- 1Centre for Chronic Disease Control, New Delhi, India
- 2Centre for Health Analytics, Research, and Trends, Trivedi School of Biosciences, Ashoka University, Sonipat, India
Pollutants in ambient air have been linked with type-2 diabetes mellitus (T2DM) in low as well as high pollution scenarios. Mechanisms of action include disruption of endothelial function, imbalance in the autonomic nervous system and direct translocation. Although reductions in exposure to ambient air pollution (AP) could translate to meaningful clinical and public health benefits, policy changes targeting AP are usually at the population level, multisectoral, and time consuming. The human gut microbiome (GM) is an ecosystem within individuals which has been linked with health in both beneficial and detrimental ways. During the last decade, mechanistic and epidemiological research on GM suggests altered microbial diversity and differential composition influencing T2DM through inflammation, metabolites, and microbial functions. This offers a scope to design individual level interventions to target AP related T2DM through GM. In this paper, we presented a combination approach of evidence synthesis by literature review and application of causal inference framework to investigate the role of GM in the association of AP and T2DM, to help design epidemiological studies and direct data analysis. We formulated Directed Acyclic Graphs with methodological considerations for mediatory, interacting, or effect modification role of GM in the association of AP and T2DM. Additionally, we considered the emerging links between gut and oral microbiome, the different T2DM disease patterns in South Asia, and unique co-exposures in these settings (for example, indoor air pollution).
1 Introduction
There are 437 million adults (95% uncertainty 402–477 million) living with type-2 diabetes mellitus (T2DM) globally in 2019 (1) which is projected to rise to 643 million by 2030 and 783 million by 2045 (1, 2). Air pollutants, specifically fine particulate matter (PM) from anthropogenic activities have been shown to be a major risk factor for cardiometabolic diseases including T2DM. While reductions in exposure AP could translate to meaningful clinical and public health outcomes, policy changes targeting AP are usually at the population level and multi-sectoral and thus time consuming. Host-associated microbial diversity, composition, and functions, supported by strong mechanistic evidence from animal models (3, 4) have been associated with beneficial as well as harmful health outcomes in human beings (5). Specifically, human gut microbiome (GM) has been emerging as a novel factor in the pathophysiology of several chronic disease outcomes (6, 7). Given the links between AP influencing unhealthy shifts in the GM and the associations of less healthy shifts in GM with T2DM, GM could be visualized as a mediator, an interacting factor, and/or as an effect modifier in a causal framework linking AP and T2DM (Figure 1A). This evidence, although largely from low AP exposure scenarios from developed country settings, gives rise to the potential of minimizing the harmful effects of AP on T2DM at an individual level by modulating GM or factors that influence it. Furthermore, the interactions between the gut and oral microbiome- the Gut-Oral axis- is gaining evidence (8). The Gut-Oral axis is particularly relevant in T2DM where periodontal disease, a chronic inflammatory disease of the soft tissues and bone of the oral cavity driven by aberrant host response to oral microbial pathogens, is classified as the sixth major complication of T2DM with established bidirectional associations (9).
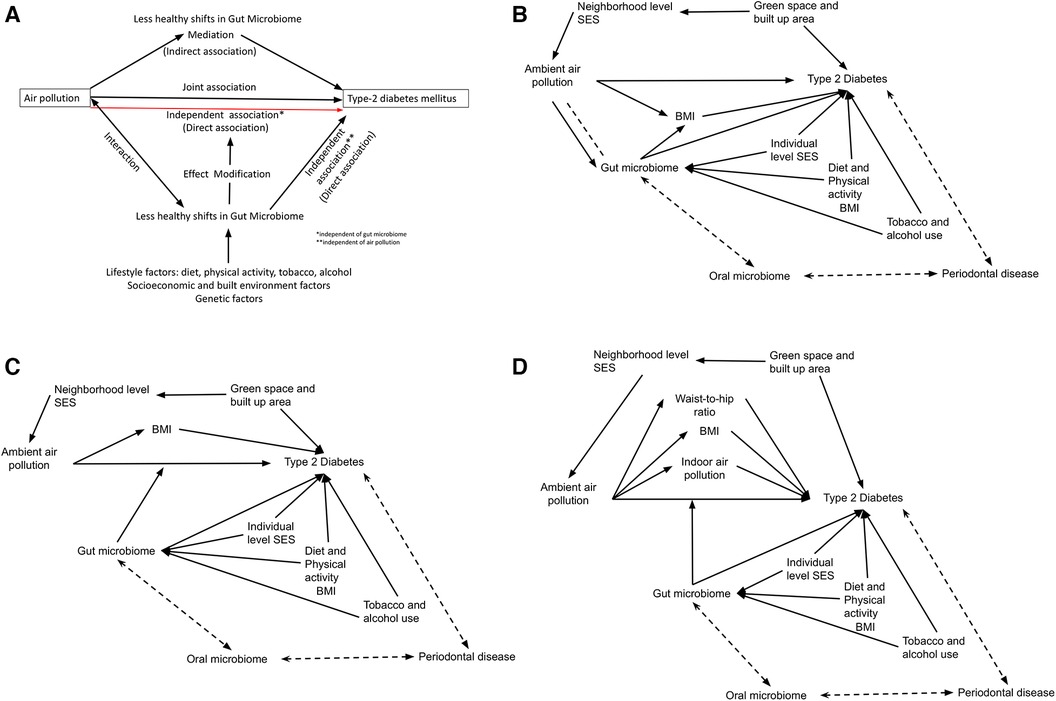
Figure 1 (A) Conceptual diagram for the role of gut microbiome in the associations between ambient air pollution and type-2 diabetes mellitus. Directed acyclic graphs showing the conceptual framework linking ambient air pollution (AP) with Type 2 diabetes (T2D) under three scenarios: (B) Mediation through gut microbiome (GM), (C) effect modification by GM, (D) South Asian scenario with co-exposures, with effect modification by GM. Biological interactions between AP and GM is represented by a dashed line in 1B. Other major confounders for each association are also shown for each main association, including neighborhood and individual level socioeconomic status (SES), green space, diet, physical activity, tobacco and alcohol use. Additionally, the gut-oral-periodontal disease pathway is shown as a bidirectional association.
