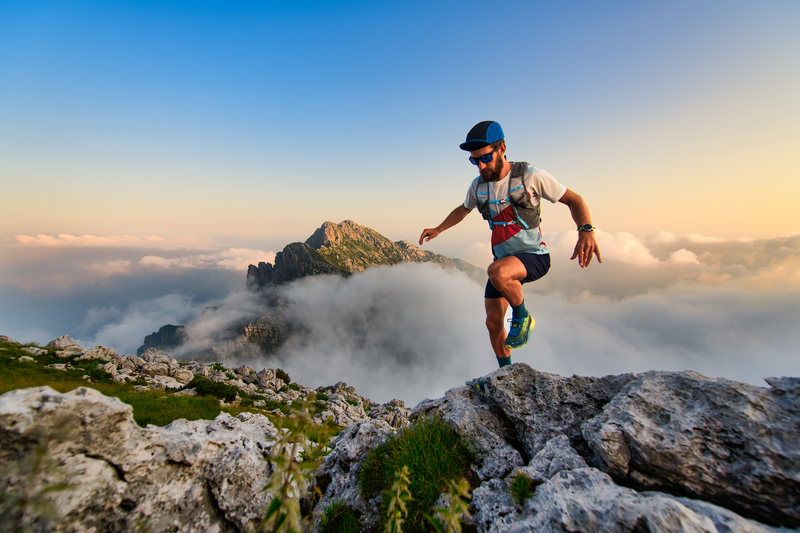
94% of researchers rate our articles as excellent or good
Learn more about the work of our research integrity team to safeguard the quality of each article we publish.
Find out more
REVIEW article
Front. Energy Res. , 19 February 2025
Sec. Energy Storage
Volume 13 - 2025 | https://doi.org/10.3389/fenrg.2025.1529608
Due to their high energy density, long life cycle, minimal self-discharge (SD), and environmental benefits, lithium-ion batteries (LIBs) have become increasingly prevalent in electronics, electric vehicles (EVs), and grid support systems. However, their usage also brings about heightened safety concerns and potential hazards. Therefore, it is crucial to promptly identify and diagnose any issues arising within these batteries to mitigate risks. Early detection and diagnosis of faults such as Battery Management Systems (BMS) malfunctions, internal short circuits (ISC), overcharging, over-discharging, aging effects, and thermal runaway (TR) are essential for mitigating these risks and preventing accidents. This study aims to provide a comprehensive overview of fault diagnosis by meticulously examining prior research in the field. It begins with an introduction to the significance of LIBs, followed by discussions on safety concerns, fault diagnosis, and the benefits of such diagnostic approaches. Subsequently, each fault is thoroughly examined, along with discussions on methods for detection and diagnosis, including both model-based and non-model-based approaches. Additionally, the study elevates the role of cloud-based technologies for real-time monitoring and enhancing fault mitigation strategies. The results show how well these approaches work to increase LIB systems’ safety, dependability, and economic feasibility while emphasizing the necessity for sophisticated diagnostic methods to support their growing use in a variety of applications.
Energy storage systems have proliferated in modern power systems due to the flexibility they provide in operation and stability (Hussain I. et al., 2020; Das et al., 2022; Chauhan et al., 2021; Barik et al., 2021; Ulutas et al., 2020). LIBs have seen a significant surge in popularity over the past few decades, firmly establishing themselves as the leading rechargeable battery technology. Currently, they dominate the battery market, powering a wide array of devices including portable electronics such as computers, EVs, mobile phones, and grid systems due to their high energy density, longer life cycle, minimal self-discharge (SD) capacity, and environmental benefits (Alqarni, 2024; Chen et al., 2020; Rao et al., 2024a; Xu et al., 2023; Rao K. D. et al., 2024; Zhao et al., 2024a). Due to this popularity, there are various fault diagnosis approaches developed as shown in Figure 1. Energy density stands out as the most notable feature distinguishing LIBs from other types like nickel-metal hydride (NiMH), nickel-cadmium (NiCd), and lead-acid batteries, as illustrated in Figure 2. LIBs, being lightweight and small, possess a high electrochemical potential, ultimately resulting in higher energy density (Kaushik et al., 2024; Keshan and Thornburg, 2016; Olabi et al., 2023).
Figure 2. Comparison among different battery technologies (A) in terms of volumetric and specific energy densities and (B) quantification in terms of energy density.
LIBs typically exhibit longer life cycles compared to other battery types, making them cost-effective as they can undergo multiple charge-discharge cycles before capacity degradation occurs (Alqarni, 2024; Chen et al., 2020; Rao et al., 2024a; Xu et al., 2023; Rao K. D. et al., 2024; Zhao et al., 2024a). Unlike lead-acid batteries that may require multiple replacements throughout a product’s lifespan, LIBs enable continuous product operation until the end of their life. Moreover, LIBs demonstrate minimal SD rates, allowing them to retain charge over a long duration of time (Bajagain, 2017; Roth et al., 2023). Although SD rates can be reduced by maintaining the battery above its nominal voltage and lowering the temperature, performance degradation remains inevitable (Liao et al., 2022). The intricate electrochemical reactions within LIBs impose limitations on their safe performance, posing challenges to their widespread adoption, particularly in EVs (Zou et al., 2023; Du et al., 2024).
