- 1German Aerospace Center (DLR), Remote Sensing Technology Institute, Oberpfaffenhofen, Germany
- 2Department of Aerospace and Geodesy, Technische Universität München, Munich, Germany
- 3National Space Science Center, Chinese Academy of Sciences, Beijing, China
Precise knowledge about aerosols in the lower atmosphere (optical properties and vertical distribution) is particularly important for studying the Earth’s climatic and weather conditions. Measurements from satellite sensors in sun-synchronous and geostationary orbits can be used to map distributions of aerosol parameters in global or regional scales. The new-generation sensor Tropospheric Monitoring Instrument (TROPOMI) onboard the Copernicus Sentinel-5 Precursor (S5P) measures a wide variety of atmospheric trace gases and aerosols that are associated with climate change and air quality using a number of spectral bands between the ultraviolet and the shortwave infrared. In this study, we perform a sensitivity analysis of the forward model parameters and instrument information that are associated with the retrieval accuracy of aerosol layer height (ALH) and optical depth (AOD) using the oxygen (O2) A-band. Retrieval of aerosol parameters from hyperspectral satellite measurements requires accurate surface representation and parameterization of aerosol microphysical properties and precise radiative transfer calculations. Most potential error sources arising from satellite retrievals of aerosol parameters, including uncertainties in aerosol models, surface properties, solar/satellite viewing geometry, and wavelength shift, are analyzed. The impact of surface albedo accuracy on retrieval results can be dramatic when surface albedo values are close to the critical surface albedo. An application to the real measurements of two scenes indicates that the retrieval works reasonably in terms of retrieved quantities and fit residuals.
1 Introduction
Estimating aerosol optical properties and vertical distribution appears to be a challenging task because of real-time variations in aerosol microphysical properties. Remote sensing techniques for measuring aerosol properties from space have been developing rapidly and can be classified into two major groups. Active remote sensors such as the Cloud-Aerosol Lidar with Orthogonal Polarization (CALIOP) instrument measure backscattered signal and have the capability to accurately profile the scattering/absorption owing to aerosols/clouds in the atmosphere, whereas passive remote sensors can by far not offer the same level of details, but provide a global mapping of aerosol properties. Although aerosol height information with a vertical resolution as fine as 30 m can be obtained, the CALIOP observations possess a limited spatial coverage (Winker et al., 2009). In regard to passive sensors, considerable effort has been put into derive aerosol vertical information by employing the O2–O2 absorption band (∼ 477 nm), e.g., from the Ozone Monitoring Instrument (OMI) (Park et al., 2016; Chimot et al., 2017, 2018; Choi et al., 2019). Absorption of reflected sunlight by O2 in its A band (∼ 760 nm) has been extensively used to derive cloud height information, and the relevant studies can be found in Kokhanovsky et al. (2006a), Kokhanovsky et al. (2006b), Wang et al. (2008), Lelli et al. (2014), Loyola Rodriguez et al. (2007), Loyola et al. (2018). A number of passive satellite sensors have been launched to monitor aerosol properties on a global or regional scale using spectral information at various wavelengths. Atmospheric absorption in the O2 A-band provides an opportunity to derive vertical distributions of aerosols as a result of the dynamic range of optical depth in this spectral domain. Recently, a great amount of efforts have been made to retrieve the aerosol height information from the O2 A-band, e.g., the Scanning Imaging Absorption Spectrometer for Atmospheric Chartography (SCIAMACHY) (Corradini and Cervino, 2006; Kokhanovsky and Rozanov, 2010; Sanghavi et al., 2012), the Global Ozone Mapping Experiment (GOME) (Koppers and Murtagh, 1997) and GOME-2 (Tilstra et al., 2019), the Greenhouse Gases Observing Satellite (GOSAT) (Frankenberg et al., 2012), the Orbiting Carbon Observatory-2 (OCO-2) (Zeng et al., 2020). Some studies also focused on the joint use of O2 A and B bands for vertical profiling of aerosols (Ding et al., 2016; Xu et al., 2017b).
As a new generation of hyperspectral sensor, the Tropospheric Monitoring Instrument onboard the Copernicus Sentinel-5 Precursor satellite (TROPOMI/S5P, hereafter referred to as TROPOMI) (Veefkind et al., 2012) was designed to be a push-broom grating spectrometer observing trace gas concentrations and aerosol/cloud properties that are associated with air quality, ozone layer, and climate forcing. The satellite flies in a sun-synchronous orbit at 824 km altitude with an Equator crossing time of 13:30 local solar time, allowing to achieve a full daily global surface coverage thanks to a wide swath of 108°(∼ 2,600 km). The recorded TROPOMI spectra cover the ultraviolet–visible (UV–Vis, 270–500 nm), near-infrared (NIR, 675–775 nm), and shortwave infrared (SWIR, 2,305–2,385 nm). TROPOMI is the first Copernicus mission for atmospheric monitoring, launched on October 13, 2017, for a nominal lifetime of 7 years. In addition to the broad spectral coverage, TROPOMI can map global distributions of a broad range of air pollutants with a spatial resolution as high as 5.5 × 3.5 km2 (7.0 × 3.5 km2 prior to August 6, 2019). Band 6 of TROPOMI covers the O2 A-band and records the radiances and solar irradiances with a spectral sampling of 0.125–0.126 nm and a spectral resolution of 0.34–0.35 nm. The first calibration observations showed 3,000–5,000 and 250–700 for the high- and low-albedo signal-to-noise ratios, respectively. The main products of Band 6 are aerosols (height) and clouds (height and optical thickness). Further details of the instrument and measurement characteristics can be found in Kleipool et al. (2018), Ludewig et al. (2020).
Aerosol parameters like UV aerosol index, aerosol layer height (ALH) and optical depth (AOD) are useful to the global monitoring of air pollution in the lower atmosphere. The TROPOMI operational ALH retrieval algorithms in the O2 A-band were developed by the Royal Netherlands Meteorological Institute (KNMI) and use a neural network based forward model and the optimal estimation method for inversion (Rodgers, 2000). For more details about the operational retrieval algorithms see (Sanders and de Haan, 2013; Sanders et al., 2015; Nanda et al., 2018a; Nanda et al., 2018b).
Deriving aerosol information from satellite measurements remains a critical challenge in terms of retrieval sensitivity and accuracy. This is in general an underdetermined task and often requires several assumptions to be made with respect to the properties of aerosol and surface (Kokhanovsky and Rozanov, 2010). Li et al. (2009) also discussed several critical factors affecting the accuracy of aerosol remote sensing, including the assumptions in the aerosol model, treatment of the underlying surface, sensor calibration, and cloud screening. In a conventional retrieval framework, an operational retrieval handles the minimization of the objective function, which should include sufficiently fast radiative transfer computations, and is capable of dealing with large amount of satellite measurements and needs to converge robustly. Retrievals from synthetic measurements are necessary and important for analyzing the impact of forward and instrument model parameters on the retrieval output and exploring the expected retrieval performance using real measurements. Based on these experiments, an optimal retrieval setup for realistic measurement conditions and a better understanding of instrument characteristics could be achieved.
We have developed a conventional retrieval framework dedicated to estimating aerosol and cloud parameters from satellite measurements. Retrieval applications to the Earth Polychromatic Imaging Camera (EPIC) onboard the Deep Space Climate Observatory (DSCOVR) satellite were reported (Molina García et al., 2018a; Molina García et al., 2018b; Sasi et al., 2020a; Sasi et al., 2020b). In this work, we adapt the framework to the TROPOMI measurements, and the primary objective is to evaluate the retrieval feasibility and accuracy of aerosol parameters (ALH and AOD) using the O2 A-band of TROPOMI. Concerning the associated retrieval error characterization for the O2 A-band, only a few sensitivity studies were carried out (Hollstein and Fischer, 2014; Sanders et al., 2015). In this study, we extend the sensitivity analysis by taking into account more inputs during the inversion, i.e., different models for aerosol microphysical parameterization, surface properties, solar/viewing geometry, and wavelength shift. These inputs and information are considered to likely affect the retrieval accuracy and this sensitivity analysis aims to quantify the impact and importance of each input. Additionally, an application with real TROPOMI data can help us to better understand the measurement characteristics and retrieval performance in reality. We seek a characterization of the associated retrieval error by reasonbly assuming uncertainties on the crucial inputs identified in the sensitivity analysis. Accordingly, the retrieval could be further optimized by refining these inputs.
The remainder of the article is formulated as follows: A brief description of the retrieval algorithm is given in Section 2. Section 3 analyzes the sensitivity of retrieved aerosol parameters to different parameters and information associated with the instrument itself and radiative transfer calculations. A retrieval application using real TROPOMI measurements is given in Section 4. Section 5 concludes the study.