High levels of ambient air pollution (AP) and a high burden of type-2 diabetes mellitus (T2DM) are two major public health hazards faced by several low- and middle-income countries (LMICs). Asia, with the most populous countries of the world (i.e., China and India), is at the epicenter of T2DM burden accounting for 40% of total global burden. Specifically in India, the prevalence of T2DM increased from 26·0 million in 1990 to 81·0 million in 2019 with an annual rate of change at 2.9% during this period (vs. 1.9% globally and 1.5% in China) (2). The GBD 2019 study reports 14.3% deaths due to T2DM and 15% of disability-adjusted life-years (DALYs) being attributable to AP in Southeast Asia (vs. 13.4% of all deaths and 13.6% of DALYs globally) (10).
The causal links between AP, GM and T2DM are complicated by multiple lifestyle and environmental factors that regulate GM that may confound associations with T2DM, a complex multifactorial disease. This further limit the ability to investigate the precise less healthy shifts in GM that may be causally associated with T2DM (11). The aim of this paper was to address whether GM was a potential modifiable factor in the causal association of AP and T2DM. We synthesized the evidence to date by summarizing systematic reviews and meta-analysis in the selected topic published until December 2023. We quantified the summary findings as appropriate and identified the research gaps. We then applied a causal inference framework, to help design future epidemiological studies and direct data analysis, to investigate the role of GM in the association of AP and T2DM. We utilized the combination approach of “Evidence Synthesis for Constructing Directed Acyclic Graphs” framework, proposed by Ferguson et al., in 2020 (12).
2 Methods
2.1 Evidence synthesis
First, using PubMed searches and limiting the keywords to the exposure and outcome we summarized the published systematic reviews and meta-analysis for the association between AP and T2DM stratified by high and low pollution scenarios. We also summarized systematic reviews for the association between AP and GM, and GM and T2DM. We extracted information on exposures, outcome, summary estimates of associations, and corresponding 95% confidence interval (CI), and heterogeneity measure, and covariates or confounders assessments. Second, we summarized all published evidence (reviews and original studies) investigating the mediating role of GM in the association between AP and T2DM with information on exposures, outcome, magnitude of associations, and covariates or confounders assessments. Further, for meta-analytical reviews on AP and T2DM, the effect estimates and CIs were converted to percentage excess risk of odds ratio (OR)/relative risk (RR)/hazard ratio (HR); % excess risk = (OR/HR/RR − 1) × 100 per 10 μg/m3 increase of AP (13). For meta-analytical reviews on GM and T2DM, we summarized the meta-analytic mean difference of the outcome. For all systematic reviews, we summarized the statistically significant associations. Overall, we classified the strength of the evidence as sufficiently conclusive (consistent positive association in ≥50% of meta-analysis), limited positive (consistent positive association <50% of meta-analysis or dose-response evidence in systematic review), limited suggestive (consistent positive association in original studies) or no association.
2.2 Constructing directed acyclic graphs
Directed acyclic graphs (DAGs), a causal inference method, are diagrammatic representation of causal relationships between exposure, outcome, and co-factors based on evidence in the existing literature. DAGs help to distinguish the types of factors such as mediators (factors associated with both exposure and outcome in the causal pathway), confounders (factors associated with both exposure and outcome but not in the causal pathway), and colliders (factors caused by exposure and outcome independently), and identify an appropriate adjustment set for confounders, to direct data analysis (14–16). We formulated DAGs to design studies to investigate the role of GM in the association of AP and T2DM. We mapped exposure (AP) and outcome (T2DM) with all reported covariates in the literature; translated the information after considering temporality, plausibility of associations, and the strength of the supporting evidence and integrated the evidence to synthesise DAGs. The methodological considerations for the role of GM in the association of AP and T2DM included mediation (i.e., GM is the intermediate factor in the causal pathway between AP and T2DM) or effect modification (i.e., GM in a subgroup influences the association between AP and T2DM) or interaction (i.e., joint association of GM and AP with T2DM) (15, 16). Additionally, we considered the emerging links between gut and oral microbiome, the different T2DM disease patterns in South Asia, and unique co-exposures in these settings (for example, indoor air pollution).
3 Results
3.1 Evidence synthesis
3.1.1 Ambient air pollution and type-2 diabetes mellitus
A PubMed search using the keywords “air pollution” AND “diabetes mellitus” in combination with filter for review and manual searches of the reference list showed a result of 10 meta-analysis (2014–2023), 2 systematic reviews (2014–2018), and 10 reviews (2018–2021). Published systematic reviews (n = 2) (17, 18) and meta-analysis (n = 9; full-text was unavailable for one) (19–27) examining the associations between AP and T2DM are summarized in Supplementary Table S1 by low- and high-pollution scenarios. Exposure to various air pollutants, including particulate matter [PM2.5, PM10, elemental carbon (EC), Black Carbon (BC)], and gaseous pollutants such as O3 (ozone), CO (carbon monoxide), SO2 (sulphur dioxide), NO/NO2 (nitrogen oxides), and traffic-related pollutants, have been investigated with incident or prevalent T2DM. Evidence linking AP with T2DM mostly arises from developed countries with low levels of AP (for example, with annual mean of PM2.5: 7.7–28.5 μg/m3 vs. 33.7–147.0 μg/m3 in developing countries). Percent excess risk for long-term AP exposures on incident T2DM ranged between 10.0 (02.0, 18.0) and 39.0 (14.0, 68.0) for PM2.5; 11.0 (00.0, 22.0) and 34.0 (22.0, 47.0) for PM10; and 11.0 (07.0–16.0) and 13.0 (04.0, 22.0) for nitrogen oxides. The evidence was sufficiently conclusive for temporal associations of PM2.5 (n = 7 meta-analysis) but limited for PM10 and nitrogen oxides (n = 2 meta-analysis). While the associations weakened for prevalent T2DM, it became insignificant for T2DM mortality. The evidence for long-term exposure for other pollutants were inconclusive. Short-term exposures to PM2.5 was associated with 6%–49% increase in diabetes related mortality and 1%–3% increase in hospitalizations per 10 μg/m3 (22, 27). A detailed set of confounders and effect modifiers reported in these studies are summarized in Supplementary Table S1.
