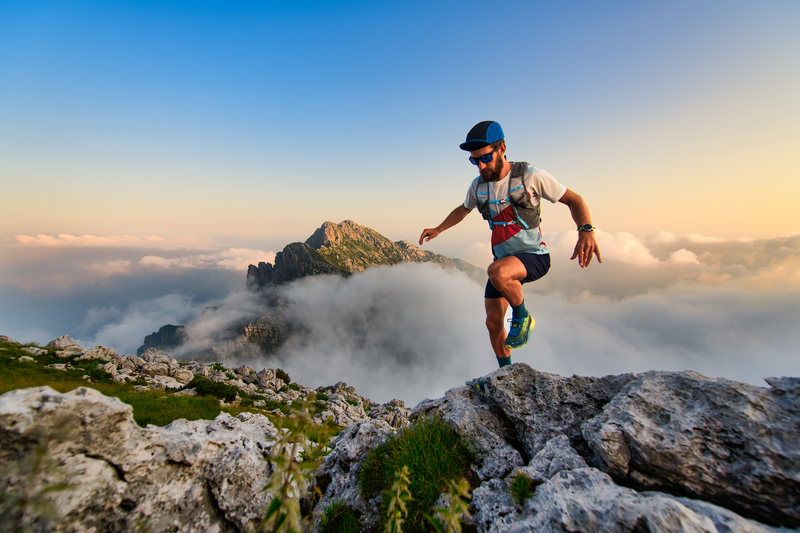
94% of researchers rate our articles as excellent or good
Learn more about the work of our research integrity team to safeguard the quality of each article we publish.
Find out more
ORIGINAL RESEARCH article
Front. Environ. Sci. , 08 February 2022
Sec. Toxicology, Pollution and the Environment
Volume 10 - 2022 | https://doi.org/10.3389/fenvs.2022.814678
Heavy metal contamination in lakes caused by the rapid industrialization and urbanization is a serious problem. In this study, 12 heavy metals were systematically surveyed in aquatic environment and organisms of Dianchi Lake. Results showed that heavy metals pollutions in surface water exhibited a decreasing order of Ba > Fe > Zn > Mn > As > Ni > Cr > Cu > Pb > Cd > Co, equipped a consistency in spatial distribution, seriously contaminating the northern and southern parts. The average concentration of sedimentary heavy metals appeared in an order of Fe > Mn > Zn > Ba > Cu > Pb > Cr > As > Ni > Co > Cd > Ag. The main existing fraction (51.9–75.0%) of Cu, Pb, Cr, As, Fe, Co, Ni, Ag, and Ba in sediments was residual fraction, whereas the exchangeable fraction (40.9–62.0%) was the dominant component for Cd, Zn, and Mn. Among the selected aquatic organisms, Cu, Pb, Zn, and Ag possessed a strong bioaccumulation effect, followed by Mn, Fe, Co, and Ni. Ecological risk assessment indicated that Cu, Cr, and Zn were the dominant heavy metal contaminants in surface water; Cd presented the disastrous risk and accounted for the considerable proportion of ecological risk in sediments. Human health risk evaluation showed that the selected aquatic products of Dianchi Lake were not absolutely safe, and As was the major contributor. This study systematically revealed heavy metal distributions in aquatic environments, which was conductive to environmental safety and human health.
Heavy metal pollution in aquatic ecosystem has become a serious environmental problem in the world because of its potential toxicity and accumulation in organisms (Peng et al., 2009; Tang et al., 2010; Fu et al., 2013). These contaminants in aquatic environment not only generate direct toxic effects on aquatic organisms, but also bring potential threats to human health through the domestic water and food chain (Järup, 2003; Liu et al., 2018). In addition, heavy metals tend to accumulate in sediments and become the internal source of water pollution (Bradl, 2004; Zhang et al., 2007). After resuspension, heavy metals in sediments can be released into surface water again, thereby causing secondary pollution (Kelderman and Osman, 2007; Baran and Tarnawski, 2015). Therefore, systematically studying the distribution of heavy metals in surface water, sediments, and organisms is necessary for contamination control and environmental management. Moreover, identifying the pollution source will provide an important reference for ecosystem restoration and remediation (Wang J.-H. et al., 2019).
The monitoring, risk assessment, and prevention of heavy metal pollution have been widely concerned for several decades (Kumar et al., 2019). Long-term heavy metal pollution is regarded to disrupt the aquatic ecological balance and cause serious adverse effects on aquatic ecosystem (Jaiswal et al., 2018). In freshwater ecosystem, numerous natural and anthropogenic sources contribute to the heavy metal pollution, including direct atmospheric precipitation, geological process, and discharge of abundant human activities (Saha and Paul, 2018). For urban and suburban lakes, these environmental problems usually become more prominent because of the intensive human impact when compared with remote lakes (Cheng et al., 2015). With the rapid development of industrialization and urbanization in China, suburban and urban lakes have received considerable pollutants impacted by human activities and suffered ecological deterioration (Li et al., 2020; Qian et al., 2020). Previous literature indicated that the major rivers and lakes in China had been generally polluted by heavy metals at different levels, with the sedimentary pollution proportion over 80% (Wang et al., 2010). Hence, focusing on the pollution level and bioaccumulation of heavy metals in urban and suburban lakes is important to compare the contribution of rapid economic development to heavy metal pollution with remote plain lakes (Wei and Wen, 2012; Fu et al., 2014). Although heavy metal investigation and risk assessment in Chinese lakes have been reported, the comprehensive heavy metal evaluation of surface water, sediments, and organisms in a typical urban plateau lake is still limited (Tang et al., 2010; Fu et al., 2013; Cheng et al., 2015). When a large number of these contaminants are transported into the aquatic ecosystem, the bioaccumulation of metals and biomagnification of the food chain may cause a series of environmental problems, such as ecosystem degradation and public health risks (Altindag and Yigit, 2005; Xia et al., 2019). However, few research reports have focused on the bioaccumulation of heavy metals, their interaction with environmental parameters, and the transmission of metals from edible aquatic organisms to humans (Yi et al., 2011; Fu et al., 2014).
Dianchi Lake, located in the southwest of China near Kunming City, is the largest freshwater plateau lake in China with an altitude of 1886.5 m above sea level (Figure 1). In general, the lake provides water for agriculture, industry, drinking, and other activities. However, since the last century, rapid industrialization and urbanization in the watershed had contributed a serious eco-environmental problem in and around the lake (Guo et al., 2017). The lake receives numerous contaminants from its connected rivers and suffers from serious anthropogenic pollution, gradually evolving into a eutrophic lake (Wang B. et al., 2019). Reports indicated that the eutrophication of Dianchi Lake was closely correlated to human activities (Cao et al., 2016). In recent years, the heavy metal evaluation in Dianchi Lake has also been carried out (Li et al., 2020). However, previous studies have only focused on the concentrations of heavy metals in sediments, and their pollution levels in multiple environmental media and source identifications are lacking, particularly for several trace elements such as Co, Ag, Ba, and Ni (Kumar et al., 2019; Qian et al., 2020). These less concerned heavy metals are also closely related to certain human health diseases (Yi et al., 2011; Li et al., 2014). Meanwhile, several aquatic species in Dianchi Lake are generally important food resources, and their human health risks must be considered. Chinese white prawn, Macrobrachium nipponense, Hemisalanx prognathus Regan, and Rhinogobius giurinus are four of the major commercial aquatic products consumed frequently by local residents. Although several studies had investigated heavy metals in fish community, only few of them addressed the transportation of metals in aquatic environments to the high trophic level and result in potential health risks through the food chain (Yang et al., 2017; Qian et al., 2020). Hence, comprehensive investigation of various heavy metals in Dianchi Lake ecosystem is of great significance for pollutant control and restoration. Furthermore, human health and ecological risk assessment of heavy metals in surface water and sediments, associating with bioaccumulation, will provide valuable basic information and important management strategies.
