- 1Department of Epidemiology, School of Public Health, Cheeloo College of Medicine, Shandong University, Jinan, Shandong, China
- 2State Key Laboratory of Infectious Disease Prevention and Control, National Institute for Communicable Disease Control and Prevention, Chinese Center for Disease Control and Prevention, Beijing, China
- 3Institute for Nutrition and Food Hygiene, Beijing Center for Disease Prevention and Control, Beijing, China
- 4Institute of Geographical Sciences and Natural Resources Research, Chinese Academy of Sciences, Beijing, China
- 5Zhejiang Provincial Center for Disease Control and Prevention, Hangzhou, China
Background: Scrub typhus (ST) is a climate-sensitive infectious disease. However, the nonlinear relationship between important meteorological factors and ST incidence is not clear. The present study identified the quantitative relationship between ST incidence and meteorological factors in southern China.
Methods: The weekly number of ST cases and simultaneous meteorological variables in central Guangdong Province from 2006 to 2018 were obtained from the National Notifiable Infectious Disease Reporting Information System and the Meteorological Data Sharing Service System, respectively. A quasi-Poisson generalized additive model combined with a distributed lag nonlinear model (DLNM) was constructed to analyze the lag-exposure-response relationship between meteorological factors and the incidence of ST.
Results: A total of 18,415 ST cases were reported in the study area. The estimated effects of meteorological factors on ST incidence were nonlinear and exhibited obvious lag characteristics. A J-shaped nonlinear association was identified between weekly mean temperature and ST incidence. A reversed U-shaped nonlinear association was noted between weekly mean relative humidity and ST incidence. The risk of ST incidence increased when the temperature ranged from 24°C to 28°C, the relative humidity was between 78% and 82%, or the precipitation was between 50 mm and 150 mm, using the medians as references. For high temperatures (75th percentile of temperature), the highest relative risk (RR) was 1.18 (95% CI: 1.10–1.27), with a lag effect that lasted 5 weeks. High relative humidity (75th percentile of relative humidity) and high precipitation (75th percentile of precipitation) could also increase the risk of ST.
Conclusion: This study demonstrated the nonlinear relationship and the significant positive lag effects of temperature, relative humidity, and precipitation on the incidence of ST. Between particular thresholds, temperature, humidity, and levels of precipitation increased the risk of ST. These findings suggest that relevant government departments should address climate change and develop a meteorological conditions-depend strategy for ST prevention and control.
Introduction
Scrub typhus (ST) is caused by Orientia tsutsugamushi, and it is an acute febrile vector-borne infectious disease (Elliott et al., 2019). ST is transmitted by the bite of chigger mite larvae, and it is a serious public health issue in the Asia-Pacific region that threatens the health of more than one billion people in this area (Kelly et al., 2009). The World Health Organization estimates that one million cases occur annually (Kwak et al., 2015). The distribution range of ST has expanded in recent years, and the number of cases has increased (Weitzel et al., 2019; Roberts et al., 2021). However, no vaccine effectively prevents the disease (Musa et al., 2021).
China has a serious ST burden. Approximately 162 million people in southern China are at risk of infection, and more than 20,000 cases were reported in 2016 (Zheng et al., 2019; Li et al., 2020). There are two focal types of ST in China, the summer type in South China and the autumn-winter type in North China. Human infections in the summer-type foci typically occur between March and November, with a peak occurrence between June and August, and it is transmitted by the Leptotrombidium deliense mite (Li et al., 2000). The rodent hosts of this type include wild and commensal rats: Rattus losea, R. tanezumi, and R. norvegicus. Because of the active host areas, people living in urban and rural areas may be infected, and field workers in farmlands and forest areas are prone to infection. The autumn-winter type foci have different hosts, vector species and seasonal dynamics of incidence of ST. Li et al. (2020) reported the detailed demographic features of reported cases in China from 2006 to 2016. Farmers (73%) were the most affected group, with 86% of cases occurring in rural areas. In Guangzhou, the metropolis of southern China, 54.01% (2590) of the total cases from 2006 to 2014 occurred in farmers (Wei et al., 2017). These results suggested that farmers are the most sensitive population to ST.
The summer-type focus of ST is the dominant focus in China, and it is responsible for most ST cases in the country, especially in Fujian, Guangdong, Guangxi, and Yunnan Provinces (Li et al., 2019; Yue et al., 2019). Cases from these four provincial areas accounted for 65.2% of the total cases reported from 2006 to 2017, and cases from Guangdong accounted for 27.2% (Yue et al., 2019). Approximately 50% of reported cases in Guangdong province occurred in the central area (Zhaoqing, Qingyuan and Guangzhou) (Figure 1). Due to the high incidence in the summer-type foci in this region, it is necessary to identify the related factors of high incidence and find the complex mechanism to provide scientific evidence for ST prevention and control.
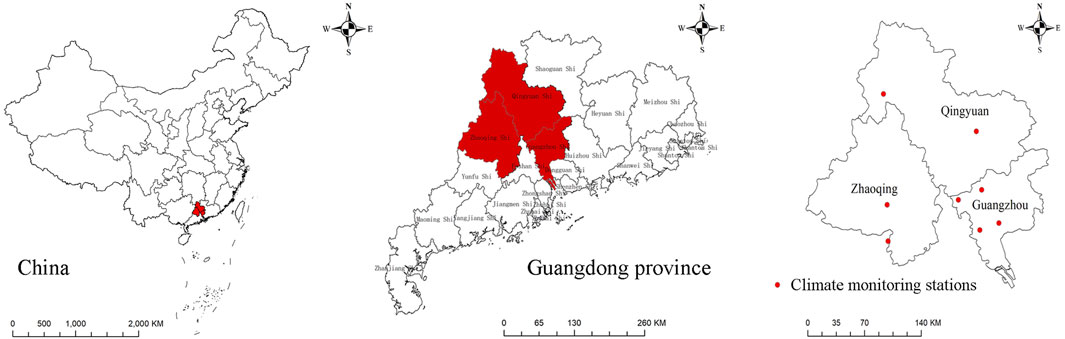
FIGURE 1. The geographic location of the central study area (in red) of Guangdong Province in China and the climate monitoring stations.