The plausible mechanisms underlying the association between AP and T2DM involve chronic inflammation, and insulin resistance, but also oxidative stress, alterations in insulin signaling, dysregulated glucose metabolism, and beta (β)-cell function (25–27). The exposure-response function of AP with chronic diseases indicates a steep increase in risk from 0 to 50 µg/m3 and a subsequent plateauing or non-linear associations (28). Although the number of studies at higher levels were much fewer than those at lower levels, systematic evidence suggests similar magnitude of associations between low- and high-pollution regions (26).
3.1.2 Indoor air pollution and type-2 diabetes mellitus
Long-term exposure to indoor air pollution (IAP) has similar effects as ambient AP, wherein a 6.5% of T2DM deaths and 5.9% of DALYs were attributed to household PM2.5 (10). Nevertheless, while the age-standardized rate of DALYs due to ambient air pollution increased by over 85%, it decreased by 37.9% for IAP during this period. This is found to affect the elderly, women and people of low social status disproportionately (29, 30). For example, long-duration cooking with solid fuel was associated with decreased insulin level among women, which was more pronounced among women of low monthly income (30). According to the Health Effect Institute, long-term exposure to IAP through household burning of solid fuels for cooking contributed to 2.31 million deaths (4% of total global deaths) and 91.5 million DALYs in 2019 (31). Nearly 95% of this burden is from South Asia, Sub-saharan Africa, Southeast Asia, East Asia and Oceania Asia (31).The prevalence of solid fuel use for cooking in these regions is as high as >30%–95%, although there is considerable decrease over time (31).
3.1.3 Gut microbiome and type-2 diabetes mellitus
A PubMed search using the keywords “gut microbiome” OR “gastrointestinal microbiome” AND “diabetes mellitus” in combination with filter for review and manual searches of the reference list revealed 75 review papers (2011–2023) and eight systematic reviews and meta-analysis (2018–2023). Published systematic reviews (N = 4; full-text unavailable for one) (32–35) and meta-analysis (N = 3) (36–38) examining the associations between GM and T2DM are summarized in Supplementary Table S2. Limited but high-quality randomized control trials (RCTs; n = 2 meta-analysis) in diabetic patients showed dietary intervention with high-fibre diet and probiotics Lactobacillus sp., Bifidobacterium sp. and Actinoplanes lowered glycated hemoglobin levels (HbA1c) and improved insulin sensitivity. Moderate quality RCTs (n = 2 meta-analysis) also showed that dietary intervention with low-fat, low-carbohydrate, and high-fibre diets lowered fasting blood glucose and HbA1c levels by increasing diversity and abundances of beneficial bacteria such as Roseburia, Ruminococcus, Lactobacillus, Bacteroides, Lachnospira, Fusobacterium prausnitzii, Eubacterium rectale, Akkermansia muciniphilia. Further, limited but suggestive evidence from mechanistic studies in animals indicated probiotic supplementation with Bacteroides sp. reduced insulin resistance (n = 1 meta-analysis). However, the findings from observational studies yielded mixed results with conflicting reports on specific bacterial species (n = 2 meta-analysis). The set of covariates assessments reported in these studies are summarized in Supplementary Table S2. The plausible mechanisms include modulating inflammation, interactions with dietary components, influencing gut permeability, glucose and lipid metabolism, insulin sensitivity and thus the overall energy homeostasis (32, 35). Standard definitions of microbial features described in this paper are outlined in Supplementary Figure S1.
3.1.4 Gut-oral axis in T2DM
The bidirectional association between oral diseases, specifically the periodontal disease, and T2DM is well established and periodontal disease is classified as a major sixth complication of T2DM (9, 39, 40) Systematic reviews and meta-analysis of epidemiological studies strongly support the bi-directional relationship of periodontal disease and T2DM, leading to the formulation of European Federation of Periodontology/American Academy of Periodontology (EFP/AAP, 2013) and the EFP and the International Diabetes Federation consensus reports (2018). The consensus statements include (1) poor glycemic control is associated with poorer periodontal outcomes. (2) periodontal disease is associated with hyperglycemia and increased insulin resistance in diabetes, increased risk for incident diabetes and diabetic complications such as retinopathy, neuropathy, and cardiovascular complications (OR: 1.2–6.6) and mortality (HR: 3.5–4.5). Periodontal therapy improves serum HbA1C levels (0.27%–0.48% after 3 months) (39, 40). The suggested mechanistic pathways for these associations include shared disease progression through chronic inflammation, acetylated glycated end-products, and oral pathogens (9). Periodontal pathogens such as Tanerella forsythia, Porphyromonas gingivalis, Fusobacterium nucleatum, Aggregatibacter actinomycetemcomitans are few well studied oral microbiome (41). The concept of enteral and systemic entry of oral pathogens into the gastrointestinal tract and the interaction between oral and gut microbiome has plausible mechanistic evidence (8). The gut-oral axis contributing to the disease progression through shared pathways of chronic inflammation and dysregulated metabolic factors is rapidly emerging and strongly suggestive for T2DM (8).
3.1.5 Air pollution and gut microbiome
A PubMed search using the keywords “air pollution” AND “gut microbiome” OR “gastrointestinal microbiome” in combination with filter for review revealed 1 systematic review (42) including human studies (N = 12) and animal studies (N = 36) and five review papers (2017–2020). The findings of the systematic review are shown in Good quality human studies across the life-course strongly indicated lower gut diversity and shifts in GM composition with dose-response function for PM exposure (average exposure in studies ranged from 8.4 μg/m3–102 μg/m3) (42). Prenatal and post-natal black carbon exposure explained 6%–17% of bacterial diversity among healthy children. Nitrogen oxides and ozone exposures explained 4%–11% variation of GM composition. These have also been linked to lower gut microbial diversity, gene functional pathways related to insulin release (43). AP exposure increased Porphyromonadaceae, Mucisprillum, and Campylobacter, and decreased Akkermansia muciniphila. While animal studies supported the findings in general, the reported bacterial taxa were different. Plausible biological mechanisms include increased gut damage, inflammation, oxidative stress, and gut permeability. The set of covariates assessments reported in these studies are summarized in Supplementary Table S3.