FIGURE 1. Location of Lake Dianchi and sampling sites in the study area: S1–S5 in Caohai of Lake Dianchi, S6–S10 in Northern Waihai, S11–S15 in Central Waihai, and S16–S19 in Southern Waihai.
In the present study, distributions, risks, and source identifications of 12 heavy metals were focused on, including Cu, Cd, Cr, As, Pb, Zn, Mn, Fe, Co, Ag, Ba, and Ni. In particular, the species sensitivity distribution (SSD) model was used to evaluate the risk of heavy metal pollution in surface water; the geoaccumulation index (Igeo) and potential ecological risk index (RI) were selected to assess the sedimentary risk, and the bioaccumulation factor (BAF) was applied to illustrate the impact of heavy metals on organisms. The main purposes of this study included four aspects: 1) to systematically investigate the concentration of heavy metals in surface water, sediments, and organisms; 2) to identify the pollution source of heavy metals in Dianchi Lake; 3) to assess the ecological and human health risks of these contaminants in different media; and 4) to provide guidance for pollutant management and aquatic production consumption in Dianchi Lake.
The Dianchi Lake (N24°40′-25°02′, E102°02′-102°47′), covering approximately 298 km2 of water area and 2,920 km2 of watershed area, is the largest freshwater lake in the Yunnan–Guizhou Plateau of Southwest China. The lake is separated into two parts by artificial water conservancy facilities (Figure 1). The northern part (Caohai) is adjacent to Kunming City, having only 3% of the total lake area and an average depth of 2.5 m. The southern part (Waihai) accounts for the most part of the lake area, having an average depth of 4.4 m. The climate of the Dianchi Lake is characterized by subtropical southwest monsoon, with an annual mean temperature of 14.4°C and an average precipitation of 1,000 mm (Wang et al., 2018). The hydraulic retention time of surface water in the Dianchi Lake is approximately 2.7 years, which limits the self-purification capacity of the lake (Li et al., 2020). Since the last century, rapid population and economic growth in this area have resulted in a serious eco-environmental problem, such as eutrophication and heavy metal pollution (Ma and Wang, 2015).
The locations of sampling samples in the lake were presented in Figure 1 and Supplementary Table S1. All samples were collected from the Dianchi Lake in July 2014. Organism samples, including shrimps and fishes, were stochastically obtained according to the actual situation (Supplementary Table S8). Specifically, shrimps include C. Prawn (CWP) and M. Nipponense (MBN), and fish includes h. Prognathus Regan (HPR) and R. Giurinus (RGG). Mixed water samples from three depths (∼0.5, ∼1.5, and ∼2.5 m above the bottom) were collected. Surface sediment samples (0–10 cm) were obtained using a Peterson dredge. Organism samples were obtained by a trawl. The above-mentioned collected samples were stored in a −4°C freezer until laboratory analysis.
Twelve heavy metals, including Cu, Cd, Cr, As, Pb, Zn, Mn, Fe, Co, Ag, Ba, and Ni, were measured in surface water, sediments, and organism samples. Four different forms of heavy metals in sediments were extracted, which were classified into residual, oxidizable, reducible, and exchangeable. The detailed sedimentary fractionation procedure was presented in Supplementary Table S2. Before heavy metal analysis, sediment and organism samples were pretreated according to previous reports (Fu et al., 2013; Xing et al., 2013). All samples were treated using microwave digestion and analyzed by inductively coupled plasma mass spectrometry.
Electrical conductivity (EC), dissolved oxygen (DO), water temperature (WT), pH, suspended solid (SS), total dissolved solid (TDS), oxidation–reduction potential (ORP), and chlorophyll a (Chla) were determined in situ using EXO2 detector (YSI, United States). Other environmental parameters, including total nitrogen (TN), nitrate nitrogen (NO3−-N), nitrite nitrogen (NO2−-N), ammonium nitrogen (NH4+-N), activated phosphorous (PO43−-P), total phosphorus (TP), and chemical oxygen demand (CODMn), were measured according to the standard methods in the laboratory (APHA, 1998). The moisture content of sediments was measured by drying at 105°C, and sedimentary organic matter (SOM) was determined by calcination at 550°C.
Pearson correlation analysis was performed to study the relationship among heavy metals. Principal component analysis (PCA) was used to identify the significant clusters and potential sources of heavy metals. A geostatistical approach called inverse distance weighting was applied to evaluate the distribution of heavy metals in unsampled areas and generate the spatial map. These statistical procedures for heavy metals in surface water and sediments were similar to our previous works (Wang et al., 2017a; Liu et al., 2020). All data in this study were analyzed by SPSS 19.0, Origin 8.0, and ArcGIS 10.4.
The bioaccumulation factor (BAF) has been widely applied to quantify the bioaccumulation of environmental pollutants in previous studies (Hao et al., 2019). In this study, the BAF illustrated the impact of heavy metal concentrations in surface water on aquatic organisms (Ahmed et al., 2019). In general, BAF is the radio between heavy metal concentrations in the organisms and those in their main living environment (Qiu, 2015; Zhang et al., 2015a). Therefore, BAFs for each freshwater organism sample and selected heavy metals were calculated with the following formula Eq. 1:
where Corganism is the heavy metal concentration in freshwater organisms (mg/Kg), and Cfreshwater is the concentration of heavy metals in a freshwater (μg/L) or sediment (mg/Kg) medium. BAF-freshwater can be categorized according to the following ranges: BAF <1 indicates low probability of accumulation; 1 < BAF < 5 indicates moderate, and BAF >5 indicates highly bio-accumulative (Arnot and Gobas, 2006). As for BAF-sediment, the calculated value >1 indicates a potential accumulation of heavy metals, and the accumulative effect makes a significant difference when the BAF-sediment exceeds 100 (Zhang et al., 2015b).
The ecological risks of selected heavy metals in surface water were evaluated using SSD, which were introduced in detail in our previous study (Liu et al., 2018). In recent years, the SSD method has been widely used in risk assessment because of its simplicity and specific ecological significance (Xu et al., 2015). This risk assessment model has two important indicators: the potentially affected fraction (PAF) and concentration with 5% cumulative probability (HC5). The fundamental principle is illustrated in Supplementary Figure S1.
The RI, initially proposed by a Swedish scientist in 1980, has been proven to be an effective method and widely used to evaluate sedimentary pollution (Hakanson, 1980; Zhao et al., 2018). In this evaluation model, the toxicity characteristic, contaminant level, and background value of heavy metals were considered. The RI value was calculated using the following Eq. 2:
where
According to previous literature, the high heavy metal background value in this study area might overestimate the adverse effect of metals when using the RI and hazard quotient (HQ) models (Qian et al., 2020). Therefore, the Igeo was selected to assess the risks of heavy metals in sediments, whose calculation formula Eq. 3 was as follows:
where Cn is the measured concentration of each heavy metal in sediment samples; Bn is the geochemical background concentration of the corresponding metal in this study area. The soil evolvement and its influence on the eco-environment were important, representing the various geochemical processes in this area (Yuan et al., 2014). Therefore, the mean concentration of heavy metals in local soils was used as the background value (Bn) for sediments. According to the soil element background value investigation performed by China National Environmental Monitoring Center, the Bn values were identified as 46.3 mg/kg for Cu, 0.218 mg/kg for Cd, 65.2 mg/kg for Cr, 18.4 mg/kg for As, 40.6 mg/kg for Pb, 89.7 mg/kg for Zn, 626 mg/kg for Mn, 52,200 mg/kg for Fe, 17.5 mg/kg for Co, 0.152 mg/kg for Ag, 346 mg/kg for Ba, and 42.5 mg/kg for Ni. Constant term 1.5 was the background matrix correction factor originated by lithospheric effects (Reddy et al., 2004). Based on the Igeo, the degree of risk is divided into seven levels: Igeo ≤0 (practically unpolluted), 0 < Igeo <1 (unpolluted to moderately polluted), 1 < Igeo <2 (moderately polluted), 2 < Igeo <3 (moderately to heavily polluted), 3 < Igeo <4 (heavily polluted), 4 < Igeo <5 (heavily to extremely polluted), and Igeo >5 (extremely polluted; Bhuiyan et al., 2010).