Climate change has had a great influence on infectious diseases, especially vector-borne infectious diseases (Shuman, 2010; Ogden, 2017; Booth, 2018). ST was also identified as a climate-sensitive infectious disease in many studies from different areas (Kim and Jang, 2010; Seto et al., 2017; Ranjan and Prakash, 2018). The chigger mite is an ectothermic arthropod that is highly climate-dependent (Kwak et al., 2015). In the past years, several studies (Kwak et al., 2015; Wu et al., 2016; Yu et al., 2018) found that meteorological factors, such as temperature, relative humidity, and precipitation, affected ST incidence. For instance, Wu et al. (2016) found a positive correlation between temperature, precipitation and the incidence of ST in mainland China through Poisson regression. Sun et al. (2017) used panel negative binomial regression method to find that relative humidity is an influential factor affecting the spatiotemporal distribution of ST. However, these studies are all based on the assumption of linear relationship, and the role of meteorological factors on health outcomes often has nonlinear and lag effects (Gasparrini et al., 2015; Dudney et al., 2021; Lowe et al., 2021). For example, Sun et al. (2018) found a reversed U-shaped curve between temperature and Severe fever with thrombocytopenia syndrome, with a 24-week lag effect of lower temperature. Limited studies (Wei et al., 2017; Bhopdhornangkul et al., 2021) have paid attention to the nonlinear and lag effects of meteorological factors on ST on the monthly time scale, and only one on the weekly time scale (Lu et al., 2021). However, the nonlinear and lag effect of ST were not clearly explained, nor was the specific meteorological thresholds that increase the risk of ST.
The distributed lag nonlinear model (DLNM) is used for time series data to show potentially nonlinear and delayed effects of the associations between exposure and response, especially in assessing the relationship between meteorological factors, air pollution, and health outcomes (Gasparrini et al., 2010; Gasparrini, 2011, 2014; Peng et al., 2017). Many researchers used DLNMs to explore the nonlinear relationships between meteorological factors and health outcomes, such as mortality (Wu et al., 2013), occupational diseases (Neophytou et al., 2018), and infectious diseases (Limper et al., 2016; Sun et al., 2018; Zhao et al., 2018; Lowe et al., 2021). The results of these studies improve our understanding of the epidemic mechanism of these diseases and help us develop more scientific prevention and control strategies.
The present study identified the nonlinear relationship between ST incidence and meteorological factors while accounting for lag time in central Guangdong Province in South China using DLNMs on the weekly time scale, and found the specific meteorological thresholds that increase the risk of ST. This information helps us understand the ecology of ST and provides scientific evidence for the establishment of an early warning system and prevention strategies for ST.
Methods
Study area
Guangzhou, Qingyuan, and Zhaoqing (111°21'∼114°3′E, 22°26' ∼25°11′N) cover an area of approximately 41,300 square kilometers in central Guangdong Province (Figure 1), with a population of approximately 20.31 million in 2010 and 26.83 million in 2020. This region has a subtropical monsoon climate that is warm and humid throughout the year with hot and rainy summers. The metropolis Guangzhou is located in the delta area of the Pearl River in the southern area of this region. The north and west areas are hilly areas. Seventy percent of the population in this area lives in Guangzhou and approximately 15% live in Qingyuan and Zhaoqing, respectively.
Data collection
Weekly numbers of ST cases at the county level from 2006 to 2018 were obtained from the Chinese Center for Disease Control and Prevention (China CDC) through the Chinese National Notifiable Infectious Disease Reporting Information System (CNNDS) (http://www.chinacdc.cn/). All cases, including clinical and laboratory-confirmed cases, were diagnosed according to the diagnostic criteria issued by the Chinese Center for Disease Control and Prevention (http://www.chinacdc.cn/tzgg/200901/t20090105_40316.html).
Daily meteorological data were downloaded from the China Meteorological Data Sharing Service System (http://data.cma.gov.cn/). Because temperature, humidity, and rainfall are critical factors in the development, disease transmission, and survival of chiggers (Kwak et al., 2015; Bhopdhornangkul et al., 2021), the present study primarily focused on these three variables. Weekly meteorological variables, including weekly mean temperature (Tmean, °C), weekly mean relative humidity (RH, %), and weekly cumulative precipitation (mm), were calculated based on daily meteorological data according to time. There were 8 weather monitoring stations in the study area, and we calculated averages for each meteorological variable to represent the whole level (Figure 1). After the weekly onset number of ST cases was merged with weekly meteorological data, we created the serial ST weather database and used Excel to manage the database.
Statistical analysis
Descriptive analyses were performed to describe the characteristics of ST cases and meteorological factors. Spearman correlation analysis was performed on ST cases and meteorological factors.
To determine whether there were nonlinear and lag effects of meteorological factors on ST, the present study used DLNMs combined with quasi-Poisson generalized regression to identify the associations between ST incidence and meteorological factors. Based on the application of cross-basis, a bi-dimensional function expressed by the combination of two-basis functions, DLNM simultaneously represents nonlinear exposure-response dependencies and lag effects (Gasparrini et al., 2010).
The model is expressed as follows:
where t is the week of the observation, Yt is the observed weekly count of ST cases in week t, α is the intercept, and cb(
The quasi-Akaike information criterion (QAIC) was used to determine the degrees of freedom (DF) for meteorological factors and lag. A lower QAIC value indicates an improvement in model fit (Gasparrini, 2011). The following degrees of freedom for meteorological factors and their corresponding lags were used in the final model: 4 and 4, respectively, for temperature; 4 and 4, respectively, for relative humidity; and 4 and 4, respectively, for precipitation. Based on the length of the life cycle of chigger mites (the entire cycle may take only 2–3 months and allow at least 2 generations per year) (Elliott et al., 2019), the maximum lag was selected as 25 weeks. The median values of the studied meteorological factors were used as the reference to estimate the relative risks (RRs). The relative risks of ST incidence were estimated using different meteorological factor structures relative to the reference value. Extreme low temperature, low temperature, high temperature, and extreme high temperature were defined as the 5th percentile (P5), 25th percentile (P25), 75th percentile (P75), and 95th percentile (P95) of temperature, respectively. Relative humidity and rainfall were also defined in the same way. A two-sided p value < 0.05 was considered statistically significant. All statistical analyses were performed in R software (version 3.6.2) with the ‘dlnm’ and ‘splines’ packages.
Sensitivity analysis
To assess the robustness of the model, we performed sensitivity analysis by altering the degrees of freedom of meteorological factors and the corresponding lag: Tmean (df = 3–5, lag df = 3–5), RH (df = 3–5, lag df = 3–5), and precipitation (df = 3–5, lag df = 3–5).
Ethical approval
The disease surveillance data used in this study were obtained from the NDSS with approval from the Chinese Center for Disease Control and Prevention. All patient-identifying information was removed before data analyses. The Ethical Review Committee (ERC) of the Institute for Environmental Health and Related Product Safety, Chinese CDC approved this study (NO. 201606).