2 Theory
We have developed an algorithm dedicated to aerosol parameters retrieval from hyperspectral satellite sensors like TROPOMI. The theoretical concepts of atmospheric retrieval are presented in this section. This physical retrieval algorithm consists of a forward model in which radiative transfer of electromagnetic radiation through the atmosphere is calculated, and an inversion process in which a nonlinear minimization problem is solved. The purpose of the forward model is to simulate the signal received by the sensor as a function of atmospheric parameters and surface properties of interest, employing the discrete ordinate method to solve the radiative transfer equation. Inverse problems arising in atmospheric retrieval are typically ill-posed in the sense that perturbations in the data can cause large errors in the retrieval result. Our retrieval problem is formulated as a nonlinear least squares problem and can be solved by the Gauss–Newton method with the aid of Tikhonov regularization. In this study, the retrieval relies on the TROPOMI O2 A-band (758–771 nm). The recorded radiances and solar irradiances are converted to the reflectances. The inversion returns the best estimates of the retrieval target by approximating the measured reflectances with the simulated ones. In the forward model, aerosols are assumed as a single atmospheric layer with a fixed thickness of 0.5 km. The retrieval target ALH is defined as the middle height of this aerosol layer. Section 2.1 describes the physical and mathematical fundamentals of radiative transfer and different models for characterizing aerosol microphysical properties. Section 2.2 presents the inversion procedure and associated approaches.
2.1 Radiative Transfer
In a pseudo-spherical atmosphere, the radiative transfer equation for the diffuse radiance I(r, Ω) at point r in direction Ω = (μ, φ) is given by
where
is the source function summing the contributions of the single and multiple scattering terms Jss(r, Ω) and Jms(r, Ω), respectively, σext and σsct are the extinction and scattering coefficients, respectively, F0 is the incident solar flux, P the scattering phase function, Ω0 = (−μ0, φ0) with μ0 > 0 the incident solar direction, and
where cos Θ = Ω ⋅Ω′. The boundary conditions associated to the radiative transfer (Eq. 1) consist in the top-of-atmosphere boundary condition (r = rTOA),
and the surface boundary condition (r = rs),
where for a Lambertian surface, A is the surface albedo, and the notations Ω+ and Ω− stand for upward and downward directions, respectively.
The aerosol particles are modeled as components, while the size distribution dN(a)/d ln a of an aerosol component is chosen a log-normal distribution, characterized by the modal radius rmod, the standard deviation σ, and the total number of particles N0. If these parameters together with the (wavelength-dependent) refractive index maer are specified, the size averaged extinction and scattering cross sections, as well as the expansion coefficients of the size averaged phase function are computed as
respectively, where amin and amax are the lower and upper bounds of the size distribution, p(a) = (1/N0)dN(a)/da is the probability density function associated to the number size distribution, and Cext(a), Csct(a), and χn(a) are computed by an electromagnetic scattering code for a spherical particle of radius a. The aerosol components are externally mixed to form aerosol models. For an aerosol model m consisting of N aerosol components, the extinction and scattering cross sections, and the expansion coefficients of the phase function are computed as
respectively, where the weight
A set of aerosol models is an ensemble of a variety of aerosol models corresponding to different aerosol types. In the forward model, the following sets of aerosol models are taken into account:
• Set I The aerosol models employed in the Moderate Resolution Imaging Spectroradiometer (MODIS) aerosol retrieval algorithm (Levy et al., 2007a; Levy et al., 2007b). There are three fine-dominated (spherical) and one coarse-dominated (spheroid) aerosol models that differ by the single scattering albedo
• Set II The aerosol models employed in the Ozone Monitoring Instrument (OMI) Multiwavelength aerosol retrieval algorithm (Torres et al., 1998). There are five major aerosol types, i.e., weakly absorbing, biomass burning, desert dust, marine, and volcanic, whereby each type consists of several aerosol models depending on their optical properties and particle size distribution.
• Set III The aerosol models (mixtures of sulfate, dust, see salt, black carbon, and organic carbon components) obtained by a cluster analysis using the Goddard Chemistry Aerosol Radiation and Transport (GOCART) model (Chin et al., 2002; Taylor et al., 2015).
• Set IV The aerosol models (mixtures of water-insoluble, water-soluble, soot, sea-salt, mineral, mineral transported, and sulfate components) included in the Optical Properties of Aerosols and Clouds (OPAC) dataset (Hess et al., 1998; Thomas et al., 2009).
The radiative transfer computation relies on the discrete ordinate method with matrix exponential (Doicu and Trautmann, 2009a; Doicu and Trautmann, 2009b). To deal with computationally expensive radiative transfer calculations in the TROPOMI O2 A-band absorption channel, several acceleration techniques, as for example, the telescoping technique (Spurr, 2008; Efremenko et al., 2013), the method of false discrete ordinate, the correlated k-distribution method (Goody et al., 1989), and principal component analysis (Natraj et al., 2005, 2010) are implemented.
In Section 3.1 we investigate the impact of different aerosol models on the retrieval performance.
2.2 Inversion
The retrieval is performed by using the method of Tikhonov regularization (Tikhonov, 1963). Essentially, the inverse problem is solved by minimizing the objective function,
where
The goal of minimizing the Tikhonov function (Eq. 5) is to search for a solution providing a compromise between the residual term
where
is the regularized generalized inverse matrix, and Ki the Jacobian matrix of F at xλ,i.
The penalty term
The iterative process is terminated when a favorable convergence is reached in accordance with predefined stopping tolerances. The favorable convergence is based on two tests:
1. the x-convergence test, which checks if the sequence xλ,i is converging and the change in xλ satisfies a predefined criteria, and
2. the relative-function-convergence test, which checks if the relative change in
3 Sensitivity to Expected Error Sources
In practice, several assumptions in the forward model (e.g., aerosol model, instrument parameters etc.) are required. Nevertheless, it is of importance to investigate how imperfect forward model inputs and instrumental knowledge contribute to the retrieval performance. In this section, we analyze the impact of different inputs used in the forward and instrument models on the retrieval of aerosol properties from the O2 A-band spectral measurements. Two types of uncertainty are discussed: 1) model uncertainty (aerosol model) and 2) parameter uncertainty (surface albedo, solar/sensor viewing geometry, and wavelength calibration). Only the most representative error sources identified in the consortium for satellite remote sensing of aerosols (also from previous studies, e.g., (Sanders and de Haan, 2013; Sanders et al., 2015) were considered in this study. The sensitivity analysis was based on simulated reflectance spectra that largely resemble typical TROPOMI measurements in the O2 A-band.
Here, the state vector x consists of two components, i.e., AOD τaer and ALH haer. The principle of AOD retrieval lies on the aerosol scattering and absorption features in the O2 A-band. The ALH retrieval depends mainly on a narrow oxygen absorption band (between 760 and 762 nm) where aerosol layer will attenuate the oxygen absorption below. The inversion results in this section are represented as retrieval errors (with respect to the true state) due to various inputs in the forward and instrument models.
3.1 Aerosol Model
Each set of aerosol models described in Section 2.1 is a collection of aerosol models that are employed to parameterize the aerosol microphysical properties for specific aerosol types, including the corresponding scattering and absorption properties, which plays an important role in an accurate retrieval. In this section, its influence on the retrieval of AOD and ALH is discussed. For an assumption of aerosol microphysical properties, three sets of aerosol models were considered in this analysis: Sets I, II, and III that have been used in satellite retrievals and weather/climate model simulations, respectively.
The simulated reflectance spectra (758–771 nm) using three sets of aerosol models are compared in Figure 1. “MODABS” from Set I, “MODABS” from Set II, and “Organic + Sulfate” from Set III were considered as the three models should represent the same aerosol characteristics. For Sets II and III, three values of 0.0, 0.5, and 1.0 for the effective radius Reff were chosen. An aerosol loading scenario with 0.5 and 3.5 km for AOD and ALH, respectively, was considered. 0.05, 30°, 0°, and 180°were chosen for the surface albedo, solar and viewing zenith, and relative azimuth angles, respectively.
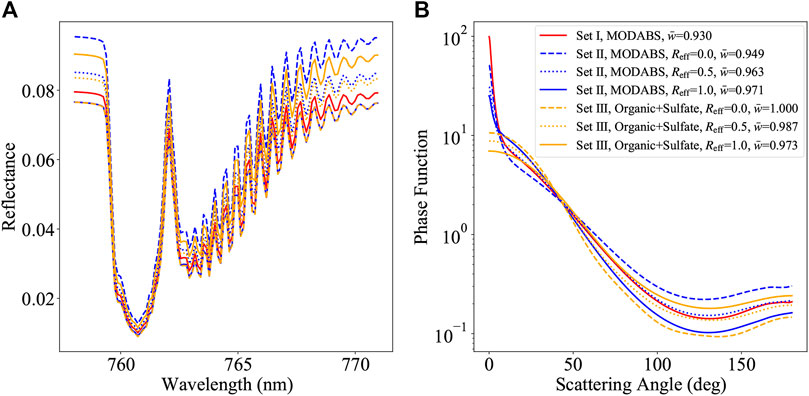
FIGURE 1. (A): Simulated reflectances as functions of wavelength in the TROPOMI O2 A-band (758–771 nm) using aerosol models from Sets I, II and III, respectively. (B): The corresponding phase functions as functions of scattering angle.