Human health risk assessment is the approach of estimating contaminant adverse effects on humans through aquatic products, and target hazard quotients (THQ) is regarded as an effective evaluation model (USEPA, 2014; Qian et al., 2020). Fishery and shrimp resources are important food resources for local residents around Dianchi Lake; therefore, evaluating the potential health risk related to their long-term consumptions is important (Guo et al., 2017; Wang et al., 2019). In general, no significant health risk is found if THQ is less than 1, but a potential health risk will occur if the index is greater than 1. The THQ value was obtained by the following formula Eq. 4 (Yi et al., 2011; Qian et al., 2020):
where THQ is the target hazard quotient; EFr is the exposure frequency (365 days/year); EDt is the exposure duration (70 years, average lifetime); FIR is the food ingestion rate (134 g/day, wet weight); Cfactor is the conversion factor (0.085) that is used to convert fresh weight into dry weight; C is the heavy metal concentration in fish (mg/Kg); RfDo is the oral reference dose (mg/kg/day, Supplementary Table S11); BWa is the average adult body weight (60 kg); and ATn is the average exposure time for non-carcinogens (assuming 70 years). Total THQ (TTHQ) was calculated to estimate the additive effects of exposure to all the metals accumulated in fish using Eq. 5:
The surface waters were weakly alkaline, with mean pH of 9.3 (ranging from 7.9 to 10.0). During the sampling period, the average WT and DO were 23.9°C and 9.77 mg/L, respectively. The EC, TDS, SS, and CODMn values ranged from 503 to 647 μS/cm (average 540 μS/cm), 331.5–435.5 mg/L (average 358.9 mg/L), 29–176 mg/L (average 84 mg/L), and 6.0–27.3 mg/L (average 15.8 mg/L), respectively. The mean concentrations of TN and TP were 4.62 and 0.21 mg/L, with the maximum of 9.56 and 0.56 mg/L, respectively. These typical water quality parameters suggested that the lake has suffered serious eutrophic pollution and algae bloom (Supplementary Table S3; Qian et al., 2020). As shown in Supplementary Table S4, the sediments in Dianchi Lake were reductive, with the mean pH and ORP values of 6.9 and −209.5 mV, respectively. The average SOM was 16.0%, ranging from 10.0 to 41.1%. Given the long-term eutrophication and weakly hydrodynamic processes of Dianchi Lake, a large number of nutrients had been enriched in sediments (Zhu et al., 2010). The mean concentration of sedimentary TN and TP was 5,626 and 3,584 mg/kg, respectively. According to the U.S. Environmental Protection Agency, sediment was regarded as heavily polluted when sedimentary TN > 2000 mg/kg and TP > 650 mg/kg (USEPA, 2014). Important phosphorus industrial bases were found in China around Dianchi Lake, which might indicate the high phosphorus content in sediments (Zhu et al., 2010). However, the mean concentration of TN and TP in sediments of Taihu Lake (a eutrophic lake in China) was only 1,110 and 930 mg/kg, respectively (Fang et al., 2019). Therefore, based on the water and sediment quality characteristics of Dianchi Lake and previous literature, Dianchi Lake was generally regarded as a typical hyper-eutrophic lake, which was suffering from the deterioration of the ecological environment (Huang et al., 2014; Cao et al., 2016).
The pollution level of heavy metals in surface water exhibited a wide range, and the average concentration was arranged in a decreasing order: Ba (average 171.73 μg/L) > Fe (average 146.70 μg/L) > Zn (average 20.64 μg/L) > Mn (average 4.32 μg/L) > As (average 2.78 μg/L) > Ni (average 2.05 μg/L) > Cr (average 1.54 μg/L) > Cu (average 1.36 μg/L) > Pb (average 0.54 μg/L) > Cd (average 0.22 μg/L) > Co (average 0.13 μg/L, Figure 2 and S3). Nearly all heavy metals equipped a great consistency in spatial distribution, seriously contaminated in the north and south part but less polluted in the middle part (Figure 2). This differential spatial distribution might be due to the following reasons: the northern part was connected with Kunming City, and the southern part was densely distributed with residential communities. In general, the city and high-density population could remarkably contribute to the heavy metal pollution (Islam et al., 2015). By contrast, for example, the average Cu concentration in Dianchi Lake (1.4 μg/L) was lower than that in Poyang Lake (5.4 μg/L), Taihu Lake (2.9 μg/L), and Chaohu Lake (3.4 μg/L) but slightly higher than that in Liangzi Lake (1.1 μg/L). The Pb pollution level in this lake was lower than that in Poyang Lake, Taihu Lake, Chaohu Lake, and Liangzi Lake, whose average concentrations were 4.4, 3.8, 6.3, and 10.1 μg/L, respectively (Liu et al., 2018). The different metal pollution levels in various lakes of China were probably due to the different physical geography backgrounds and human activity impacts, thereby suggesting that the systematic investigation of metal pollution levels in different lakes was important.
FIGURE 2. Spatial variations of heavy metal concentration in surface water, including Cu, Cd, Pb, Zn, Cr, As, Mn, Fe, Co, Ni, and Ba. Ag was not presented because of its low concentration in surface water.
The average concentration of sedimentary heavy metals appeared in a decreasing order: Fe (average 50,720.35 mg/kg) > Mn (average 813.03 mg/kg) > Zn (average 496.80 mg/kg) > Ba (average 273.35 mg/kg) > Cu (average 146.19 mg/kg) > Pb (average 108.83 mg/kg) > Cr (average 74.78 mg/kg) > As (average 61.95 mg/kg) > Ni (average 45.81 mg/kg) > Co (average 15.24 mg/kg) > Cd (average 13.20 mg/kg) > Ag (average 2.06 mg/kg). Concentrations of Cu, Cd, Pb, Zn, As, Ni, and Ag in sediments had a similar spatial distribution. Remarkably, a decreasing trend was found from the northern (S1–S4) to the southern (S5–S12) part of Dianchi Lake (p < 0.05; Figure 3). As for concentrations of Cr, Mn, Fe, Co, and Ba in sediments, their spatial distributions were basically consistent, varying within a limited range (Figure 3). Fe was the most abundant metal in the sediment, exceeding the pollution level of other metals. According to previous literature and available data, several heavy metals were selected for comparison with published metal levels in Chinese lake sediments (Supplementary Table S14). In this study, the mean concentration of heavy metals was consistent with the earlier report in Dianchi Lake (Yuan et al., 2014). Interestingly, contrary to that of surface water, the heavy metal concentration in sediments of Dianchi Lake was significantly higher than that of Chaohu Lake and Taihu Lake. For example, the concentrations of Cu and Pb in sediments of Dianchi Lake were about 8.5 times and 73.3 times higher than that of Chaohu Lake, respectively. This phenomenon was probably due to the following reasons. First, Dianchi Lake had higher density and biomass of algae compared with the other lakes, and the algae biomass could easily uptake or adsorb metals from water (De Philippis et al., 2011; Wang et al., 2019). Second, the average depth of Dianchi Lake (approximately 5.0 m) was deeper than that of Taihu Lake (approximately 1.8 m) and Chaohu Lake (approximately 3.0 m) (Shang and Shang, 2007; Xu et al., 2010; Zhang et al., 2019). The deeper water depth in Dianchi Lake could resist sediment re-suspension by wind wave, which could reduce the metal release from sediments (Gao et al., 2005; Bai et al., 2012).