Numerous LIBs have been implicated in explosions and fire incidents, significantly damaging the reputation of associated market sectors and causing economic problems (Hu et al., 2020; Chen et al., 2022; Shen R. et al., 2024; Ubaldi, 2024). Poor heat dissipation and an uneven temperature distribution in battery packs are the causes of thermal runaway. Heat accumulation from LIBs high energy density can result in internal deterioration and exothermic reactions, which can cause explosions or fires. By enhancing heat transfer and temperature homogeneity, effective thermal management such as the use of liquid cooling plates with porous media helps avoid thermal runaway.
Over time, the implementation of safety features has played a pivotal role in mitigating various risk factors associated with battery performance, thereby enhancing overall functionality (Close et al., 2024; Simpa et al., 2024). It is imperative to closely focus on advancing battery material systems, designing BMS, optimizing the structural aspects of energy storage systems, and other related areas to ensure the highest level of safety and stability when these systems are actively utilized in EVs (Akhil et al., 2024). These aspects are critical in the field of system engineering.
Furthermore, the establishment of an efficient and reliable real-time BMS is paramount for the security and wellbeing of LIBs. This advanced system is equipped to handle a wide range of tasks, including charging, equalization, monitoring the state of charge (SOC), and ensuring effective thermal management (Kumar et al., 2023). Additionally, it monitors the state of health (SOH) and potential faults of a LIB. Failure to diagnose and manage the faults effectively might lead to serious harm to LIBs from even minor issues (Hu et al., 2020).
Incidents like EV battery fires generally result from both internal and external faults, stemming from various factors such as mechanical abuse, electrical abuse, and thermal abuse (Linja-Aho, 2024; Mishra et al., 2024; Hao et al., 2024; Lalinde et al., 2024). As illustrated in Figure 3, these issues arise during battery utilization and may lead to TR. Mechanical abuse, for instance, involves impacts from needle punctures or crashes into battery cells, both cases of which can be detrimental (Chang et al., 2023). On the other hand, electrical abuse covers problems like overcharging, external short circuits (ESC), over-discharging, and other malfunctions. Thermal abuse occurs in LIBs due to when the system is subjected to higher demanding conditions, including rising temperatures (Chang et al., 2023). Early warnings in battery performance can significantly mitigate all safety incidents and eventually create a safer environment for all EV drivers. This research on the diagnosis and mitigation of faults in LIBs ensures safety and reliability in EVs and other applications of battery technology (Zhao et al., 2024b; Ranga et al., 2023). The need to minimize the risk of faults with fires and explosions in the LIBs is addressed by an all-rounded understanding of the causes of battery failures: mechanical, electrical, and thermal abuses. Effective fault diagnosis and management strategies profit not only the wellbeing of EV drivers but also the market sectors involved, as reputation and loss of revenues due to economic battery accident losses are also protected. Moreover, it also contributes to creating a safer environment by enhancing the comprehensive safety and stability of LIB systems to make people feel more confident about the widespread acceptance of EVs and other battery-powered technologies (Rao et al., 2023a; Svetlík et al., 2024). Ultimately, the findings of this research endeavor are very far-reaching, depicting progressive moves towards even advanced forms of battery technology for a more sustainable, secure future in practice.
Figure 3. The Cell Thermal Runaway evolution causes are listed in (A), damages are depicted in (B), types of abuse are categorized in (C), battery parts are impacted in (D) and fire or explosions are depicted using (E).
The environmental effects of LIBs have gained more attention due to their growing demand. It is necessary to understand the distribution of effects throughout the life cycle of LIBs, to identify all the relevant environmental hotspots and to develop mitigation plans for impacts. The goal of this study is to thoroughly examine how LIBs affect the environment and offer solutions to lower costs and adverse consequences. Most of the literature did not include the cloud-based technique for fault diagnosis. In this regard, this paper highlights the cloud-based techniques for fault mitigation and diagnosis.
The rest of this paper is organized as follows: In section 2, the safety concerns that are associated with LIBs during their operation are discussed. In section 3, the benefits of fault diagnosis were discussed. In section 4, the types of faults and each fault that generally occurs were discussed. In section 5, the model-based and non-model-based methods that were previously reported by the researchers to detect/diagnose the faults that occur in LIBs are reviewed. Finally, Section 6 concludes and offers some future research directions.