The left panel of Figure 1 shows that the reflectance using Set I lies between the highest and lowest reflectances using Sets II and III. By using Reff = 1.0 and Reff = 0.0 for Sets II and III, respectively, the simulated reflectances are almost equivalent. Nevertheless, the spectra do not match perfectly between the three sets of aerosol models. For example, the blue solid line (“Organic + Sulfate” from Set III, Reff = 0.0) and the yellow dotted line (“MODABS” from Set II, Reff = 1.0) are close. Both spectra agree well between 760 and 762 nm where contains majority of aerosol height information, while slight discrepancies can be found elsewhere. The phase functions (P) and single scattering albedos (
To study the influence of aerosol models on the retrieval results, an experiment using synthetic spectra simulated with “MODABS” from Set I was carried out. In the subsequent retrieval procedure, “MODABS” from Set II and “Organic + Sulfate” from Set III were used, respectively. In this case, the underlying surface was assumed to be a dark surface (with an albedo of 0.05). The retrieval errors of ALH and AOD using Sets II and III (with Reff = 0.0, 0.1, 0.2, … , 1.0) are shown in Figures 2, 3, respectively. Figures 2, 3 reveal that applying an inappropriate aerosol model may result in significant retrieval errors. In both figures, the error of ALH reaches up to 1.9 km when the true value is 9.5 km, and the largest negative error of AOD is about −0.5 when the true value is 2.0. Interestingly, the retrieval error gradually increases in Figure 2 with increasing Rreff, while the error in Figure 3 behaves in an opposite way. Although the differences in the simulated reflectance spectra between the models characterizing the same aerosol type found in Figure 1 can result in different retrieval outputs, its impact is estimated to be moderate.
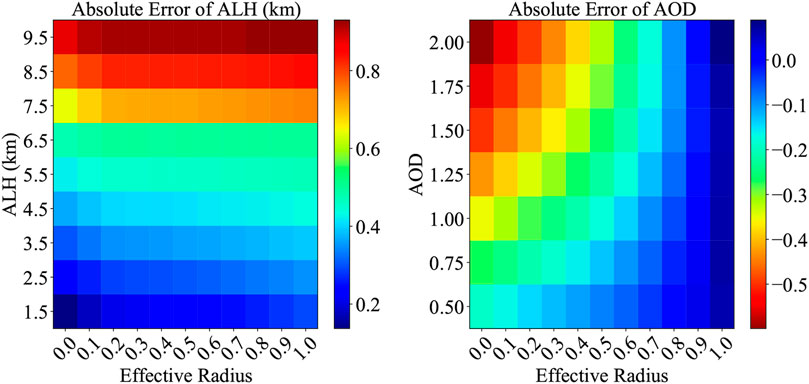
FIGURE 2. Retrieval (absolute) errors of ALH and AOD using “MODABS” from Set II. The synthetic spectra were simulated using “MODABS” from Set I.
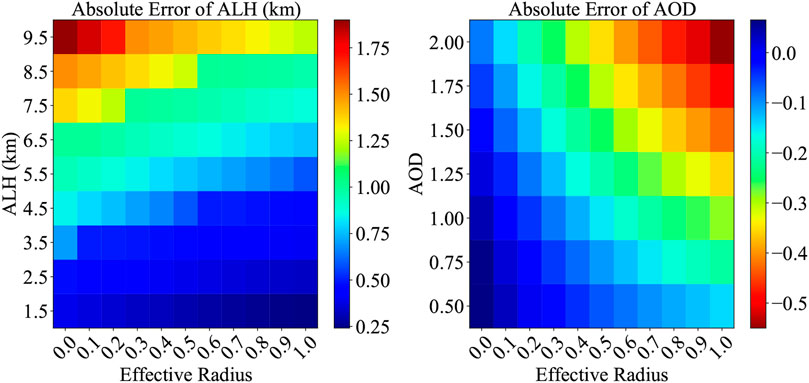
FIGURE 3. Retrieval (absolute) errors of ALH and AOD using “Organic + Sulfate” from Set III. The synthetic spectra were simulated using “MODABS” from Set I.
3.2 Surface Albedo
Apart from the aerosol parameterization, the previous sensitivity studies (Sanders et al., 2015; Nanda et al., 2018a) demonstrated that the accuracy of surface properties could greatly influence the aerosol retrieval from the O2 A-band measurements.
The sensitivity of the reflectance spectrum to the retrieved parameter can be described by its partial derivative with respect to this parameter. The partial derivatives of the reflectance (in terms of the natural logarithm) with respect to ALH and AOD as functions of surface albedo are illustrated in Figure 4. The left panel of Figure 4 indicates that the derivatives decrease faster with the decreasing ALH when the value of AOD was assumed to be identical. As can be seen from the right panel of Figure 4, the derivative curves do not differ significantly between the three values of AOD.
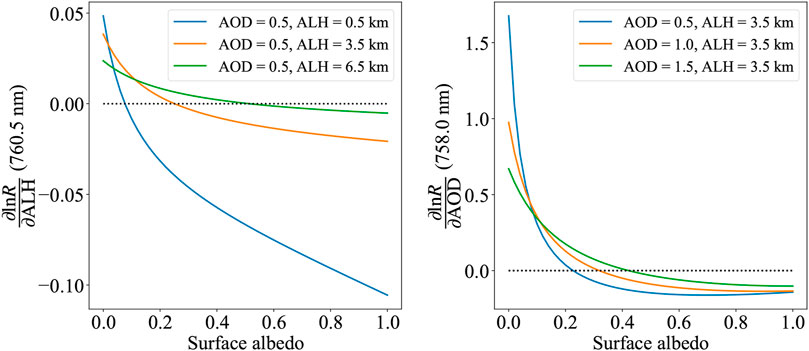
FIGURE 4. Derivative of reflectance spectrum with respect to ALH and AOD as functions of surface albedo.
A surface albedo at the turning point where the partial derivative of the reflectance with respect to ALH or AOD is zero (dotted line), is called the critical surface albedo (Seidel and Popp, 2012), namely, this special surface albedo is “critical” for retrieval of ALH or AOD. It is noteworthy that the critical surface albedo varies with the value of ALH or AOD. Figure 4 illustrates that the value of the critical surface albedo increases substantially with the increasing ALH (left panel for a fixed AOD), while the value of the critical surface albedo increases gradually when AOD increases (right panel for a fixed ALH).
The relative retrieval errors resulting from the uncertainty in the surface albedo is shown in Figure 5. In this case, 0.5 and 3.5 km were used as the true values of AOD and ALH, respectively, “MODABS” from Set I was used as the aerosol model. Over darker or less bright surface (with albedo values of 0.05 and 0.15), the uncertainty of the surface albedo seems to produce less impact on the aerosol retrieval. Apparently, the retrieval error is more pronounced over a brighter surface (with albedo values of 0.5 and 0.9). When the value of surface albedo is around the critical surface albedo, an error of 5% in the surface albedo could yield errors of about 180% (green line in the left panel of Figure 5) and 80% (green line in the right panel of Figure 5) in the retrieved ALH and AOD, respectively.
The critical surface albedo is between 0.2 and 0.3 for ALH (orange line in the left panel of Figure 4) and AOD (blue line in the right panel of Figure 4). Along with Figure 5, an indication is that an overestimated surface albedo tends to introduce an underestimation of ALH and an overestimation of AOD for surface albedos higher than the critical surface albedo, whereas for surface albedos lower than the critical surface albedo, an underestimated surface albedo is inclined to cause an overestimation of ALH and an underestimation of AOD. When the surface albedo is close to the critical surface albedo, the retrieval can be exceptionally challenging even though the error of the surface albedo is small. In Figure 5, the largest retrieval errors of ALH and AOD correspond to the case with surface albedo of 0.3. These findings are consistent with the implications from (Seidel and Popp, 2012).
The consequence of using inaccurate surface albedos in retrieval can be serious, and a joint-fitting of the surface albedo cannot guarantee an improved retrieval accuracy, albeit with more computational effort (Sanders et al., 2015). In practice, the geometry-dependent effective Lambertian equivalent reflectivity (GE_LER) (Loyola et al., 2020) can be considered to be a reliable alternative. Its retrieval algorithm is based on the framework called the “Full-Physics Inverse Learning Machine” (FP-ILM) (Xu et al., 2017a; Efremenko et al., 2017; Hedelt et al., 2019). In contrast to LER climatologies, GE_LER takes into account the drastically improved spatial resolution of TROPOMI and represents actual surface conditions accurately. The retrieved GE_LER data has been included in the operational TROPOMI products for cloud and UV/VIS trace gases O3, SO2, and HCHO.
3.3 Geometry
In this section, we discuss the influence of solar and sensor viewing geometry parameters on the aerosol retrieval. The simulation was performed by assuming an error in the solar zenith angle (SZA) and viewing zenith angle (VZA), respectively.