FIGURE 3. Heavy metal concentrations in sediment samples. Blue: residual fraction; Green: oxidizable fraction; Red: reducible fraction; Black: exchangeable fraction.
The characteristic of heavy metal fractions was important to reveal their potential mobility and toxicity (Maiz et al., 2000). The main existing fraction of Cu, Pb, Cr, As, Fe, Co, Ni, Ag, and Ba in sediments was residual fraction, with values of 60.95, 57.06, 66.56, 61.98, 75.03, 39.36, 51.90, 91.94, and 63.41%, respectively (Figure 3 and Supplementary Figure S2). For Cd, Zn, and Mn, the exchangeable fraction was the dominant component, accounting for 62.05, 49.08, and 40.94%, respectively (Figure 3 and Supplementary Figure S2). Based on the bioavailability of heavy metals, a decreasing order was found: exchangeable > oxidizable > reducible > residual (Li et al., 2000; Prasad et al., 2006). The exchangeable fraction was generally regarded as the most unstable sedimentary part, which exhibited a strong relationship with water environments (Alves et al., 2007). Therefore, the environmental mobility and risk of heavy metals showed a positive correlation with this fraction proportion. In this study, the exchangeable fraction of heavy metals was arranged in the following order: Cd (62.5%) > Zn (49.08%) > Mn (40.94%) > CO (21.82%) > Ni (20.52%) > Ba (5.35%) > Cr (4.27%) > Cu (3.51%) > As (3.40%) > Fe (3.30%) > Pb (2.05%) > Ag (0.09%), indicating their different interactions with environments. This result suggested that Cd, Zn, Mn, Co, and Ni had the strongest association with the aquatic ecosystem in Dianchi Lake and likely reflected highly potential risks.
CWP, MBN, HPR, and RGG were selected to evaluate metal bioaccumulation because they were usually consumed by the local residents. Heavy metal levels in selected organisms showed great differences (Supplementary Table S9), and the concentration of heavy metals in selected fish (HPR and RGG) was significantly lower (p < 0.05) than that in surveyed shrimp (CWP and MBN). These results indicated that the ability of benthic shrimp to accumulate heavy metals was stronger than that of fish, which was consistent with previous studies (De Mora et al., 2004; Yang et al., 2010). Firstly, benthic shrimps mainly live in the sediment-water interface, which probably straightly affected by the heavy metals in the sediment (2-3 orders of magnitude higher than that in the water body). Secondly, metal concentrations in organisms were also adjusted by their biological metabolisms (Markert, 1987).
In the present study, the BAF of organisms to heavy metal in surface water (BAF-water) was further explored (Figure 4 and Supplementary Table S10). Different organisms exhibited distinct bio-accumulative capacities in response to various heavy metals. In our study, a high accumulative possibility of Ag in HPR; Cu and Ag in CWP; Cu, Pb, Zn, and Ag in MBN; and Pb, Zn, and Ag in RGG was found. Notably, Cd, Cr, As, and Ba presented a low accumulative probability for all selected organisms. Moreover, Mn, Fe, Co, and Ni showed a moderate accumulative probability for at least one species. Hence, based on the probability heatmap between organisms and heavy metals (Figure 4), we found that Cu, Pb, Zn, and Ag possessed a strong bioaccumulation effect, followed by Mn, Fe, Co, and Ni. In general, Cu and Zn were regarded as a crucial biological trace element and demanded for abundant enzymatic oxidation–reduction activities; however, excessive levels of these two metals could also cause high toxicity (Bonanno and Giudice, 2010; Wang et al., 2017b; Wei et al., 2020). Pb and Ag were immobile in aquatic environment, and they showed toxicity. When Pb and Ag were absorbed by organisms through the food chain, they would exist for a long period (Samecka and Kempers, 2001).
FIGURE 4. Bioaccumulation factor of organisms to heavy metals in surface water (BAF-water). Green: low probability; Blue: moderate probability; Red: high probability.
PCA and CA were applied to identify and explain the pollution source of heavy metals (Figure 5 and Supplementary Figure S4). In surface water, two principal components were extracted, which accounted for 62% of the total variance in the data matrix. The first principal component (PCA1) in surface water generated 37% of the total variance, which was primarily characterized by heavy metals. Among the heavy metals, Ag, Zn, Co, Fe, Mn, Cr, Cu, Cd, and Pb were the most important, followed by Ni and As, and Ba was relatively small. The second principal component (PCA2) accounted for 25.36% of the total variance, which was heavily weighted by conventional water quality parameters. Our results indicated that the pollution sources of heavy metals and eutrophic elements in surface water were probably inconsistent because of three reasons. First, heavy metals were strictly controlled in the effluent of sewage treatment plant when compared with organic contaminants (Ignatowicz, 2017). Second, heavy metals induced by non-point source pollution tended to be precipitated under the long-distance water transport, whereas nutrients were gradually accumulated (Ouyang et al., 2016; Jeong et al., 2020). Lastly, heavy metals cannot be easily degraded, and the sedimentary resuspension would lead to their release, whereas the conventional pollutants were biodegradable (Baran and Tarnawski, 2015). In sediments, two principal components were extracted, which accounted for 69% of the total variance in the data matrix. The first principal component (PCA1), including Ag, Zn, Cd, Pb, Cu, As, and Ni, was primarily characterized by most heavy metals. However, the second principal component (PCA2) was completely dominated by Fe, Mn, and Ba, whereas Cr and Co remarkably contributed to both axes. In particular, PCA1 and PCA2 accounted for 47 and 22%, respectively. PCA1 probably originated from anthropogenic activities, as Cu and Pb were strongly associated with human activities (Audry et al., 2004). However, PCA2 was heavily weighted by Fe and Mn, which might be closely related to the surrounding mining areas (Zhao et al., 2018).
In our previous study on the SSD model, five heavy metals were selected to evaluate their ecological risks in Dianchi Lake because of their occurrence and toxicity. These metals with HC5 values of 7.76 (Cd), 2.29 (Cr), 2.09 (Cu), 12.59 (Pb), and 31.62 (Zn) posed great toxicity to aquatic environments (Supplementary Table S7; Liu et al., 2018). In general, these metals were considered to be at risks only when their concentrations exceeded individual HC5 values (Hose and Van den Brink, 2004). The maximum concentration of Cu, Cr, Cd, Pb, and Zn in Dianchi Lake was 4.06, 3.17, 0.71, 2.25, and 74.49 μg/L, respectively. Therefore, Cu, Cr, and Zn exhibited ecological risks with the maximum PAF of 14, 8, and 13%, respectively (Supplementary Table S7). Therefore, about 14% of species in Dianchi Lake were probably adversely impacted by Cu, whereas 8% by Cr and 13% by Zn. Therefore, based on the SSD model, Cu, Cr, and Zn were the dominant heavy metal contaminants in surface water of Dianchi Lake. These heavy metals should be strictly controlled to ensure the health of aquatic organisms in Dianchi Lake.
The Igeo was applied to evaluate sedimentary metal contamination in Dianchi Lake, and the calculated result was presented in Figure 6B. The mean values of Igeo for Cr, Mn, Fe, Co, Ni, and Ba were lower than 0, suggesting that no pollution was caused by these metals. However, the average of Cu, Pb, and As ranged from 0 to 1, indicating unpolluted to moderately polluted. Cd, Zn, and Ag showed heavily polluted (average Igeo 3.24), moderately polluted (average Igeo 1.08), and moderately to heavily polluted (average Igeo 2.81), respectively. Regarding the individual Igeo value, the pollution status in sediments followed the order of Cd > Ag > Zn > As > Cu > Pb > Mn > Cr > Ni > Fe > Co > Ba (Figure 6B). The result of this methodology indicated that Cd, Zn, and Ag were the most polluted metals, which was consistent with previous literature in this area (Qian et al., 2020). Hence, the anthropogenic inputs of heavy metals in this area were Cd, Zn, and Ag, with Igeo >1.