All types of batteries, including LIBs, are the widely used batteries in EVs (Tappeta et al., 2022). This demands safety concerns should be taken into consideration. LIBs have potential safety risks since they are operated at extremely high energy densities (Ralls et al., 2023). The use of LIBs in EVs has been retarded due to the explosions and fire accidents significantly resulting in economic issues for the battery market. This proposed the interest in safety measurements and is being developed (Zhao et al., 2024a; Chen et al., 2021).
The chemistry of the battery, operating conditions, environment, and damage acceptance have important impacts on battery safety (Zhang Y. et al., 2023). Due to the electrochemical system instability internal failure occurs. The cathode material is one of the parts in LIBs that costs the most (around 36% of battery system) and this has a considerable influence on the overall electrochemical capability of the battery. Hence, the primary safety issue is associated with cathode material composition. The application of lithium metal at the anode has been restricted as they form dendrites during the reaction. Graphite has been used in LIBs for the last 20 years as a safe and eco–friendly material. On the other hand, this also brings with its associated demerits, which include low capacity that leads to poor Coulombic efficiency and poor rate performance causing problems with LIB performance. It is, thus, a big effort in the proper selection of the anode material yet with improvement in one performance characteristic, other properties are often disturbed (Chen et al., 2021; Cheng et al., 2021). Temperature and voltage are the other two variables that govern battery reactions. These temperature, Voltage, and electrochemical reactions change according to the environmental conditions in which the battery is operated and cause internal disturbances that affect the safety of LIBs. As shown in Figure 4, the safety strategies can be applied to LIB both internally and externally to mitigate the safety issues.
Figure 4. Strategies to improve LIB safety (Raijmakers et al., 2019).
Though the battery is operated in normal operating conditions the heat generated by them is not completely resolved particularly on higher temperature days or in larger battery packs (Patel, 2023; Raijmakers et al., 2019). When the temperature of the battery increases drastically, causes the TR which is an uncontrollable state of battery heat generation. TR will happen even more quickly than mechanical, electrical, and thermal abuse situations during accidents (Menale et al., 2022). Most of the accidents that have been reported in recent times are attributed to mechanical abusive loading. Industry standards, therefore, give rise to significant concerns regarding the safety of the mechanical aspect. This includes but is not limited to, the potential risks associated with drop, penetration, mechanical shock, crush, vibration, and rollover (Ralls et al., 2023).
Additionally, there are safety concerns related to the cell, modules, pack, and vehicle levels. These concerns have been highlighted in (Liu et al., 2020). To meet the prerequisites for voltage, current, power, and energy, a considerable number of lithium batteries are commonly interconnected in series and parallel configurations (Liu et al., 2020; Rao et al., 2021; Kumar, 2024). This interconnection allows for the formation of battery modules and packs within EVs. These battery modules and packs serve as a means of large-scale energy storage and distributed energy storage. However, it is important to acknowledge that as the system becomes more complex due to the numerous components being connected together, it also becomes more susceptible to a variety of faults (Mitra and Mukhopadhyay, 2024). These faults encompass various types, such as cell faults, BMS faults, sensor faults, overcharging, over-discharging, TR, ESCs, and ISCs (Zhao et al., 2024a). Each of these faults poses unique risks and could potentially compromise the overall safety and functionality of the system. Thus, in order to guarantee the safe functioning of electric cars and the efficient storage of energy, it is imperative to fully comprehend and resolve these issues. BMS technology has advanced quickly, making it possible to quickly identify and detect failure signals (Huang et al., 2024; Rao C. et al., 2024). This allows the operator to be notified as soon as a fault arises. In order to prevent any potential battery safety accidents, the battery will undergo a continuous charging process until it reaches a state of TR. This charging process will persist even in the event of failures in various subsystems within the BMS, such as the balancing circuits, upper computer communication, and measurement circuits. If these malfunctions are not addressed, there might be a serious risk to the battery system’s safety (Parekh et al., 2020; Donati, 2024).
In this tech-filled world, LIBs are everywhere, running everything from phones to electric cars (Xu et al., 2023; Tappeta et al., 2022; Parekh et al., 2020). Keeping them safe and reliable is very important. The role of LIBs is widespread and due to the reliance on these energy storage systems, the need for efficient fault diagnosis has emerged as a critical aspect of ensuring their reliability and safety.
The multifaceted benefits of fault diagnosis in LIBs.
I. Fault diagnosis allows the identification of possible faults in LIBs before they become actual safety threats. It can prevent failures that may lead to fires or explosions since it has the capability to identify abnormalities such as overcharging, over-discharging, or ISCs.
II. In general, LIBs have a longer cycle life owing to the early detection and fixing of defects. Consumers can correct the shortcomings in terms of capacity decay or uneven cell aging and then apply remedial measures like alterations in charging and discharging operations to minimize the effect on the cycle life of the battery.
III. Fault diagnosis can help in better understanding the SOH and SOC of the battery. Such information allows the users to utilize their systems or devices in the best way possible, optimizing them for best performance. By detecting and replacing fault cells, a ripple effect that could impact the performance of the entire battery pack could be avoided.