Figure 6 shows the relative retrieval errors of AOD and ALH due to the relative error of SZA. As expected, when dealing with measurements at higher SZAs, the retrieval accuracy is more sensitive to the error of SZA. With an error of 5% in SZA at 75°, the relative error of retrieved ALH and AOD reaches up to 20 and 90%, respectively.
Figure 7 shows the relative retrieval errors of AOD and ALH due to the relative error of VZA. Likewise, the retrieval error of both parameters increases with the increasing VZA. The relative error of retrieved ALH and AOD is up to 30 and 20%, respectively. As compared to Figure 6, VZA seems to impose less impact on the retrieved aerosol parameters. In reality, the accuracy of measured SZA and VZA is within 1% and estimated to make a minor impact on retrieval results.
3.4 Wavelength Calibration
An accurate wavelength calibration of the radiance and solar irradiance measurements is required during the Level-1b and Level-2 data processing. A wavelength shift is an offset found in the spectral position of a measured signal. It is anticipated that uncertainty in the wavelength can introduce an error in the retrieval output. Here, we performed retrievals by assuming a wavelength shift with different combinations of aerosol parameters over two surface types (with albedo values of 0.05 and 0.15). Figures 8, 9 depicts the retrieval errors of ALH and AOD as functions of the wavelength shift as well as the fit residuals. In Figure 8, the true value of ALH was assumed to be 1.5, 3.5, 5.5, and 7.5 km, respectively, and the true value of AOD was fixed to be 0.5; whereas in Figure 9, the true value of AOD was 0.5, 1.0, 1.5, and 2.0, respectively, and the true value of ALH was fixed to be 3.5 km.
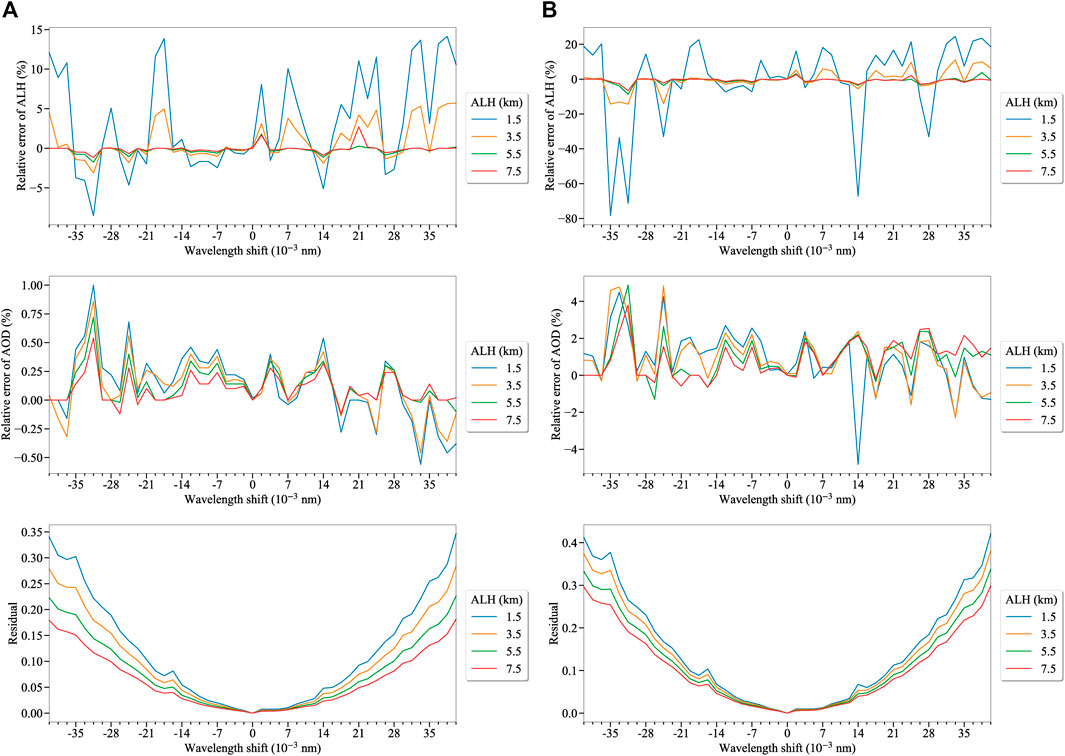
FIGURE 8. Relative retrieval errors of ALH and AOD as functions of wavelength shift as well as the corresponding fit residuals. The retrievals were performed with true values of a fixed AOD ( ∼ 0.5) and varying ALH. Results with surface albedo values of 0.05 (A) and 0.15 (B) are plotted.
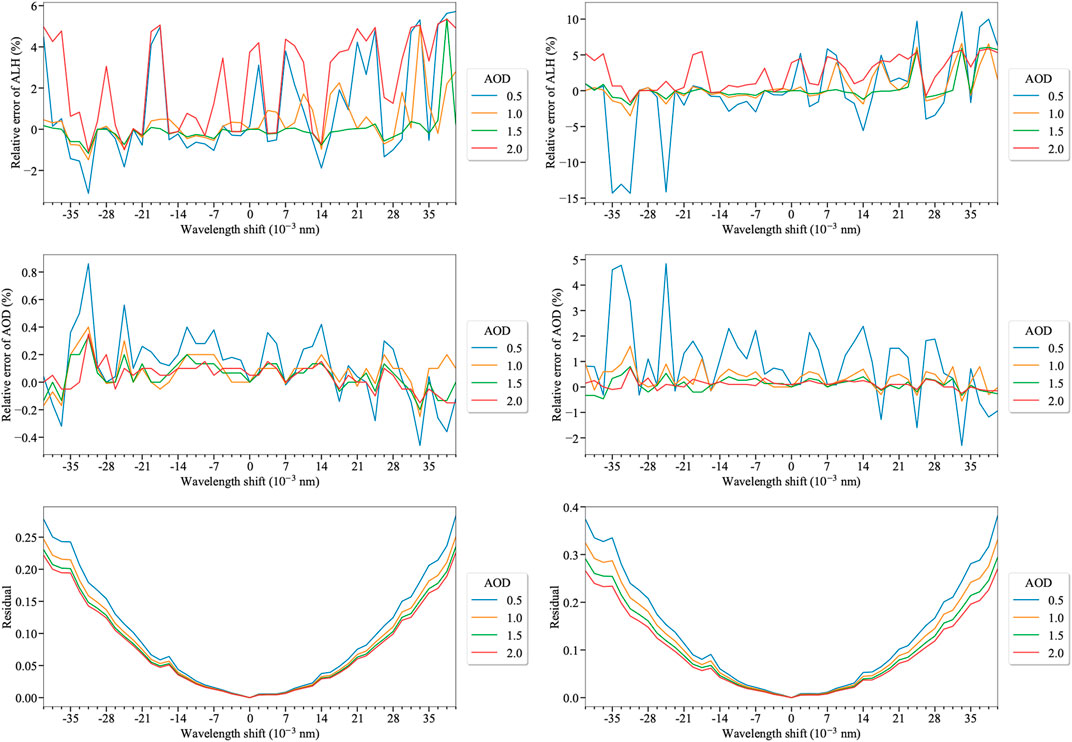
FIGURE 9. The same as in Figure 8 but with true values of a fixed ALH ( ∼ 3.5 km) and varying AOD.
The wavelength shift has a seemingly greater impact on the retrieval results over the brighter surface. The retrieval errors of ALH and AOD and the corresponding residuals are “augmented” by the larger surface albedo. The residual plots show a monotonic increase with the increasing wavelength shift, whereas the retrieval errors appear to increase oscillatingly with the increasing wavelength shift. The ALH retrieval only relies on the information from a narrow range (760–762 nm), and therefore, the error plots indicate a more pronounced impact on the ALH retrieval when the wavelength shift increases, as compared to the AOD retrieval.
Based on the synthetic analysis, uncertainties in surface albedo and wavelength calibration could cause significant effects on the retrieval output and should not be neglected when dealing with retrievals from real measurements.
4 Application to Real Data
In this section, we present the retrieval results using the real TROPOMI measurements. We considered two scenes on June 22, 2018 and June 6, 2020, respectively. Figure 10 displays the corresponding true-color images from the Visible Infrared Imaging Radiometer (VIIRS) on the Suomi National Polar-orbiting Partnership (Suomi NPP) satellite. The red rectangular region indicates the chosen TROPOMI scene. The first TROPOMI scene observed a part of Atlantic ocean near West Africa with latitudes between 10.0 and 12.0°N and longitudes between 22.0 and 24.0°W. The second TROPOMI scene detected a desert dust aerosol case over the Sahara with latitudes between 12.0 and 21.0°N and longitudes between 16.0 and 20.0°W.