FIGURE 6. Risk assessment for selected heavy metals in sediments: (A) RI of selected heavy metals in sediments. As: brownness; Cr: pink; Zn: green; Pb: blue; Cd: red; Cu: black. (B) Igeo of selected heavy metals in sediments. The box plots display the values from surface. water samples (median, 25 and 75% quartiles [boxes], 10 and 90% percentiles [whiskers]).
In addition, the calculated RI values of sedimentary metals were summarized in Figure 6A and Supplementary Table S13. The RI values from S1 to S4 (Caohai, closely related to Kunming city) were more than 900, indicating that these sediments in Caohai were heavily polluted, which showed more disastrous risks than other areas. In general, the RI value was clearly related to the degree of anthropogenic disturbance (Yi et al., 2011). About 45% of sediment samples (S1, S2, S3, S4, S6, S7, S8, S15, and S17) were disastrous risks (RI > 300), and the remaining 55% (S5, S9, S10, S11, S12, S13, S14, S16, S18, and S19) showed very high risks (200 < RI < 300). In particular, the contributions for individual metals were in the order of Cd (94.6%) > Cr (1.8%) = As (1.8%) > Cu (0.8%) > Pb (0.7%) > Zn (0.3%). Remarkably, Cd presented disastrous risks and accounted for the considerable proportion of ecological risks in sediments, whereas other metals (As, Zn, Cr, Cu, and Pb) showed low-to-moderate risks. Sediments in Dianchi Lake, particularly Northern Caohai, suffered from potential ecological risks caused by heavy metals, and Cd was the most important contaminant element. Based on the RI and Igeo, heavy metals in sediments of Dianchi Lake exhibited high ecological risks.
Based on the bioaccumulation of heavy metals (Figure 4), the investigated organisms in Dianchi Lake exhibited different accumulation effects on these contaminants. Long-term consumption of these polluted aquatic productions might cause human health risks (Kumar et al., 2019). Therefore, the human health threat from four common edible organisms was evaluated for residents around the lake. Given the absence of Fe and Co human chronic ingestion data, the remaining 10 heavy metals were used in human health risk assessment. The calculated THQ and total THQ (TTHQ) values were presented in Supplementary Table S12 and Figure 7. Human health risks of selected aquatic products decreased in the order of MBN (1.998) > CWP (1.450) > RGG (1.213) > HPR (0.355), and THQ of As was the major contributor to TTHQ. This study was consistent with previous reports in Dianchi Lake, in which As in aquatic products showed the most significant health risk (Qian et al., 2020). Except for the THQ of As in CWP (1.119) and MBN (1.187), the THQ of other metals to each aquatic consumption was generally less than 1, indicating that residents would not experience significant health risks from the intake of individual metal through selected organisms (Supplementary Table S12). Therefore, human health risks induced by As were found in the consumption of CWP, MBN, and RGG. Considering the impact of heavy metal pollution on human health, this study revealed that HPR was a priority of healthy food resource in Dianchi Lake. The heavy metals in the environment had various chemical forms that exhibited different toxicity to human health, and the THQ >1 might not suggest people who were experiencing direct adverse health effects (Yi et al., 2011; Jia et al., 2018). In our future work, evaluating human health risks of metals by considering chemical speciation was necessary.
FIGURE 7. Contributions of THQ to TTHQ for the 10 selected heavy metals via consumption of four aquatic species collected from Dianchi Lake. Red dash line indicated the acceptable total THQ threshold value (<1).
The distribution, ecological risk, and source identification of heavy metals in surface water, sediments, and organisms of Dianchi Lake had been systematically investigated. The pollution level of heavy metals exhibited a wide range in surface water, with a decreasing order of Ba > Fe > Zn > Mn > As > Ni > Cr > Cu > Pb > Cd > Co. Nearly all heavy metals in surface water equipped a great consistency in spatial distribution, seriously contaminating the northern and southern parts. We found that the residual and exchangeable fractions of heavy metals were primarily presented in sediments. The primary existing fraction of Cu, Pb, Cr, As, Fe, Co, Ni, Ag, and Ba was residual fraction, whereas the exchangeable fraction was the dominant component for Cd, Zn, and Mn, which suggested that Cd, Zn, Mn, Co, and Ni had the strongest association with the aquatic ecosystem in Dianchi Lake. Furthermore, the average concentration of sedimentary heavy metals appeared in a decreasing order: Fe > Mn > Zn > Ba > Cu > Pb > Cr > As > Ni > Co > Cd > Ag. We found that Cu, Pb, Zn, and Ag possessed a strong bioaccumulation effect, followed by Mn, Fe, Co, and Ni. Ecological risk assessment indicated that Cu, Cr, and Zn were the dominant heavy metal contaminants in surface water, whereas Cd and Ag were the most polluted metals in sediments. As in selected aquatic products had the most significant health risk, and HPR was a priority of healthy food resource for residents. In addition, this study was based on one sampling. Thus, the heavy metal pollution may be influenced by seasons. However, given the relatively stable nature of heavy metals and the period of exchange water cycle (4 years) of this lake, the spatial distribution of the heavy metals of sediment shows no significant change with the seasons. In conclusion, this research was of great significant to reveal the migration, transformation and enrichment of heavy metals in a typical urban plateau lake, and provided effective information for rational management and control of heavy metal pollution.
The original contributions presented in the study are included in the article/Supplementary Material, further inquiries can be directed to the corresponding author.
XL: Software, Data analysis, Writing—original draft. JZ: Investigation, Project administration, Writing—review and editing. XH: Writing—review and editing. LZ: Software, Writing—review and editing. CY: Writing—review and editing. EL: Investigation, Funding acquisition, Writing—review and editing. ZW: Investigation, Project administration, Supervision, Funding acquisition, Writing—eview and editing.
This work was supported by the Youth Innovation Promotion Association, Chinese of Academy of Sciences (2018369), Key Project of Hubei Province Natural Science Foundation (2020CFA110) and National Natural Science Foundation of China (NSFC: 41601545; 41671512).
The authors declare that the research was conducted in the absence of any commercial or financial relationships that could be construed as a potential conflict of interest.
All claims expressed in this article are solely those of the authors and do not necessarily represent those of their affiliated organizations, or those of the publisher, the editors and the reviewers. Any product that may be evaluated in this article, or claim that may be made by its manufacturer, is not guaranteed or endorsed by the publisher.
The Supplementary Material for this article can be found online at: https://www.frontiersin.org/articles/10.3389/fenvs.2022.814678/full#supplementary-material
Ahmed, A. S. S., Rahman, M., Sultana, S., Babu, S. M. O. F., and Sarker, M. S. I. (2019). Bioaccumulation and Heavy Metal Concentration in Tissues of Some Commercial Fishes from the Meghna River Estuary in Bangladesh and Human Health Implications. Mar. Pollut. Bull. 145, 436–447. doi:10.1016/j.marpolbul.2019.06.035
Altindag, A., and Yigit, S. (2005). Assessment of Heavy Metal Concentrations in the Food Web of lake Beysehir, Turkey. Chemosphere 60, 552–556. doi:10.1016/j.chemosphere.2005.01.009
Alves, J. P. H., Passos, E. A., and Garcia, C. A. B. (2007). Metals and Acid Volatile Sulfide in Sediment Cores from the Sergipe River Estuary, Northeast, Brazil. J. Braz. Chem. Soc. 18, 758. doi:10.1590/s0103-50532007000400013
APHA (1998). Standard Methods for the Examination of Water and Wastewater. 20th edn. Washington, DC: American Public Health Association.