3.1.6 Air pollution, gut microbiome, and type-2 diabetes mellitus
Studies examining the role of GM in the associations between AP and T2DM are very limited to date (Supplementary Table S4). A PubMed search using the keywords (“air pollution”[MeSH Terms] OR (“air”[All Fields] AND “pollution”[All Fields]) OR “air pollution”[All Fields]) AND (“gastrointestinal microbiome”[MeSH Terms] OR (“gastrointestinal”[All Fields] AND “microbiome”[All Fields]) OR “gastrointestinal microbiome”[All Fields] OR (“gut”[All Fields] AND “microbiome”[All Fields]) OR “gut microbiome”[All Fields]) AND (“diabete”[All Fields] OR “diabetes mellitus”[MeSH Terms] OR (“diabetes”[All Fields] AND “mellitus”[All Fields]) OR “diabetes mellitus”[All Fields] OR “diabetes”[All Fields] OR “diabetic”[All Fields] OR “diabetics”[All Fields]) revealed a review of five animal studies (2013–2018) and three human studies (2015–2018) (44) and an additional two original research articles (45, 46). Limited evidence from prospective and population based studies from high pollution regions suggest a 4.3–37.8% of associations between PM exposure and impaired glucose metabolism and T2DM mediated through GM either directly or via lipid metabolism. Specifically, the abundance of Oribacterium and Shuttleworthia, reduction in Firmicutes, Proteobacteria, and Verrucomicrobia might explain the correlations between AP and T2DM (45, 46). Mechanistic evidence supports inflammatory pathway for the role of GM in the associations between AP and T2DM. The set of covariates assessments reported in these studies are summarized in Supplementary Table S4.
3.1.7 Pathophysiology of diabetes in South Asians
South Asian populations show certain distinct T2DM disease patterns compared to the Western populations and possibly to other Asian populations in the East and South-East Asia (47–52). For instance, systematic evidence reveals that South Asians exhibit a high prevalence of early-onset T2DM (≤55 years of age) and a high prevalence of T2DM at a lower BMI. They also have also have a higher risk for microvascular complications, possibly associated with earlier mortality compared to Western populations (47, 50). Different body fat distribution and lipid metabolism coupled with dysregulated metabolic pathways (i.e., poor insulin secretion and beta-cell dysfunction) are suggested to explain, at least in part, these differences in disease patterns in South Asians (47, 51). South Asians tend to have a low lean mass, visceral (abdominal) and ectopic (in skeletal muscles and liver) adiposity leading to elevated triglycerides and possibly a pro-inflammatory high-density lipoprotein cholesterol (47, 53). Certain lifestyle factors (diet rich in saturated fat and low in omega-3 fatty acid with sedentary activity), genetic susceptibility (e.g., family history, specific genetic variants with high heritability) and evolutionary biology (historical undernourishment, low lean mass, visceral and ectopic adiposity) are suggested to influence this pattern among South Asians (47, 50, 51).
3.2 Directed acyclic graph (DAG) approach
Table 1 summarizes the strength of evidence for the causal associations between AP-T2DM, GM-T2DM, AP-GM, and AP-GM-T2DM, with the reported set of confounders and effect modifiers. Interacting factors in any of these associations are little explored. (i) Considering causal associations of AP with T2DM and GM with T2DM wherein both AP and GM can be considered as independent exposures (i.e., independent of each other's influence) against T2DM as the outcome—GM could be an interacting factor in the association between AP and T2DM (i.e., joint effect of the causal effects of AP and GM on T2DM that could be more than the sum or product of their individual effects 14–16) (Figure 1B) (ii) Considering causal associations of AP with T2DM and GM with T2DM and moderate causal associations of AP with GM—GM could be a mediator in the association between AP and T2DM (i.e., AP exposure affecting GM in an intermediate pathway resulting in T2DM outcome 14–16) (Figure 1B). (iii) Epidemiological evidence indicates inequities in the health effect of AP, where individuals belonging to lower socioeconomic groups or vulnerable communities bear a larger impact from AP (54, 55). These same population subgroups are also likely to have dietary patterns or other lifestyle practices that promote less healthy GM shifts, higher adiposity and higher burden of cardiometabolic diseases (56). Thus, this could be hypothesized as an effect modification pathway wherein different levels of shifts in GM can influence variations in the causal associations of AP with T2DM 14–16) (Figure 1C). Further, in case of highly polluted LMIC settings, one needs to account for co-exposures such as indoor air pollution and pathophysiological characteristics such as low body mass index, but high visceral fat, related reduced beta cell dysfunction (Figure 1D). Further, indoor air pollution in LMICs is often affected by ambient levels due to open windows and lack of insulation, which creates a potential mediation pathway (ambient to indoor to outcome). Hence if the main exposure of the interest is ambient AP, indoor AP might be considered as a mediator. In contrast if indoor AP is the main exposure of interest, ambient AP needs to be treated as a confounder.