IV. In addition, faster fault detection minimizes possible safety risks and is economically beneficial. It is relatively cheaper for heavy users of LIBs since their cost-saving measures will minimize the need for expensive repairs or replacements.
V. The car industry uses LIBs primarily as the power source in EVs. In such a context, fault diagnosis assists in the attainment of reliability of the EV’s drivetrain and avoidance of unscheduled malfunctions which subsequently establishes customer confidence in adopting EVs.
VI. Recycling and responsible disposal are the two faces of responsible LIBs management. Using fault diagnostics, environmentally friendly recycling technologies may apply: this determines which particular battery has just reached, or will shortly reach, its end of the life cycle, thus reducing the environmental impact associated with wasted batteries and promoting sustainable energy storage alternatives.
VII. New advances in problem diagnostic technology now ensure continuous LIB monitoring. Using predictive maintenance models, it would thus become possible for the models to allow users to foresee possible problems and take action before these prove harmful to battery performance.
The different types of faults are reviewed in this section Battery overcharging, over-discharging, TR, ISCs/ESCs, and accelerated degradation are some faults that cannot be easily detected due to the less knowledge of the operation within the Li cells. Among these faults, TR and accelerated degradation are the most dangerous ones as they affect the LIB application and in turn affect directly the user (Rangarajan et al., 2022; Lalinde et al., 2022). Figure 5 depicts the fault classification in LIBs.
When a BMS fails in stopping the charging of cells then more energy enters into the cell even after the source is fully charged and goes beyond the recommended voltage which results in overcharging of the LIB (Lalinde et al., 2022; Wang et al., 2024; Mago, 2024; Juarez-Robles et al., 2020). A battery’s cathode becomes over-delighted whereas the anode becomes over-lithiated when it is overcharged, causing several adverse effects (Rana et al., 2023) (Giuliano et al., 2011). Over-discharge causes side reactions in the cathode, such as irreversible structural changes and the breakdown of active materials. The electrolyte oxidizes and TR occurs as a result of those side reactions, which produce a lot of heat and oxygen (Liu S. et al., 2023). Dendrites of metallic lithium will occur on the anode surface when the anode is fully loaded with intercalated lithium. Ohmic resistance may increase (ORI) as a result of the deposited lithium reacting with the electrolyte to thicken the solid electrolyte interphase (SEI) film. The TR process is accelerated by the ORI because it increases the joule heat (Q = I2Rt) (Chen et al., 2024).
Over-discharge is a phenomenon that occurs when a cell is discharged beyond the lower safe voltage limit determined by the coupling of the electrode chemistry, as stated in reference (Menale et al., 2022). It is important to note that even when a deep over-discharge (between 2.0 and 0.0 V) takes place, there are no observable negative impacts on the thermal stability of the cells. Surprisingly, no harmful effects were observed, apart from the generation of gas and the swelling of the cell. It is interesting to highlight that over-discharging, although it has the potential to cause permanent capacity loss and have a detrimental effect on the cycle-life of the cells, did not exhibit any such consequences, as mentioned in reference (Li et al., 2024). Overcharging is one of the most abusive conditions that causes the degradation of LIBs (Tappeta et al., 2022; Ouyang et al., 2023; Rao et al., 2023b). Generally, it occurs in the cells that are connected in series as they are inconsistent in voltage and capacity in individual cells (Ouyang et al., 2023; Le et al., 2023). A small over-discharge event that appears may be difficult for a BMS to identify, this results in many reversible or irreversible changes in LIBs (Sun et al., 2023; Park et al., 2024).
In Kurzweil et al. (2022) focuses on the challenges of overcharging and over-discharging in automotive LIBs. The battery dynamic simulation model proves its effectiveness in analyzing LIB with varying parameters. When combined with equivalent circuit model (ECM) analysis, the model adeptly identifies failures in automotive LIBs, thereby improving the safety of battery use in vehicles. Experimental evidence indicates that under slight overcharging cycling, the battery’s failure behavior, mechanism, and diagnosis do not lead to TR. This suggests that local micro-internal factors are not the predominant contributors to LIB TR. The assesses lithium battery health under dynamic discharge conditions across temperatures. Extracting features from voltage and current data during tests on various cell types, the study proposes three health indicators strongly correlated with battery capacity. Using a Gaussian Process Regression (GPR) model, accurate battery capacity estimation is achieved with a Mean Absolute Error (MAE) of 0.3578%. The method maintains accuracy across diverse conditions and temperatures, validated through excellent results for unknown batteries (Liu S. et al., 2023).
