusto-Silva et al., 2019). Exchange of methane between surficial water and overlying atmosphere depends on the concentration gradient between air and water and on physical processes at the interface, usually parameterized as a gas transfer velocity (k). Gas transfer velocities are influenced by atmospheric stability and, in water, are altered by currents, wind and convection, as well as rain, temperature and organic surficial films. Melack (2016) summarized estimates of k available for rivers and lakes in the Amazon basin. Results reported by Ulseth et al. (2019) indicate quite large k values can occur in high-energy montane streams due to bubble entrainment. Recent studies by MacIntyre et al. (2019) and MacIntyre et al. (2021) have used near-surface measurements of dissipation rates of turbulent kinetic energy and hydrodynamic theory to calculate k under low wind conditions in open water and within flooded forests.
Though gas transfer velocity can be parameterized based on wind speed (Wanninkhof 1992; Cole and Caraco 1998), the dependence of k on the dissipation rate of turbulent kinetic energy (ϵ) has theoretical and empirical evidence. The surface renewal model, k = c1 (ϵν)1/4) Sc−n has an explicit dependence on dissipation rates, where dissipation rates have units of m2 s−3, ν is kinematic viscosity (m s−2), Sc is the Schmidt number, n is −1/2 or −2/3, and c1 is an empirical coefficient. Dissipation rates depend on the shear just below the air-water interface and are augmented if cooling or heating are appreciable relative to shear (MacIntyre et al., 2021). This model has been applied to Amazon flooded forests (MacIntyre et al., 2019) and reservoirs (MacIntyre et al., 2021) and confirmed with indirect estimates of k using results of experiments with floating chambers (Amaral et al., 2020).
Stratification within tropical lakes and reservoirs can be appreciable as a result of intense heating when winds are light (e.g., Augusto-Silva et al., 2019). In that case, near-surface values of dissipation rate can be higher than observed under cooling. With stratification retarding the downward mixing of heat, much of the turbulence produced by wind is dissipated. Values of k computed using the surface renewal model during heating averaged 10 cm h−1 but reached 18 cm h−1 for winds up to 4 m s−1, were independent of wind speed, and increased with heating (MacIntyre et al., 2021). Hence, the fluxes estimated from wind-based models for many of the lakes in the Amazon basin are likely underestimated.
CH4 ebullition, a major mechanism for evasion to the atmosphere, is regulated by the production and accumulation of CH4 in sediments and processes leading to the release of bubbles from the sediments. Release of bubbles can be influenced by variations in hydrostatic pressure caused by a drop in atmospheric pressure or drop in water level. Other factors include currents, waves, shear-stress at the sediment-water interface and possibly sediment disturbances by benthic organisms (Barbosa et al., 2021).
Vertical and horizontal water movements connect littoral, pelagic and benthic regions of lakes and wetlands (MacIntyre and Melack 1995) and are likely to influence CH4 concentrations and fluxes. One-dimensional (e.g., DYRESM, Yeates and Imberger 2003) and three-dimensional (e.g., AEM3D, Hodges and Dallimore 2019) hydrodynamic models include processes induced by surface heat fluxes, wind, inflows and outflows. One-dimensional models characterize a lake as a single water column in the vertical and are computationally efficient permitting long-term and regional applications. Three-dimensional models divide a lake into grids in three directions, resolve spatial variability of thermal structure, internal waves, horizontal exchanges and calculate dissipation rates of turbulent kinetic energy, a key term in the surface renewal model of gas exchange. An example of an application of a three-dimensional model to a floodplain lake illustrates spatial differences in diel cycles of stratification and mixing (Figures 4A–D), and circulations driven by wind-induced basin-scale internal waves when the near-surface water is stratified (Figures 4E,F). Three-dimensional hydrodynamic modeling has demonstrated that diel differences in water temperature between floating plant mats and open water as well as basin-scale motions can cause lateral exchanges linking vegetated habitats to open water (Amaral et al., 2021). Higher CH4 concentrations in herbaceous plant mats than in open water suggest that vegetated habitats can be sources of CH4 to other regions (Bartlett et al., 1988; Barbosa et al., 2020).
FIGURE 4. Three-dimensional modeling of thermal structure in Lake Janauacá. (A) The net heat flux and wind speed at site one on map in panel d; (B,C) AEM3D simulated temperatures of water column at sites 1,2 over 5 days; (D) bathymetry of Lake Janauacá, white dots indicate sites 1,2 shown in panels b and c, and white dashed line marks orientation of transect C1; (E) AEM3D simulated near-surface temperatures at the time marked by the vertical blue dashed line in panel a (25 August 2016, 14:29:30 h); (F) AEM3D simulated temperatures and horizontal velocities along transect C1 at the time marked by the vertical blue dashed line in panel a (25 August 2016, 14:29:30 h). Numbered black lines in panels b, c, e and f are the isotherms at 1°C intervals. Application of AEM3D to Lake Janauacá is described in Amaral et al. (2021).
In a summary of results from the Wetland and Wetland CH4 Inter-comparison of Models Project (WETCHIMP), Melton et al. (2013) stated that the models disagreed in their simulations of wetland areal extent and methane emissions, in both space and time, and had parameter and structural uncertainty, and noted that lakes and rivers were not included. Moreover, mechanistic models of methane production and evasion appropriate for tropical floodplains are not available (Riley et al., 2011), though relevant conceptual models have been proposed (Cao et al., 1996; Potter 1997; Potter et al., 2014). While several models have potentially useful components or formulations (e.g., Walter and Heimann 2000; Tang et al., 2010; Bloom et al., 2012; Wania et al., 2013; Ringeval et al., 2014; Tan et al., 2015; Lu et al., 2016), no spatially explicit model exists that incorporates the inundation dynamics, ecological characteristics and limnological conditions of tropical floodplains and wetlands. Of special importance is inclusion of plant functional groups common in these habitats combined with appropriate algorithms to estimate their productivity, as these plants supply most of the organic carbon subsequently released as methane (Melack and Engle 2009; Melack et al., 2009). Algorithms are required that simulate stratification and mixing of the water column with concomitant influence on the extent of anoxia and on air-water gas exchange.
Current regional biogeochemical models of methane emissions from wetlands calculate grid-averaged methane fluxes based on soil temperature and carbon availability or heterotrophic respiration. Examples of these models include the Joint United Kingdom Land Environment Simulator (JULES) (Clark et al., 2011; McNorton et al., 2016), the Lund-Potsdam-Jena model (LPJ-WSL; Sitch et al., 2003; Zhang et al., 2016), a CH4 biogeochemistry model based on the Integrated Biosphere Simulator (TRIPLEX-GHG; Zhu et al., 2014), the LPX-Bern model (Ringeval et al., 2014), a revision of TEM-MDM (Liu et al., 2020) and WetCHARTs (Bloom et al., 2017), JPL-WHyMe (Wania et al., 2010), the Dynamic Land Ecosystem Model (DLEM, Zhang et al., 2017), and CLM4Me (Riley et al., 2011; Meng et al., 2012). A methane model for Amazon peatlands is under development (Yuan et al., 2020). One problem with these models is their use of molecular diffusion through soil layers that is not appropriate for the turbulence-based gas transfer needed for conditions with surface inundation. Inundation is usually simulated as overland hillslope flows and an approximated terrain model, and does not represent the large seasonal inundation variations and hydrologic fluxes present in the Amazon basin. Climatic inputs are typically obtained from reanalysis products with insufficient temporal resolutions to force diel processes. The plant functional groups included do not represent the aquatic plants common in the Amazon basin. Furthermore, these models do not explicitly include biogeochemical processes, such as methanogenesis and methane oxidation, or physical processes, such as mixing through the water, lateral exchanges, ebullition, or air-water exchange via turbulent mixing. Also, they do not simulate dissolved oxygen concentrations and thus the impact of dissolved oxygen on the methane oxidation or prescribe bulk dissolved oxygen concentrations to the grids (Wania et al., 2010; Riley et al., 2011).
Results from an atmospheric transport model and ATTO mixing ratios (Botia et al., 2020) were compared to six versions of the extended ensemble available in WetCHARTs v1.3.1 (Bloom et al., 2017) to account for variability in wetland extent and the temperature-dependent CH4 respiration fraction (Q10) (Figure 5). All WetCHARTs’ simulations used precipitation from ERA5 (C3S, 2017) to drive the temporal variability of methane emissions and the CARDAMOM model for heterotrophic respiration, but the wetland spatial extent was varied. The simulations ending in three used the Global Lakes and Water Database (Lehner and Döll 2004) and those ending in four used the sum of all wetland and freshwater land cover types in GLOBCOVER (Bontemps et al., 2011). Each pair of simulations had a different temperature dependence of the CH4 respiration fraction (Q10), according to the second digit of each simulation (Q10 = 1,2,3). The variability in the WetCHARTs simulations indicates that when using a different wetland extent but the same temperature dependence of the CH4 respiration fraction (Q10), the effect on the integrated CH4 signal can be about 10 ppb (i.e., lines 913 and 914). This effect seems to be larger at lower Q10 values. When using a different Q10 and the same wetland extent (e.g., line 914 with 924 or 934), the differences are larger when comparing the Q10 = 1 with the Q10 = 2 simulations than between the Q10 = 2 and Q10 = 3 simulations. Furthermore, these comparisons suggest that WetCHARTs fluxes for the ATTO footprint are too high, and that the seasonality of WetCHART-derived mixing ratios at ATTO are 1 month out of phase when compared to the ATTO observations. When accounting for transport errors (not shown here), the simulated wetland signal at ATTO is still higher than the observations (Botia et al., 2020).
FIGURE 5. Seasonal cycle of the regional signal (as measurements - background concentration) for simulated and observed mixing ratios of CH4 at ATTO, expressed as parts per billion (ppb). The observed mixing ratios at ATTO (measurement height 79 m) are shown as a black line and the error bars represents ±1 sigma (standard deviation). This methodology was applied for CO2 at ATTO by Botía et al. (2021). The background concentrations are from the inversion available via the Copernicus Atmosphere Monitoring Service (CAMS) (Segers and Houweling 2020). For the simulated and observed mixing ratios, only daytime values (13:00–17:00 h local time) were used to ensure well-mixed conditions in the planetary boundary layer. WetCHARTs v1.3.1 is used for the simulated contribution of wetlands to the integrated CH4 signal at ATTO. The set of WetCHARTs ensemble members show different temperature dependence factors for the CH4 respiration fraction (Q10) and different wetland extents.
Potter et al. (2014) built a model for Amazon floodplain lakes based on the supply of organic carbon, as a key factor determining methane production. The LAKE model (Stepanenko et al., 2016) includes most major processes operative in lakes, but the methane module has only been tested on a small boreal lake. Zimmermann et al. (2021) used measurements and a one-dimensional physical model of a small temperate lake to examine how seasonal or more frequent thermocline deepening influenced methane emission and consumption by methanotrophs. Though conceptually relevant, this model would require revision because diel cycles of stratification and mixing are common in shallow Amazon lakes.
The Arctic Lake Biogeochemical Model (ALBM) is a one-dimensional process-based, biogeochemical model that simulates the thermal and methane dynamics of lakes (Tan et al., 2015). The model has been applied to arctic and boreal lakes on seasonal time scales (Tan et al., 2015; Guo et al., 2020), but the thermal module and several other aspects need revisions for conditions in shallow, warm waters. In section 6.2 we discuss alternative models of the relevant physical processes. By combining sensitivity analyses and calibration and validation with appropriate data, the models have been shown to perform reasonably well for specific lakes. However, to apply these models on a regional scale is more difficult as success depends on the calibration and validation sites being representative of the region, and on the availability of data for lakes in the region. An application of ALBM to the Amazon basin produced reasonable results for open water of lakes that further modifications are likely to improve (Figure 6). The parameters used for the Amazon basin were calibrated with data from two well-studied floodplain lakes (Janauacá and Calado) with relevant references cited above.
FIGURE 6. Mean annual methane fluxes for only lakes, expressed as mmoles of CH4 m−2 y−1, weighted averaged by lake area within 0.5° × 0.5° grid cells for 2004 through 2006 as modeled by ALBM (Tan et al., 2015; Guo et al., 2020). Both the Amazon and Tocantins basins are shown.
Several biogeochemical and physical processes need to be incorporated or improved in mechanistic methane models for floodplain lakes. New formulations of gas transfer velocity and inclusion of lateral exchanges and diel stratification and mixing, as described in section 6, are recommended. Developing parameterizations for fluxes through trees and herbaceous plants, oxic methane production, anaerobic methane oxidation and supply of carbon by autotrophic growth and hydrological inputs are challenging but important. To improve modeling of ebullition, inclusion of changes in hydrostatic pressure and from disturbances of the sediments may help.
Statistical models offer another approach for estimating lake and reservoir emissions, albeit with limitations related to data availability
DelSontro et al. (2016) analyzed the relationship between emission rates and the predictor variables of lake size, chlorophyll a, total phosphorus and total nitrogen using simple and multiple linear regression for lake and reservoirs distributed around the world. Though tropical reservoirs were included, Amazon floodplain lakes are not represented in this analysis. Among the variables evaluated only chlorophyll a had a positive effect on total methane emission. For comparison, a statistical model applied to Lake Janauacá found variables related to CH4 production (temperature, dissolved organic carbon) and consumption (dissolved nitrogen, oxygenated water column), as important to dissolved CH4 concentrations (Barbosa et al., 2020).