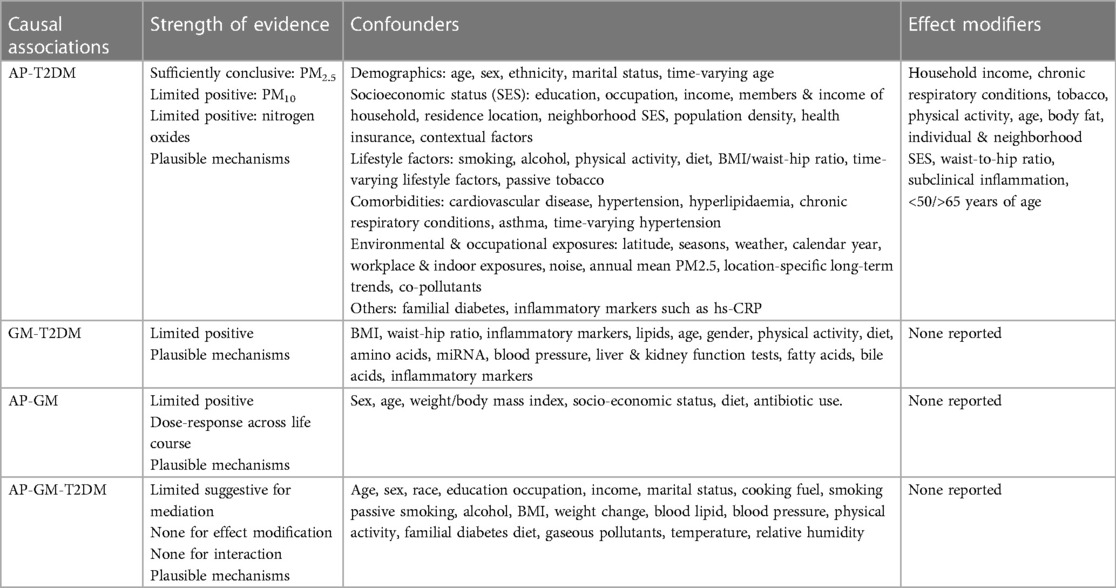
Table 1 Summary of evidence synthesis for ambient air pollution (AP), gut microbiome (GM) and type-2 diabetes mellitus (T2DM).
3.2.1 Quantitative study designs
Among commonly used study designs, a cross-sectional or a longitudinal design can be used to study these associations, depending on whether T2DM was assessed once or multiple times over time. In addition, AP could be treated as a time-varying exposure and GM could be assessed at one or more time points. Within a cross-sectional design, to evaluate the potential role of GM in the epidemiological studies for the association of AP with T2DM, we would recommend first testing for mediation through GM if any, while adjusting for an appropriate set of neighborhood level and individual factors. Mediation analysis refers to a statistical analysis that assesses the amount of association between the exposure and outcome that is mediated through a mediator (57). We recommend using a causal mediation analysis with a counterfactual approach over a traditional mediation analysis (58, 59). This approach provides a segregation of the total effect of exposure (joint association) on outcome into direct (independent association) and indirect effects (mediated association). In case of mediation over time, recent approaches using two generalized mixed effect models for the mediator and the outcome can be used (60). If the joint or mediated associations are small in magnitude as well as statistically insignificant, we would recommend testing for effect modification, by stratifying across quartiles of microbial diversity and testing for significant interaction between AP and microbial diversity. For ease of exposition, we are considering “GM diversity” as a representative variable of GM composition as evidence on specific shifts in the composition is sparse. It is to be noted here, that for all the analyses described above, other confounders need to be appropriately controlled to avoid bias in estimations.
Propensity score-based methods can be utilized to account for measured confounders within each of the above approaches (61). If we consider AP or GM as the exposure, these can be modeled against measured confounders to construct scores that indicate the probability of being exposed given the confounders. Since AP (for e.g., annual average PM2.5) and GM (for e.g., microbial alpha diversity) are inherently continuous variables, generalized propensity scores may be constructed and participants can either be a) weighted using an inverse of the scores or b) be matched based on deciles of these scores (62).
4 Conclusion
In this review, we have discussed the potential role of GM in the pathways linking ambient air pollution and T2DM, and which could lead to interventions or strategies at an individual level to reduce the risk of AP attributable T2DM. We have also discussed the causal inference framework to investigate the role of GM in the association of AP and T2DM, to help design studies and direct data analysis. We observed “sufficient evidence” linking AP to T2DM (10-39% excess risk for different pollutants for T2DM incidence), and ‘limited yet positive evidence’ linking AP with GM (4-17% of GM variation explained by different pollutants with dose-response). Further, although the evidence linking GM with T2DM is less quantified, it is supported through experimental studies. However, the evidence linking AP, GM and T2DM is at a nascent stage with five studies conducted with human participants and conflicting information on specific targetable GM composition influencing these associations. Studies investigating these links between AP, GM and T2DM in large cohort studies, particularly in LMICs would be of great clinical and public health significance, given the large burden of T2DM, diversity in exposure and unique pathophysiology of the population. Leveraging existing cohort studies with comprehensive repeated information on sociodemographic, lifestyle and environmental factors, to incorporate the aspect of GM would be advantageous if AP exposures are available at fine spatiotemporal resolution. Findings from such studies could facilitate mechanistic studies to identify specific unhealthy GM shifts and to understand the progression of T2DM starting from non-diabetic to pre-diabetics to T2DM. These findings could inform potential interventions to tackle the high burden of T2DM, a significant portion of which is attributable to AP.
Author contributions
SM: Conceptualization, Methodology, Visualization, Writing – original draft, Writing – review & editing. SK: Data curation, Formal Analysis, Investigation, Software, Writing – review & editing. KS: Conceptualization, Investigation, Writing – original draft, Writing – review & editing.
Funding
The author(s) declare financial support was received for the research, authorship, and/or publication of this article.
KS was awarded the Yusuf Hamied Faculty Fellowship at the Columbia Mailman School of Public Health, New York, USA (Year: 2020). SM's work was partly supported by the Fogarty International Center of the National Institutes of Health, National Institute of Environmental Health Sciences and National Cancer Institute, under the GEOHealth HUB research grant (Award Number U01 TW010097).
Conflict of interest
The authors declare that the research was conducted in the absence of any commercial or financial relationships that could be construed as a potential conflict of interest.
Publisher's note
All claims expressed in this article are solely those of the authors and do not necessarily represent those of their affiliated organizations, or those of the publisher, the editors and the reviewers. Any product that may be evaluated in this article, or claim that may be made by its manufacturer, is not guaranteed or endorsed by the publisher.