Common sensors include Hall effect sensors for voltage and current monitoring, thermocouples, and resistance temperature detectors for temperature measurements (Liu and Kang, 2023) (Xiong et al., 2024). However, various factors such as manufacturing defects, harsh environments, and mechanical shocks can compromise sensor integrity. Sensor faults manifest as biases, drifts, scaling errors, or complete failures, directly impacting measurement signals (Seba et al., 2024) (Shen D. et al., 2024). These faults can be additive or multiplicative, influencing critical metrics like voltage in battery systems. Accurate management over battery charging, state estimation, fault diagnosis, and overall system performance depends on the detection and resolution of sensor problems. When sensor problems are not promptly detected, data might become erroneous. This can impact the multi-state estimate of battery characteristics and could result in the BMS malfunctioning or giving the wrong directives (Schmid et al., 2021).
When proposing a scheme to attract fault detection and isolation (FDI), it is important to focus on detecting any faults in the current or voltage sensors of a single battery cell. This can be achieved through the use of nonlinear parity equations and sliding mode observers (Xu et al., 2024). Hazard analysis of the battery system should also be conducted, which involves using structural analysis theory to evaluate the detectability and isolation of sensor faults within the battery system (Schmid et al., 2021). While an extended Kalman filter (EKF) can be used to identify current or voltage sensor faults in a single battery cell, achieving fault isolation can be challenging. It is worth noting that there is a significant gap in the existing literature regarding FDI for sensors specifically in a battery pack (Obrien et al., 2023). The robust approach that utilizes sensor data, including battery expansion force and gas levels, to detect ISCs in batteries has been discussed (Cai et al., 2020). It specifically addresses ISC events characterized by a swift voltage drop and recovery, without a substantial change in surface temperature, a challenge for traditional measurements. The simulation demonstrates the sensor’s rapid response to such events. However, further exploration is needed to determine optimal threshold values for gas and force detection. Notably, the simulation overlooks sensor drift and model mismatch in aged cells, necessitating additional research to handle potential bias and errors in detection quantities.
One of the primary responsibilities that a BMS undertakes is the essential objective of reducing and mitigating the potential hazards and dangers that are inherently connected with the utilization and operation of a LIB. This paramount function not only encompasses the safeguarding and preservation of the battery itself, but it also extends to the protection and well-being of the individuals who interact with and rely upon the battery’s functionalities and capabilities. Through the implementation of various precautionary measures, the BMS diligently strives to proactively minimize and curtail any potential risks or adverse consequences that may arise from the usage of the LIB, thus ensuring the utmost safety and security for both the battery and its users (Tran and Fowler, 2020).
Figure 6 illustrates how the BMS performs fault diagnosis on LIBs. It can protect a battery from many common problems, but it cannot protect against all possible problems. For example, it cannot prevent damage caused by lithium coating, a phenomenon where lithium ions accumulate on the battery and its surface and can cause the battery to swell or even explode. Although a BMS can monitor the battery and its temperature and cut power when the temperature becomes too high, it cannot prevent the battery from being exposed to extreme temperatures. This is a serious problem because temperatures that are too high or too low can seriously damage the battery. As mentioned earlier, BMS cannot prevent lithium plating. This can cause the battery to expand and potentially explode, creating a significant safety hazard. It also can prevent many of the common chemical reactions that can damage a battery, but it cannot prevent all of them like, it cannot prevent the battery from reacting with certain chemicals or substances that cause damage to the LIBs (Zou et al., 2023).
Figure 6. Illustration of BMS fault diagnosis (Tran and Fowler, 2020).
A technique for diagnosing power battery faults in EVs using enhanced Radial basis function (RBF) neural networks. The lithium iron phosphate battery pack’s six parameters are its variables, and its fault levels are called targets. The CAN bus is used to collect experimental data. Then, to identify battery defect information, the Probabilistic Neural Network (PNN) and General Regression Neural Network (GRNN) algorithms are used. Parameter and sample size concerns are then discussed and compared. The outcomes validate the efficacy of GRNN and PNN algorithms in detecting battery issues by demonstrating the impact of parameters and sample size on neural network performance (Wang et al., 2021).
Future studies will incorporate the SOH parameter for precise battery protection, validating the accuracy and feasibility of the new fault diagnosis method. A novel approach is proposed for diagnosing faults in batteries, which is based on a modified version of Shannon entropy. This method aims to identify cells within battery systems that exhibit abnormal voltage fluctuations. This is accomplished by computing the voltage sequence’s Shannon entropy inside a shifting time window, which enables real-time implementation. A sensitivity factor (SF) is developed to precisely determine the magnitude of anomalous voltage fluctuations, and it is found to be both accurate and efficient. Utilizing battery operating data from a cloud monitoring platform, the diagnostic model is tested in order to further improve the problem diagnosis’ accuracy. A thorough examination is guaranteed by the 0.1 Hz sampling frequency of the data. It is discovered throughout the validation phase that the diagnostic findings contain a sizable number of false alarms, suggesting the need for improvement (Liu Q. et al., 2023).