Results
From 2006 to 2018, 18,415 ST cases were reported in the study area, which accounted for 12.98% of reported cases in mainland China and 47.85% of cases in Guangdong Province. The number of reported cases increased greater than 10 times, from 294 cases in 2006 to 2955 cases in 2018. A summary of weekly ST cases and meteorological variables is shown in Table 1. Figure 2 shows that ST cases and meteorological factors exhibited obvious seasonal and periodicity patterns. The disease occurs all year, primarily in the 20th - 45th weeks. Therefore, ST cases have obvious seasonal distribution characteristics, with a higher incidence in summer and autumn, and the number of ST cases showed an increasing trend (Figure 3).

TABLE 1. Summary of weekly ST cases and meteorological variables in Central Guangdong Province in China, 2006–2018.
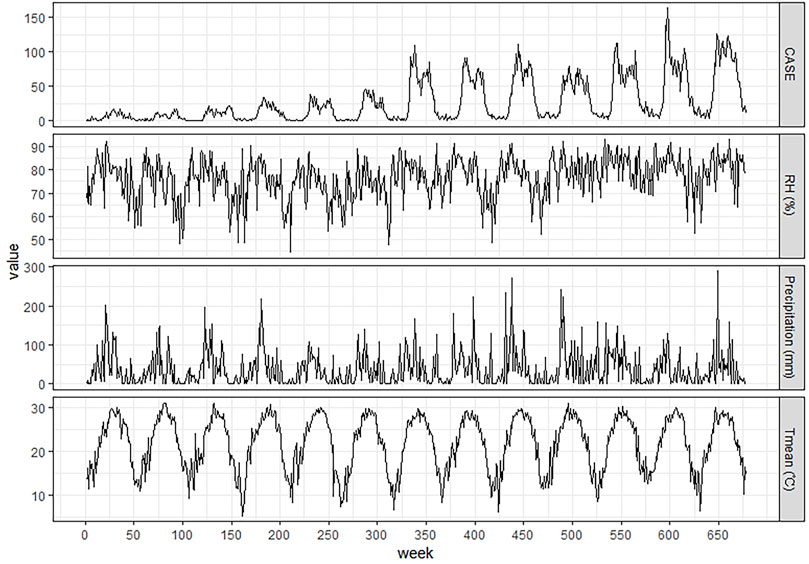
FIGURE 2. Weekly time-series distributions of ST cases and meteorological variables in Central Guangdong Province in China, 2006–2018.
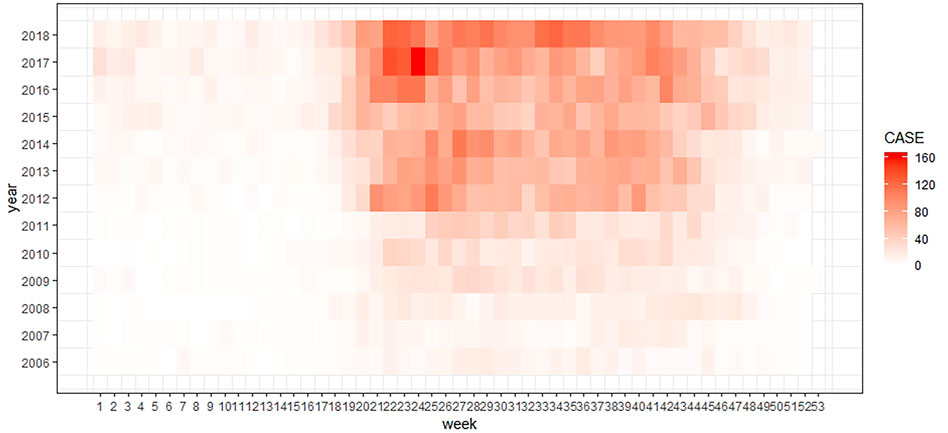
FIGURE 3. Heatmap of the weekly distribution of ST cases in Central Guangdong Province in China, 2006–2018.
The 3D plots of separate effects of weekly meteorological factors on ST incidence in each lag week and the corresponding overall relative risk plots of the relationship between them are shown in Figure 4.
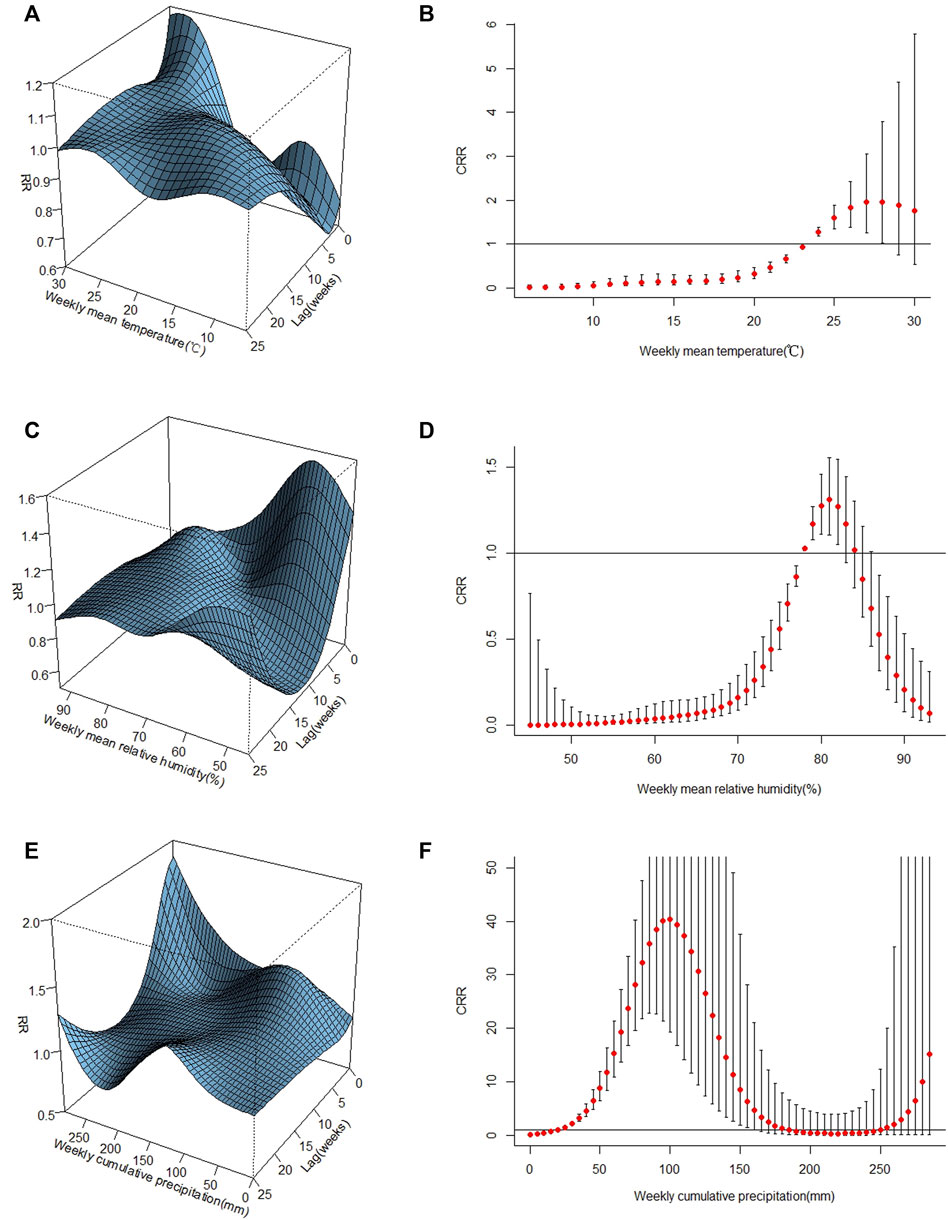
FIGURE 4. Plots of the relationships between ST and meteorological factors in Central Guangdong Province. (A,C,E) the three-dimensional plots of temperature, relative humidity, and precipitation, respectively; (B,D,F) the overall relative risk plots of temperature, relative humidity, and precipitation, respectively.