Using eddy covariance data from wetland sites largely in the north temperate zone, Knox et al. (2021) applied several statistical techniques to examine the importance of a variety of physical and biological predictors of the timing and magnitude of CH4 fluxes. While quite informative with regard to the wetlands included, the only site in tropical South America is in the Pantanal, a seasonal savanna wetland. Though the Pantanal shares similarities to the Moxos wetlands, the majority of floodplain and other wetlands in the Amazon basin are not represented in the analyses.
While it is well known that all models have biases from limitations and assumptions within the models and as a result of the data used in calibration and validation, given the logistic and scientific challenges with measurements in the vast and complex Amazon basin, models of all types can surely contribute to understanding and projections of methane emissions.
Estimates of methane fluxes at different spatial scales illustrate varied approaches and limitations. Early estimates of regional methane fluxes made for the mainstem Solimões-Amazon floodplain and the whole basin were limited by lack of data on areas of inundation and aquatic habitats and few measurements of fluxes (e.g., Bartlett et al., 1988; Devol et al., 1990). The first estimates that incorporated remote sensing of inundation and habitat areas were done by Melack et al. (2004) for the mainstem Solimões-Amazon floodplain and a 1.77 million km2 area in the central basin (Hess et al., 2003). They combined habitat-specific methane fluxes with seasonal changes in the area of aquatic habitats, derived from active and passive microwave remote sensing, and applied Monte Carlo error propagation to establish uncertainties. By assuming similar fluxes by habitat and adding approximations for particular regions (e.g., Moxos), an extrapolation to whole lowland basin was made. For the central basin quadrant, annual emission was estimated as 9 ± 1.7 Tg CH4, and if extrapolated to the basin below 500 m asl, resulted in approximately 29 Tg CH4 y−1. The largest omission from these estimates is evasion from seasonally flooded forests. The measurements of emissions for trees, extrapolated to the Amazon basin, by Pangala et al. (2017; 15.1 ± 1.8 to 21.2 ± 2.5 Tg CH4 y−1) and Gauci et al. (2021; 12.7 to 21.1 Tg CH4 y−1 for inundated trees plus 2.2 to 3.6 Tg CH4 y−1 for riparian zones with water tables below the surface) substantially increase regional fluxes. However, considerable uncertainty in these estimates stems from the lack of adequate data on the abundance and emitting surface areas of the trees and the high variability in fluxes per unit area of trees.
As part of the Science Panel for the Amazon (SPA) report, released at COP26 (Gatti L. et al., 2021; Mahli et al., 2021), J. Melack, L. Basso and S. Pangala provided bottom-up estimates of methane emission from aquatic environments in the combined hydrological Amazon and Tocantins basins, and for the Amazon forest biome. A total area of aquatic habitats for these two basins of 970,500 km2 was used to generate their bottom-up estimate of approximately 51 Tg CH4 y−1. However, this estimate does not include seasonal and interannual variations or a rigorous uncertainty analysis. A second estimate of methane emissions was done for the Amazon biogeographic province that encompasses tropical forests in much of Amapá, French Guiana, Guyana, Suriname, and southern Venezuela and eastern Colombia that drains into the Orinoco River (Albert et al., 2021). This estimate had added uncertainty because of the lack of data for most of the northeastern areas outside of the hydrological Amazon basin.
Improvements needed in bottom-up estimates are inclusion of seasonal variations in flooding and aquatic habitats, as noted in sections 2,3, and more measurements of all fluxes, summarized in section 4. An example of doing so is provided by Barbosa et al. (2021) for a floodplain lake with fringing inundated forests and herbaceous plants. They combined seasonal variations in ebullitive, diffusive and tree-mediated fluxes with variations in water depths and areal coverage of aquatic habitats, i.e., weighting fluxes by seasonal variations in habitat areas and habitat-specific fluxes. This approach was especially important for ebullition for which the fraction of total emission from water surfaces varied from 1% (rising and high water) to 89% (low water) in open water of the lake, and from 73% (rising water) to 93% (falling water) in flooded forests.
An example of the challenges and compromises in regionalization is offered for a reach of the mainstem Solimões-Amazon rivers and associated floodplains (Table 1). The values in Table 1 provide a sense of the relative importance of different habitats as sources of methane to the atmosphere based on areal fluxes and habitat areas for low and high water conditions. However, the fluxes should be viewed with caution because of the uncertainties associated with the data and calculations. As summarized in section 5, several studies provide measurements of methane fluxes from water surfaces in river channels and floodplain habitats. Those selected here were judged representative and offering the most complete data. To fully use data from all studies is not possible because actual datasets are not available in most publications. The fluxes from trees are especially difficult to extrapolate because of the large variance in per tree fluxes and the very limited measurements of the surface areas of trees. Fluxes from floodplain soils during low water periods are not included.
TABLE 1. Methane emission for reach of the mainstem Solimões-Amazon rivers and floodplains from 73.57o W, 4.5o S (confluence of Marañón and Ucayali rivers) to 51.4o W, 0.46o S (Gurupá Island). Areas of aquatic habitats from Melack and Hess (2010) and of rivers from Allen and Pavelsky (2018). Mg = 106 g.
In an analysis for the reach of the mainstem Solimões-Amazon floodplain from 54o to 70o W, annual fluxes of 1.7 ± 0.4 Tg CH4 were calculated with 67% of the uncertainty attributed to habitat-specific fluxes and 33% owed to uncertainties in areal estimates of inundation and vegetative cover (Melack et al., 2004). To do so, the methane emission rate per unit area for the mainstem Solimões-Amazon floodplain, representing a mixture of habitats, and using a mean annual flooded area of 42,700 km2 (determined as monthly means from Sippel et al. (1998) summed over 12 months and divided by 12) was estimated as ∼40 Mg CH4 km−2 y−1.
Several aircraft campaigns provide methane flux estimates for different regions and periods. Beck et al. (2012) estimated fluxes for the lowland Amazon during November as 36 ± 12 mg CH4 m−2 d−1 and during May as 43 ± 19 mg CH4 m−2 d−1. Miller et al. (2007) collected vertical profiles of methane over 4 years at sites near Santarém and Manaus and calculated average emissions of ∼27 mg CH4 m−2 d−1. Wilson et al. (2016) applied the TOMCAT model using aircraft vertical profiles and estimated methane emissions of 36.5 to 41.1 Tg CH4 y−1in 2010 and 31.6 to 38.8 Tg CH4 y−1 in 2011 for an area of 5.8 × 106 km−2; non-combustion emissions represented 92–98% of total emissions. Pangala et al. (2017) estimated emissions of 42.7 ± 5.6 Tg CH4 y−1 for an area of 6.77 × 106 km2 based on vertical profiles from 2010 to 2013; 10% of emission was attributed to biomass burning.
The most comprehensive record is provided by Basso et al. (2021), who used lower-troposphere vertical profiles of methane concentration from four sites in the Brazilian Amazon basin between 2010 and 2018 and a column budget technique to calculate emissions of 46.2 ± 10.3 Tg CH4 y−1 with no temporal trend. This finding of no temporal trend during a period with exceptionally high and low river stages and varying inundation areas suggests further examination of how methane fluxes could have varied through these years.
Basso et al. (2021) used the methodology of Gatti L. V. et al. (2021) to estimate fluxes for three subregions and combined these as a weighted flux scaled to an area of 7.25 million km2, a region that includes parts of Venezuela, and all of Suriname, French Guyana and Guiana plus coastal watersheds in Brazil and upper Tocantins basin. The large region to the west of their sampling locations near Tefé and Tabatinga was not sampled and has some environments not represented by the regions to the east, such as the Peruvian peatlands and Andean highlands. Based on concurrent measurements of carbon monoxide, 17% of the sources were attributed biomass burning and 83% mainly to wetlands. Causes for seasonality in wetland fluxes and for the larger signals in the northeast are not fully understood, indicating that more studies are needed.
Based on SCIAMACHY data, Bergamaschi et al. (2009) calculated total Amazon emissions of 47.5 to 53.0 Tg CH4 y−1 in 2004 for an area of 8.6 × 106 km2. Based on an inversion model, Fraser et al. (2014) estimated an emission of 59.0 ± 3.1 Tg CH4 y−1 from tropical South America (approximately ∼9.7 × 106 km2) in 2010. Tunnicliffe et al. (2020) using inverse modelling estimates and GOSAT measurements reported mean emissions for the Brazilian Amazon wetlands (and not clearly defined) lower than other estimates (9.2 ± 1.8 Tg CH4 y−1). Wilson et al. (2021) using GOSAT data and an inverse model estimated total emissions from natural, agricultural and biomass burning sources of 38.2 ± 5.3 (between 2010 and 2013) and 45.6 ± 5.2 Tg CH4 y−1 (between 2014 and 2017) for the Amazon basin (approximately ∼6.0 × 106 km2). Given the different regions represented and the mixture of sources, it is difficult to compare these estimates with each other or with the aircraft or bottom-up values.
Methane emissions for the Amazon basin, extracted from the WetCharts ensemble of wetland models (Bloom et al. (2017) at monthly resolution for 2009 and 2010 by Basso et al. (2021), are 39.4 ± 10.3 Tg CH4 y−1, and results are similar to emissions derived from aircraft samples except for the northeastern portion of the basin. Gedney et al. (2019) provided estimates of methane flux for the Amazon basin using the JULES model that varied from ∼24 to ∼58 Tg CH4 y−1, a rather wide range that stems from problems with inundation estimates and parameterization of methane fluxes. As part of an application of ALBM to global lakes (Guo et al., 2020), an estimate for only lakes in the Amazon and Tocantins basins, based a static lake area from Messager et al. (2016) and calibration with data from lakes Janauacá and Calado, yielded estimates of ∼0.7 Tg CH4 y−1 and ∼0.1 Tg CH4 y−1, respectively. This estimate is similar to the estimate of ∼0.7 Tg CH4 y−1 in Mahli et al. (2021) for the same regions based on extrapolation of bottom-up measurements of fluxes and similar lakes areas.
Given that an important source of uncertainty in the global methane budget is attributed to emissions from wetlands and other inland waters (Saunois et al., 2020), further improvements in measurements and the understanding of large sources of methane emissions from aquatic ecosystems in the Amazon basin and similar systems elsewhere are clearly a priority. Our review has identified several key ways to do so: 1) Application of remote sensing techniques to better characterize the spatial extent and temporal variations in inundation and aquatic habitats. 2) Increased sampling frequency and duration of methane fluxes and related environmental conditions in all aquatic systems using a combination of approaches including ground-based, aircraft and satellite measurements. 3) Conducting experimental studies to improve understanding of biogeochemical and physical processes. In particular, more studies of the complex roles of microbial assembles and their response to environmental conditions, and of methane emissions in relation to qualities of organic matter and biogeochemical conditions in sediments would improve predictive capabilities. 4) Further development of models appropriate for hydrological and ecological conditions throughout the Amazon basin.
Current hydrological models provide estimates of variations in inundation, and remote sensing products include inundated areas, though the longest time-series underestimate areas in some habitats and high-resolution products are temporally sparse. Mapping inundated lands during low water season poses special challenges in the Amazon basin (Fleischmann et al., 2021). Biogeochemical wetland models do not incorporate key processes and need to use better inundation estimates. For example, Fleischmann et al. (2021) judged that the WAD2M product used in several global methane models does not represent well the inundation dynamics of various wetland complexes in the Amazon basin. Large uncertainties stem from the paucity of measurements of methane fluxes throughout the Amazon basin. Particularly large gaps exist for the Llanos de Moxos, peatlands throughout the Amazon basin, coastal systems, interfluvial wetlands and habitats above 500 m. Streams and medium-sized rivers have very few data. Measurements during low water periods in exposed areas with herbaceous plants and floodable forests are rare. Measurements above and below the dams are available for few hydroelectric projects. Diel, seasonal and interannual variations are very seldom documented. To evaluate potential impacts of increased temperatures and atmospheric CO2 concentrations on different plants and other organisms would benefit from a combination of experiments and modeling. With the increased availability of in situ sensors and automatic recording systems, improved temporal coverage is possible, though logistic challenges remain. The results from an observing system, as suggested by Bloom et al. (2016) for the Amazon basin, would provide valuable data.
As illustrated for specific lakes or subregions, spatially and temporally representative measurements combined with remote sensing-based inundation and habitat estimates can produce seasonally-weighted fluxes (e.g., Rosenqvist et al., 2002; Barbosa et al., 2021). Mechanistically correct and properly calibrated models provide a complementary approach. Models of autotrophic productivity as a source of the organic carbon that fuels methanogenesis and influences other conditions need to use functional traits appropriate to the ecology of the Amazon. Expanded use of hydrological models linked to carbon supply from uplands to aquatic systems (e.g., Lauerwald et al., 2017; Hastie et al., 2019) is also recommended.
Recent results can be used to develop robust regional estimates of methane fluxes from aquatic environments in the Amazon basin, and these approaches can be applied elsewhere. Multiyear measurements of methane concentrations obtained from aircraft (e.g., Basso et al., 2021) and towers, such as the ATTO facility (e.g., Botía et al., 2020), when combined with atmospheric transport models, provide spatially integrated methane concentrations in the regions of influence. However, attributing sources of the methane requires examination of the inundated area and methane fluxes represented by the gridded regions of influence. A critical aspect is applying uncertainty analyses that incorporate natural variability and evaluate systematic biases of the measurements.