Arnot, J. A., and Gobas, F. A. (2006). A Review of Bioconcentration Factor (BCF) and Bioaccumulation Factor (BAF) Assessments for Organic Chemicals in Aquatic Organisms. Environ. Rev. 14, 257–297. doi:10.1139/a06-005
Audry, S., Schäfer, J., Blanc, G., and Jouanneau, J.-M. (2004). Fifty-year Sedimentary Record of Heavy Metal Pollution (Cd, Zn, Cu, Pb) in the Lot River Reservoirs (France). Environ. Pollut. 132, 413–426. doi:10.1016/j.envpol.2004.05.025
Bai, Y., Shi, Q., Wen, D., Li, Z., Jefferson, W. A., Feng, C., et al. (2012). Bacterial Communities in the Sediments of Dianchi Lake, a Partitioned Eutrophic Waterbody in China. PloS one 7, e37796. doi:10.1371/journal.pone.0037796
Baran, A., and Tarnawski, M. (2015). Assessment of Heavy Metals Mobility and Toxicity in Contaminated Sediments by Sequential Extraction and a Battery of Bioassays. Ecotoxicology 24, 1279–1293. doi:10.1007/s10646-015-1499-4
Bhuiyan, M. A. H., Parvez, L., Islam, M. A., Dampare, S. B., and Suzuki, S. (2010). Heavy Metal Pollution of Coal Mine-Affected Agricultural Soils in the Northern Part of Bangladesh. J. Hazard. Mater. 173, 384–392. doi:10.1016/j.jhazmat.2009.08.085
Bonanno, G., and Lo Giudice, R. (2010). Heavy Metal Bioaccumulation by the Organs of Phragmites Australis (Common Reed) and Their Potential Use as Contamination Indicators. Ecol. Indicators 10, 639–645. doi:10.1016/j.ecolind.2009.11.002
Bradl, H. B. (2004). Adsorption of Heavy Metal Ions on Soils and Soils Constituents. J. Colloid Interf. Sci. 277, 1–18. doi:10.1016/j.jcis.2004.04.005
Cao, X., Wang, Y., He, J., Luo, X., and Zheng, Z. (2016). Phosphorus Mobility Among Sediments, Water and Cyanobacteria Enhanced by Cyanobacteria Blooms in Eutrophic Lake Dianchi. Environ. Pollut. 219, 580–587. doi:10.1016/j.envpol.2016.06.017
Cheng, H., Li, M., Zhao, C., Yang, K., Li, K., Peng, M., et al. (2015). Concentrations of Toxic Metals and Ecological Risk Assessment for Sediments of Major Freshwater Lakes in China. J. Geochemical Exploration 157, 15–26. doi:10.1016/j.gexplo.2015.05.010
De Mora, S., Fowler, S. W., Wyse, E., and Azemard, S. (2004). Distribution of Heavy Metals in marine Bivalves, Fish and Coastal Sediments in the Gulf and Gulf of Oman. Mar. Pollut. Bull. 49, 410–424. doi:10.1016/j.marpolbul.2004.02.029
De Philippis, R., Colica, G., and Micheletti, E. (2011). Exopolysaccharide-producing Cyanobacteria in Heavy Metal Removal from Water: Molecular Basis and Practical Applicability of the Biosorption Process. Appl. Microbiol. Biotechnol. 92, 697–708. doi:10.1007/s00253-011-3601-z
Fang, J. Q., Qi, C., Zhang, X. H., Han, R. M., Huang, H. X., Wang, Z. S., et al. (2019). [Spatial Distribution and Pollution Evaluation of Carbon, Nitrogen, and Phosphorus in Sediments of Zhushan Bay at Taihu Lake]. Huan Jing Ke Xue 40, 5367–5374. doi:10.13227/j.hjkx.201905127
Fu, J., Hu, X., Tao, X., Yu, H., and Zhang, X. (2013). Risk and Toxicity Assessments of Heavy Metals in Sediments and Fishes from the Yangtze River and Taihu Lake, China. Chemosphere 93, 1887–1895. doi:10.1016/j.chemosphere.2013.06.061
Fu, J., Zhao, C., Luo, Y., Liu, C., Kyzas, G. Z., Luo, Y., et al. (2014). Heavy Metals in Surface Sediments of the Jialu River, China: Their Relations to Environmental Factors. J. Hazard. Mater. 270, 102–109. doi:10.1016/j.jhazmat.2014.01.044
Gao, L., Zhou, J. M., Yang, H., and Chen, J. (2005). Phosphorus Fractions in Sediment Profiles and Their Potential Contributions to Eutrophication in Dianchi Lake. Environ. Geol. 48, 835–844. doi:10.1007/s00254-005-0005-3
Guo, W., Yang, F., Li, Y., and Wang, S. (2017). New Insights into the Source of Decadal Increase in Chemical Oxygen Demand Associated with Dissolved Organic Carbon in Dianchi Lake. Sci. Total Environ. 603-604, 699–708. doi:10.1016/j.scitotenv.2017.02.024
Hakanson, L. (1980). An Ecological Risk index for Aquatic Pollution control.a Sedimentological Approach. Water Res. 14, 975–1001. doi:10.1016/0043-1354(80)90143-8
Hao, Z., Chen, L., Wang, C., Zou, X., Zheng, F., Feng, W., et al. (2019). Heavy Metal Distribution and Bioaccumulation Ability in marine Organisms from Coastal Regions of Hainan and Zhoushan, China. Chemosphere 226, 340–350. doi:10.1016/j.chemosphere.2019.03.132
Hose, G. C., and Van den Brink, P. J. (2004). Confirming the Species-Sensitivity Distribution Concept for Endosulfan Using Laboratory, Mesocosm, and Field Data. Arch. Environ. Contam. Toxicol. 47, 511–520. doi:10.1007/s00244-003-3212-5
Huang, C., Wang, X., Yang, H., Li, Y., Wang, Y., Chen, X., et al. (2014). Satellite Data Regarding the Eutrophication Response to Human Activities in the Plateau lake Dianchi in China from 1974 to 2009. Sci. Total Environ. 485-486, 1–11. doi:10.1016/j.scitotenv.2014.03.031
Ignatowicz, K. (2017). The Impact of Sewage Sludge Treatment on the Content of Selected Heavy Metals and Their Fractions. Environ. Res. 156, 19–22. doi:10.1016/j.envres.2017.02.035
Islam, M. S., Ahmed, M. K., Raknuzzaman, M., Habibullah -Al- Mamun, M., and Islam, M. K. (2015). Heavy Metal Pollution in Surface Water and Sediment: a Preliminary Assessment of an Urban River in a Developing Country. Ecol. Indicators 48, 282–291. doi:10.1016/j.ecolind.2014.08.016
Jaiswal, A., Verma, A., and Jaiswal, P. (2018). Detrimental Effects of Heavy Metals in Soil, Plants, and Aquatic Ecosystems and in Humans. J. Environ. Pathol. Toxicol. Oncol. 37, 183–197. doi:10.1615/jenvironpatholtoxicoloncol.2018025348
Järup, L. (2003). Hazards of Heavy Metal Contamination. Br. Med. Bull. 68, 167–182. doi:10.1093/bmb/ldg032