The estimated effect of weekly mean temperature and ST incidence was nonlinear, with a peak RR at the highest mean temperature by lag 0 (Figure 4A). A J-shaped nonlinear association was found between weekly mean temperature and ST incidence (Figure 4B). The cumulative risk of ST increased with increasing weekly mean temperature. When the temperature was between 24°C and 28°C, the RR and 95% CI values were greater than 1.
In contrast to the two other meteorological variables, a reversed U-shaped nonlinear association was noted between weekly mean relative humidity and ST incidence (Figures 4C,D). The risk of ST increased when the relative humidity was between 78% and 82%, with a peak relative risk of approximately 81%.
The nonlinear association and lag effects between weekly cumulative precipitation and ST incidence are illustrated in Figure 4E, and a peak RR at the highest mean precipitation by lag 0 was noted. When the weekly cumulative precipitation was between 50 mm and 150 mm, the cumulative risk increased and then decreased, with a peak at approximately 100 mm (Figure 4F).
Relative risks by lag at specific meteorological factors were estimated (Figure 5 and Supplementary Table S2) using the median values as references. Extreme low temperature and low temperature (P5, P25) decreased the risk of ST incidence, and high temperatures (P75) increased the risk with lag effects that lasted for 5 weeks. The RRs were 1.18 (95% CI: 1.10–1.27) and 1.18 (95% CI: 1.06–1.32) in P75 and P95, respectively, with the highest risk at lag 0 (Figure 5A). Lower relative humidity mainly decreased the risk of disease (P5 and P25). High relative humidity (P75) increased the RR, with a lag effect that lasted for 9 weeks, and the highest RR was 1.06 (95% CI: 1.04–1.08). However, the lag effect of relative humidity at P95 was insignificant (Figure 5B).
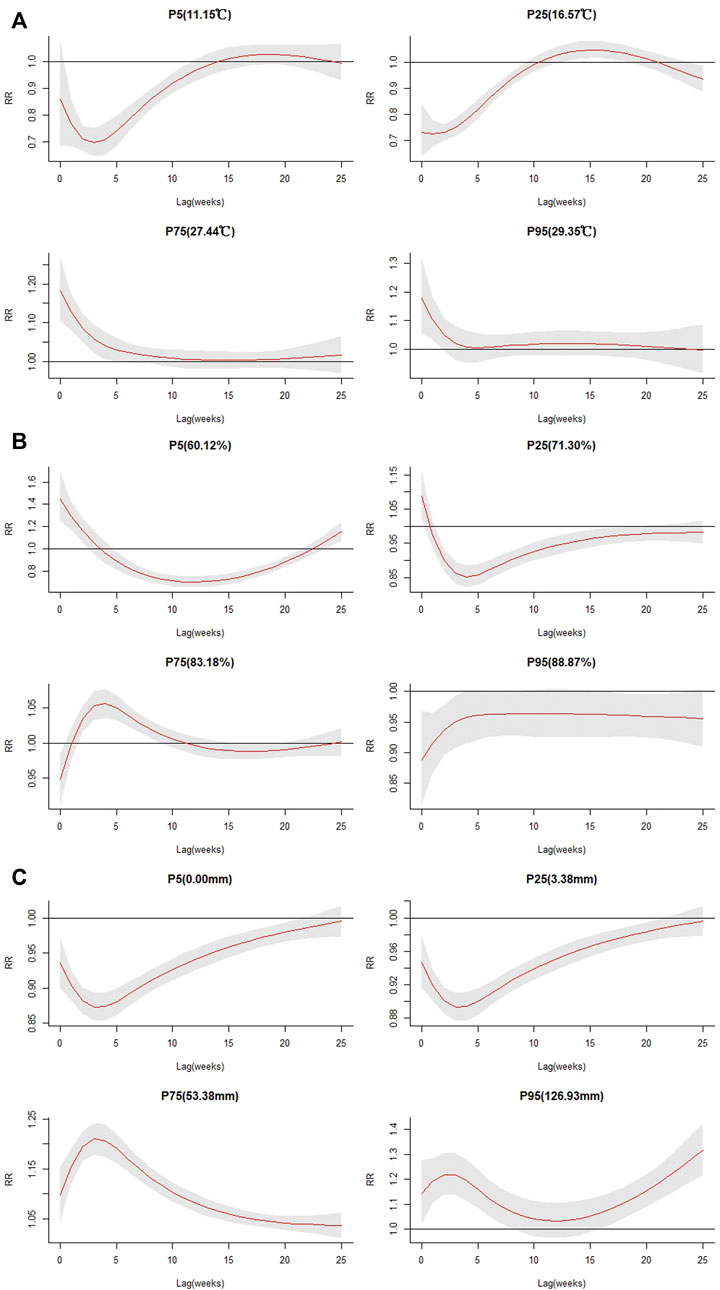
FIGURE 5. Plots of relative risk by lag for specific meteorological factors. (A) weekly mean temperature; (B) weekly mean relative humidity; (C) weekly cumulative precipitation.