Indeed, incorporating all these approaches to an area as vast and diverse as the Amazon basin is a daunting challenge. Though serendipity and national and international initiatives will continue to influence research opportunities, we suggest a few examples. Promising planned remote sensing missions are SWOT and NISAR (mentioned in section 2). The SWOT mission will simultaneously measure surface water elevation, slope and extent and provide the basis for calculations of river discharge and monitoring of lake water levels. NISAR will complement other SAR missions by providing global 12-days coverage of L-band radar, which penetrates forest canopies to detect inundation under seasonally flooded forests. Cost-effective sampling designs depend on institutional infrastructure, interested and trained personnel, funding and international coordination. Hence, many studies were concentrated in the vicinity of research institutes or universities or were conducted as surveys along major rivers. Less accessible or remote regions or habitats, such as the Llanos de Moxos, other interfluvial wetlands and Andean slopes, are likely to be significant sources of methane and will require extra effort and expense. In contrast, streams and medium-sized rivers are surprisingly under-sampled given their proximity to established research centers. Applications of advanced methods in microbial ecology and experimental manipulations, including characterization of methanogenic and methanotrophic assembles and their temporal variability in abundance and activity, would strengthen modeling efforts and predictive understanding. Creative individuals will continue to develop new ideas and methods and venture into unexplored areas.
The ideas represented in our review evolved from discussions among all co-authors over several years. JM developed and wrote the manuscript and all co-authors contributed with results and revisions.
National Aeronautics and Space Administration (NASA grant NNX17AK49G) and the US National Science Foundation (NSF DEB grant 1753856) to JM and SM supported data analysis and writing by JM, SM, and WZ. Sao Paulo Research Foundation (FAPESP grants 2018/14006-4 and 2019/23654-2) supported data analysis by LB. Bundesministerium für Bildung und Forschung (grants 01LB1001A and 01LK1602A) and the International Max Planck Research School for Global Biogeochemical Cycles (IMPRS-gBGC) supported data analysis and writing by SB.
SB acknowledges the ATTO team running the site, and Jost Lavric and David Walter for data handling and processing, and thanks Anthony A. Bloom for providing version 1.3.1 of WetCHARTs for the STILT simulations.
The authors declare that the research was conducted in the absence of any commercial or financial relationships that could be construed as a potential conflict of interest.
All claims expressed in this article are solely those of the authors and do not necessarily represent those of their affiliated organizations, or those of the publisher, the editors and the reviewers. Any product that may be evaluated in this article, or claim that may be made by its manufacturer, is not guaranteed or endorsed by the publisher.
Abril, G., Guérin, F., Richard, S., Delmas, R., Galy-Lacaux, C., Gosse, P., et al. (2005). Carbon Dioxide and Methane Emissions and the Carbon Budget of a 10-year Old Tropical Reservoir (Petit Saut, French Guiana). Glob. Biogeochem. Cycles 19, 1–16. doi:10.1029/2005gb002457
Albert, J., Hoorn, C., Malhi, Y., Phillips, O., Encalada, A. C., Steege, H., et al. (2021). “The Multiple Viewpoints for the Amazon: Geographic Limits and Meanings,” in Amazon Assessment Report. Editors C. Nobre, A. Encalada, E. Anderson, F. H. Roca Alcazar, and M. Bustamante (New York, USA: United Nations Sustainable Development Solutions Network). www.theamazonwewant.org/spa-reports.
Alin, S. R., Rasera, M. d. F. F. L., Salimon, C. I., Richey, J. E., Holtgrieve, G. W., Krusche, A. V., et al. (2011). Physical Controls on Carbon Dioxide Transfer Velocity and Flux in Low-Gradient River Systems and Implications for Regional Carbon Budgets. J. Geophys. Res. 116, G01009. doi:10.1029/2010jg001398
Allen, G. H., and Pavelsky, T. M. (2018). Global Extent of Rivers and Streams. Science 361, 585–588. doi:10.1126/science.aat0636
Almeida, R. M., Shi, Q., Gomes-Selman, J. M., Angarita, H., Barros, N., Forsberg, B. R., et al. (2019). Reducing the Greenhouse Gas Footprint of Amazon Hydropower with Optimal Dam Planning. Nat. Comm. doi:10.1038/s41467-019-12179-5
Alsdorf, D., Han, S.-C., Bates, P., and Melack, J. (2010). Seasonal Water Storage on the Amazon Floodplain Measured from Satellites. Remote Sens. Environ. 114, 2448–2456. doi:10.1016/j.rse.2010.05.020
Amaral, J. H. F., Melack, J. M., Barbosa, P. M., Borges, A. V., Kasper, D., Cortés, A. C., et al. (2021). Inundation, Hydrodynamics and Vegetation Influence Carbon Dioxide Concentrations in Amazon Floodplain Lakes. Ecosystems. doi:10.1007/s10021-021-00692-y
Amaral, J. H. F., Melack, J. M., Barbosa, P. M., MacIntyre, S., Kasper, D., Cortes, A. C., et al. (2020). Carbon Dioxide Fluxes to the Atmosphere from Waters within Flooded Forests in the Amazon Basin. J. Geophys. Res. Biogeosci. 125, e2019JG005293. doi:10.1029/2019jg005293
Amaral, J., Suhett, A., Melo, S., and Farjalla, V. (2013). Seasonal Variation and Interaction of Photodegradation and Microbial Metabolism of DOC in Black Water Amazonian Ecosystems. Aquat. Microb. Ecol. 70, 157–168. doi:10.3354/ame01651
Apers, S., De Lannoy, G. J. M., Baird, A. J., Cobb, A. R., Dargie, G. C., del Aguila Pasquel, J., et al. (2022). Tropical Peatland Hydrology Simulated with a Global Land Surface Model. J. Adv. Model. Earth Sys 14, e2021MS002784. doi:10.1029/2021MS002784
Arnesen, A. S., Silva, T. S. F., Hess, L. L., Novo, E. M. L. M., Rudorff, C. M., Chapman, B. D., et al. (2013). Monitoring Flood Extent in the Lower Amazon River Floodplain Using ALOS/PALSAR ScanSAR Images. Remote Sens. Environ. 130, 51–61. doi:10.1016/j.rse.2012.10.035
Augusto-Silva, P. B., MacIntyre, S., de Moraes Rudorff, C., Cortés, A., and Melack, J. M. (2019). Stratification and Mixing in Large Floodplain Lakes along the Lower Amazon River. J. Gt. Lakes. Res. 45, 61–72. doi:10.1016/j.jglr.2018.11.001
Barbosa, P. M., Farjalla, V. F., Melack, J. M., Amaral, J. H. F., da Silva, J. S., and Forsberg, B. R. (2018). High Rates of Methane Oxidation in an Amazon Floodplain Lake. Biogeochemistry 137, 351–365. doi:10.1007/s10533-018-0425-2
Barbosa, P. M., Melack, J. M., Amaral, J. H. F., Linkhorst, A., and Forsberg, B. R. (2021). Large Seasonal and Habitat Differences in Methane Ebullition on the Amazon Floodplain. J. Geophys. Res. Biogeosci. 126, e2020JG005911. doi:10.1029/2020JG005911
Barbosa, P. M., Melack, J. M., Amaral, J. H. F., MacIntyre, S., Kasper, D., Cortés, A., et al. (2020). Dissolved Methane Concentrations and Fluxes to the Atmosphere from a Tropical Floodplain Lake. Biogeochemistry 148, 129–151. doi:10.1007/s10533-020-00650-1
Barbosa, P. M., Melack, J. M., Farjalla, V. F., Amaral, J. H. F., Scofield, V., and Forsberg, B. R. (2016). Diffusive Methane Fluxes from Negro, Solimões and Madeira Rivers and Fringing Lakes in the Amazon Basin. Limnol. Oceanogr. 61, S221–S237. doi:10.1002/lno.10358
Barichivich, J., Gloor, E., Peylin, P., Brienen, R. J. W., Schöngart, J., Espinoza, J. C., et al. (2018). Recent Intensification of Amazon Flooding Extremes Driven by Strengthened Walker Circulation. Sci. Adv. 4, eaat8785. doi:10.1126/sciadv.aat8785
Barros, N., Cole, J. J., Tranvik, L. J., Prairie, Y. T., Bastviken, D., Huszar, V. L. M., et al. (2011). Carbon Emission from Hydroelectric Reservoirs Linked to Reservoir Age and Latitude. Nat. Geosci. 4, 593–596. doi:10.1038/ngeo1211
Bartlett, K. B., Crill, P. M., Sebacher, D. I., Harriss, R. C., Wilson, J. O., and Melack, J. M. (1988). Methane Flux from the Central Amazonian Floodplain. J. Geophys. Res. 93, 1574–1582. doi:10.1029/jd093id02p01571
Bartlett, K., Crill, P. M., Bonassi, J., Richey, J. E., and Harriss, R. (1990). Methane Flux from the Amazon River Floodplain: Emissions during Rising Water. J. Geophys. Res. 95 (16), 773788. doi:10.1029/jd095id10p16773
Basso, L. S., Gatti, L. V., Gloor, M., Miller, J. B., Domingues, L. G., Correia, C. S. C., et al. (2016). Seasonality and Interannual Variability of CH4 Fluxes from the Eastern Amazon Basin Inferred from Atmospheric Mole Fraction Profiles. J. Geophys. Res. Atmos. 121, 168–184. doi:10.1002/2015jd023874
Basso, L. S., Marani, L., Gatti, L. V., Miller, J. B., Gloor, M., Melack, J., et al. (2021). Amazon Methane Budget Derived from Multi-Year Airborne Observations Highlights Regional Variations in Emissions. Commun. Earth Environ. 2, 246. doi:10.1038/s43247-021-00314-4
Beck, V., Chen, H., Gerbig, C., Bergamaschi, P., Bruhwiler, L., Houweling, S., et al. (2012). Methane Airborne Measurements and Comparison to Global Models during BARCA. J. Geophys. Res. 117, a–n. doi:10.1029/2011JD017345
Beighley, R. E., and Gummadi, V. (2011). Developing Channel and Floodplain Dimensions with Limited Data: a Case Study in the Amazon Basin. Earth Surf. Process. Landforms 36, 1059–1071. doi:10.1002/esp.2132
Belger, L., Forsberg, B. R., and Melack, J. M. (2011). Carbon Dioxide and Methane Emissions from Interfluvial Wetlands in the Upper Negro River Basin, Brazil. Biogeochemistry 105, 171–183. doi:10.1007/s10533-010-9536-0
Bento, M. d. S., Barros, D. J., Araújo, M. G. d. S., Da Róz, R., Carvalho, G. A., do Carmo, J. B., et al. (2021). Active Methane Processing Microbes and the Disproportionate Role of NC10 Phylum in Methane Mitigation in Amazonian Floodplains. Biogeochemistry 156, 293–317. doi:10.1007/s10533-021-00846-z
Bergamaschi, P., Frankenberg, C., Meirink, J. F., Krol, M., Villani, M. G., Houweling, S., et al. (2009). Inverse Modeling of Global and Regional CH4 Emissions Using SCIAMACHY Satellite Retrievals. J. Geophys. Res. 114, D22301. doi:10.1029/2009JD012287
Bertassoli, D. J., Sawakuchi, H. O., de Araújo, K. R., de Camargo, M. G. P., Alem, V. A. T., and Pereira, T. S. (2021). How Green Can Amazon Hydropower Be? Net Carbon Emission from the Largest Hydropower Plant in Amazonia. Sci. Adv. 7, eabe1470.
Bloom, A. A., Bowman, K. W., Lee, M., Turner, A. J., Schroeder, R., Worden, J. R., et al. (2017). A Global Wetland Methane Emissions and Uncertainty Dataset for Atmospheric Chemical Transport Models (WetCHARTs Version 1.0). Geosci. Model. Dev. 10, 2141–2156. doi:10.5194/gmd-10-2141-2017
Bloom, A. A., Lauvaux, T., Worden, J., Yadav, V., Duren, R., Sander, S. P., et al. (2016). What Are the Greenhouse Gas Observing System Requirements for Reducing Fundamental Biogeochemical Process Uncertainty? Amazon Wetland CH4 Emissions as a Case Study. Atmos. Chem. Phys. 16, 15199–15218. doi:10.5194/acp-16-15199-2016
Bloom, A. A., Palmer, P. I., Fraser, A., and Reay, D. S. (2012). Seasonal Variability of Tropical Wetland CH4 Emissions: the Role of the Methanogen-Available Carbon Pool. Biogeosciences 9, 2821–2830. doi:10.5194/bg-9-2821-2012
Bontemps, S., Defourny, P., van Bogaert, E., Arino, O., Kalagirou, V., and Ramos Peres, J. (2011). Globcover Products Description and Validation Report. Technical report, ESA. URL: epic.awi.de/id/eprint/31014/16/GLOBCOVER2009_284 (Validation_Report_2-2.pdf.285.
Borrel, G., Jézéquel, D., Biderre-Petit, C., Morel-Desrosiers, N., Morel, J.-P., Peyret, P., et al. (2011). Production and Consumption of Methane in Freshwater Lake Ecosystems. Res. Microbiol. 162, 832–847. doi:10.1016/j.resmic.2011.06.004
Botía, S., Galkowski, M., Marshall, J., Koch, T., Lavric, J. V., Walter, D., et al. (2020b). Six Years of Atmospheric CO2, CH4 and CO at the Amazon Tall Tower Observatory: A New Opportunity to Study Processes on Seasonal and Inter-annual Scales. Abstract GC009-0013. Fall 2020 American Geophysical Union conference.