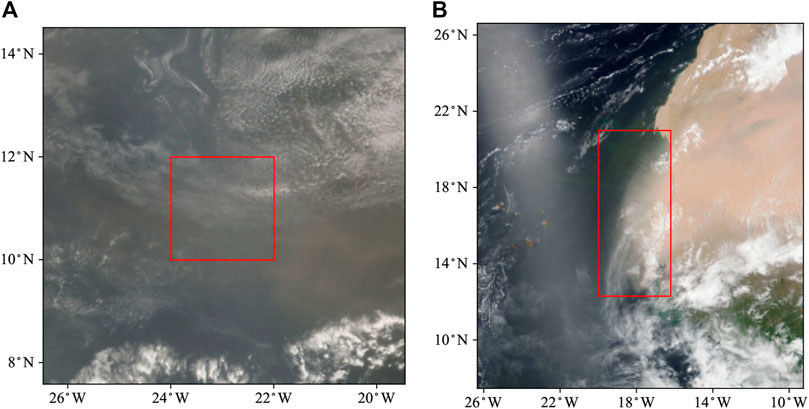
FIGURE 10. VIIRS true-color images on June 22, 2018 (A) and June 6, 2020 (B). The red rectangular region displays the chosen TROPOMI scene.
Table 1 describes the TROPOMI O2 A-band measurements and the input parameters for retrieval. The aerosol models “MODABS” and “DUST” from Set II were selected for the retrieval of the two scenes, respectively. The cloud parameters were taken from the operational TROPOMI cloud products (OCRA/ROCINN) whose retrieval algorithms were described by Loyola et al. (2018). Inaccuracy in surface properties could play a crucial role in retrieval, as discussed in Section 3.2. Instead of fitting it as an additional parameter in the state vector or using LER climatologies, the surface albedo was taken from the retrieved GE_LER product. The a priori state xa was updated with the retrieval from the previous processed pixel.
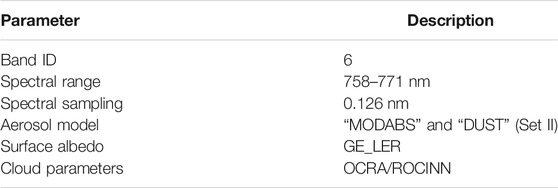
TABLE 1. Spectral characteristics of TROPOMI O2 A-band measurements and the main input parameters for retrieval.
We removed pixels with a cloud fraction greater than 0.15 that ensures a sufficient number of valid retrievals without significant cloud contamination. The pixels with the TROPOMI UV aerosol index below 0.0 were not processed. The maximum SZA for processing was 75°.
The inversion calculation carried out by the computer code (yet to be optimized) typically converges in less than five iterations. This translate to a 2–3 min processing time of one pixel on an up-to-date desktop. The retrieval results of ALH and AOD for the two selected TROPOMI scenes are plotted in Figures 11, 12, respectively, which seem to capture the spatial patterns seen from Figure 10 under different aerosol loading scenarios. The retrieved ALH on June 22, 2018 looks higher in the south, and the lowest values of ALH can be found in the northeast where the highest values of AOD are located. Due to heavy cloud contamination on June 6, 2020, a small number of valid pixels were processed. Nevertheless, the spatial distribution of desert dust aerosols is well described by the retrieved aerosol parameters. For reference, the operational retrieval products processed by KNMI were plotted in the bottom row of Figures 11, 12. As compared to the operational products, our retrieved aerosol parameters capture nearly the same spatial pattern and the ALH results are slightly underestimated. However, our retrieved AOD values seem evidently lower than the operational ones. Different surface albedo data and aerosol microphysical properties used in the two retrieval algorithms are possibly the main factors explaining these discrepancies. A comprehensive validation is needed in the future.
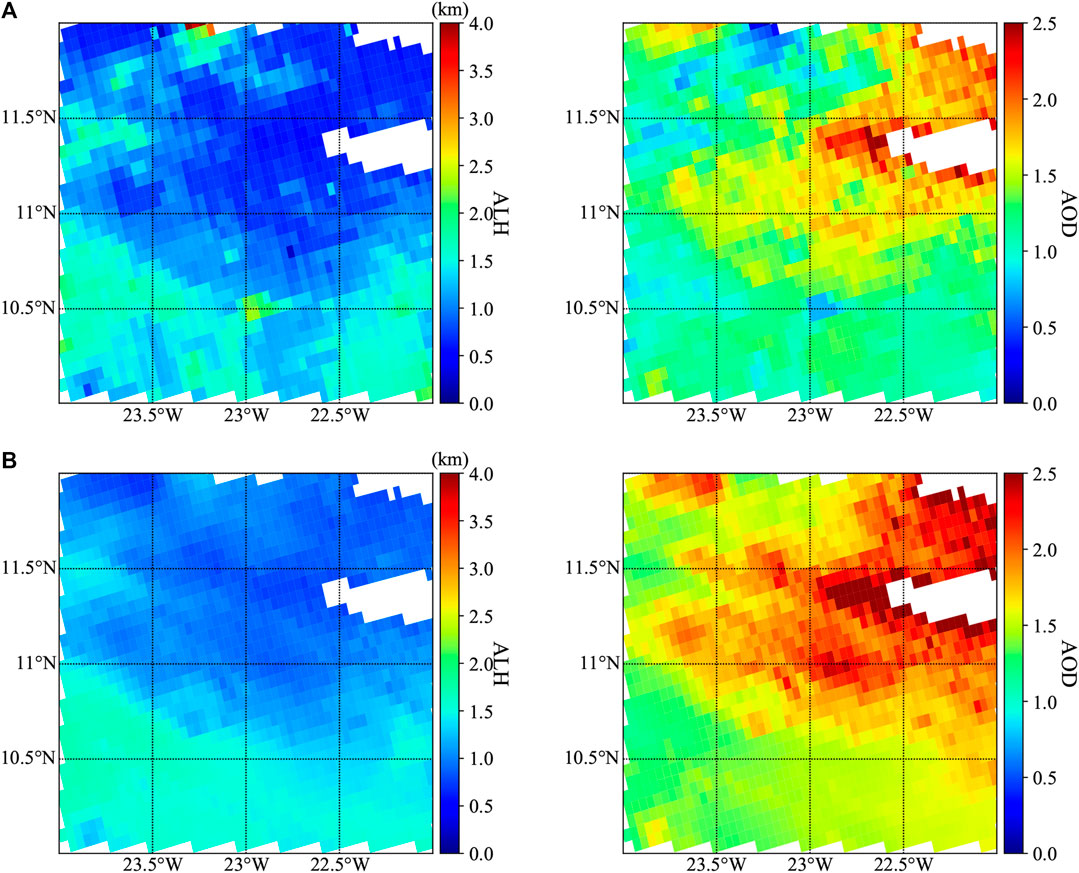
FIGURE 11. Retrieved ALH and AOD for TROPOMI scene on June 22, 2018 between our results (A) and the operational product (B).
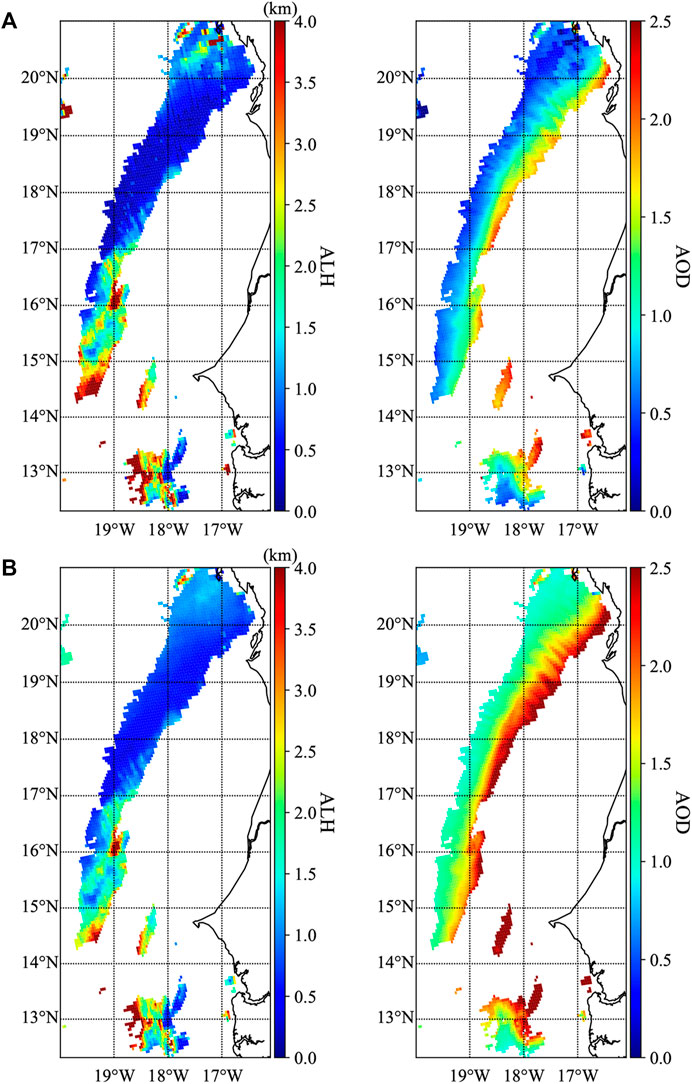
FIGURE 12. Retrieved ALH and AOD for TROPOMI scene on June 6, 2020 between our results (A) and the operational product (B).