Figure 5C shows that extreme low precipitation and lower precipitation (P5, P25) decreased the risk of ST incidence, and high precipitation (P75) increased the risk. In the curve of P95 of precipitation, the highest RR was 1.32 (95% CI: 1.22–1.43), with a lag effect that lasted for 8 weeks. The specific RR and 95% CI values are shown in Supplementary Table S2.
The sensitivity analysis showed that the model was relatively robust to changed degrees of freedom (Supplementary Figure S1).
Discussion
China is an important epidemic area of ST. Most ST cases were reported in South China, especially in Guangdong and Yunnan Provinces (Li et al., 2019; Yue et al., 2019). By constructing DLNMs, this study explored the nonlinear relationship and quantitative associations between meteorological factors and ST incidence in the central area of Guangdong with weekly data. A J-shaped nonlinear association was identified between weekly mean temperature and ST incidence. While a reversed U-shaped nonlinear association was noted between weekly mean relative humidity and ST incidence. The risk of ST incidence increased when the temperature ranged from 24°C to 28°C, the relative humidity was between 78% and 82%, or the precipitation was between 50 mm and 150 mm. These results demonstrated more complex relationships between meteorological factors and ST than previous researches and can help us understand the mechanism of ST epidemics.
The seasonal distribution of ST cases in this research (Figure 3) indicated that the study area was a summer-type focus. L. deliense is the main vector of ST in this type of focus, and typically causes the ST epidemic in summer. One species of wild rat, Rattus losea, and two species of commensal rat, R. tanezumi and R. norvegicus, are important host animals of ST in South China (Wu and Jiang, 2007). Therefore, people contact chigger mites in farmland, bush areas, parks, and backyards in residential areas and may be infected even in urban areas (Wei et al., 2014; Wei et al., 2017).
Climate plays an important role in the transmission of infectious disease and may affect health outcomes via various mechanisms, e.g., the density of hosts and vectors and the spread of pathogens (Patz et al., 2014). ST is a climate-sensitive infectious disease, and previous studies indicated that meteorological factors, especially temperature, precipitation and relative humidity, had positive effects on ST (Li et al., 2014; Wei et al., 2017; Zheng et al., 2019). However, human incidence does not always fluctuate immediately and linearly in response to a change in meteorological factors. This study examined the nonlinear relationship and lag effects between meteorological factors and ST incidence on a weekly time scale, which is more accurate compared to the studies on a monthly time scale (Yang et al., 2014; Sun et al., 2017; Wei et al., 2017).
The present study found a positive effect of temperature on ST incidence, which is consistent with the studies in northern China (Yang et al., 2014), in southern China (Wei et al., 2017) and in Korea (Kwak et al., 2015). Meanwhile, this study showed that the risk of ST increased when the temperature was between 24°C and 28°C, and the risk decreased when the temperature was higher than 28°C (Figure 4B). The nonlinear effect of temperature is biologically plausible. High temperature is conducive to the activity of chigger mites, such as spawning rates and the abundance and distribution of rodents (Van Peenen et al., 1976). Considering that temperature may also affect human activities and their interactions with vectors via activities, such as farming or hiking, which may also affect the occurrence of ST. The activities of humans and host animals are reduced at temperatures higher than 28°C, and the risk of exposure to chigger mites is then decreased. Besides, in this study, the results showed that low temperature (P25) could reduce the risk of ST, while high temperature (P75) increased the risk with a 5-week lag effect. Different from Wei et al. (2017) which was conducted on a monthly basis, the results of this study explained the effect of high temperature on the risk of different lag weeks, and considered that temperature is an important indicator of the risk of ST.
Relative humidity also exhibited a significant relationship with the occurrence and transmission of some vector-borne diseases (Xiang et al., 2018). A reversed U-shaped nonlinear association was noted between relative humidity and ST incidence, meanwhile the range of 78% and 82% could increase the risk of ST. This result is different from Yao et al. (2019), which found that relative humidity below 63% in foci in southern China is a risk factor for ST. The reason for the difference may be that compared with this study area, the foci in southern China are a large geographical area with diverse climate types. The interpretation of the results of this study is as follows, one possible reason is that high relative humidity provides suitable life conditions for chiggers (Clopton and Gold, 1993). Rubio and Simonetti (2009) reported that chiggers survived and thrived well at relative humidity greater than 50%, and their activity decreased when the relative humidity was less than 50%. The results of the present study further showed that the risk of the disease began to decline when the relative humidity was greater than 82%. Because days with greater than 82% humidity were mostly rainy days, people living in hilly areas preferred to stay at home and had less contact with chigger mites. What’s more, Wei et al. (2017) found that the 10% increase in relative humidity could lead to an increase in the risk of ST after a 4-month lag. And Sun et al. (2017) found that relative humidity with lags of 1 or 2 months is an influential factor affecting the spatiotemporal distribution of ST. While this study showed that higher relative humidity (P75) increased the RR with the lag effect lasting for 9 weeks. The analysis of the lag effect in weeks of the present study can more accurately reflect the effect of relative humidity on ST.
Precipitation is also an important factor that significantly affects the incidence of ST and other rodent-borne diseases (Li et al., 2014; Chang et al., 2019). The nonlinear relationship between precipitation and ST incidence was reported in some previous studies (Wei et al., 2017; Wangrangsimakul et al., 2020). However, the results of the present research were more complex than the studies mentioned above. When the weekly cumulative precipitation was between 30 mm and 150 mm, the cumulative risk increased and then decreased, with a peak at approximately 100 mm (Figure 4F). Several mechanisms may explain this complex nonlinear association. First, precipitation may increase the growth of vegetation, which directly or indirectly makes survival and reproduction easier for rodents and causes a high rodent density (Ernest et al., 2000). Second, appropriate rainfall is good for the activity and survival of chigger mites. A study from Thailand showed that rainfall positively influenced the activity of L. deliense, with more chiggers mites found on sentinel rodents in the wetter season, and the infection rate of O. tsutsugamushi was greater during the same season (Frances et al., 1999).
