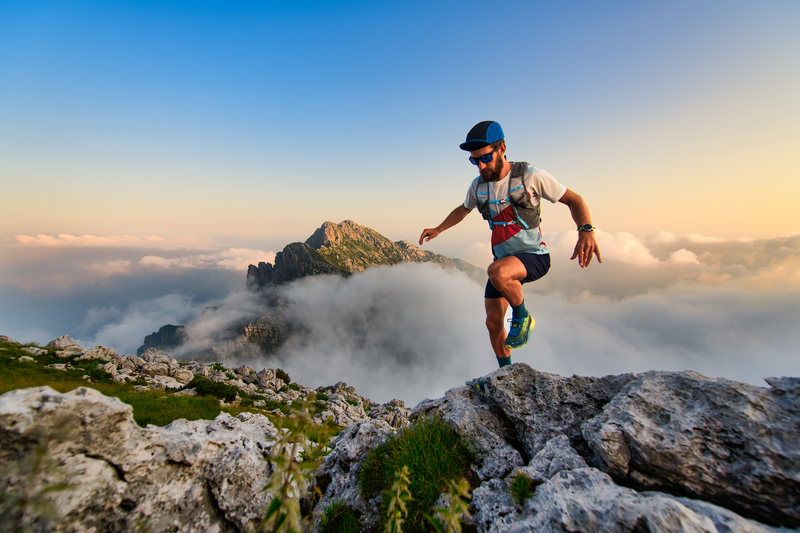
94% of researchers rate our articles as excellent or good
Learn more about the work of our research integrity team to safeguard the quality of each article we publish.
Find out more
BRIEF RESEARCH REPORT article
Front. Energy Res.
Sec. Sustainable Energy Systems
Volume 13 - 2025 | doi: 10.3389/fenrg.2025.1561763
This article is part of the Research Topic Advanced Data-Driven Uncertainty Optimization for Planning, Operation, and Analysis of Renewable Power Systems View all 15 articles
The final, formatted version of the article will be published soon.
You have multiple emails registered with Frontiers:
Please enter your email address:
If you already have an account, please login
You don't have a Frontiers account ? You can register here
This paper proposes an investment efficiency-oriented strategy for power grid infrastructure planning with high penetration of renewable energy sources. Firstly, a multi-objective investment portfolio optimization model based on data envelopment analysis is proposed to improve the cost efficiency of power grid infrastructure planning. Then, an evolutionary algorithm based on super-efficiency hyperplane projection transformation is developed to obtain the optimal Pareto frontier of multi-objective investment portfolio. Furthermore, a superefficiency envelope model with non-radial relaxation variables is formulated to identify optimal investment efficiency-oriented solution from the Pareto frontier set. Comparative case studies have been implemented to demonstrate the superior performance of the proposed strategy for investment efficiency enhancement of power grid infrastructure planning.
Keywords: Cost efficiency, Investment portfolio, Power grid planning, Renewable Energy, multiobjective optimization
Received: 16 Jan 2025; Accepted: 17 Feb 2025.
Copyright: © 2025 Wu, Zhou, Yan, Sang and Wang. This is an open-access article distributed under the terms of the Creative Commons Attribution License (CC BY). The use, distribution or reproduction in other forums is permitted, provided the original author(s) or licensor are credited and that the original publication in this journal is cited, in accordance with accepted academic practice. No use, distribution or reproduction is permitted which does not comply with these terms.
* Correspondence:
Jiong Yan, State Grid Hubei Electric Power Company Limited Economic and Technical Research Institute, Wuhan, China
Disclaimer: All claims expressed in this article are solely those of the authors and do not necessarily represent those of their affiliated organizations, or those of the publisher, the editors and the reviewers. Any product that may be evaluated in this article or claim that may be made by its manufacturer is not guaranteed or endorsed by the publisher.
Research integrity at Frontiers
Learn more about the work of our research integrity team to safeguard the quality of each article we publish.