Table 2 lists the propagated retrieval error with taking into account the most important error sources that are figured out in Section 3. According to a thorough comparison with the OMI LER for clear sky scenarios (Loyola et al., 2020), an error of 0.01 was added to the original values of surface albedo from GE_LER, causing a mean bias of 0.130 3 km and 0.091 8 on the retrieved ALH and AOD, respectively. For wavelength calibration, we computed the effect of a shift of 0.007 nm in the nominal wavelength grid for the radiance (with the spectral bin size is 0.10 nm). A mean bias of 0.079 3 km and 0.003 0 on the retrieved ALH and AOD was achieved. Please note that a wavelength shift of 0.007 nm in the case of TROPOMI is already quite large and used here as a conservative estimate.
Figure 13 compares the observed and modeled reflectance spectra on June 22, 2018 (top row) and June 6, 2020 (bottom row). The modeled reflectance spectra were simulated with the retrieved aerosol quantities after convergence. We randomly chosen four pixels with converged retrieval runs from each scene. The relative residuals turn out to be higher near 760 nm where the reflectance is rather low. For all pixels, the simulated and observed reflectance spectra reach a good agreement, providing an evidence of an overall good fit.
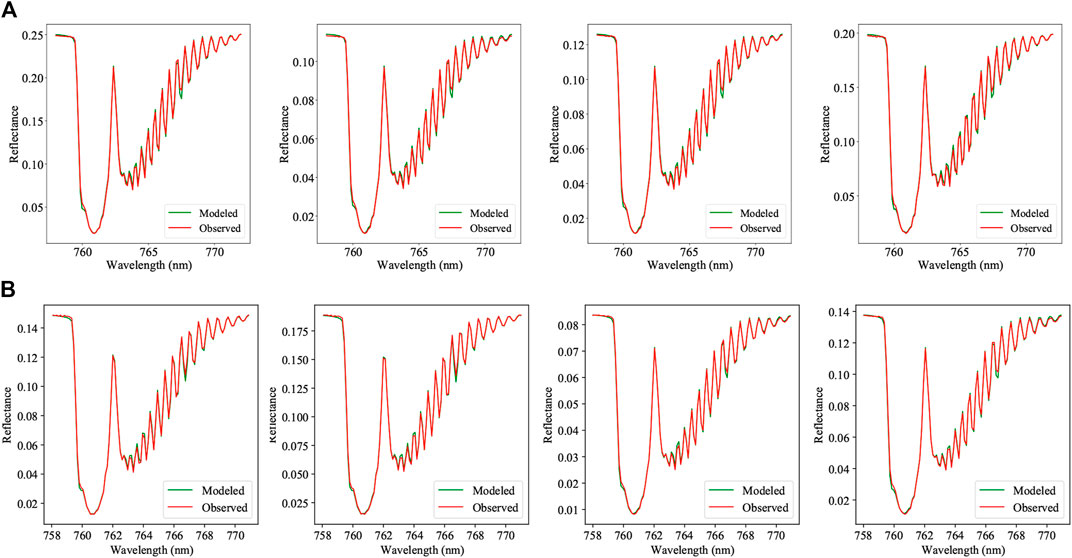
FIGURE 13. Observed and modeled reflectance spectra for four random TROPOMI ground pixels on June 22, 2018 (A) and June 6, 2020 (B).
5 Conclusion
A conventional retrieval algorithm for hyperspectral satellite remote sensing that meets the scientific requirements should enable efficient radiative transfer calculations and reliable inversion computation. We have developed an aerosol retrieval algorithm for estimating aerosol parameters (ALH and AOD) from the O2 A-band of TROPOMI onboard the S5P satellite. The key objective of this study was to investigate the impact of forward and instrument model parameters on the retrieval result. The aerosol model for microphysical properties, solar/viewing geometry, surface properties, wavelength calibration have been taken into account.
Aerosol models play an important role in accurately describing aerosol microphysical properties under various measurement conditions. The forward and retrieval simulations using aerosol models from three sets have been compared for the same aerosol type. An inaccurate aerosol model could have a moderate loss of accuracy of retrieved aerosol parameters. Choosing an appropriate aerosol model would be useful in the operational data processing. However, this is not an easy job since for a given measurement it is likely that not just one aerosol model delivers the good fit. Rao et al. (2021) have developed an optimized model selection schemed based on the Bayesian approach and are currently validating its applicability to the real TROPOMI measurements.
As expected, an error of the surface albedo can contribute largely to the accuracy of aerosol retrievals, particularly if the surface albedo is around the critical surface albedo. We suggest that the GE_LER product can be employed instead of LER climatologies based on low-resolution measurements or fitting the surface albedo simultaneously.
The solar and viewing zenith angles represent the solar and viewing geometry and their accuracy are important to the retrieval accuracy as well. An enhancement in the retrieval algorithm is needed particularly when dealing with satellite measurements at higher SZAs. Another instrument parameter worthy of attention is the wavelength uncertainty. The wavelength shift can potentially deteriorate the quality of the retrieved parameters (particularly of ALH), although it may have a minor impact on the fit residuals.
Retrievals using real TROPOMI O2 A-band data recorded on June 22, 2018 and June 6, 2020 have been performed. The retrieved aerosol parameters resemble both aerosol loading scenarios identified in the VIIRS images and the simulated spectra well approximate the observed ones, that have proved the application feasibility of the algorithm itself. Inaccurate surface albedo was supposed to be the most important error sources in practice and reliable measurements of surface albedo are required.
The future work will focus on a comprehensive global/regional validation with other satellite-based (e.g., CALIPO) and ground-based measurements. For efficiency purposes, the development of a retrieval framework using machine learning techniques is ongoing.
Data Availability Statement
The raw data supporting the conclusion of this article will be made available by the authors, without undue reservation.
Author Contributions
Conceptualization, JX and LR; methodology, JX and AD; software and visualization, LR; investigation, DE and DL; data curation, LR; writing–original draft preparation, LR and JX; writing–review and editing, DE, DL, and AD; supervision, JX and AD; project administration, DE and DL.
Funding
This research was supported by the DLR programmatic (Nachwuchsgruppe “Retrieval der nächsten Generation”, 2472469) and the CAS “Pioneering Initiative Talents Program” under Grant E1RC2WB2. The work of LR was partly funded by the Chinese Scholarship Council.
Conflict of Interest
The authors declare that the research was conducted in the absence of any commercial or financial relationships that could be construed as a potential conflict of interest.
Publisher’s Note
All claims expressed in this article are solely those of the authors and do not necessarily represent those of their affiliated organizations, or those of the publisher, the editors and the reviewers. Any product that may be evaluated in this article, or claim that may be made by its manufacturer, is not guaranteed or endorsed by the publisher.
Acknowledgments
The authors are grateful to the DLR’s UPAS team for processing the cloud and GE_LER products from TROPOMI.