When cumulative weekly rainfall ranged from 150 to 250 mm, the relative risk decreased (Figure 4F). This kind of relationship was also reported by Lu et al. (2021), which analyzed weekly data of Guangzhou using the DLNM. While Li et al. (2014) and Wei et al. (2017) reported a simple positive relationship between rainfall and the risk of ST in Guangzhou with monthly data. Because Wei et al. (2017) also used DLNM in the data analysis, it suggested that the different relationship between precipitation and ST risk may be related to the partitioning method of data, the setting of parameters or other reasons. The reason for this result may be that when the rainfall exceeds a certain level, people’s outdoor activities such as field work and park exercise will be reduced, and the chigger mite contact with people will be greatly reduced, and the risk of disease will be reduced.
A scientific understanding of the nonlinear relationship between meteorological factors and the incidence of ST will help us accurately evaluate the impact of climate change on ST. Combined with the results of this study, it can be concluded that meteorological factors are important influences on the ST epidemic, and the effects are complex. These results suggest that relevant government authorities should strengthen the prevention and control of ST according to the meteorological conditions and the trend of climate change. These findings also suggest that rodent and mite breeding sites should be controlled during warm and humid seasons. In addition, public health education about the relationship between meteorological factors and ST, and some response measures should be enhanced for sensitive groups of ST. Due to the lag effect of high temperature, the response measures should last for at least 5 weeks. Furthermore, some studies reported that economic development and land-use change also affected ST epidemics (Wardrop et al., 2013; Ranjan and Prakash, 2018; Shah et al., 2019). Therefore, more research on the influencing factors of ST should be performed to elucidate the complex epidemic mechanism of ST and develop scientific intervention and prevention strategies.
Several limitations should be mentioned. First, the ST data were downloaded from the CNNDS, which is a passive disease surveillance system. Underreporting is inevitable due to problematic access to medical resources, mild symptoms, and a lack of help from the hospital. Second, ST incidence may be affected by complex interactions between humans, vector and host density, other meteorological factors, socioeconomic factors, and urbanization level, which were not considered in the model construction. Our results may not be appropriate for other regions with different climate zones.
Conclusion
The present study demonstrated the nonlinear relationship and significant lag effects of temperature, relative humidity, and precipitation on the incidence of ST in the central area of Guangdong Province. Between particular thresholds, temperature, relative humidity, and precipitation were positively related to the risk of ST. However, the relationships were negative outside of the thresholds. These results improve our understanding of the mechanisms of the ST epidemic under the influence of meteorological factors. Therefore, accurate intervention and prevention strategies and protocols may be developed accordingly.
Data availability statement
The data analyzed in this study is subject to the following licenses/restrictions: the dataset were obtained from the Chinese Center for Disease Control and Prevention (China CDC), further inquiries can be directed to the corresponding author. Requests to access these datasets should be directed to LL, luliang@icdc.cn.
Author contributions
WL: Conceptualization, Formal analysis, Validation, Writing—original draft. YN: Methodology, Writing—review and editing. HR: Methodology, Software. WS: Methodology, Software. WM: Writing—review and editing. XL: Writing—review and editing. GL: Writing—review and editing. JW: Resources. QL: Supervision. LL: Supervision, Funding acquisition, Project administration.
Funding
This study was supported by the Special Foundation of Basic Science and Technology Resources Survey of Ministry of Science and Technology, China (grant No. 2017FY101202).
Acknowledgments
We thank the staff members of the various CDCs and the National Meteorological Information Center of China for their valuable assistance in collecting the data used in this study.
Conflict of interest
The authors declare that the research was conducted in the absence of any commercial or financial relationships that could be construed as a potential conflict of interest.
The reviewer QL declared an affiliation with several of the authors YL, XL, GL, JW, QL, and LL to the handling editor. Handling editor declared personal affiliation with author HR.
Publisher’s note
All claims expressed in this article are solely those of the authors and do not necessarily represent those of their affiliated organizations, or those of the publisher, the editors and the reviewers. Any product that may be evaluated in this article, or claim that may be made by its manufacturer, is not guaranteed or endorsed by the publisher.
Supplementary material
The Supplementary Material for this article can be found online at: https://www.frontiersin.org/articles/10.3389/fenvs.2022.849681/full#supplementary-material
References
Bhopdhornangkul, B., Meeyai, A. C., Wongwit, W., Limpanont, Y., Iamsirithaworn, S., Laosiritaworn, Y., et al. (2021). Non-linear effect of different humidity types on scrub typhus occurrence in endemic provinces, Thailand. Heliyon 7 (2), e06095. doi:10.1016/j.heliyon.2021.e06095
Booth, M. (2018). Climate change and the neglected tropical diseases. Adv. Parasitol. 100, 39–126. doi:10.1016/bs.apar.2018.02.001
Chang, Y. C., Kuo, K. C., Sun, W., Lin, J. N., Lai, C. H., and Lee, C. H. (2019). Clinicoepidemiologic characteristics of scrub typhus and murine typhus: A multi-center study in southern taiwan. J. Microbiol. Immunol. Infect. 52 (5), 769–778. doi:10.1016/j.jmii.2019.04.004
Clopton, R. E., and Gold, R. E. (1993). Distribution and seasonal and diurnal activity patterns of Eutrombicula alfreddugesi (Acari: Trombiculidae) in a forest edge ecosystem. J. Med. Entomol. 30 (1), 47–53. doi:10.1093/jmedent/30.1.47
Dudney, J., Willing, C. E., Das, A. J., Latimer, A. M., Nesmith, J. C. B., and Battles, J. J. (2021). Nonlinear shifts in infectious rust disease due to climate change. Nat. Commun. 12 (1), 5102. doi:10.1038/s41467-021-25182-6
Elliott, I., Pearson, I., Dahal, P., Thomas, N. V., Roberts, T., and Newton, P. N. (2019). Scrub typhus ecology: A systematic review of Orientia in vectors and hosts. Parasit. Vectors 12 (1), 513. doi:10.1186/s13071-019-3751-x
Ernest, S. K. M., Brown, J. H., and Parmenter, R. R. (2000). Rodents, plants, and precipitation: Spatial and temporal dynamics of consumers and resources. Oikos 88 (3), 470–482. doi:10.1034/j.1600-0706.2000.880302.x
Frances, S. P., Watcharapichat, P., Phulsuksombati, D., Tanskul, P., and Linthicum, K. J. (1999). Seasonal occurrence of Leptotrombidium deliense (Acari: Trombiculidae) attached to sentinel rodents in an orchard near Bangkok, Thailand. J. Med. Entomol. 36 (6), 869–874. doi:10.1093/jmedent/36.6.869
Gasparrini, A., Armstrong, B., and Kenward, M. G. (2010). Distributed lag non-linear models. Stat. Med. 29 (21), 2224–2234. doi:10.1002/sim.3940
Gasparrini, A. (2011). Distributed lag linear and non-linear models in R: The package dlnm. J. Stat. Softw. 43 (8), 1–20. doi:10.18637/jss.v043.i08
Gasparrini, A., Guo, Y., Hashizume, M., Lavigne, E., Zanobetti, A., Schwartz, J., et al. (2015). Mortality risk attributable to high and low ambient temperature: A multicountry observational study. Lancet 386 (9991), 369–375. doi:10.1016/s0140-6736(14)62114-0
Gasparrini, A. (2014). Modeling exposure-lag-response associations with distributed lag non-linear models. Stat. Med. 33 (5), 881–899. doi:10.1002/sim.5963
Kelly, D. J., Fuerst, P. A., Ching, W. M., and Richards, A. L. (2009). Scrub typhus: The geographic distribution of phenotypic and genotypic variants of Orientia tsutsugamushi. Clin. Infect. Dis. 48 (3), S203–S230. doi:10.1086/596576
Kim, S. H., and Jang, J. Y. (2010). Correlations between climate change-related infectious diseases and meteorological factors in Korea. J. Prev. Med. Public Health 43 (5), 436–444. doi:10.3961/jpmph.2010.43.5.436
Kwak, J., Kim, S., Kim, G., Singh, V. P., Hong, S., and Kim, H. S. (2015). Scrub typhus incidence modeling with meteorological factors in South Korea. Int. J. Environ. Res. Public Health 12 (7), 7254–7273. doi:10.3390/ijerph120707254
Li, G., Wang, Y., Yue, Y., Xu, L., Mu, Q., Zhao, N., et al. (2019). Division of epidemic areas for summer- and autumn-type scrub typhus in China. Chin J Vector Biol Control 30 (3), 233–236. doi:10.11853/j.issn.1003.8280.2019.03.001
Li, J., Zheng, X., and XI, Z. (2000). Review on the studies of chigger mites and scrub typhus in China. Chin. J. Public Health (09), 1–3.