Botía, S., Gerbig, C., Marshall, J., Lavric, J. V., Walter, D., Pöhlker, C., et al. (2020a). Understanding Nighttime Methane Signals at the Amazon Tall Tower Observatory (ATTO). Atmos. Chem. Phys. 20, 6583–6606. doi:10.5194/acp-20-6583-2020
Bridgham, S. D., Cadillo-Quiroz, H., Keller, J. K., and Zhuang, Q. (2013). Methane Emissions from Wetlands: Biogeochemical, Microbial, and Modeling Perspectives from Local to Global Scales. Glob. Change Biol. 19, 1325–1346. doi:10.1111/gcb.12131
C3S (2017). ERA5: Fifth Generation of ECMWF Atmospheric Reanalyses of the Global Climate. URL: https://cds.climate.copernicus.eu/cdsapp#!/home.
Caldwell, S. L., Laidler, J. R., Brewer, E. A., Eberly, J. O., Sandborgh, S. C., and Colwell, F. S. (2008). Anaerobic Oxidation of Methane: Mechanisms, Bioenergetics, and the Ecology of Associated Microorganisms. Environ. Sci. Techn. 42, 6791–6799.
Cao, M., Marshal, S., and Gregson, K. (1996). Global Carbon Exchange and Methane Emission from Natural Wetlands: Application of a Process-Based Model. J. Geophys. Res. 101 (14399-14), 414. doi:10.1029/96jd00219
Cardoso, S. J., Enrich-Prast, A., Pace, M. L., and Roland, F. (2014). Do models of Organic Carbon Mineralization Extrapolate to Warmer Tropical Sediments? Limnol. Oceanogr. 59, 48–54.
Carmo, J. B. d., Keller, M., Dias, J. D., Camargo, P. B. d., and Crill, P. (2006). A Source of Methane from Upland Forests in the Brazilian Amazon. Geophys. Res. Lett. 33, L04809. doi:10.1029/2005gl025436
Cassol, H. L. G., Domingues, L. G., Sanchez, A. H., Basso, L. S., Marani, L., Tejada, G., et al. (2020). Determination of Region of Influence Obtained by Aircraft Vertical Profiles Using the Density of Trajectories from the HYSPLIT Model. Atmosphere 11, 1073. doi:10.3390/atmos11101073
Castello, L., McGrath, D. G., Hess, L. L., Coe, M. T., Lefebvre, P. A., Petry, P., et al. (2013). The Vulnerability of Amazon Freshwater Ecosystems. Conserv. Lett. 6, 217–229. doi:10.1111/conl.12008
Chambers, J. Q., Tribuzy, E. S., Toledo, L. C., Crispim, B. F., Higuchi, N., J. dos Santos, J., et al. (2004). Respiration from a Tropical Forest Ecosystem: Partitioning of Sources and Low Carbon Use Efficiency. Ecol. Applic 14, S72–S88.
Chapman, B., Mcdonald, K., Shimada, M., Rosenqvist, A., Schroeder, R., and Hess, L. (2015). Mapping Regional Inundation with Spaceborne L-Band SAR. Remote Sens. 7, 5440–5470. doi:10.3390/rs70505440
Clark, D. B., Mercado, L. M., Sitch, S., Jones, C. D., Gedney, N., Best, M. J., et al. (2011). The Joint UK Land Environment Simulator (JULES), Model Description - Part 2: Carbon Fluxes and Vegetation Dynamics. Geosci. Model. Dev. 4, 701–722. doi:10.5194/gmd-4-701-2011
Coe, M. T., Costa, M. H., and Howard, E. (2007). Simulating the Surface Waters of the Amazon River Basin: Impacts of New River Geomorphic and Dynamic Flow Parameterizations. Hydrol. Process. 22, 2542–2553.
Cole, J. J., and Caraco, N. F. (1998). Atmospheric Exchange of Carbon Dioxide in a Low-Wind Oligotrophic Lake Measured by the Addition of SF6. Limnol. Oceanogr. 43, 647–656. doi:10.4319/lo.1998.43.4.0647
Conrad, R., Ji, Y., Noll, M., Klose, M., Claus, P., and Enrich-Prast, A. (2014). Response of the Methanogenic Microbial Communities in Amazonian Oxbow Lake Sediments to Desiccation Stress. Environ. Microbiol. 16, 1682–1694. doi:10.1111/1462-2920.12267
Conrad, R., Klose, M., Claus, P., and Enrich-Prast, A. (2010). Methanogenic Pathway, 13C Isotope Fractionation, and Archaeal Community Composition in the Sediment of Two Clear-Water Lakes of Amazonia. Limnol. Ocean. 55, 689–702. doi:10.4319/lo.2009.55.2.0689
Conrad, R., Noll, M., Claus, P., Klose, M., Bastos, W. R., and Enrich-Prast, A. (2011). Stable Carbon Isotope Discrimination and Microbiology of Methane Formation in Tropical Anoxic Lake Sediments. Biogeosciences 8, 795–814. doi:10.5194/bg-8-795-2011
Crill, P. M., Bartlett, K. B., Wilson, J. O., Sebacher, D. I., Harriss, R. C., Melack, J. M., et al. (1988). Tropospheric Methane from an Amazonian Floodplain Lake. J. Geophys. Res. 93, 1564–1570. doi:10.1029/jd093id02p01564
Crippa, M., Oreggioni, G., Guizzardi, D., Muntean, M., Schaaf, E., Lo Vullo, E., et al. (2019). Fossil CO2 and GHG Emissions of All World Countries. doi:10.2760/655913
Dacey, J. W. H. (1981). Pressurized Ventilation in the Yellow Waterlily. Ecology 62, 1137–1147. doi:10.2307/1937277
Dalmagro, H. J., Zanella de Arruda, P. H., Vourlitis, G. L., Lathuillière, M. J., de S. Nogueira, J., Couto, E. G., et al. (2019). Radiative Forcing of Methane Fluxes Offsets Net Carbon Dioxide Uptake for a Tropical Flooded Forest. Glob. Change Biol. 25, 1967–1981. doi:10.1111/gcb.14615
Deemer, B. R., Harrison, J. A., Li, S., Beaulieu, J. J., DelSontro, T., Barros, N., et al. (2016). Greenhouse Gas Emissions from Reservoir Water Surfaces: A New Global Synthesis. BioScience 66, 949–964. doi:10.1093/biosci/biw117
del Aguila-Pasquel, J. (2017). Methane Fluxes and Porewater Dissolved Organic Carbon Dynamics from Different Peatland Types in the Pastaza-Marañon Basin of the Peruvian Amazon. Houghton, MI: Michigan Technological University. Open Access Master's thesis. doi:10.37099/mtu.dc.etdr/494
DelSontro, T., Beaulieu, J. J., and Downing, J. A. (2018). Greenhouse Gas Emissions from Lakes and Impoundments: Upscaling in the Face of Global Change. Limnol. Oceanogr. Lett. 3, 64–75. doi:10.1002/lol2.10073
DelSontro, T., Kunz, M. J., Kempter, T., Wüest, A., Wehrli, B., and Senn, D. B. (2011). Spatial Heterogeneity of Methane Ebullition in a Large Tropical Reservoir. Environ. Sci. Technol. 45, 9866–9873. doi:10.1021/es2005545
Delwiche, K. B., Knox, S. H., Malhotra, A., Fluet-Chouinard, E., McNicol1, G., Feron, S., et al. (2021). FLUXNET- CH4: A Global, Multi-Ecosystem Dataset and Analysis of Methane Seasonality from Freshwater Wetlands. Earth Sys. Sci. Data Disc. doi:10.5194/essd-2020-307
Departamento Nacional da Produção Mineral (1978). Projeto RadamBrasil. Folha SA 20: Manaus. Rio de Janeiro, Brazil: Departamento Nacional da Produção Mineral.
Devol, A. H., Richey, J. E., Clark, W. A., King, S. L., and Martinelli, L. A. (1988). Methane Emissions to the Troposphere from the Amazon Floodplain. J. Geophys. Res. 93, 1583–1592. doi:10.1029/jd093id02p01583
Devol, A., Richey, J., Forsberg, B., and Martinelli, L. (1994). “Environmental Methane in the Amazon River Floodplain,” in Global Wetlands: Old World and New. Editor W. J. Mitsch (Elsevier), 151–165.
Devol, A., Richey, J., Forsberg, B., and Martinelli, L. (1990). Seasonal Dynamics in Methane Emissions from the Amazon River Floodplain to the Troposphere. J. Geophys. Res. 95, 417–426. doi:10.1029/jd095id10p16417
Draper, F. C., Roucoux, K. H., Lawson, I. T., Mitchard, E. T. A., Honorio Coronado, E. N., Lähteenoja, O., et al. (2014). The Distribution and Amount of Carbon in the Largest Peatland Complex in Amazonia. Environ. Res. Lett. 9. doi:10.1088/1748-9326/9/12/124017
Engle, D. L., Melack, J. M., Doyle, R. D., and Fisher, T. R. (2008). High Rates of Net Primary Production and Turnover of Floating Grasses on the Amazon Floodplain: Implications for Aquatic Respiration and Regional CO2 Flux. Glob. Change Biol. 14, 369–381. doi:10.1111/j.1365-2486.2007.01481.x
Engle, D., and Melack, J. M. (2000). Methane Emissions from the Amazon Floodplain: Enhanced Release during Episodic Mixing of Lakes. Biogeochemistry 51, 71–90. doi:10.1023/a:1006389124823
Espinoza, J.-C., Marengo, J. A., Schongart, J., and Jimenez, J. C. (2021). The New Historical Flood of 2021 in the Amazon River Compared to Major Floods of the 21st Century: Atmospheric Features in the Context of the Intensification of Floods. Weather Clim. Extrem. 35, 100406. doi:10.1016/j.wace.2021.100406
Espinoza Villar, J. C., Guyot, J. L., Ronchail, J., Cochonneau, G., Filizola, N., Fraizy, P., et al. (2009). Contrasting Regional Discharge Evolutions in the Amazon Basin (1974-2004). J. Hydrology 375, 297–311. doi:10.1016/j.jhydrol.2009.03.004
Fassoni-Andrade, A. C., Fleischmann, A. S., Papa, F., Paiva, R. C. D., Wongchuig, S., Melack, J. M., et al. (2021). Amazon Hydrology from Space: Scientific Advances and Future Challenges. Rev. Geophys. 59, e2020RG000728. doi:10.1029/2020RG000728
Fassoni-Andrade, A. C., Paiva, R. C. D. d., Rudorff, C. d. M., Barbosa, C. C. F., and Novo, E. M. L. d. M. (2020). High-resolution Mapping of Floodplain Topography from Space: A Case Study in the Amazon. Remote Sens. Environ. 251, 112065. doi:10.1016/j.rse.2020.112065
Ferreira-Ferreira, J., Silva, T. S. F., Streher, A. S., Affonso, A. G., de Almeida Furtado, L. F., Forsberg, B. R., et al. (2015). Combining ALOS/PALSAR Derived Vegetation Structure and Inundation Patterns to Characterize Major Vegetation Types in the Mamirauá Sustainable Development Reserve, Central Amazon Floodplain, Brazil. Wetl. Ecol. Manage 23, 41–59. doi:10.1007/s11273-014-9359-1
Finn, D. R., Ziv-El, M., van Haren, J., Park, J. G., del Aguila-Pasquel, J., Urquiza–Muñoz, J. D., et al. (2020). Methanogens and Methanotrophs Show Nutrient-dependent Community Assemblage Patterns across Tropical Peatlands of the Pastaza-Marañón Basin, Peruvian Amazonia. Front. Microbiol. 11, 746. doi:10.3389/fmicb.2020.00746
Fleischmann, A. S., Paiva, R. C. D., Collischonn, W., Siqueira, V. A., Paris, A., Moreira, D. M., et al. (2020). Trade‐Offs between 1‐D and 2‐D Regional River Hydrodynamic Models. Water Resour. Res. 56. doi:10.1029/2019WR026812
Fleischmann, A. S., Papa, F., Fassoni-Andrade, A., Melack, J. M., Wongchuig, S., Paiva, R. C. D., et al. (2021). How Much Inundation Occurs in the Amazon River Basin? Remote Sens. Environ. Prepr. doi:10.1002/essoar.10508718.1
Fluet-Chouinard, E., Lehner, B., Rebelo, L. M., Papa, F., and Hamilton, S. K. (2015). Development of a Global Inundation Map at High Spatial Resolution from Topographic Downscaling of Coarse-Scale Remote Sensing Data. Remote Sens. Environ. 158, 348–361. doi:10.1016/j.rse.2014.10.015
Forsberg, B. R., Dunne, T., Melack, J. M., Venticinque, E., Goulding, M., Barthem, R., et al. (2017). Potential Impact of New Andean Dams on the Amazon Fluvial Ecosystems. PLOS One 12 (8), e0182254.
Forsberg, B. R., Melack, J. M., Richey, J. E., and Pimentel, T. P. (2017). Planktonic Photosynthesis and Respiration in Amazon Floodplain Lakes. Hydrobiologia 800, 187–206.