In LIBs, SCs appear both internally and externally. LIBs have an insulating separator layer between the two electrodes (anode and cathode), when this insulating separator fails both electrodes lose contact between them, and then ISCs occur (Tran and Fowler, 2020; Yuan et al., 2023; Huang et al., 2021). This condition results in TR because the electrolyte tends to break down through an exothermic reaction. When an SC arises it builds up some heat this heat is the main reason for the TR of LIBs. As LIBs have higher capacity they undergo TR from ISCs (Abu et al., 2023). ESCs in LIBs may occur when the tabs are linked via a low resistance channel, or when electrolyte leaks from the expanding cell as a result of gas production from side reactions during overcharge, immersion in water, or impact deformation. According to a study (An et al., 2023), an ESC causes limited current to flow through the negative electrode’s Li-ion diffusion, while the positive electrode’s electrolyte breakdown produces heat that causes TR. Excessive discharge of a cell’s stored energy can also result from an ESC (Shen D. et al., 2024).
Detecting soft SCs involves utilizing various methods such as thermal analysis, which makes use of a 3D electrochemical-thermal model to simulate different scenarios of ISCs (Khosravi et al., 2024). Another approach to estimating faults entails employing an extended ECM that accounts for both ISCs and ESCs. Since the nonlinearity of the battery OCV-SOC curve poses a challenge, a systematic self-regulating mechanism is proposed, which involves the utilization of Takagi-Sugeno fuzzy system modeling and optimized Gaussian membership functions across different SOC ranges (Meng et al., 2020). In addition to this, there exists a method for diagnosing short-circuit faults in battery module components, which relies on voltage cosine similarity and extracts features from the battery’s ISC fault. With the use of this technique, fault-signal characteristics may be effectively separated by creating a two-dimensional feature vector and enhancing eigenvectors with a gain multiple (Wu et al., 2023).
Exothermic chain reactions within LIBs cause a phenomenon known as TR. These reactions typically result in a sudden rise in the battery’s internal temperature, which causes the battery’s internal structures to become unstable and deteriorate, which may to the battery’s complete failure. There is a chance of TR from different kinds of thermal, electrical, and mechanical abuse (Shahid and Agelin-Chaab, 2022). If TR starts at the battery level, it spreads to the batteries next to it and eventually to the battery pack. Figure 7 shows how the damage in one cell passes to the entire battery.
In the present era, there exists a multitude of data centers dedicated to storing an extensive reservoir of historical data pertaining to new energy vehicles, encompassing a wide range of information including but not limited to current, voltage, and temperature readings (Choi et al., 2021). By harnessing the knowledge derived from the analysis of these vast repositories of historical data, one can effectively anticipate and issue timely warnings regarding TR phenomena. The application of methodologies rooted in the realm of big data holds the promise of augmenting the generalization capabilities of these warning systems. Furthermore, the experimental process entails the deliberate accumulation of a substantial volume of laboratory-generated TR data by means of various fault injection techniques, acupuncture, and collision simulations, amongst others. This particular approach serves as a complementary means of verifying and validating the efficacy of data-driven algorithms, thus lending further credence to their practicality and effectiveness (Kim et al., 2021; Guo and He, 2022; Huria et al., 2012).
The method of predicting TR, which is specifically based on fault injection, allows researchers to investigate the consequences of particular incentives on the occurrence of battery TR, as evidenced by signals from battery sensors. Nonetheless, the majority of data sources in this methodology rely on simulation data from an ECM, experiments designed to trigger battery TR, or a combination of both. Despite ongoing enhancements to the model and triggering techniques, this set of approaches still encounters difficulties when applied to real-vehicle data for the purpose of early warning of TR (Zhang X. et al., 2023). Enhancing the intrinsic safety of LIBs, particularly their resistance to TR, can be achieved through material modification. Passive defense design helps minimize secondary damage during abusive conditions, while an early detection algorithm becomes crucial for alerting passengers about potential faults (Hu et al., 2024).
Two broad categories can be used to group the research methods for fault diagnosis which include model-based and non-model-based methods (Lalinde et al., 2024; Wu et al., 2022).
In the model–based fault diagnosis technique, a physical model of the battery is created and this model can be used to compute the battery parameter values with accuracy. Then, these values are compared with the gathered battery parameters. A defect is said to happen if there is a difference between them that is greater than a certain value (threshold value) (Wu et al., 2022). In general, there are different categories to estimate the states Figure 8 outlines the standard approach for state estimate. The different works that have been done previously in the fault diagnosis sector of LIBs using model-based techniques and the properties of the proposed method are depicted in Table 1.