Li, T., Yang, Z., Dong, Z., and Wang, M. (2014). Meteorological factors and risk of scrub typhus in Guangzhou, southern China, 2006-2012. BMC Infect. Dis. 14, 139. doi:10.1186/1471-2334-14-139
Li, Z., Xin, H., Sun, J., Lai, S., Zeng, L., Zheng, C., et al. (2020). Epidemiologic changes of scrub typhus in China, 1952-2016. Emerg. Infect. Dis. 26 (6), 1091–1101. doi:10.3201/eid2606.191168
Limper, M., Thai, K. T., Gerstenbluth, I., Osterhaus, A. D., Duits, A. J., and van Gorp, E. C. (2016). Climate factors as important determinants of dengue incidence in curaçao. Zoonoses Public Health 63 (2), 129–137. doi:10.1111/zph.12213
Lowe, R., Lee, S. A., O'Reilly, K. M., Brady, O. J., Bastos, L., Carrasco-Escobar, G., et al. (2021). Combined effects of hydrometeorological hazards and urbanisation on dengue risk in Brazil: A spatiotemporal modelling study. Lancet Planet. Health 5 (4), e209–e219. doi:10.1016/s2542-5196(20)30292-8
Lu, J., Liu, Y., Ma, X., Li, M., and Yang, Z. (2021). Impact of meteorological factors and southern oscillation index on scrub typhus incidence in Guangzhou, southern China, 2006-2018. Front. Med. 8, 667549. doi:10.3389/fmed.2021.667549
Musa, T. H., Ahmad, T., Wana, M. N., Li, W., Musa, H. H., Sharun, K., et al. (2021). The epidemiology, diagnosis and management of scrub typhus disease in China. Hum. Vaccin. Immunother. 17 (10), 3795–3805. doi:10.1080/21645515.2021.1934355
Neophytou, A. M., Picciotto, S., Brown, D. M., Gallagher, L. E., Checkoway, H., Eisen, E. A., et al. (2018). Exposure-lag-response in longitudinal studies: Application of distributed-lag nonlinear models in an occupational cohort. Am. J. Epidemiol. 187 (7), 1539–1548. doi:10.1093/aje/kwy019
Ogden, N. H. (2017). Climate change and vector-borne diseases of public health significance. FEMS Microbiol. Lett. 364 (19), 186. doi:10.1093/femsle/fnx186
Patz, J. A., Frumkin, H., Holloway, T., Vimont, D. J., and Haines, A. (2014). Climate change: Challenges and opportunities for global health. Jama 312 (15), 1565–1580. doi:10.1001/jama.2014.13186
Peng, Z., Wang, Q., Kan, H., Chen, R., and Wang, W. (2017). Effects of ambient temperature on daily hospital admissions for mental disorders in shanghai, China: A time-series analysis. Sci. Total Environ. 590-591, 281–286. doi:10.1016/j.scitotenv.2017.02.237
Ranjan, J., and Prakash, J. A. J. (2018). Scrub typhus re-emergence in India: Contributing factors and way forward. Med. Hypotheses 115, 61–64. doi:10.1016/j.mehy.2018.03.019
Roberts, T., Parker, D. M., Bulterys, P. L., Rattanavong, S., Elliott, I., Phommasone, K., et al. (2021). A spatio-temporal analysis of scrub typhus and murine typhus in Laos; implications from changing landscapes and climate. PLoS Negl. Trop. Dis. 15 (8), e0009685. doi:10.1371/journal.pntd.0009685
Rubio, A. V., and Simonetti, J. A. (2009). Ectoparasitism by Eutrombicula alfreddugesi larvae (Acari: Trombiculidae) on Liolaemus tenuis lizard in a Chilean fragmented temperate forest. J. Parasitol. (Faisalabad). 95 (1), 244–245. doi:10.1645/ge-1463.1
Seto, J., Suzuki, Y., Nakao, R., Otani, K., Yahagi, K., and Mizuta, K. (2017). Meteorological factors affecting scrub typhus occurrence: A retrospective study of yamagata prefecture, Japan, 1984-2014. Epidemiol. Infect. 145 (3), 462–470. doi:10.1017/S0950268816002430
Shah, H. A., Huxley, P., Elmes, J., and Murray, K. A. (2019). Agricultural land-uses consistently exacerbate infectious disease risks in Southeast Asia. Nat. Commun. 10 (1), 4299. doi:10.1038/s41467-019-12333-z
Shuman, E. K. (2010). Global climate change and infectious diseases. N. Engl. J. Med. Overseas. Ed. 362 (12), 1061–1063. doi:10.1056/NEJMp0912931
Sun, J., Lu, L., Yang, J., Liu, K., Wu, H., and Liu, Q. (2018). Association between Severe fever with thrombocytopenia syndrome incidence and ambient temperature. Am. J. Trop. Med. Hyg. 98 (5), 1478–1483. doi:10.4269/ajtmh.17-0991
Sun, Y., Wei, Y. H., Yang, Y., Ma, Y., de Vlas, S. J., Yao, H. W., et al. (2017). Rapid increase of scrub typhus incidence in Guangzhou, southern China, 2006-2014. BMC Infect. Dis. 17 (1), 13. doi:10.1186/s12879-016-2153-3
Van Peenen, P. F., Lien, J. C., Santana, F. J., and See, R. (1976). Correlation of chigger abundance with temperature at a hyperendemic focus of scrub typhus. J. Parasitol. 62 (4), 653–654. doi:10.2307/3279442
Wangrangsimakul, T., Elliott, I., Nedsuwan, S., Kumlert, R., Hinjoy, S., Chaisiri, K., et al. (2020). The estimated burden of scrub typhus in Thailand from national surveillance data (2003-2018). PLoS Negl. Trop. Dis. 14 (4), e0008233. doi:10.1371/journal.pntd.0008233