Frankenberg, C., Aben, I., Bergamaschi, P., Dlugokencky, E. J., Van Hees, R., Houweling, S., et al. (2011). Global Column-Averaged Methane Mixing Ratios from 2003 to 2009 as Derived from SCIAMACHY: Trends and Variability. J. Geophys. Res. Atmos. 116, 1–12. doi:10.1029/2010JD014849
Frankenberg, C., Bergamaschi, P., Butz, A., Houweling, S., Meirink, J. F., Notholt, J., et al. (2008). Tropical Methane Emissions: A Revised View from SCIAMACHY Onboard ENVISAT. Geophys. Res. Lett. 35. doi:10.1029/2008GL034300
Frappart, F., Seyler, F., Martinez, J.-M., Leon, J. G., and Cazenave, A. (2005). Flooplain Water Storage in the Negro River Basin Estimated from Microwave Remote Sensing of Inundated Area and Water Levels. Remote Sens. Environ. 99, 387–399.
Fraser, A., Palmer, P. I., Feng, L., Bösch, H., Parker, R., Dlugokencky, E. J., et al. (2014). Estimating Regional Fluxes of CO2 and CH4 Using Space-Borne Observations of XCH4: XCO2. Atmos. Chem. Phys. 14, 12883–12895.
Gabriel, G. V. M., Oliveira, L. C., Barros, D. J., Bento, M. S., Neu, V., Toppa, R. H., et al. (2020). Methane Emission Suppression in Flooded Soil from Amazonia. Chemosphere 250, 126263. doi:10.1016/j.chemosphere.2020.126263
Gatti, L., Melack, J., Basso, L. S., Restrepo-Coupe, N., Pangala, S., Saleska, S. R., et al. (2021a). “Amazon Carbon Budget. Cross-Chapter,” in Amazon Assessment Report. Editors C. Nobre, A. Encalada, E. Anderson, F. H. Roca Alcazar, and M. Bustamante (New York, USA: United Nations Sustainable Development Solutions Network). Available at: www.theamazonwewant.org/spa-reports.
Gatti, L. V., Basso, L. S., Miller, J. B., Gloor, M., Domingues, L. G., Cassol, H. L. G., et al. (2021b). Amazonia as a Carbon Source Linked to Deforestation and Climate Change. Nature 595, 388–393.
Gauci, V., Figueiredo, V., Gedney, N., Pangala, S. R., Stauffer, T., Weedon, G. P., et al. (2021). Non-flooded Riparian Amazon Trees Are a Regionally Significant Methane Source. Phil. Trans. R. Soc. A, 3802020044620200446. doi:10.1098/rsta.2020.0446
Gedney, N., Huntingford, C., Comyn-Platt, E., and Wiltshire, A. (2019). Significant Feedbacks of Wetland Methane Release on Climate Change and the Causes of Their Uncertainty. Environ. Res. Lett. 14, 084027. doi:10.1088/1748-9326/ab2726
Gloor, M., Brienen, R. J. W., Galbraith, D., Feldpausch, T. R., Schöngart, J., Guyot, J. L., et al. (2013). Intensification of the Amazon Hydrological Cycle over the Last Two Decades. Geophys. Res. Lett. 40, 1729–1733. doi:10.1002/grl.50377
Gloor, M., Gatti, L. V., Wilson, C., Parker, R. J., Boesch, H., Popa, E., et al. (2021). Large Methane Emissions from the Pantanal during Rising Water-Levels Revealed by Regularly Measured Lower Troposphere CH4 Profiles. Glob. Biogeochem. Cycles 35, e2021GB006964. doi:10.1029/2021GB006964
Gontijo, J. B., Paula, F. S., Venturini, A. M., Yoshiura, C. A., Borges, C. D., Moura, J. M. S., et al. (2021). Not just a Methane Source: Amazonian Floodplain Sediments Harbour a High Diversity of Methanotrophs with Different Metabolic Capabilities. Molec. Ecol. 30, 2560–2572.
Griffis, T. J., Roman, T. D., Wood, J. D., Deventer, M. J., Fachin, L., Rengifo, J., et al. (2020). Hydrometeorological Sensitivities of Net Ecosystem Carbon Dioxide and Methane Exchange of an Amazonian Palm Swamp Peatland. Agric. For. Meteorol. 295. doi:10.1016/j.agrformet.2020.108167
Grossart, H.-P., Frindte, K., Dziallas, C., Eckert, W., and Tang, K. W. (2011). Microbial Methane Production in Oxygenated Water Column of an Oligotrophic Lake. Proc. Nat. Acad. Sci. 108, 19657–19661.
Guérin, F., and Abril, G. (2007). Significance of Pelagic Aerobic Methane Oxidation in the Methane and Carbon Budgets of a Tropical Reservoir. J. Geophys. Res. Biogeosci. 112. doi:10.1029/2006JG000393
Guérin, F., Abril, G., Richard, S., Burban, B., Reynouard, C., Seyler, P., et al. (2006). Methane and Carbon Dioxide Emissions from Tropical Reservoirs: Significance of Downstream Rivers. Geophys. Res. Lett. 33. doi:10.1029/2006GL027929
Gumbricht, T., Roman-Cuesta, R. M., Verchot, L., Herold, M., Wittmann, F., Householder, E., et al. (2017). An Expert System Model for Mapping Tropical Wetlands and Peatlands Reveals South America as the Largest Contributor. Glob. Chang. Biol. 23, 3581–3599. doi:10.1111/gcb.13689
Guo, M., Zhuang, Q., Tan, Z., Shurpali, N., Juutinen, S., Kortelainen, P., et al. (2020). Rising Methane Emissions from Boreal Lakes Due to Increasing Ice-free Days. Environ. Res. Lett. 15, 064008. doi:10.1088/1748-9326/ab8254
Guseva, S., Aurela, M., Cortés, A., Lotsar, E., MacIntyre, S., Mammarella, I., et al. (2021). Physical Drivers of Near-Surface Turbulence in a Regulated River. Water Resour. Res. 57, e2020WR027939.
Guyot, J. L., Jouanneau, J. M., Soares, L., Boaventura, G. R., Maillet, N., and Lugane, C. (2007). Clay Mineral Composition of River Sediments in the Amazon Basin. Catena 71, 340–356.
Hamilton, S. K., Melack, J. M., Goodchild, M. F., and Lewis, W. M. (1992). “Estimation of the Fractual Dimension of Terrain from Lake Size Distributions,” in Lowland Floodplain Rivers: Geomorphological Perspectives. Editors P. A. Carling, and G. E. Petts (New York: John Wiley & Sons), 145–163.
Hamilton, S. K., Sippel, S. K., and Melack, J. M. (2002). Comparison of Inundation Patterns Among Major South American Floodplains. J. Geophys. Res. 107–D20. doi:10.29/2000JD000306
Hamilton, S., Sippel, S., and Melack, J. M. (1995). Oxygen Depletion and Carbon Dioxide Production in Waters of the Pantanal Wetland of Brazil. Biogeochemistry 30, 115–141.
Hastie, A., Lauerwald, R., Ciais, P., and Regnier, P. (2019). Aquatic Carbon Fluxes Dampen the Overall Variation of Net Ecosystem Productivity in the Amazon Basin: An Analysis of the Interannual Variability in the Boundless Carbon Cycle. Glob. Chang. Biol. 25, 2094–2111. doi:10.1111/gcb.14620
Hawes, J. E., Peres, C. A., Riley, L. B., and Hess, L. L. (2012). Landscape-scale Variation in Structure and Biomass of Amazonian Seasonally Flooded and Unflooded Forests. For. Ecol. Manage. 281, 163–176.
Hedges, J. I., Clark, W. A., Quay, P. D., Richey, J. E., Devol, A., and Santos, U. (1986). Composition and Fluxes of Particulate Organic Material in the Amazon River. Limnol. Oceanogr. 31, 717–738.
Hess, L. L., Melack, J. M., Affonso, A. G., Barbosa, C., Gastil, M., and Novo, E. M. L. M. (2015). Amazonian Wetlands: Extent, Vegetative Cover, and Dual Season Inundation Area. Wetlands 35, 745–756.
Hess, L. L., Melack, J. M., Novo, E. M. L. M., Barbosa, C. C. F., and Gastil, M. (2003). Dual-season Mapping of Wetland Inundation and Vegetation for the Central Amazon Basin. Remote Sens. Environ. 87, 404–428.
Hess, L. L., Novo, E. M. L. M., Slaymaker, D. M., Holt, J., Steffen, C., Valeriano, D. M., et al. (2002). Geocoded Digital Videography for Validation of Land Cover Mapping in the Amazon Basin. Int. J. Remote Sens. 7, 1527–1556.
Hodges, B. R., and Dallimore, C. (2019). Aquatic Ecosystem Model: AEM3D, User Manual. Australia: Hydronumerics. Docklands.
Isidorova, A., Grasset, C., Mendonça, R., and Sobek, S. (2019). Methane Formation in Tropical Reservoirs Predicted from Sediment Age and Nitrogen. Sci. Rep. 9, 11017. doi:10.1038/s41598-019-47346-7
Jati, S. R. (2014). Emissão de CO2 e CH4 nas savanas úmidas de Roraima. Manaus, Brazil: M.Sc. thesis. Instituto Nacional de Pesquisas da Amazonia.
Jensen, K., McDonald, K., Podest, E., Rodriguez-Alvarez, N., Horna, V., and Steiner, N. (2018). Assessing L-Band GNSS-Reflectometry and Imaging Radar for Detecting Sub-canopy Inundation Dynamics in a Tropical Wetlands Complex. Remote Sens. 10, 1431. doi:10.3390/rs10091431
Ji, X., Lesack, L. F. W., Melack, J. M., Wang, S., Riley, W. J., and Shen, C. (2019). Seasonal Patterns and Controls of Hydrological Fluxes in an Amazon Floodplain Lake with a Surface-Subsurface Processes Model. Water Resour. Res. 55, 3056–3075. doi:10.1029/2018WR023897
Ji, Y., Angel, R., Klose, M., Claus, P., Marotta, H., Pinho, L., et al. (2016). Structure and Function of Methanogenic Microbial Communities in Sediments of Amazonian Lakes with Different Water Types. Environ. Microbiol. 18, 5082–5100. doi:10.1111/1462-2920.13491
W. J. Junk (Editor) (1997). “Central Amazon Floodplain: Ecology of a Pulsing System, (Berlin, Germany: Spinger), 126
Junk, W. J., and Piedade, M. T. F. (1997). “Plant Life in the Floodplain with Special Reference to Herbaceous Plants,” in Central Amazon Floodplain: Ecology of a Pulsing System. Editor W. J. Junk (Berlin, Germany: Spinger: Ecological Studies), 126, 147–185.
Junk, W. J., Piedade, M. T. F., Schöngart, J., Cohn-Haft, M., Adeney, J. M., and Wittmann, F. (2011). A Classification of Major Naturally-Occurring Amazonian Lowland Wetlands. Wetlands 31, 623–640.
Junk, W. J., Piedade, M., Wittmann, F., Schöngart, J., and Parolin, P. (Editors) (2010). Amazonian Floodplain Forests: Ecophysiology, Ecology, Biodiversity and Sustainable Management (Berlin, Germany: Springer). Ecological Studies 210.
Kasischke, E. S., Melack, J. M., and Dodson, M. C. (1997). The Use of Imaging Radar for Ecological Applications-A Review. Remote Sens. Environ. 59, 141–156.
Keller, M., Varner, R., Dias, J. D., Silva, H., Crill, P., Oliveira, R. C., et al. (2005). Soil–atmosphere Exchange of Nitrous Oxide, Nitric Oxide, Methane, and Carbon Dioxide in Logged and Undisturbed Forest in the Tapajos National Forest, Brazil. Earth Interact. 9 (23), 1–28.
Kemenes, A., Forsberg, B. R., and Melack, J. M. (2011). CO2 Emissions from a Tropical Hydroelectric Reservoir (Balbina, Brazil). J. Geophys. Res. Biogeosci. 116, G03004. doi:10.1029/2010JG001465
Kemenes, A., Forsberg, B. R., and Melack, J. M. (2016). Downstream Emissions of CH4 and CO2 from Amazon Hydroelectric Reservoirs (Tucuruí, Samuel and Curuá-Una). Inland Waters 6, 295–302.
Kemenes, A., Forsberg, B. R., and Melack, J. M. (2007). Methane Release below a Hydroelectric Dam. Geophys. Res. Lett. 34, L12809.
Knox, S. H., Bansal, S., McNicol, G., Schafer, K., Sturtevant, C., Ueyama, M., et al. (2021). Identifying Dominant Environmental Predictors of Freshwater Wetland Methane Fluxes across Diurnal to Seasonal Time Scales. Glob. Change Biol. 27, 3582–3604. doi:10.1111/gcb.15661
Koschorreck, M. (2000). Methane Turnover in Exposed Sediments of an Amazon Floodplain Lake. Biogeochemistry 50, 195–206.
Kosten, S., van den Berg, S., Mendonça, R., Paranaíba, J. R., Roland, F., Sobek, S., et al. (2018). Extreme Drought Boosts CO2 and CH4 Emissions from Reservoir Drawdown Areas. Inland waters. 8, 329–340. doi:10.1080/20442041.2018.1483126
Lähteenoja, O., Reátegui, Y. R., Räsänen, M., Torres, D. D. C., Oinonen, M., and Page, S. (2012). The Large Amazonian Peatland Carbon Sink in the Subsiding Pastaza-Marañón Foreland Basin, Peru. Glob. Change Biol. 18, 164–178. doi:10.1111/j.1365-2486.2011.02504.x
Lähteenoja, O., Ruokolainen, K., Schulman, L., and Alvarez, J. (2009). Amazonian Floodplains Harbour Minerotrophic and Ombrotrophic Peatlands. Catena 79, 140–145.