Supplementary material
The Supplementary Material for this article can be found online at: https://www.frontiersin.org/articles/10.3389/fenvh.2024.1339674/full#supplementary-material
References
1. International Diabetes Federation. Diabetes facts & figures. (2021). Available online at: https://idf.org/aboutdiabetes/what-is-diabetes/facts-figures.html (Accessed August 6, 2023)
2. Global Burden of Disease Collaborative Network. Global Burden of Disease Study 2019 (GBD 2019) Results. Seattle, United States: Institute for Health Metrics and Evaluation (IHME) (2020). Available online at: https://vizhub.healthdata.org/gbd-results/
3. Ridaura VK, Faith JJ, Rey FE, Cheng J, Duncan AE, Kau AL, et al. Gut microbiota from twins discordant for obesity modulate metabolism in mice. Science. (2013) 341(6150):1241214. doi: 10.1126/science.1241214
4. Campbell SC, Wisniewski PJ, Noji M, McGuinness LR, Häggblom MM, Lightfoot SA, et al. The effect of diet and exercise on intestinal integrity and microbial diversity in mice. PloS one. (2016) 11(3):e0150502. doi: 10.1371/journal.pone.0150502
5. Walker RL, Vlamakis H, Lee JW, Besse LA, Xanthakis V, Vasan RS, et al. Population study of the gut microbiome: associations with diet, lifestyle, and cardiometabolic disease. Genome medicine. (2021) 13:1–6. doi: 10.1186/s13073-020-00808-4
6. Shreiner AB, Kao JY, Young VB. The gut microbiome in health and in disease. Curr Opin Gastroenterol. (2015) 31(1):69. doi: 10.1097/MOG.0000000000000139
7. Lynch SV, Pedersen O. The human intestinal microbiome in health and disease. N Engl J Med. (2016) 375(24):2369–79. doi: 10.1056/NEJMra1600266
8. Tan X, Wang Y, Gong T. The interplay between oral microbiota, gut microbiota and systematic diseases. J Oral Microbiol. (2023) 15(1):2213112. doi: 10.1080/20002297.2023.2213112
9. Polak D, Shapira L. An update on the evidence for pathogenic mechanisms that may link periodontitis and diabetes. J Clin Periodontol. (2018) 45(2):150–66. doi: 10.1111/jcpe.12803
10. Burkart K, Causey K, Cohen AJ, Wozniak SS, Salvi DD, Abbafati C, et al. Estimates, trends, and drivers of the global burden of type 2 diabetes attributable to PM2·5 air pollution, 1990–2019: an analysis of data from the global burden of disease study 2019. Lancet Planet Health. (2022) 6(7):e586–600. doi: 10.1016/S2542-5196(22)00122-X
11. Ahn J, Hayes RB. Environmental influences on the human microbiome and implications for noncommunicable disease. Annu Rev Public Health. (2021) 42:277–92. doi: 10.1146/annurev-publhealth-012420-105020
12. Ferguson KD, McCann M, Katikireddi SV, Thomson H, Green MJ, Smith DJ, et al. Evidence synthesis for constructing directed acyclic graphs (ESC-DAGs): a novel and systematic method for building directed acyclic graphs. Int J Epidemiol. (2020) 49(1):322–9. Erratum in: Int J Epidemiol. 2020 Feb 1;49(1):353. doi: 10.1093/ije/dyz150
13. de Bont J, Jaganathan S, Dahlquist M, Persson Å, Stafoggia M, Ljungman P. Ambient air pollution and cardiovascular diseases: an umbrella review of systematic reviews and meta-analyses. J Intern Med. (2022) 291(6):779–800. doi: 10.1111/joim.13467
14. Tennant PWG, Murray EJ, Arnold KF, Berrie L, Fox MP, Gadd SC, et al. Use of directed acyclic graphs (DAGs) to identify confounders in applied health research: review and recommendations. Int J Epidemiol. (2021) 50(2):620–32. doi: 10.1093/ije/dyaa213
15. Bours MJL. Using mediators to understand effect modification and interaction. J Clin Epidemiol. (2023) 163:117–21. doi: 10.1016/j.jclinepi.2023.09.005
16. Corraini P, Olsen M, Pedersen L, Dekkers OM, Vandenbroucke JP. Effect modification, interaction and mediation: an overview of theoretical insights for clinical investigators. Clin Epidemiol. (2017) 9:331–8. Erratum in: Clin Epidemiol. 2018 Mar 01;10:223. Erratum in: Clin Epidemiol. 2019 Apr 09;11:245. doi: 10.2147/CLEP.S129728
17. Jaganathan S, Jaacks LM, Magsumbol M, Walia GK, Sieber NL, Shivasankar R, et al. Association of long-term exposure to fine particulate matter and cardio-metabolic diseases in low- and middle-income countries: a systematic review. Int J Environ Res Public Health. (2019) 16(14):2541. doi: 10.3390/ijerph16142541
18. Dendup T, Feng X, Clingan S, Astell-Burt T. Environmental risk factors for developing type 2 diabetes mellitus: a systematic review. Int J Environ Res Public Health. (2018) 15(1):78. doi: 10.3390/ijerph1501007 8.29304014
19. He D, Wu S, Zhao H, Qiu H, Fu Y, Li X, et al. Association between particulate matter 2.5 and diabetes mellitus: a meta-analysis of cohort studies. J Diabetes Investig. (2017) 8(5):687–96. doi: 10.1111/jdi.12631
20. Eze IC, Hemkens LG, Bucher HC, Hoffmann B, Schindler C, Künzli N, et al. Association between ambient air pollution and diabetes mellitus in Europe and North America: systematic review and meta-analysis. Environ Health Perspect. (2015) 123(5):381–9. doi: 10.1289/ehp.1307823
21. Balti EV, Echouffo-Tcheugui JB, Yako YY, Kengne AP. Air pollution and risk of type 2 diabetes mellitus: a systematic review and meta-analysis. Diabetes Res Clin Pract. (2014) 106(2):161–72. doi: 10.1016/j.diabres.2014.08.010