Wardrop, N. A., Kuo, C. C., Wang, H. C., Clements, A. C., Lee, P. F., and Atkinson, P. M. (2013). Bayesian spatial modelling and the significance of agricultural land use to scrub typhus infection in Taiwan. Geospat. Health 8 (1), 229–239. doi:10.4081/gh.2013.69
Wei, Y., Huang, Y., Li, X., Ma, Y., Tao, X., Wu, X., et al. (2017). Climate variability, animal reservoir and transmission of scrub typhus in Southern China. PLoS Negl. Trop. Dis. 11 (3), e0005447. doi:10.1371/journal.pntd.0005447
Wei, Y., Luo, L., Jing, Q., Li, X., Huang, Y., Xiao, X., et al. (2014). A city park as a potential epidemic site of scrub typhus: A case-control study of an outbreak in Guangzhou, China. Parasit. Vectors 7, 513. doi:10.1186/s13071-014-0513-7
Weitzel, T., Martinez-Valdebenito, C., Acosta-Jamett, G., Jiang, J., Richards, A. L., and Abarca, K. (2019). Scrub typhus in continental Chile, 2016–20181. Emerg. Infect. Dis. 25 (6), 1214–1217. doi:10.3201/eid2506.181860
Wu, G., and Jiang, Z. (2007). Prevention and control of tsutsugamushi disease. Int. J. Med. Parasit. Dis. 34 (4), 169. doi:10.3760/cma.j.issn.1673-4122.2007.04.001
Wu, W., Xiao, Y., Li, G., Zeng, W., Lin, H., Rutherford, S., et al. (2013). Temperature-mortality relationship in four subtropical Chinese cities: A time-series study using a distributed lag non-linear model. Sci. Total Environ. 449, 355–362. doi:10.1016/j.scitotenv.2013.01.090
Wu, Y. C., Qian, Q., Soares Magalhaes, R. J., Han, Z. H., Hu, W. B., Haque, U., et al. (2016). Spatiotemporal dynamics of scrub typhus transmission in mainland China, 2006-2014. PLoS Negl. Trop. Dis. 10 (8), e0004875. doi:10.1371/journal.pntd.0004875
Xiang, J., Hansen, A., Liu, Q., Tong, M. X., Liu, X., Sun, Y., et al. (2018). Impact of meteorological factors on hemorrhagic fever with renal syndrome in 19 cities in China, 2005-2014. Sci. Total Environ. 636, 1249–1256. doi:10.1016/j.scitotenv.2018.04.407
Yang, L. P., Liu, J., Wang, X. J., Ma, W., Jia, C. X., and Jiang, B. F. (2014). Effects of meteorological factors on scrub typhus in a temperate region of China. Epidemiol. Infect. 142 (10), 2217–2226. doi:10.1017/s0950268813003208
Yao, H., Wang, Y., Mi, X., Sun, Y., Liu, K., Li, X., et al. (2019). The scrub typhus in mainland China: Spatiotemporal expansion and risk prediction underpinned by complex factors. Emerg. Microbes Infect. 8 (1), 909–919. doi:10.1080/22221751.2019.1631719
Yu, H., Sun, C., Liu, W., Li, Z., Tan, Z., Wang, X., et al. (2018). Scrub typhus in jiangsu province, China: Epidemiologic features and spatial risk analysis. BMC Infect. Dis. 18 (1), 372. doi:10.1186/s12879-018-3271-x
Yue, Y., Ren, D., Liu, X., Wang, Y., Liu, Q., and Li, G. (2019). Spatio-temporal patterns of scrub typhus in mainland China, 2006-2017. PLoS Negl. Trop. Dis. 13 (12), e0007916. doi:10.1371/journal.pntd.0007916
Zhao, Q., Li, S., Cao, W., Liu, D. L., Qian, Q., Ren, H., et al. (2018). Modeling the present and future incidence of pediatric hand, foot, and mouth disease associated with ambient temperature in mainland China. Environ. Health Perspect. 126 (4), 047010. doi:10.1289/ehp3062
Keywords: scrub typhus, meteorological factors, distributed lag nonlinear model, guangdong province, lagged effect
Citation: Li W, Niu Y, Ren H, Sun W, Ma W, Liu X, Li G, Wang J, Liu Q and Lu L (2022) Climate-driven scrub typhus incidence dynamics in South China: A time-series study. Front. Environ. Sci. 10:849681. doi: 10.3389/fenvs.2022.849681
Received: 06 January 2022; Accepted: 12 October 2022;
Published: 21 October 2022.
Edited by:
Peng Bi, University of Adelaide, AustraliaReviewed by:
Meghnath Dhimal, Nepal Health Research Council, NepalQun Li, Chinese Center For Disease Control and Prevention, China
Ramesh C. Dhiman, National Institute of Malaria Research (ICMR), India
Copyright © 2022 Li, Niu, Ren, Sun, Ma, Liu, Li, Wang, Liu and Lu. This is an open-access article distributed under the terms of the Creative Commons Attribution License (CC BY). The use, distribution or reproduction in other forums is permitted, provided the original author(s) and the copyright owner(s) are credited and that the original publication in this journal is cited, in accordance with accepted academic practice. No use, distribution or reproduction is permitted which does not comply with these terms.
*Correspondence: Liang Lu, bHVsaWFuZ0BpY2RjLmNu