Lauerwald, R., Regnier, P., Camino-Serrano, M., Guenet, B., Guimberteau, M., Ducharne, A., et al. (2017). ORCHILEAK (Revision 3875): a New Model Branch to Simulate Carbon Transfers along the Terrestrial-Aaquatic Continuum of the Amazon Basin. Geosci. Model. Dev. 10, 3821–3859. doi:10.5194/gmd-10-3In821-2017
Lehner, B., and Döll, P. (2004). Development and Validation of a Global Database of Lakes, Reservoirs and Wetlands. J. Hydrol. 296, 1–22.
Leitão, R. P., Zuanon, J., Mouillot, D., Leal, C. G., Hughes, R. M., Kaufmann, P. R., et al. (2018). Disentangling the Pathways of Land Use Impacts on the Functional Structure of Fish Assemblages in Amazon Streams. Ecography 41, 219–232.
Lesack, L. F. W., and Melack, J. M. (1995). Flooding Hydrology and Mixture Dynamics of Lake Water Derived from Multiple Sources in an Amazon Floodplain Lake. Water Resour. Res. 31, 329–345.
Linkhorst, A., Hiller, C., DelSontro, T., Azevedo, G., Barros, N., Mendonça, R. F., et al. (2020). Comparing Methane Ebullition Variability across Space and Time in a Brazilian Reservoir. Limnol. Oceanogr. 65, 1623–1634. doi:10.1002/lno.11410
Liu, L., Zhuang, Q., Oh, Y., Shurpali, N. J., Kim, S., and Poulter, B. (2020). Uncertainty Quantification of Global Net Methane Emissions from Terrestrial Ecosystems Using a Mechanistically Based Biogeochemistry Model. J. Geophys. Res. Biogeosci 125, e2019JG005428. doi:10.1029/2019JG005428
Lu, X., Zhuang, Q., Liu, Y., Zhou, Y., and Aghakouchak, A. (2016). A Large-Scale Methane Model by Incorporating the Surface Water Transport. J. Geophys. Res. Biogeosci. 121. doi:10.1002/2016JG003321
Lunt, M. F., Palmer, P. I., Lorente, A., Borsdorff, T., Landgraf, J., Parker, R. J., et al. (2021). Rain-fed Pulses of Methane from East Africa during 2018–2019 Contributed to Atmospheric Growth Rate. Environ. Res. Lett. 16, 024021.
Ma, S., Worden, J. R., Bloom, A. A., Zhang, Y., Poulter, B., Cusworth, D. H., et al. (2021). Satellite Constraints on the Latitudinal Distribution and Temperature Sensitivity of Wetland Methane Emissions. AGU Adv. 2, e2021AV000408. doi:10.1029/2021AV000408
Macedo, M. N., Coe, M. T., DeFries, R., Uriarte, M., Brando, P. M., Neill, C., et al. (2013). Land-use-driven Stream Warming in Southeastern Amazonia. Philos. Trans. R. Soc. Lond B Biol. Sci. 368, 20120153. doi:10.1098/rstb.2012.0153
MacIntyre, S., Amaral, J. H. F., Barbosa, P. M., Cortés, A., Forsberg, B. R., and Melack, J. M. (2019). Turbulence and Gas Transfer Velocities in Sheltered Flooded Forests of the Amazon Basin. Geophys. Res. Lett. doi:10.1029/2019GL083948
MacIntyre, S., Amaral, J. H., and Melack, J. M. (2021). Turbulence in the Upper Mixed Layer under Light Winds: Implications for Fluxes of Climate-Warming Trace Gases. J. Geophys. Res. Oceans 126, e2020JC017026. doi:10.1029/2020JC017026
MacIntyre, S., and Melack, J. M. (1995). Vertical and Horizontal Transport in Lakes: Linking Littoral, Benthic, and Pelagic Habitats. J. N. Amer. Benth. Soc. 14, 599–615.
Malhi, Y., Melack, J., Gatti, L. V., Ometto, J., Kesselmeier, J., Wolff, S., et al. (2021). “Chapter 6 – Biogeochemical Cycles: Carbon and Other Elements,” in Amazon Assessment Report. Editors C. Nobre, A. Encalada, E. Anderson, F. H. Roca Alcazar, and M. Bustamante (New York, USA: United Nations Sustainable Development Solutions Network). Available at: www.theamazonwewant.org/spa-reports.
Marani, L., and Alvala, P. C. (2007). Methane Emissions from Lakes and Floodplains in Pantanal, Brazil. Atmos. Environ. 41, 1627–1633.
Marengo, J. A., and Espinoza, J. C. (2016). Extreme Seasonal Droughts and Floods in Amazonia: Causes, Trends and Impacts. Intern. J. Clim. 36, 1033–1050.
Martinelli, L. A., Victoria, R. L., de Camargo, P. B., Piccolo, M. C., Mertes, L., Richey, J. E., et al. (2003). Inland Variability of Carbon–Nitrogen Concentrations and δ13C in Amazon Floodplain (Várzea) Vegetation and Sediment. Hydrol. Process. 17, 1419–1430.
Martinson, G. O., Werner, F. A., Scherber, C., Conrad, R., Corre, M. D., Flessa, H., et al. (2010). Methane Emissions from Tank Bromeliads in Neotropical Forests. Nat. Geosci. 3, 766–769.
Mayorga, E., Aufdenkampe, A. K., Masiello, C. A., Krusche, A. V., Hedges, J. I., Quay, P. D., et al. (2005). Young Organic Matter as a Source of Carbon Dioxide Outgassing from Amazonian Rivers. Nature 436, 538–541. doi:10.1038/nature03880
Mayorga, E., and Aufdenkampe, A. (2002). Processing of Bioactive Elements in the Amazon River System. Ecohydrology of South American Rivers and Wetlands. IAHS Special Publication 6, 1–23.
McNorton, J., Gloor, E., Wilson, C., Hayman, G. D., Gedney, N., Comyn-Platt, E., et al. (2016). Role of Regional Wetland Emissions in Atmospheric Methane Variability. Geophys. Res. Lett. 43, 11433–11444. doi:10.1002/2016GL070649
Meade, R. H., Rayol, J. M., Conceição, S. C. Da., and Natividade, J. R. G. (1991). Backwater Effects in the Amazon River Basin. Environ. Geol. Water Sci. 18, 105–114. doi:10.1007/BF01704664
Melack, J. M., Amaral, J. H. F., Kasper, D., Barbosa, P. M., and Forsberg, B. R. (2021). Limnological Perspectives on Conservation of Aquatic Ecosystems in the Amazon Basin. Aquat. Cons. Mar. Freshw. Ecosys. 31, 1041–1055.
Melack, J. M. (2016). “Aquatic Ecosystems,” in Interactions between Biosphere, Atmosphere and Human Land Use in the Amazon Basin. Editors L. Nagy, B. R. Forsberg, and P. Artaxo (Berlin, Germany: Springer), 227, 117–145.)Ecol. Stud.
Melack, J. M., and Coe, M. T. (2021). Amazon Floodplain Hydrology and Implications for Aquatic Conservation. Aquat. Cons. Mar. Freshw. Ecosys. 31, 1029–1040.
Melack, J. M., and Engle, D. (2009). An Organic Carbon Budget for an Amazon Floodplain Lake. Verh. Intern. Ver. Limnol. 30, 1179–1182.
Melack, J. M., and Forsberg, B. (2001). “Biogeochemistry of Amazon Floodplain Lakes and Associated Wetlands,” in Biogeochemistry of the Amazon Basin. Editors M. E. McClain, R. L. Victoria, and J. E. Richey (Oxford, U.K.: Oxford University Press), 235–276.
Melack, J. M., Hess, L. L., Gastil, M., Forsberg, B. R., Hamilton, S. K., Lima, I. B. T., et al. (2004). Regionalization of Methane Emissions in the Amazon Basin with Microwave Remote Sensing. Glob. Change Biol. 10, 530–544.
Melack, J. M., and Hess, L. L. (2010). “Remote Sensing of the Distribution and Extent of Wetlands in the Amazon Basin,” in Amazonian Floodplain Forests: Ecophysiology, Ecology, Biodiversity and Sustainable ManagementEcological Studies. Editors W. J. Junk, M. Piedade, F. Wittmann, J. Schöngart, and P. Parolin (Berlin, Germany: Springer), 210, 43–59.)
Melack, J. M., Novo, E. M. L. M., Forsberg, B. R., Piedade, M. T. F., and Maurice, L. (2009). “Floodplain Ecosystem Processes,”. Editors J. Gash, M. Keller, and P. Silva-Dias (Washington, D.C: American Geophysical Union. Geophys. Monogr. Series), 186, 525–541.Amaz. Glob. Change
Melack, J. M. (2004). “Remote Sensing of Tropical Wetlands,” in Manual of Remote Sensing. Editor S. Ustin. 3rd ed. (New York, USA: John Wiley & Sons), 4, 319–343.
Melo, M. L., Bertilsson, S., Amaral, J. H. F., Barbosa, P. M., Forsberg, B. R., and Sarmento, H. (2019). Flood Pulse Regulation of Bacterioplankton Community Composition in an Amazonian Floodplain Lake. Freshw. Biol. 64, 108–120.
Melton, J. R., Wania, R., Hodson, E. L., Poulter, B., Ringeval, B., Spahni, R., et al. (2013). Present State of Global Wetland Extent and Wetland Methane Modelling: Conclusions from a Model Inter-comparison Project (WETCHIMP). Biogeosciences 10, 753–788.
Meng, L., Hess, P. G. M., Mahowald, N. M., Yavitt, J. B., Riley, W. J., Subin, Z. M., et al. (2012). Sensitivity of Wetland Methane Emissions to Model Assumptions: Application and Model Testing against Site Observations. Biogeosciences 9, 2793–2819. doi:10.5194/bg-9-2793-2012
Messager, M. L., Lehner, B., Grill, G., Nedeva, I., and Schmitt, O. (2016). Estimating the Volume and Age of Water Stored in Global Lakes Using a Geo-Statistical Approach. Nat. Commun. 7, 13603–13611. doi:10.1038/ncomms13603
Miguez-Macho, G., and Fan, Y. (2012). The Role of Groundwater in the Amazon Water Cycle: 1. Influence on Seasonal Streamflow, Flooding and Wetlands. J. Geophys. Res. 117 (D15). doi:10.1029/2012JD017539
Miller, J. B., Gatti, L. V., d’Amelio, M. T. S., Crotwell, A. M., Dlugokencky, E. J., Bakwin, P., et al. (2007). Airborne Measurements Indicate Large Methane Emissions from the Eastern Amazon Basin. Geophys. Res. Lett. 34. doi:10.1029/2006GL029213
Moreira-Turcq, P., Bonnet, M. P., Amorim, M., Bernardes, M., Lagane, C., Maurice, L., et al. (2013). Seasonal Variability in Concentration, Composition, Age, and Fluxes of Particulate Organic Carbon Exchanged between the Floodplain and Amazon River. Glob. Biogeochem. Cycles 27, 119–130.
Nardi, F., Annis, A., Di Baldassarre, G., Vivoni, E. R., and Grimaldi, S. (2019). GFPLAIN250m, a Global High-Resolution Dataset of Earth's Floodplains. Sci. Data 6, 180309. doi:10.1038/sdata.2018.309
Neu, V., Neill, C., and Krusche, A. V. (2011). Gaseous and Fluvial Carbon Export from an Amazon Forest Watershed. Biogeochemistry 105, 133–147.
Nisbet, E. G., Dlugokencky, E. J., Manning, M. R., Lowry, D., Fisher, R. E., France, J. L., et al. (2016). Rising Atmospheric Methane: 2007–2014 Growth and Isotopic Shift. Glob. Biogeochem. Cycles 30, 1356–1370. doi:10.1002/2016GB005406
Nobre, A. D., Cuartas, L. A., Hodnett, M., Renn, C. D., Rodrigues, G., Silveira, A., et al. (2011). Height above the Nearest Drainage—A Hydrologically Relevant New Terrain Model. J. Hydrol. 404, 13–29. doi:10.1016/j.jhydrol.2011.03.051
O'Loughlin, F. E., Paiva, R. C. D., Durand, M., Alsdorf, D. E., and Bates, P. D. (2016). A Multi-Sensor Approach towards a Global Vegetation Corrected SRTM DEM Product. Remote Sens. Environ. 182, 49–59.
Oliveira Junior, E. S., van Bergen, T. J. H. M., Nauta, J., Budiša, A., Aben, R. C. H., Weideveld, S. T. J., et al. (2020). Water Hyacinth’s Effect on Greenhouse Gas Fluxes: A Field Study in a Wide Variety of Tropical Water Bodies. Ecosystems. doi:10.1007/s10021-020-00564-x
Paiva, R. C. D., Buarque, D. C., Collischonn, W., Bonnet, M. P., Frappart, F., Calmant, S., et al. (2013). Large-scale Hydrologic and Hydrodynamic Modeling of the Amazon River Basin. Water Resour. Res. 49, 1226–1243. doi:10.1002/wrcr.20067
Pandey, S., Houweling, S., Krol, M., Aben, I., Monteil, G., Nechita-Banda, N., et al. (2017). Enhanced Methane Emissions from Tropical Wetlands during the 2011 La Niña. Sci. Rep. 7, 45759. doi:10.1038/srep45759
Pangala, S. R., Enrich-Prast, A., Basso, L. S., Peixoto, R. B., Bastviken, D., Hornibrook, E. R. C., et al. (2017). Large Emissions from Floodplain Trees Close the Amazon Methane Budget. Nature 552, 230–234. doi:10.1038/nature24639
Paranaíba, J. R., Aben, R., Barros, N., Quadra, G., Annika Linkhorst, A., Amado, A. M., et al. (2022). Cross-continental Importance of CH4 Emissions from Dry Inland-Waters. Sci.Total Environ. 814, 151925.