References
Chimot, J., Veefkind, J. P., Vlemmix, T., de Haan, J. F., Amiridis, V., Proestakis, E., et al. (2017). An Exploratory Study on the Aerosol Height Retrieval from OMI Measurements of the 477 Nm O2 − O2 Spectral Band Using a Neural Network Approach. Atmos. Meas. Tech. 10, 783–809. doi:10.5194/amt-10-783-2017
Chimot, J., Veefkind, J. P., Vlemmix, T., and Levelt, P. F. (2018). Spatial Distribution Analysis of the OMI Aerosol Layer Height: a Pixel-By-Pixel Comparison to CALIOP Observations. Atmos. Meas. Tech. 11, 2257–2277. doi:10.5194/amt-11-2257-2018
Chin, M., Ginoux, P., Kinne, S., Torres, O., Holben, B. N., Duncan, B. N., et al. (2002). Tropospheric Aerosol Optical Thickness from the GOCART Model and Comparisons with Satellite and Sun Photometer Measurements. J. Atmos. Sci. 59, 461–483. doi:10.1175/1520-0469(2002)059⟨0461:TAOTFT⟩2.0.CO;2
Choi, W., Lee, H., Kim, J., Ryu, J.-Y., Park, S. S., Park, J., et al. (2019). Effects of Spatiotemporal O4 Column Densities and Temperature-dependent O4 Absorption Cross-Section on an Aerosol Effective Height Retrieval Algorithm Using the O4 Air Mass Factor from the Ozone Monitoring Instrument. Remote Sensing Environ. 229, 223–233. doi:10.1016/j.rse.2019.05.001
Corradini, S., and Cervino, M. (2006). Aerosol Extinction Coefficient Profile Retrieval in the Oxygen A-Band Considering Multiple Scattering Atmosphere. Test Case: SCIAMACHY Nadir Simulated Measurements. J. Quantitative Spectrosc. Radiative Transfer 97, 354–380. doi:10.1016/j.jqsrt.2005.05.061
Ding, S., Wang, J., and Xu, X. (2016). Polarimetric Remote Sensing in Oxygen A and B Bands: Sensitivity Study and Information Content Analysis for Vertical Profile of Aerosols. Atmos. Meas. Tech. 9, 2077–2092. doi:10.5194/amt-9-2077-2016
Doicu, A., and Trautmann, T. (2009a). Discrete-ordinate Method with Matrix Exponential for a Pseudo-spherical Atmosphere: Scalar Case. J. Quantitative Spectrosc. Radiative Transfer 110, 146–158. doi:10.1016/j.jqsrt.2008.09.014
Doicu, A., and Trautmann, T. (2009b). Discrete-ordinate Method with Matrix Exponential for a Pseudo-spherical Atmosphere: Vector Case. J. Quantitative Spectrosc. Radiative Transfer 110, 159–172. doi:10.1016/j.jqsrt.2008.09.013
Efremenko, D., Doicu, A., Loyola, D., and Trautmann, T. (2013). Acceleration Techniques for the Discrete Ordinate Method. J. Quantitative Spectrosc. Radiative Transfer 114, 73–81. doi:10.1016/j.jqsrt.2012.08.014
Efremenko, D. S., Loyola R., D. G., Hedelt, P., and Spurr, R. J. D. (2017). Volcanic SO2 Plume Height Retrieval from UV Sensors Using a Full-Physics Inverse Learning Machine Algorithm. Int. J. Remote Sensing 38, 1–27. doi:10.1080/01431161.2017.1348644
Frankenberg, C., Hasekamp, O., O'Dell, C., Sanghavi, S., Butz, A., and Worden, J. (2012). Aerosol Information Content Analysis of Multi-Angle High Spectral Resolution Measurements and its Benefit for High Accuracy Greenhouse Gas Retrievals. Atmos. Meas. Tech. 5, 1809–1821. doi:10.5194/amt-5-1809-2012
Goody, R., West, R., Chen, L., and Crisp, D. (1989). The Correlated-K Method for Radiation Calculations in Nonhomogeneous Atmospheres. J. Quant. Spectrosc. Radiat. Transf. 42, 437–651. doi:10.1016/0022-4073(89)90044-7
Hedelt, P., Efremenko, D. S., Loyola, D. G., Spurr, R., and Clarisse, L. (2019). Sulfur Dioxide Layer Height Retrieval from Sentinel-5 Precursor/TROPOMI Using FP_ILM. Atmos. Meas. Tech. 12, 5503–5517. doi:10.5194/amt-12-5503-2019
Hess, M., Koepke, P., and Schult, I. (1998). Optical Properties of Aerosols and Clouds: The Software Package OPAC. Bull. Amer. Meteorol. Soc. 79, 831–844. doi:10.1175/1520-0477(1998)079<0831:opoaac>2.0.co;2
Hollstein, A., and Fischer, J. (2014). Retrieving Aerosol Height from the Oxygen a Band: a Fast Forward Operator and Sensitivity Study Concerning Spectral Resolution, Instrumental Noise, and Surface Inhomogeneity. Atmos. Meas. Tech. 7, 1429–1441. doi:10.5194/amt-7-1429-2014
Kleipool, Q., Ludewig, A., Babić, L., Bartstra, R., Braak, R., Dierssen, W., et al. (2018). Pre-launch Calibration Results of the TROPOMI Payload On-Board the Sentinel-5 Precursor Satellite. Atmos. Meas. Tech. 11, 6439–6479. doi:10.5194/amt-11-6439-2018
Kokhanovsky, A. A., Rozanov, V. V., Nauss, T., Reudenbach, C., Daniel, J. S., Miller, H. L., et al. (2006a). The Semianalytical Cloud Retrieval Algorithm for SCIAMACHY I. The Validation. Atmos. Chem. Phys. 6, 1905–1911. doi:10.5194/acp-6-1905-2006
Kokhanovsky, A. A., and Rozanov, V. V. (2010). The Determination of Dust Cloud Altitudes from a Satellite Using Hyperspectral Measurements in the Gaseous Absorption Band. Int. J. Remote Sensing 31, 2729–2744. doi:10.1080/01431160903085644
Kokhanovsky, A. A., von Hoyningen-Huene, W., Rozanov, V. V., Noël, S., Gerilowski, K., Bovensmann, H., et al. (2006b). The Semianalytical Cloud Retrieval Algorithm for SCIAMACHY II. The Application to MERIS and SCIAMACHY Data. Atmos. Chem. Phys. 6, 4129–4136. doi:10.5194/acp-6-4129-2006
Koppers, G. A. A., and Murtagh, D. P. (1997). “Retrieval of Height Resolved Aerosol Optical Thickness in the Atmospheric Band,” in Radiative Transfer in the Absorption Bands of Oxygen: Studies of Their Significance in Ozone Chemistry and Potential for Aerosol Remote Sensing (Stockholm, Sweden: Stockholm University).
Lelli, L., Kokhanovsky, A. A., Rozanov, V. V., Vountas, M., and Burrows, J. P. (2014). Linear Trends in Cloud Top Height from Passive Observations in the Oxygen A-Band. Atmos. Chem. Phys. 14, 5679–5692. doi:10.5194/acp-14-5679-2014
Levy, R. C., Remer, L. A., and Dubovik, O. (2007a). Global Aerosol Optical Properties and Application to Moderate Resolution Imaging Spectroradiometer Aerosol Retrieval over Land. J. Geophys. Res. 112. doi:10.1029/2006JD007815
Levy, R. C., Remer, L. A., Mattoo, S., Vermote, E. F., and Kaufman, Y. J. (2007b). Second-generation Operational Algorithm: Retrieval of Aerosol Properties over Land from Inversion of Moderate Resolution Imaging Spectroradiometer Spectral Reflectance. J. Geophys. Res. Atmos. 112. doi:10.1029/2006JD007811
Li, Z., Zhao, X., Kahn, R., Mishchenko, M., Remer, L., Lee, K.-H., et al. (2009). Uncertainties in Satellite Remote Sensing of Aerosols and Impact on Monitoring its Long-Term Trend: a Review and Perspective. Ann. Geophys. 27, 2755–2770. doi:10.5194/angeo-27-2755-2009
Loyola, D. G., Gimeno García, S., Lutz, R., Argyrouli, A., Romahn, F., Spurr, R. J. D., et al. (2018). The Operational Cloud Retrieval Algorithms from TROPOMI on Board Sentinel-5 Precursor. Atmos. Meas. Tech. 11, 409–427. doi:10.5194/amt-11-409-2018
Loyola, D. G., Xu, J., Heue, K.-P., and Zimmer, W. (2020). Applying FP_ILM to the Retrieval of Geometry-dependent Effective Lambertian Equivalent Reflectivity (GE_LER) Daily Maps from UVN Satellite Measurements. Atmos. Meas. Tech. 13, 985–999. doi:10.5194/amt-13-985-2020
Ludewig, A., Kleipool, Q., Bartstra, R., Landzaat, R., Leloux, J., Loots, E., et al. (2020). In-flight Calibration Results of the TROPOMI Payload on Board the Sentinel-5 Precursor Satellite. Atmos. Meas. Tech. 13, 3561–3580. doi:10.5194/amt-13-3561-2020
Molina García, V., Sasi, S., Efremenko, D. S., Doicu, A., and Loyola, D. (2018a). Linearized Radiative Transfer Models for Retrieval of Cloud Parameters from EPIC/DSCOVR Measurements. J. Quantitative Spectrosc. Radiative Transfer 213, 241–251. doi:10.1016/j.jqsrt.2018.03.008
Molina García, V., Sasi, S., Efremenko, D. S., Doicu, A., and Loyola, D. (2018b). Radiative Transfer Models for Retrieval of Cloud Parameters from EPIC/DSCOVR Measurements. J. Quantitative Spectrosc. Radiative Transfer 213, 228–240. doi:10.1016/j.jqsrt.2018.03.014
Nanda, S., de Graaf, M., Sneep, M., de Haan, J. F., Stammes, P., Sanders, A. F. J., et al. (2018a). Error Sources in the Retrieval of Aerosol Information over Bright Surfaces from Satellite Measurements in the Oxygen A Band. Atmos. Meas. Tech. 11, 161–175. doi:10.5194/amt-11-161-2018
Nanda, S., Veefkind, J. P., de Graaf, M., Sneep, M., Stammes, P., de Haan, J. F., et al. (2018b). A Weighted Least Squares Approach to Retrieve Aerosol Layer Height over Bright Surfaces Applied to GOME-2 Measurements of the Oxygen A Band for forest Fire Cases over Europe. Atmos. Meas. Tech. 11, 3263–3280. doi:10.5194/amt-11-3263-2018
Natraj, V., Jiang, X., Shia, R.-l., Huang, X., Margolis, J. S., and Yung, Y. L. (2005). Application of Principal Component Analysis to High Spectral Resolution Radiative Transfer: A Case Study of the Band. J. Quantitative Spectrosc. Radiative Transfer 95, 539–556. doi:10.1016/j.jqsrt.2004.12.024
Natraj, V., Shia, R.-L., and Yung, Y. L. (2010). On the Use of Principal Component Analysis to Speed up Radiative Transfer Calculations. J. Quantitative Spectrosc. Radiative Transfer 111, 810–816. doi:10.1016/j.jqsrt.2009.11.004
Park, S. S., Kim, J., Lee, H., Torres, O., Lee, K.-M., and Lee, S. D. (2016). Utilization of O4 Slant Column Density to Derive Aerosol Layer Height from a Space-Borne UV-Visible Hyperspectral Sensor: Sensitivity and Case Study. Atmos. Chem. Phys. 16, 1987–2006. doi:10.5194/acp-16-1987-2016
Rao, L., Xu, J., Efremenko, D. S., Loyola, D. G., and Doicu, A. (2021). Optimization of Aerosol Model Selection for TROPOMI/S5P. Remote Sens 13. doi:10.3390/rs13132489
Rodgers, C. (2000). Inverse Methods For Atmospheric Sounding: Theory And Practise. Singapore: World Scientific.