22. Park SK, Wang W. Ambient air pollution and type 2 diabetes: a systematic review of epidemiologic research. Curr Environ Health Rep. (2014) 1(3):275–86. doi: 10.1007/s40572-014-0017-9
23. Wang B, Xu D, Jing Z, Liu D, Yan S, Wang Y. Effect of long-term exposure to air pollution on type 2 diabetes mellitus risk: a systemic review and meta-analysis of cohort studies. Eur J Endocrinol. (2014) 171(5):R173–82. doi: 10.1530/EJE-14-0365
24. Janghorbani M, Momeni F, Mansourian M. Systematic review and metaanalysis of air pollution exposure and risk of diabetes. Eur J Epidemiol. (2014) 29(4):231–42. doi: 10.1007/s10654-014-9907-2
25. Kutlar Joss M, Boogaard H, Samoli E, Patton AP, Atkinson R, Brook J, et al. Long-term exposure to traffic-related air pollution and diabetes: a systematic review and meta-analysis. Int J Public Health. (2023) 68:1605718. doi: 10.3389/ijph.2023.1605718
26. Liu F, Chen G, Huo W, Wang C, Liu S, Li N, et al. Associations between long-term exposure to ambient air pollution and risk of type 2 diabetes mellitus: a systematic review and meta-analysis. Environ Pollut. (2019) 252(Pt B):1235–45. doi: 10.1016/j.envpol.2019.06.033
27. Yang BY, Fan S, Thiering E, Seissler J, Nowak D, Dong GH, et al. Ambient air pollution and diabetes: a systematic review and meta-analysis. Environ Res. (2020) 180:108817. doi: 10.1016/j.Envres.2019.108817
28. Mandal S, Jaganathan S, Kondal D, Schwartz JD, Tandon N, Mohan V, et al. PM2.5 exposure, glycemic markers and incidence of type 2 diabetes in two large Indian cities. BMJ Open Diabetes Res Care. (2023) 11(5):e003333. doi: 10.1136/bmjdrc-2023-003333
29. Wang K, Zhang Y, Wang Y, Liu J, Zhou P, Yuan Y, et al. Secular trends in global burden of diabetes attributable to particulate matter pollution from 1990 to 2019. Environ Sci Pollut Res Int. (2022) 29(35):52844–56. doi: 10.1007/s11356-022-19510-6
30. Kang N, Song X, Zhang C, Li R, Yuchi Y, Liao W, et al. Association of household air pollution with glucose homeostasis markers in Chinese rural women: effect modification of socioeconomic status. Ecotoxicol Environ Saf. (2022) 248:114283. doi: 10.1016/j.ecoenv.2022.114283
31. Health Effects Institute. State of Global Air. Special Report. Boston, MA: Health Effects Institute (2020). Available online at: https://www.stateofglobalair.org/ (Accessed August 9, 2023)
32. Sadagopan A, Mahmoud A, Begg M, Tarhuni M, Fotso M, Gonzalez NA, et al. Understanding the role of the gut microbiome in diabetes and therapeutics targeting leaky gut: a systematic review. Cureus. (2023) 15(7):e41559. doi: 10.7759/cureus.41559
33. Letchumanan G, Abdullah N, Marlini M, Baharom N, Lawley B, Omar MR, et al. Gut microbiota composition in prediabetes and newly diagnosed type 2 diabetes: a systematic review of observational studies. Front Cell Infect Microbiol. (2022) 12:943427. doi: 10.3389/fcimb.2022.943427
34. Wagenaar CA, van de Put M, Bisschops M, Walrabenstein W, de Jonge CS, Herrema H, et al. The effect of dietary interventions on chronic inflammatory diseases in relation to the microbiome: a systematic review. Nutrients. (2021) 13(9):3208. doi: 10.3390/nu13093208
35. Umirah F, Neoh CF, Ramasamy K, Lim SM. Differential gut microbiota composition between type 2 diabetes mellitus patients and healthy controls: a systematic review. Diabetes Res Clin Pract. (2021) 173:108689. doi: 10.1016/j.diabres.2021.108689
36. Proença IM, Allegretti JR, Bernardo WM, de Moura DTH, Ponte Neto AM, Matsubayashi CO, et al. Fecal microbiota transplantation improves metabolic syndrome parameters: systematic review with meta-analysis based on randomized clinical trials. Nutr Res. (2020) 83:1–14. doi: 10.1016/j.nutres.2020.06.018
37. Ojo O, Feng QQ, Ojo OO, Wang XH. The role of dietary fibre in modulating gut Microbiota dysbiosis in patients with type 2 diabetes: a systematic review and meta-analysis of randomised controlled trials. Nutrients. (2020) 12(11):3239. doi: 10.3390/nu12113239
38. Löe H. Periodontal disease. The sixth complication of diabetes mellitus. Diabetes Care. (1993) 16(1):329–34. doi: 10.2337/diacare.16.1.329
39. Chapple ILC, Genco R, Working Group 2 of the joint EFP/AAP Workshop. Diabetes and periodontal diseases: consensus report of the joint EFP/AAP workshop on periodontitis and systemic diseases. J Periodontol. (2013) 84(4 Suppl):S106–12. doi: 10.1902/jop.2013.1340011
40. Sanz M, Ceriello A, Buysschaert M, Chapple I, Demmer RT, Graziani F, et al. Scientific evidence on the links between periodontal diseases and diabetes: consensus report and guidelines of the joint workshop on periodontal diseases and diabetes by the international diabetes federation and the European federation of periodontology. J Clin Periodontol. (2018) 45(2):138–49. doi: 10.1111/jcpe.12808
41. Graves DT, Corrêa JD, Silva TA. The oral microbiota is modified by systemic diseases. J Dent Res. (2019) 98(2):148–56. doi: 10.1177/0022034518805739
42. Van Pee T, Nawrot TS, van Leeuwen R, Hogervorst J. Ambient particulate air pollution and the intestinal microbiome; a systematic review of epidemiological, in vivo and, in vitro studies. Sci Total Environ. (2023) 878:162769. doi: 10.1016/j.scitotenv.2023.162769