Jeong, H., Choi, J. Y., Lee, J., Lim, J., and Ra, K. (2020). Heavy Metal Pollution by Road-Deposited Sediments and its Contribution to Total Suspended Solids in Rainfall Runoff from Intensive Industrial Areas. Environ. Pollut. 265, 115028. doi:10.1016/j.envpol.2020.115028
Jia, Y., Wang, L., Li, S., Cao, J., and Yang, Z. (2018). Species-specific Bioaccumulation and Correlated Health Risk of Arsenic Compounds in Freshwater Fish from a Typical Mine-Impacted River. Sci. Total Environ. 625, 600–607. doi:10.1016/j.scitotenv.2017.12.328
Kelderman, P., and Osman, A. A. (2007). Effect of Redox Potential on Heavy Metal Binding Forms in Polluted Canal Sediments in Delft (The Netherlands). Water Res. 41, 4251–4261. doi:10.1016/j.watres.2007.05.058
Kumar, V., Parihar, R. D., Sharma, A., Bakshi, P., Singh Sidhu, G. P., Bali, A. S., et al. (2019). Global Evaluation of Heavy Metal Content in Surface Water Bodies: A Meta-Analysis Using Heavy Metal Pollution Indices and Multivariate Statistical Analyses. Chemosphere 236, 124364. doi:10.1016/j.chemosphere.2019.124364
Li, X., Liu, E., Zhang, E., Lin, Q., Yu, Z., Nath, B., et al. (2020). Spatio-temporal Variations of Sedimentary Metals in a Large Suburban lake in Southwest China and the Implications for Anthropogenic Processes. Sci. Total Environ. 707, 135650. doi:10.1016/j.scitotenv.2019.135650
Li, X., Shen, Z., Wai, O. W. H., and Li, Y.-s. (2000). Chemical Partitioning of Heavy Metal Contaminants in Sediments of the Pearl River Estuary. Chem. Speciation Bioavailability 12, 17–25. doi:10.3184/095422900782775607
Li, Z., Ma, Z., van der Kuijp, T. J., Yuan, Z., and Huang, L. (2014). A Review of Soil Heavy Metal Pollution from Mines in China: Pollution and Health Risk Assessment. Sci. Total Environ. 468-469, 843–853. doi:10.1016/j.scitotenv.2013.08.090
Liu, X., Wang, X., Zhang, L., Fan, W., Yang, C., Li, E., et al. (2020). Impact of Land Use on Shallow Groundwater Quality Characteristics Associated with Human Health Risks in a Typical Agricultural Area in Central China. Environ. Sci. Pollut. Res. Int. 28 (2), 1712–1724. doi:10.1007/s11356-020-10492-x
Liu, X., Wang, Z., Wang, X. L., Chao, Y., Xinxin, S., Xiaorong, L. V., et al. (2018). Ecological Risks Assessment of Selected Heavy Metals in the Waters of Chinese Lakes Based on Species Sensitivity Distributions. J. Lake Sci. 30, 1206–1217. doi:10.18307/2018.0504
Ma, G., and Wang, S. (2015). Temporal and Spatial Distribution Changing Characteristics of Exogenous Pollution Load into Dianchi Lake, Southwest of China. Environ. Earth Sci. 74, 3781–3793. doi:10.1007/s12665-015-4721-z
Maiz, I., Arambarri, I., Garcia, R., and Millán, E. (2000). Evaluation of Heavy Metal Availability in Polluted Soils by Two Sequential Extraction Procedures Using Factor Analysis. Environ. Pollut. 110, 3–9. doi:10.1016/s0269-7491(99)00287-0
Markert, B. (1987). Interelement Correlations in Plants. Z. Anal. Chem. 329, 462–465. doi:10.1007/bf00480087
Ouyang, W., Jiao, W., Li, X., Giubilato, E., and Critto, A. (2016). Long-term Agricultural Non-point Source Pollution Loading Dynamics and Correlation with Outlet Sediment Geochemistry. J. Hydrol. 540, 379–385. doi:10.1016/j.jhydrol.2016.06.043
Peng, J.-f., Song, Y.-h., Yuan, P., Cui, X.-y., and Qiu, G.-l. (2009). The Remediation of Heavy Metals Contaminated Sediment. J. Hazard. Mater. 161, 633–640. doi:10.1016/j.jhazmat.2008.04.061
Prasad, M. B. K., Ramanathan, A. L., Shrivastav, S. K., Anshumali, , and Saxena, R. (2006). Metal Fractionation Studies in Surfacial and Core Sediments in the Achankovil River basin in India. Environ. Monit. Assess. 121, 77–102. doi:10.1007/s10661-005-9108-2
Qian, Y., Cheng, C., Feng, H., Hong, Z., Zhu, Q., Kolenčík, M., et al. (2020). Assessment of Metal Mobility in Sediment, Commercial Fish Accumulation and Impact on Human Health Risk in a Large Shallow Plateau lake in Southwest of China. Ecotoxicology Environ. Saf. 194, 110346. doi:10.1016/j.ecoenv.2020.110346
Qiu, Y.-W. (2015). Bioaccumulation of Heavy Metals Both in Wild and Mariculture Food Chains in Daya Bay, South China. Estuarine, Coastal Shelf Sci. 163, 7–14. doi:10.1016/j.ecss.2015.05.036
Reddy, M. S., Basha, S., Sravan Kumar, V. G., Joshi, H. V., and Ramachandraiah, G. (2004). Distribution, Enrichment and Accumulation of Heavy Metals in Coastal Sediments of Alang-Sosiya Ship Scrapping Yard, India. Mar. Pollut. Bull. 48, 1055–1059. doi:10.1016/j.marpolbul.2003.12.011
Saha, P., and Paul, B. (2018). Assessment of Heavy Metal Toxicity Related with Human Health Risk in the Surface Water of an Industrialized Area by a Novel Technique. Hum. Ecol. Risk Assess. 25 (4), 966–987. doi:10.1080/10807039.2018.1458595
Samecka-Cymerman, A., and Kempers, A. J. (2001). Concentrations of Heavy Metals and Plant Nutrients in Water, Sediments and Aquatic Macrophytes of Anthropogenic Lakes (Former Open Cut Brown Coal Mines) Differing in Stage of Acidification. Sci. Total Environ. 281, 87–98. doi:10.1016/s0048-9697(01)00838-5
Shang, G. P., and Shang, J. C. (2007). Spatial and Temporal Variations of Eutrophication in Western Chaohu Lake, China. Environ. Monit. Assess. 130, 99–109. doi:10.1007/s10661-006-9381-8
Tang, W., Shan, B., Zhang, H., and Mao, Z. (2010). Heavy Metal Sources and Associated Risk in Response to Agricultural Intensification in the Estuarine Sediments of Chaohu Lake Valley, East China. J. Hazard. Mater. 176, 945–951. doi:10.1016/j.jhazmat.2009.11.131
USEPA (2014). Human Health Evaluation Manual, Supplemental Guidance: Update of Standard Default Exposure FactorOffice of Emergency and Remedial Response, 9200. Washington, D.C: Office of Emergency and Remedial Response, 1–120.