In the study (Zhang K. et al., 2023), the authors offer a novel technique for identifying soft ISC errors in LIB packs used in EVs early on. By extracting fault features from incremental capacity curves (ICC), as opposed to typical voltage measurements, the suggested method facilitates the identification of minute changes. To determine the severity of a fault and monitor its development during charge and discharge cycles, the local outlier factor (LOF) method is utilized. The efficiency and applicability of the approach are shown by simulation testing and data from actual vehicles. The results underscore the need for prompt defect identification in averting TR and guaranteeing the secure functioning of battery systems. Real-time monitoring without complicated modeling is now feasible thanks to this technique. Its efficacy complements industry demands and encourages BMS use. Utilizing current battery data reduces expenses and improves safety in an economical manner. For implementation and interpretation, the approach requires little training and is easy to use. Voltage variations and ICC are critical characteristics for identification, allowing for prompt fault intervention. With its lightweight ICC extraction and LOF, the approach has a minimal computational cost. Because of ICC’s sensitivity to fault identification, it guarantees excellent accuracy. Real-world data and BMS integration are used to validate high reliability. Robustness under various conditions may be confirmed by additional testing.
In Lai et al. (2020), the ISCs in series-connected LIB packs are examined based on the correlation between the SOC. The EKF is utilized to estimate the real-time SOC, utilizing the first-order ECM to estimate the SOC of each cell. The correlation coefficient for adjacent cells is then calculated to identify the ISC faults. This method is characterized by its speed, high accuracy, and ability to detect ISC faults of 100 Ω within 20.4 h. The industry’s adoption of this approach is limited by its lack of sensitivity for early-stage ISCs, despite its simplicity and affordability. Better detection times are provided by the SOC difference and voltage difference methods, but they are less useful in dynamic situations since they depend on high sensor precision and are susceptible to noise. If electrochemical impedance spectroscopy gives great precision but requires expensive equipment and precise measurements, impeding its wider adoption, dedicated circuitry offers fast detection at the expense of increased implementation costs.
To establish the OCV-SOC relationship, a second-order RC equivalent circuit is employed, as described in Meng et al. (2020). This relationship is subsequently fuzzified for the purpose of recognizing and calculating the ISC cells. While fuzzy logic systems offer improved accuracy but may require more complex implementation, ECMs are widely adopted in the industry due to their adaptability and ease of integration with existing systems. The practicality of methods for detecting incipient SC faults in LIBs varies. Adoption strategies include minimizing experimental restrictions by using verified models and existing literature, which can save money and time. Economically speaking, techniques such as cumulative sum control charts offer low-cost detection with few false alarms. Additionally, by identifying factors like battery current and SOC, these techniques facilitate detection and are therefore appealing for widespread industry use.
In Rao et al. (2023c), an adaptive dual Kalman filter (DKF) for precise SOC estimation has been discussed. Further, for real-time updates, it uses recursive least squares and a fractional-order ECM. With SOC estimation errors of less than ±0.01, the DKF demonstrates excellent accuracy and resilience. This method is more complex but provides higher accuracy and adaptability, essential for advanced applications. Higher implementation costs but offer better long-term savings through improved performed and efficiency. The fault detection is complex as it requires sophisticated sensors and algorithms. Multiple parameters include voltage, current, temperature and battery characteristics for precise SOC estimation. By analyzing the SOC residuals, current, and voltage (She et al., 2024), presents a diagnostic approach that relies on an electrochemical observer model and a fuzzy logic algorithm in identifying and diagnosing the faults. For typical faults, the approach offers excellent accuracy, reliability, and a reasonable computing cost; however, performance is dependent on appropriate calibration and tuning.
Lastly, some utilize equivalent circuit methodology and impedance spectroscopy technology to construct multiple battery signature fault models. A Kalman Filter (KF) method or an EKF method is employed to estimate the terminal voltage of the model and generate residuals. This was done because overcharging and overcharging can lead to changes in the model parameters (Bustos et al., 2024; Rao et al., 2024d). The probability of failure was also indicated using a probability-based method. However, this approach comes with the challenge of finding several models to execute an EKF. Although the procedure is computationally costly, it is effective. Model quality and system conditions affect accuracy and dependability.
In Zhao et al. (2024c), a hybrid neural network (HNN) and fault threshold optimization algorithm for online prediction and diagnosis of LIB temperature abnormalities. By combining convolutional neural networks (CNN) and long-short-term memory (LSTM) networks, it achieves accurate temperature predictions. Experimental validation demonstrates timely and reliable fault diagnosis with an average execution time of under 3.5 ms, showing real-time application potential and addressing existing limitations in battery management. The HNN and fault threshold optimization algorithm offer practicality with real-time application potential, making them attractive for industry adoption. Because of their affordability, simplicity of detection, and precision in identifying characteristics, they are useful instruments for enhancing battery safety and dependability but depend on quality training data and proper setup.
The Unscented Kalman Filter (UKF) has been adopted for the detection of problems such as over-charge, over-discharge, and short circuits in LIBs. The UKF outperforms the EKF in defect diagnosis with faster and better results. High-energy density LIBs modeling and simulation have been used for the purposes of fault identification (Sadhukhan et al., 2022). Damage in terms of application is saved as much as possible due to its cost-effectiveness. The UKF is supposed to be the best option in diagnosing the fault in LIBs since it has strong performance and detection efficiency. Despite its increased computing cost and dependence on exact models, it is more accurate and reliable than the EKF because it effectively handles nonlinear systems. However, it is still cost-effective and efficient.