Rodriguez, D. G. L., Thomas, W., Livschitz, Y., Ruppert, T., Albert, P., and Hollmann, R. (2007). Cloud Properties Derived from GOME/ERS-2 Backscatter Data for Trace Gas Retrieval. IEEE Trans. Geosci. Remote Sensing 45, 2747–2758. doi:10.1109/TGRS.2007.901043
Sanders, A. F. J., and de Haan, J. F. (2013). Retrieval of Aerosol Parameters from the Oxygen a Band in the Presence of Chlorophyll Fluorescence. Atmos. Meas. Tech. 6, 2725–2740. doi:10.5194/amt-6-2725-2013
Sanders, A. F. J., de Haan, J. F., Sneep, M., Apituley, A., Stammes, P., Vieitez, M. O., et al. (2015). Evaluation of the Operational Aerosol Layer Height Retrieval Algorithm for Sentinel-5 Precursor: Application to O2 A Band Observations from GOME-2A. Atmos. Meas. Tech. 8, 4947–4977. doi:10.5194/amt-8-4947-2015
Sanghavi, S., Martonchik, J. V., Landgraf, J., and Platt, U. (2012). Retrieval of the Optical Depth and Vertical Distribution of Particulate Scatterers in the Atmosphere Using O2 A- and B-Band SCIAMACHY Observations over Kanpur: a Case Study. Atmos. Meas. Tech. 5, 1099–1119. doi:10.5194/amt-5-1099-2012
Sasi, S., Natraj, V., Molina García, V., Efremenko, D. S., Loyola, D., and Doicu, A. (2020a). Model Selection in Atmospheric Remote Sensing with an Application to Aerosol Retrieval from DSCOVR/EPIC, Part 1: Theory. Remote Sens 12, 3724. doi:10.3390/rs12223724
Sasi, S., Natraj, V., Molina García, V., Efremenko, D. S., Loyola, D., and Doicu, A. (2020b). Model Selection in Atmospheric Remote Sensing with Application to Aerosol Retrieval from DSCOVR/EPIC. Part 2: Numerical Analysis. Remote Sens 12, 3656. doi:10.3390/rs12213656
Seidel, F. C., and Popp, C. (2012). Critical Surface Albedo and its Implications to Aerosol Remote Sensing. Atmos. Meas. Tech. 5, 1653–1665. doi:10.5194/amt-5-1653-2012
Spurr, R. (2008). “LIDORT and VLIDORT. Linearized Pseudo-spherical Scalar and Vector Discrete Ordinate Radiative Transfer Models for Use in Remote Sensing Retrieval Problems,”. Light Scattering Reviews. Editor A. Kokhanovsky (Berlin: Springer-Verlag), 3, 229–271.
Taylor, M., Kazadzis, S., Amiridis, V., and Kahn, R. A. (2015). Global Aerosol Mixtures and Their Multiyear and Seasonal Characteristics. Atmos. Environ. 116, 112–129. doi:10.1016/j.atmosenv.2015.06.029
Thomas, G. E., Poulsen, C. A., Sayer, A. M., Marsh, S. H., Dean, S. M., Carboni, E., et al. (2009). The GRAPE Aerosol Retrieval Algorithm. Atmos. Meas. Tech. 2, 679–701. doi:10.5194/amt-2-679-2009
Tikhonov, A. (1963). On the Solution of Incorrectly Stated Problems and a Method of Regularization. Dokl. Acad. Nauk SSSR 151, 501–504.
Tilstra, L., Tuinder, O., Wang, P., and Stammes, P. (2019). GOME-2 Absorbing Aerosol Height Algorithm Theoretical Basis Document. Tech. rep. De Bilt, Netherlands: Royal Netherlands Meteorological Institute KNMI.
Torres, O., Bhartia, P. K., Herman, J. R., Ahmad, Z., and Gleason, J. (1998). Derivation of Aerosol Properties from Satellite Measurements of Backscattered Ultraviolet Radiation: Theoretical Basis. J. Geophys. Res. 103, 17099–17110. doi:10.1029/98JD00900
Veefkind, J. P., Aben, I., McMullan, K., Förster, H., de Vries, J., Otter, G., et al. (2012). TROPOMI on the ESA Sentinel-5 Precursor: A GMES mission for Global Observations of the Atmospheric Composition for Climate, Air Quality and Ozone Layer Applications. Remote Sensing Environ. 120, 70–83. doi:10.1016/j.rse.2011.09.027
Wang, P., Stammes, P., van der A, R., Pinardi, G., and van Roozendael, M. (2008). FRESCO+: an Improved O2 A-Band Cloud Retrieval Algorithm for Tropospheric Trace Gas Retrievals. Atmos. Chem. Phys. 8, 6565–6576. doi:10.5194/acp-8-6565-2008
Winker, D. M., Vaughan, M. A., Omar, A., Hu, Y., Powell, K. A., Liu, Z., et al. (2009). Overview of the CALIPSO mission and CALIOP Data Processing Algorithms. J. Atmos. Oceanic Techn. 26, 2310–2323. doi:10.1175/2009JTECHA1281.1
Xu, J., Rao, L., Schreier, F., Efremenko, D. S., Doicu, A., and Trautmann, T. (2020). Insight into Construction of Tikhonov-type Regularization for Atmospheric Retrievals. Atmosphere 11, 1052. doi:10.3390/atmos11101052
Xu, J., Schreier, F., Doicu, A., and Trautmann, T. (2016). Assessment of Tikhonov-type Regularization Methods for Solving Atmospheric Inverse Problems. J. Quantitative Spectrosc. Radiative Transfer 184, 274–286. doi:10.1016/j.jqsrt.2016.08.003
Xu, J., Schussler, O., Rodriguez, D. G. L. D., Romahn, F., and Doicu, A. (2017a). A Novel Ozone Profile Shape Retrieval Using Full-Physics Inverse Learning Machine (FP-ILM). IEEE J. Sel. Top. Appl. Earth Observations Remote Sensing 10, 5442–5457. doi:10.1109/JSTARS.2017.2740168
Xu, X., Wang, J., Wang, Y., Zeng, J., Torres, O., Yang, Y., et al. (2017b). Passive Remote Sensing of Altitude and Optical Depth of Dust Plumes Using the Oxygen A and B Bands: First Results from EPIC/DSCOVR at Lagrange-1 point. Geophys. Res. Lett. 44, 7544–7554. doi:10.1002/2017GL073939
Keywords: aerosol retrievals, aerosol layer height, O2 A-band, radiative transfer, TROPOMI/S5P
Citation: Rao L, Xu J, Efremenko DS, Loyola DG and Doicu A (2022) Hyperspectral Satellite Remote Sensing of Aerosol Parameters: Sensitivity Analysis and Application to TROPOMI/S5P. Front. Environ. Sci. 9:770662. doi: 10.3389/fenvs.2021.770662
Received: 04 September 2021; Accepted: 27 December 2021;
Published: 14 January 2022.
Edited by:
Tianjie Zhao, Aerospace Information Research Institute (CAS), ChinaReviewed by:
Husi Letu, Aerospace Information Research Institute (CAS), ChinaYerong Wu, Hunan University of Science and Technology, China
Copyright © 2022 Rao, Xu, Efremenko, Loyola and Doicu. This is an open-access article distributed under the terms of the Creative Commons Attribution License (CC BY). The use, distribution or reproduction in other forums is permitted, provided the original author(s) and the copyright owner(s) are credited and that the original publication in this journal is cited, in accordance with accepted academic practice. No use, distribution or reproduction is permitted which does not comply with these terms.
*Correspondence: Jian Xu, xujian@nssc.ac.cn