Wang, B., Duan, X., Feng, W., He, J., Cao, S., Liu, S., et al. (2019). Health Risks to Metals in Multimedia via Ingestion Pathway for Children in a Typical Urban Area of China. Chemosphere 226, 381–387. doi:10.1016/j.chemosphere.2019.03.158
Wang, H. D., Fang, F. G., and Xie, H. F. (2010). Research Situation and Outlook on Heavy Metal Pollution in Water Environment of China. Guangdong Trace Elem. Sci. 17, 14–18. doi:10.3724/sp.j.1077.2010.10305
Wang, J.-H., Yang, C., He, L.-Q. -S., Dao, G.-H., Du, J.-S., Han, Y.-P., et al. (2019). Meteorological Factors and Water Quality Changes of Plateau Lake Dianchi in China (1990-2015) and Their Joint Influences on Cyanobacterial Blooms. Sci. Total Environ. 665, 406–418. doi:10.1016/j.scitotenv.2019.02.010
Wang, Y., Wang, W., Wang, Z., Li, G., and Liu, Y. (2018). Regime Shift in Lake Dianchi (China) during the Last 50 Years. J. Ocean. Limnol. 36, 1075–1090. doi:10.1007/s00343-018-7171-0
Wang, Y., Yang, Z., Shen, Z., Tang, Z., Niu, J., and Gao, F. (2011). Assessment of Heavy Metals in Sediments from a Typical Catchment of the Yangtze River, China. Environ. Monit. Assess. 172, 407–417. doi:10.1007/s10661-010-1343-5
Wang, Z., Du, Y., Yang, C., Liu, X., Zhang, J., Li, E., et al. (2017a). Occurrence and Ecological hazard Assessment of Selected Antibiotics in the Surface Waters in and Around Lake Honghu, China. Sci. Total Environ. 609, 1423–1432. doi:10.1016/j.scitotenv.2017.08.009
Wang, Z., Zhang, J., Li, E., Zhang, L., Wang, X., and Song, L. (2017b). Combined Toxic Effects and Mechanisms of Microsystin-LR and Copper on Vallisneria Natans (Lour.) Hara Seedlings. J. Hazard. Mater. 328, 108–116. doi:10.1016/j.jhazmat.2016.12.059
Wang, Z., Zhou, J., Zhang, C., Qu, L., Mei, K., Dahlgren, R. A., et al. (2019). A Comprehensive Risk Assessment of Metals in Riverine Surface Sediments across the Rural-Urban Interface of a Rapidly Developing Watershed. Environ. Pollut. 245, 1022–1030. doi:10.1016/j.envpol.2018.11.078
Wei, C., and Wen, H. (2012). Geochemical Baselines of Heavy Metals in the Sediments of Two Large Freshwater Lakes in China: Implications for Contamination Character and History. Environ. Geochem. Health 34, 737–748. doi:10.1007/s10653-012-9492-9
Wei, H., Wang, S., Xu, E. G., Liu, J., Li, X., and Wang, Z. (2020). Synergistic Toxicity of Microcystin-LR and Cu to Zebrafish (Danio rerio). Sci. Total Environ. 713, 136393. doi:10.1016/j.scitotenv.2019.136393
Xia, W., Chen, L., Deng, X., Liang, G., Giesy, J. P., Rao, Q., et al. (2019). Spatial and Interspecies Differences in Concentrations of Eight Trace Elements in Wild Freshwater Fishes at Different Trophic Levels from Middle and Eastern China. Sci. Total Environ. 672, 883–892. doi:10.1016/j.scitotenv.2019.03.134
Xing, W., Wu, H., Hao, B., Huang, W., and Liu, G. (2013). Bioaccumulation of Heavy Metals by Submerged Macrophytes: Looking for Hyperaccumulators in Eutrophic Lakes. Environ. Sci. Technol. 47, 4695–4703. doi:10.1021/es303923w
Xu, F.-L., Li, Y.-L., Wang, Y., He, W., Kong, X.-Z., Qin, N., et al. (2015). Key Issues for the Development and Application of the Species Sensitivity Distribution (SSD) Model for Ecological Risk Assessment. Ecol. Indicators 54, 227–237. doi:10.1016/j.ecolind.2015.02.001
Xu, H., Paerl, H. W., Qin, B., Zhu, G., and Gaoa, G. (2010). Nitrogen and Phosphorus Inputs Control Phytoplankton Growth in Eutrophic Lake Taihu, China. Limnol. Oceanogr. 55, 420–432. doi:10.4319/lo.2010.55.1.0420
Yang, F., Zhang, N., Wei, C., Liu, J., and Xie, S. (2017). Arsenic Speciation in Organisms from Two Large Shallow Freshwater Lakes in China. Bull. Environ. Contam. Toxicol. 98, 226–233. doi:10.1007/s00128-016-2018-x
Yang, Y.-H., Zhou, F., Guo, H.-C., Sheng, H., Liu, H., Dao, X., et al. (2010). Analysis of Spatial and Temporal Water Pollution Patterns in Lake Dianchi Using Multivariate Statistical Methods. Environ. Monit. Assess. 170, 407–416. doi:10.1007/s10661-009-1242-9
Yi, Y., Yang, Z., and Zhang, S. (2011). Ecological Risk Assessment of Heavy Metals in Sediment and Human Health Risk Assessment of Heavy Metals in Fishes in the Middle and Lower Reaches of the Yangtze River basin. Environ. Pollut. 159, 2575–2585. doi:10.1016/j.envpol.2011.06.011
Yuan, Z., Taoran, S., Yan, Z., and Tao, Y. (2014). Spatial Distribution and Risk Assessment of Heavy Metals in Sediments from a Hypertrophic Plateau lake Dianchi, China. Environ. Monit. Assess. 186, 1219–1234. doi:10.1007/s10661-013-3451-5
Zhang, L., Liao, Q., Shao, S., Zhang, N., Shen, Q., and Liu, C. (2015a). Heavy Metal Pollution, Fractionation, and Potential Ecological Risks in Sediments from Lake Chaohu (Eastern China) and the Surrounding Rivers. Ijerph 12, 14115–14131. doi:10.3390/ijerph121114115
Zhang, L., Shi, Z., Jiang, Z., Zhang, J., Wang, F., and Huang, X. (2015b). Distribution and Bioaccumulation of Heavy Metals in marine Organisms in East and West Guangdong Coastal Regions, South China. Mar. Pollut. Bull. 101, 930–937. doi:10.1016/j.marpolbul.2015.10.041
Zhang, L., Ye, X., Feng, H., Jing, Y., Ouyang, T., Yu, X., et al. (2007). Heavy Metal Contamination in Western Xiamen Bay Sediments and its Vicinity, China. Mar. Pollut. Bull. 54, 974–982. doi:10.1016/j.marpolbul.2007.02.010
Zhang, R., Qi, F., Liu, C., Zhang, Y., Wang, Y., Song, Z., et al. (2019). Cyanobacteria Derived Taste and Odor Characteristics in Various Lakes in China: Songhua Lake, Chaohu Lake and Taihu Lake. Ecotoxicology Environ. Saf. 181, 499–507. doi:10.1016/j.ecoenv.2019.06.046
Zhao, Y., Xu, M., Liu, Q., Wang, Z., Zhao, L., and Chen, Y. (2018). Study of Heavy Metal Pollution, Ecological Risk and Source Apportionment in the Surface Water and Sediments of the Jiangsu Coastal Region, China: a Case Study of the Sheyang Estuary. Mar. Pollut. Bull. 137, 601–609. doi:10.1016/j.marpolbul.2018.10.044
Keywords: dianchi lake, heavy metals, bioaccumulation, source identification, risk assessment
Citation: Liu X, Zhang J, Huang X, Zhang L, Yang C, Li E and Wang Z (2022) Heavy Metal Distribution and Bioaccumulation Combined With Ecological and Human Health Risk Evaluation in a Typical Urban Plateau Lake, Southwest China. Front. Environ. Sci. 10:814678. doi: 10.3389/fenvs.2022.814678
Received: 14 November 2021; Accepted: 17 January 2022;
Published: 08 February 2022.
Edited by:
Andrew Hursthouse, University of the West of Scotland, United KingdomReviewed by:
Jaswinder Singh, Khalsa College, Amritsar, IndiaCopyright © 2022 Liu, Zhang, Huang, Zhang, Yang, Li and Wang. This is an open-access article distributed under the terms of the Creative Commons Attribution License (CC BY). The use, distribution or reproduction in other forums is permitted, provided the original author(s) and the copyright owner(s) are credited and that the original publication in this journal is cited, in accordance with accepted academic practice. No use, distribution or reproduction is permitted which does not comply with these terms.
*Correspondence: Zhi Wang, endhbmdAYXBtLmFjLmNu
Disclaimer: All claims expressed in this article are solely those of the authors and do not necessarily represent those of their affiliated organizations, or those of the publisher, the editors and the reviewers. Any product that may be evaluated in this article or claim that may be made by its manufacturer is not guaranteed or endorsed by the publisher.
Research integrity at Frontiers
Learn more about the work of our research integrity team to safeguard the quality of each article we publish.