43. Fouladi F, Bailey MJ, Patterson WB, Sioda M, Blakley IC, Fodor AA, et al. Air pollution exposure is associated with the gut microbiome as revealed by shotgun metagenomic sequencing. Environ Int. (2020) 138:105604. doi: 10.1016/j.envint.2020.105604
44. Bailey MJ, Naik NN, Wild LE, Patterson WB, Alderete TL. Exposure to air pollutants and the gut microbiota: a potential link between exposure, obesity, and type 2 diabetes. Gut Microbes. (2020) 11(5):1188–202. doi: 10.1080/19490976.2020.1749754
45. Zhao L, Fang J, Tang S, Deng F, Liu X, Shen Y, et al. PM2.5 and serum metabolome and insulin resistance, potential mediation by the gut microbiome: a population-based panel study of older adults in China. Environ Health Perspect. (2022) 130(2):27007. doi: 10.1289/EHP9688
46. Liu T, Chen X, Xu Y, Wu W, Tang W, Chen Z, et al. Gut microbiota partially mediates the effects of fine particulate matter on type 2 diabetes: evidence from a population-based epidemiological study. Environ Int. (2019) 130:104882. doi: 10.1016/j.envint.2019.05.076
47. Narayan KA-O, Kanaya AA-O. Why are South Asians prone to type 2 diabetes? A hypothesis based on underexplored pathways. Diabetologia. (2020) 63(6):1103–9. doi: 10.1007/s00125-020-05132-5
48. Yoon K-H, Lee J-H, Kim J-W, Cho JH, Choi Y-H, Ko S-H, et al. Epidemic obesity and type 2 diabetes in Asia. Lancet (Lond). (2006) 368(9548):1681–8. doi: 10.1016/S0140-6736(06)69703-1
49. Unnikrishnan R, Gupta PK, Mohan V. Diabetes in South Asians: phenotype, clinical presentation, and natural history. Curr Diab Rep. (2018) 18(6):30. doi: 10.1007/s11892-018-1002-8
50. Seah JYH, Sim X, Khoo CM, Tai ES, van Dam RM. Share differences in type 2 diabetes risk between East, South, and Southeast Asians living in Singapore: the multi-ethnic cohort. BMJ Open Diabetes Res Care. (2023) 11(4):e003385. doi: 10.1136/bmjdrc-2023-003385
51. Srinivasan S, Liju S, Sathish N, Siddiqui MK, Anjana RM, Pearson ER, et al. Common and distinct genetic architecture of age at diagnosis of diabetes in south Indian and European populations. Diabetes Care. (2023) 46(8):1515–23. doi: 10.2337/dc23-0243
52. Siddiqui MK, Anjana RM, Dawed AY, Martoeau C, Srinivasan S, Saravanan J, et al. Young-onset diabetes in Asian Indians is associated with lower measured and genetically determined beta cell function. Diabetologia. (2022) 65(6):973–83. doi: 10.1007/s00125-022-05671-z
53. Dodani S, Kaeley GS, Arora S, Reddy ST. Coronary artery disease in high risk South Asian immigrants: role of dysfunctional HDL in risk prediction. Indian Heart J. (2017) 69(5):658–9. doi: 10.1016/j.ihj.2017.07.014
54. Sengupta S, Thakrar SK, Singh K, Tongia R, Hill JD, Azevedo IM, et al. Inequality in air pollution mortality from power generation in India. Environ Res Lett. (2022) 18(1):014005. doi: 10.1088/1748-9326/aca8bb
55. Spiller E, Proville J, Roy A, Muller NZ. Mortality risk from PM 2.5: a comparison of modeling approaches to identify disparities across racial/ethnic groups in policy outcomes. Environ Health Perspect. (2021) 129(12):127004. doi: 10.1289/EHP9001
56. Schultz WM, Kelli HM, Lisko JC, Varghese T, Shen J, Sandesara P, et al. Socioeconomic status and cardiovascular outcomes: challenges and interventions. Circulation. (2018) 137(20):2166–78. doi: 10.1161/CIRCULATIONAHA.117.029652
58. Rijnhart JJM, Lamp SJ, Valente MJ, MacKinnon DP, Twisk JWR, Heymans MW. Mediation analysis methods used in observational research: a scoping review and recommendations. BMC Med Res Methodol. (2021) 21(1):1–17. doi: 10.1186/s12874-021-01426-3
59. Wang C, Ahn J, Tarpey T, Yi SS, Hayes RB, Li H. A microbial causal mediation analytic tool for health disparity and applications in body mass index. Microbiome. (2023) 11(1):164. doi: 10.1186/s40168-023-01608-9
60. Bind MA, Vanderweele TJ, Coull BA, Schwartz JD. Causal mediation analysis for longitudinal data with exogenous exposure. Biostatistics. (2016) 17(1):122–34. doi: 10.1093/biostatistics/kxv029
61. Austin PC. An introduction to propensity score methods for reducing the effects of confounding in observational studies. Multivariate Behav Res. (2011) 46(3):399–424. doi: 10.1080/00273171.2011.568786
Keywords: gut microbiome, air pollution, chronic diseases, type-2 diabetes, directed acyclic graph
Citation: Mandal S, Kuriakose S and Shridhar K (2024) Investigating the role of gut microbiome in the association between ambient air pollution and type-2 diabetes mellitus: a causal inference approach using review of evidence to date. Front. Environ. Health 3: 1339674. doi: 10.3389/fenvh.2024.1339674
Received: 16 November 2023; Accepted: 28 June 2024;
Published: 18 July 2024.
Edited by:
Darby Jack, Columbia University, United StatesReviewed by:
Yuhan Yang, Georgia Institute of Technology, United States© 2024 Mandal, Kuriakose and Shridhar. This is an open-access article distributed under the terms of the Creative Commons Attribution License (CC BY). The use, distribution or reproduction in other forums is permitted, provided the original author(s) and the copyright owner(s) are credited and that the original publication in this journal is cited, in accordance with accepted academic practice. No use, distribution or reproduction is permitted which does not comply with these terms.
*Correspondence: Siddhartha Mandal, c2lkZGhhcnRoYUBjY2RjaW5kaWEub3Jn