Paranaíba, J. R., Barros, N., Almeida, R. M., Linkhorst, A., Mendonça, R., do Vale, R., et al. (2021). Hotspots of Diffusive CO2 and CH4 Emission from Tropical Reservoirs Shift through Time. J. Geophys. Res. Biogeosci. 126, e2020JG006014. doi:10.1029/2020JG006014
Parker, R. J., Boesch, H., McNorton, J., Comyn-Platt, E., Gloor, M., Wilson, C., et al. (2018). Evaluating Year-To-Year Anomalies in Tropical Wetland Methane Emissions Using Satellite CH4 Observations. Remote Sens. Environ. 211, 261–275. doi:10.1016/j.rse.2018.02.011
Parker, R. J., Wilson, C., Bloom, A. A., Comyn-Platt, E., Hayman, G., McNorton, J., et al. (2020). Exploring Constraints on a Wetland Methane Emission Ensemble (WetCHARTs) Using GOSAT Satellite Observations. Biogeosciences 17, 5669–5691.
Parrens, M., Bitar, A. A., Frappart, F., Paiva, R., Wongchuig, S., Papa, F., et al. (2019). High Resolution Mapping of Inundation Area in the Amazon Basin from a Combination of L-Band Passive Microwave, Optical and Radar Datasets. Int. J. Appl. Earth Obs. Geoinf. 81, 58–71. doi:10.1016/j.jag.2019.04.011
Pinel, S., Bonnet, M., da Silva, J., Sampaio, T. C., Garnier, J., Catry, T., et al. (2019). Flooding Dynamics within an Amazonian Floodplain: Water Circulation Patterns and Inundation Duration. Water Resour. Res. 2019WR026081. doi:10.1029/2019WR026081
Pinel, S., Bonnet, M. P., Santos da Silva, J., Daniel Moreira, D., Calmant, S., Satgé, F., et al. (2015). Correction of Interferometric and Vegetation Biases in the SRTMGL1 Spaceborne DEM with Hydrological Conditioning towards Improved Hydrodynamics Modeling in the Amazon Basin. Remote Sens. 7, 16108–16130. doi:10.3390/rs71215822
Podgrajsek, E., Sahlée, E., Bastviken, D., Natchimuthu, S., Kljun, N., Chmiel, H. E., et al. (2016). Methane Fluxes from a Small Boreal Lake Measured with the Eddy Covariance Method. Limnol. Oceanogr. 61, S41–S50. doi:10.1002/ln0.10245
Polsenaere, P. D. J., Detandt, G., Vidal, L. O., Pérez, M. A. P., Marieu, V., and Abril, G. (2013). Thermal Enhancement of Gas Transfer Velocity of CO2 in an Amazon Floodplain Lake Revealed by Eddy Covariance Measurements. Geophys. Res. Lett. 40, 1–7.
Potter, C. S. (1997). An Ecosystem Simulation Model for Methane Production and Emission from Wetlands. Glob. Biogeochem. Cycles 11, 495–506.
Potter, C. S., Melack, J. M., and Engle, D. (2014). Modeling Carbon Dynamics and Methane Emissions from Amazon Floodplain Ecosystems. Wetlands 34, 501–511.
Prigent, C., Jimenez, C., and Bousquet, P. (2020). Satellite-derived Global Surface Water Extent and Dynamics over the Last 25 Years (GIEMS-2). J. Geophys. Res. Atmos. 125, 1–18. doi:10.1029/2019JD030711
Quadra, G. R., Sobek, S., Paranaíba, J. R., Isidorova, A., Roland, F., do Vale, R., et al. (2020). High Organic Carbon Burial but High Potential for Methane Ebullition in the Sediments of an Amazonian Hydroelectric Reservoir. Biogeosciences 17, 1495–1505.
Querino, C. A. S., Smeets, C. J. P. P., Vigano, I., Holzinger, R., Moura, V., Gatti, L. V., et al. (2011). Methane Flux, Vertical Gradient and Mixing Ratio Measurements in a Tropical Forest. Atmos. Chem. Phys. 11, 7943–7953.
Rasera, M. F. F. L., Ballester, M. V. R., Krusche, A. V., Salimon, C., Montebelo, L. A., Alin, S. R., et al. (2008). Estimating the Surface Area of Small Rivers in the Southwestern Amazon and Their Role in CO2 Outgassing. Earth Interact. 12, 1–16. doi:10.1175/2008EI257.1
Raymond, P. A., Zappa, C. J., Butman, D., Bott, T. L., Potter, J., Mulholland, P., et al. (2012). Scaling the Gas Transfer Velocity and Hydraulic Geometry in Streams and Small Rivers. Limnol. Oceanogr Fluids Environ. 2, 41–53. doi:10.1215/21573689-1597669
Renó, V. F,, Novo, E. M. L. M., Suemitsu, C., Rennó, C. D., and Silva, T. S. F. (2011). Assessment of Deforestation in the Lower Amazon Floodplain Using Historical Landsat MSS/TM Imagery. Remote Sens. Environ. 11, 3446–3456.
Ribeiro, I. O., de Souza, R. A. F., Andreoli, R. V., Kayano, M. T., and dos Santos Costa, P. (2016). Spatiotemporal Variability of Methane over the Amazon from Satellite Observations. Adv. Atmos. Sci. 33, 852–864.
Richey, J., Devol, A., Wofsy, S. C., Victoria, R., and Ribeiro, M. N. G. (1988). Biogenic Gases and the Oxidation and Reduction of Carbon in Amazon River and Floodplain Waters. Limnol. Oceanogr. 33, 551–561.
Richey, J. E., Melack, J. M., Aufdenkampe, A. K., Ballester, V. M., and Hess, L. L. (2002). Outgassing from Amazonian Rivers and Wetlands as a Large Tropical Source of Atmospheric CO2. Nature 416, 617–620. doi:10.1038/416617a
Riley, W. J., Subin, Z. M., Lawrence, D. M., Swenson, S. C., Torn, M. S., Meng, L., et al. (2011). Barriers to Predicting Changes in Global Terrestrial Methane Fluxes: Analyses Using CLM4Me, a Methane Biogeochemistry Model Integrated in. CESM. Biogeosci. Disc. 8, 1733–1807.
Ringeval, B., Houweling, S., van Bodegom, P. M., Spahni, R., van Beek, R., Joos, F., et al. (2014). Methane Emissions from Floodplains in the Amazon Basin: Challenges in Developing a Process-Based Model for Global Applications. Biogeosciences 11, 1519–1558. doi:10.5194/bg-11-1519-2014
Roland, F., Darchambeau, F., Morana, C., Crowe, S. A., Thamdrup, B., and Borges, A. V. (2016). Anaerobic Methane Oxidation in an East African Great Lake (Lake Kivu). Biogeosci. Disc., 1–27. doi:10.5194/bg-2016-300
Rosenqvist, A., Forsberg, B. R., Pimentel, T., Rauste, Y. A., and Richey, J. E. (2002). The Use of Spaceborne Radar Data to Model Inundation Patterns and Trace Gas Emissions in the Central Amazon Floodplain. Int. J. Remote Sens. 23, 1303–1328.
Rosenqvist, J., Rosenqvist, A., Jensen, K., and McDonald, K. (2020). Mapping of Maximum and Minimum Inundation Extents in the Amazon Basin 2014–2017 with ALOS-2 PALSAR-2 ScanSAR Time-Series Data. Remote Sens. 12, 1326. doi:10.3390/rs12081326
Rosentreter, J. A., Borges, A. V., Deemer, B. R., Holgerson, M. A., Liu, S., Song, C., et al. (2021). Half of Global Methane Emissions Come from Highly Variable Aquatic Ecosystem Sources. Nat. Geosci. 14, 225–230. doi:10.1038/S41561-021-00715-2
Rossetti, D. F., Gribel, R., Rennó, C. D., Cohen, M. C. L., Moulatlet, G. M., Cordeiro, C. L. de. O., et al. (2017). Late Holocene Tectonic Influence on Hydrology and Vegetation Patterns in a Northern Amazonian Megafan. Catena 158, 121–130. doi:10.1016/j.catena.2017.06.022
Rudorff, C. M., Melack, J. M., and Bates, P. M. (2014a). Flooding Dynamics on the Lower Amazon Floodplain: 1. Hydraulic Controls on Water Elevation, Inundation Extent and River-Floodplain Discharge. Water Resour. Res. 50, 619–634.
Rudorff, C. M., Melack, J. M., and Bates, P. M. (2014b). Flooding Dynamics on the Lower Amazon Floodplain: 2. Seasonal and Interannual Hydrological Variability. Water Resour. Res. 50, 635–649.
Sahagian, D., and Melack, J. M. (Editors) (1998). Global Wetland Distribution and Functional Characterization: Trace Gases and the Hydrologic Cycle. Stockholm, Sweden: IGBP Report, 46.
Saunois, M., Stavert, A. R., Poulter, B., Bousquet, P., Canadell, J. G., Jackson, R. B., et al. (2020). The Global Methane Budget: 2000-2017. Earth Syst. Sci. Data 12, 1561–1623. doi:10.5194/essd-12-1561-2020
Sawakuchi, H. O., Bastviken, D., Sawakuchi, A. O., Krusche, A. V., Ballester, M. V., and Richey, J. E. (2014). Methane Emissions from Amazonian Rivers and Their Contribution to the Global Methane Budget. Glob. Chang. Biol. 20, 2829–2840. doi:10.1111/gcb.12646
Sawakuchi, H. O., Bastviken, D., Sawakuchi, A. O., Ward, N. D., Borges, C. D., Tsai, S. M., et al. (2016). Oxidative Mitigation of Aquatic Methane Emissions in Large Amazonian Rivers. Glob. Chang. Biol. 22, 1075–1085. doi:10.1111/gcb.13169
Schubert, C. J., Diem, T., and Eugster, W. (2012). Methane Emissions from a Small Wind Shielded Lake Determined by Eddy Covariance, Flux Chambers, Anchored Funnels, and Boundary Model Calculations: A Comparison. Environ. Sci. Techn. 46, 4515–4522.
Sebacher, D. I., and Harriss, R. C. (1982). A System for Measuring Methane Fluxes from Inland and Coastal Wetland Environments. J. Environ. Qual. 11, 34–37.
Segers, A., and Houweling, S. (2020). Description of the CH4 Inversion Production Chain. Tech. Rep. Copernic. Atmos. Monit. Serv. CAMS73_2018SC1_D73.5.2.2-2019_202001_production_chain_v1.
Segers, R. (1998). Methane Production and Methane Consumption: A Review of Processes Underlying Wetland Methane Fluxes. Biogeochemistry 41, 23–51. doi:10.1023/A:1005929032764
Sihi, D., Xu, X., Ortiz, M. S., O’Connell, C. S., Silver, W. L., López-Lloreda, C., et al. (2021). Representing Methane Emissions from Wet Tropical Forest Soils Using Microbial Functional Groups Constrained by Soil Diffusivity. Biogeosciences 18, 1769–1786. doi:10.5194/bg-18-1769-2021
Silva, T. S., Melack, J. M., and Novo, E. M. (2013). Responses of Aquatic Macrophyte Cover and Productivity to Flooding Variability on the Amazon Floodplain. Glob. Chang. Biol. 19, 3379–3389. doi:10.1111/gcb.12308
Silva, T. S. F., Costa, M. P. F., and Melack, J. M. (2010). Spatio-temporal Variability of Macrophyte Cover and Productivity in the Eastern Amazon Floodplain: a Remote Sensing Approach. Remote. Sens. Environ. 114, 1998–2010.
Silva, T. S. F., Melack, J., Streher, A. S., Ferreira-Ferreira, J., and Furtado, L. F. A. (2015). “Capturing the Dynamics of Amazonian Wetlands Using Synthetic Aperture Radar: Lessons Learned and Future Directions,” in Advances in Wetland Classification and Mapping. Editors R. W. Tiner, V. Klemas, and M. W. Lang (Oxford, U.K: Taylor and Francis Publ.), 455–472.
Sippel, S. J., Hamilton, S. K., Melack, J. M., and Novo, E. M. M. (1998). Passive Microwave Observations of Inundation Area and the Area/stage Relation in the Amazon River Floodplain. Int. J. Remote Sens. 19, 3055–3074.
Sippel, S. J., Hamilton, S. K., and Melack, J. M. (1992). Inundation Area and Morphometry of Lakes on the Amazon River Floodplain, Brazil. Arch. Hydrobiol. 123, 385–400.
Sitch, S., Smith, B., Prentice, I. C., Arneth, A., Bondeau, A., Cramer, W., et al. (2003). Evaluation of Ecosystem Dynamics, Plant Geography and Terrestrial Carbon Cycling in the LPJ Dynamic Global Vegetation Model. Glob. Change Biol. 9, 161–185. doi:10.1046/j.1365-2486.2003.00569
Slater, J. A., Garvey, G., Johnston, C., Haase, J., Heady, B., Kroenung, G., et al. (2006). The SRTM Data ‘finishing’ Process and Products. Photogramm. Eng. Remote Sens. 72, 237–247. doi:10.14358/PERS.72.3.237
Smith, L. K., Melack, J. M., and Hammond, D. E. (2003). Carbon, Nitrogen and Phosphorus Content and 210Pb-Derived Burial Rates in Sediments of an Amazon Floodplain Lake. Amazoniana 17, 413–436.
Smith, L., Lewis, W., and Chanton, J. P. (2000). Methane Emissions from the Orinoco River Floodplain, Venezuela. Biogeochemistry 51, 113–140.
Sobrinho, R. L., Bernardes, M. C., Abril, G., Kim, J.-H., Zell, C. I., Mortillaro, J.-M., et al. (2016). Spatial and Seasonal Contrasts of Sedimentary Organic Matter in Floodplain Lakes of the Central Amazon Basin. Biogeosciences 13, 467–482. doi:10.5194/bg-13-467-2016
Souza do Vale, R., Silva de Santana, R. A., Silva, J. T., Miller, S. D., Ferreira de Souza, R. A., Picanço, G. A. S., et al. (2016). Eddy Covariance Measurements of Latent Heat, Sensible Heat, Momentum and CO2 Fluxes on the Reservoir of the Hydroelectric Plant Curuá-Una, PA. Ciência Nat. 38, 15–20.
Stanley, E. H., Casson, N. J., Christel, S. T., Crawford, J. T., Loken, L. C., and Oliver, S. K. (2016). The Ecology of Methane in Streams and Rivers: Patterns, Controls, and Global Significance. Ecol. Monogr. 86, 146–171.
Stepanenko, V., Mammarella, I., Ojala, A., Miettinen, H., Lykosov, V., and Vesala, T. (2016). LAKE 2.0: a Model for Temperature, Methane, Carbon Dioxide and Oxygen Dynamics in Lakes. Geosci. Model. Dev. 9, 1977–2006.
Tan, Z., Zhuang, Q., and Walter Anthony, K. (2015). Modeling Methane Emissions from Arctic Lakes: Model Development and Site‐level Study. J. Adv. Model. Earth Syst. 7, 459–483. doi:10.1002/2014MS000344
Tang, J., Zhang, Q., Shannon, R. D., and White, J. R. (2010). Quantifying Wetland Methane Emissions with Process-Based Models of Different Complexity. Biogeosciences 7, 3817–3837.
Tapley, B. D., Bettadpur, S., Watkins, M., and Reigber, C. (2004). The Gravity Recovery and Climate Experiment: Mission Overview and Early Results. Geophys. Res. Lett. 31, 1–4. doi:10.1029/2004GL019920
Teh, Y. A., Murphy, W. A., Berrio, J.-C., Boom, A., and Page, S. E. (2017). Seasonal Variability in Methane and Nitrous Oxide Fluxes from Tropical Peatlands in the Western Amazon Basin. Biogeosciences 14, 3669–3683. doi:10.5194/bg-14-3669-2017
Tunnicliffe, R. L., Ganesan, A. L., Parker, R. J., Boesch, H., Gedney, N., Poulter, B., et al. (2020). Quantifying Sources of Brazil’s CH4 Emissions between 2010 and 2018 from Satellite Data. Atmos. Chem. Phys. 20, 13041–13067.
Ulseth, A. J., Hall, R. O., Boix Canadell, M., Madinger, M. L., Niayifar, A., and Battin, T. J. (2019). Distinct Air–Water Gas Exchange Regimes in Low-And High-Energy Streams. Nat. Geosci. 12, 259–263.
van Asperen, H., Alves-Oliveira, J. R., Warneke, T., Forsberg, B., Araújo, A. C., and Notholt, J. (2021). The Role of Termite CH4 Emissions on Ecosystem Scale: a Case Study in the Amazon Rain Forest. Biogeosciences 18, 2609–2625.
van Haren, J., Brewer, P. E., Kurtzberg, L., Wehr, R. N., Springer, V. L., Espinoza, R. T., et al. (2021). A Versatile Gas Flux Chamber Reveals High Tree Stem CH4 Emissions in Amazonian Peatland. Agric. For. Meteorol. 307, 108504. doi:10.1016/j.agrformet.2021.108504
van Lent, J., Hergoualc’h, K., Verchot, L., Oenema, O., and van Groenigen, J. W. (2019). Greenhouse Gas Emissions along a Peat Swamp Forest Degradation Gradient in the Peruvian Amazon: Soil Moisture and Palm Roots Effects. Mitig. Adapt. Strateg. Glob. Change 24, 625–643. doi:10.1007/s11027-018-9796-x
Venticinque, E., Forsberg, B., Barthem, R., Petry, P., Hess, L. A., Mercado, A., et al. (2016). An Explicit GIS-Based River Basin Framework for Aquatic Ecosystem Conservation in the Amazon. Earth Syst. Sci. Data 8, 651–661.
Vihermaa, L. E., Waldron, S., Garnett, M. H., and Newton, J. (2014). Old Carbon Contributes to Aquatic Emissions of Carbon Dioxide in the Amazon. Biogeosciences 11, 3635–3645.
Villa, J. A., Ju, Y., Stephen, T., Rey-Sanchez, C., Wrighton, K. C., and Bohrer, G. (2020). Plant-mediated Methane Transport in Emergent and Floating-Leaved Species of a Temperate Freshwater Mineral-Soil Wetland. Limnol. Oceanogr. 65, 1635–1650.
Waichman, A. V. (1996). Autotrophic Carbon Sources for Heterotrophic Bacterioplankton in a Floodplain Lake of Central Amazon. Hydrobiologia 341, 27–36.
Walter, B. P., and Heimann, M. (2000). A Process-Based, Climate-Sensitive Model to Derive Methane Emissions from Natural Wetlands: Application to Five Wetland Sites, Sensitivity to Model Parameters, and Climate. Glob. Biogeochem. Cycles 14, 745–765.
Wania, R., Melton, J. R., Hodson, E. L., Poulter, B., Ringeval, B., Spahni, R., et al. (2013). Present State of Global Wetland Extent and Wetland Methane Modelling: Methodology of a Model Inter-comparison Project (WETCHIMP). Geosci. Model. Dev. 6, 617–641.
Wania, R., Ross, I., and Prentice, I. C. (2010). Implementation and Evaluation of a New Methane Model within a Dynamic Global Vegetation Model: LPJ-WHyMe V1. 3.1. Geosci. Model. Dev. 3, 565–584.
Wanninkhof, R. (1992). Relationship between Gas Exchange and Wind Speed over the Ocean. J. Geophys. Res. 97, 7373–7381.
Ward, N. D., Keil, R. G., Medeiros, P. M., Brito, D. C., Cunha, A. C., Dittmar, T., et al. (2013). Degradation of Terrestrially Derived Macromolecules in the Amazon River. Nat. Geosci. 6, 530–533. doi:10.1038/ngeo1817
Wassmann, R., Thein, U. G., Whiticar, M. J., Rennenberg, H., Seiler, W., and Junk, W. J. (1992). Methane Emissions from the Amazon Floodplain: Characterization of Production and Transport. Glob. Biogeochem. Cycles 6, 3–13.
Webb, A. J., Bösch, H., Parker, R. J., Gatti, L. V., Gloor, E., Palmer, P. I., et al. (2016). CH4 Concentrations over the Amazon from GOSAT Consistent with In Situ Vertical Profile Data. J. Geophys. Res. 121, 11006–11020. doi:10.1002/2016JD025263
Wik, M., Varner, R. K., Walter Anthony, K., MacIntyre, S., and Bastviken, D. (2016). Climate-sensitive Northern Lakes and Ponds Are Critical Components of Methane Release. Nat. Geosci. doi:10.1038/NGEO2578
Wilson, C., Chipperfield, M. P., Gloor, M., Parker, R. J., Boesch, H., McNorton, J., et al. (2021). Large and Increasing Methane Emissions from Eastern Amazonia Derived from Satellite Data, 2010-2018. Atmos. Chem. Phys. 21, 10643–10669.
Wilson, C., Gloor, M., Gatti, L. V., Miller, J. B., Monks, S. A., McNorton, J., et al. (2016). Contribution of Regional Sources to Atmospheric Methane over the Amazon Basin in 2010 and 2011. Glob. Biogeochem. Cycles 30, 400–420. doi:10.1002/2015GB005300
Wilson, M. D., Bates, P. D., Alsdorf, D., Forsberg, B., Horritt, M., Melack, J., et al. (2007). Modeling Large-Scale Inundation of Amazonian Seasonally Flooded Wetlands. Geophys. Res. Lett. 34, L15404. doi:10.1029/2007GL030156
Winton, R. S., Flanagan, N., and Richardson, C. J. (2017). Neotropical Peatland Methane Emissions along a Vegetation and Biogeochemical Gradient. PLoS One 12 (10), e0187019. doi:10.1371/journal.pone.0187019
Winton, S., Benavides, J. C., Mendoza, E., Hastie, A. T., Restrepo Canola, J. F., Ortega, A. G. H., et al. (2021). Diverse Carbon-Rich Peatlands in the Amazonia and Orinoquia of Colombia. Washington, D.C.: AGU Fall 2021 meeting. abstract B51C-07.
Yamazaki, D., Kanae, S., Kim, H., and Oki, T. (2011). A Physically Based Description of Floodplain Inundation Dynamics in a Global River Routing Model. Water Resour. Res. 47, W04501.
Yeates, P. S., and Imberger, J. (2003). Pseudo Two‐dimensional Simulations of Internal and Boundary Fluxes in Stratified Lakes and Reservoirs. Intern. J. River Basin Manage. 4, 297–319. doi:10.1080/15715124.2003.9635214
Yu, X., Millet, D. B., and Henze, D. K. (2021). How Well Can Inverse Analyses of High-Resolution Satellite Data Resolve Heterogeneous Methane Fluxes? Observing System Simulation Experiments with the GEOS-Chem Adjoint Model (V35). Geosci. Model. Dev. 14, 7775–7793. doi:10.5194/gmd-14-7775-2021
Yuan, F., Ricciuto, D. M., Xu, X., Roman, D. T., Wood, J. D., Lilleskov, E., et al. (2020). Modeling Methane Emissions in an Amazonian Palm Swamp Peatland with the E3SM Land Model. AGU Fall 2020 meeting. abstract GC009-0011.
Zhang, B., Tian, H., Lu, C., Chen, G., Pan, S., Anderson, C., et al. (2017). Methane Emissions from Global Wetlands: An Assessment of the Uncertainty Associated with Various Wetland Extent Data Sets. Atmos. Environ. 165, 310–321. doi:10.1016/j.atmosenv.2017.07.001
Zhang, Z., Zimmermann, N. E., Stenke, A., Li, X., Hodson, E. L., Zhu, G., et al. (2017). Emerging Role of Wetland Methane Emissions in Driving 21st Century Climate Change. Proc. Natl. Acad. Sci. U. S. A. 114, 9647–9652. doi:10.1073/pnas.1618765114
Zhang, Z., Zimmermann, N. E., Kaplan, J. O., and Poulter, B. (2016). Modeling Spatiotemporal Dynamics of Global Wetlands: Comprehensive Evaluation of a New Sub-grid TOPMODEL Parameterization and Uncertainties. Biogeosciences 13, 1387–1408. doi:10.5194/bg-13-1387
Zhu, Q., Liu, J., Peng, C., Chen, H., Fang, X., Jiang, H., et al. (2014). Modelling Methane Emissions from Natural Wetlands by Development and Application of the TRIPLEX-GHG Model. Geosci. Model. Dev. 7, 981–999.
Keywords: wetlands, floodplains, methane fluxes, remote sensing, inundation, modeling
Citation: Melack JM, Basso LS, Fleischmann AS, Botía S, Guo M, Zhou W, Barbosa PM, Amaral JHF and MacIntyre S (2022) Challenges Regionalizing Methane Emissions Using Aquatic Environments in the Amazon Basin as Examples. Front. Environ. Sci. 10:866082. doi: 10.3389/fenvs.2022.866082
Received: 30 January 2022; Accepted: 25 April 2022;
Published: 19 May 2022.
Edited by:
Hans-Peter Grossart, University of Potsdam, GermanyReviewed by:
Renato Campello Cordeiro, Fluminense Federal University, BrazilCopyright © 2022 Melack, Basso, Fleischmann, Botía, Guo, Zhou, Barbosa, Amaral and MacIntyre. This is an open-access article distributed under the terms of the Creative Commons Attribution License (CC BY). The use, distribution or reproduction in other forums is permitted, provided the original author(s) and the copyright owner(s) are credited and that the original publication in this journal is cited, in accordance with accepted academic practice. No use, distribution or reproduction is permitted which does not comply with these terms.
*Correspondence: John M. Melack, bWVsYWNrQGJyZW4udWNzYi5lZHU=
Disclaimer: All claims expressed in this article are solely those of the authors and do not necessarily represent those of their affiliated organizations, or those of the publisher, the editors and the reviewers. Any product that may be evaluated in this article or claim that may be made by its manufacturer is not guaranteed or endorsed by the publisher.
Research integrity at Frontiers
Learn more about the work of our research integrity team to safeguard the quality of each article we publish.