Optimization algorithms for parameter identification and backstepping approaches are integrated with boundary observer design techniques to find Thermal failures in LIBs (Feng et al., 2024). This strategy reduces the number of sensors needed while preserving dependable performance by using residual evaluation and threshold calculation. It makes it possible to identify flaws early on, which facilitates prompt maintenance and stops the battery’s performance from getting worse. However, the optimization techniques for parameter identification can be modified for industrial usage with the right training and system integration, even though the execution of this method requires specialized knowledge and significant computational resources. The boundary observer design increases the precision of defect parameter identification and detection, which could lead to operational and maintenance cost savings. For the procedure to be effective, calibration is essential. Table 2 depicts the model-based techniques comparative analysis in terms of accuracy, precision, computation cost, reliability and real-time implementation. The UKF performs better in comparison to other techniques in terms of all the mentioned performance indices (Xu et al., 2017).
Non-model-based techniques were further divided into two types they are based on expert systems and based on data-driven methods. The primary goal of the expert system-based approach was to diagnose particular fault types by first building a knowledge base through experience and knowledge between fault types and fault data (Chang et al., 2023). Figure 9 describes the standard approach of data-driven methods. Table 3 lists all of the previous research, conducted in the field of LIB fault diagnosis employing non-model-based techniques, along with an arrangement of the suggested method’s properties.
A data-driven methodology is employed for the fault diagnosis of LIB packs in EV in order to identify minor faults (Wu et al., 2022). It employs ordinary least squares (OLS) for feature extraction, K-means clustering for reference cell selection, and the R-Lowess algorithm for data cleaning. The discrete Frechet algorithm is employed for fault localization and diagnosis. It has a high accuracy, modest computational cost and has been validated experimentally as dependable. In the course of battery over-discharge, the two primary principles of anomalous temperature elevation and anomalous voltage reduction were to establish rational thresholds for preliminary assessment. Subsequent to deriving statistical discoveries from initial experimental data, they subsequently calculated the likelihood of fault incidence (Kim and Kowal, 2022). Experimental data and statistical analysis support its high reliability and low computational cost. The current orientation of a continuous voltage source provided by a battery, after establishing a parallel configuration with a constant voltage source, along with the direction of current flow, can serve as a diagnostic tool to ascertain the presence of any malfunctions within the battery (Bharathraj et al., 2022). In any case, it is quite uncomfortable to monitor EVs in real-time and can be difficult at times to add a steady voltage supply. There are drawbacks like complexity and integration issues.
In Tian et al. (2024), a modified CNN technique for LIBs thermal fault detection technique has been elaborated. The model is trained with a dataset of thermal pictures and is based on a mask region-based CNN. The method is data-driven and seeks to increase fault detection efficiency and accuracy. The ability to identify heat problems in LIBs is promising, according to the results. Due to its high efficiency and precision, this method is helpful in fault detection of LIBs. The industrial acceptance would depend on the availability of thermal imaging equipment and the investment in data-driven methodologies. The approach economics would be a one-time expenditure for training and equipment, but a cost saved by early fault detection and prevention.
With a given voltage drop pattern and strategy that follows the cell voltage differences, a new framework for online ISC detection in batteries is proposed. The framework can differentiate between ESC, ISC, and incorrect connections of wires associated with voltage signals. It is possible to issue alarms and prevent false alarms for ISC using the model even when it does not achieve the high temperature. Early detection and false alarm prevention of such anomalies are offered by BMS software, thereby improving the safety aspects of batteries and the dependability of the vehicle. In all methods, early ISC detection in the EV batteries forms the key component in order to enhance the safety standards in the industries. With respect to cell abnormality detection, the proposed voltage difference-based approach is reliable, cost-effective, and very easy to implement (Gao et al., 2020).
A dual-tier fault diagnosis methodology utilizing machine learning techniques is employed to detect over-discharge phenomena in LIBs. To ascertain instances of over-discharge, the initial tier conducts a comparative analysis of the battery’s voltage against a predetermined cut-off voltage; furthermore, to ascertain previous occurrences of over-discharge, the subsequent tier implements an Extreme Gradient Boosting algorithm (Gan et al., 2021). Real-world EV data is used to validate the suggested approach. In electric cars, over-discharge is a common problem that can cause irreversible changes and battery TR. Over-discharge fault diagnosis techniques currently in use can be divided into three categories: knowledge-based, model-based, and data-driven. Data-driven techniques are popular and have been used on actual EVs. They do not require precise battery models. With characteristics based on voltage comparison, it is easily detectable, has efficient economics, and has a strong potential for industry application.
A modified Gradient Boosting Decision Tree (GBDT) algorithm and the isolation Forest (iForest) methods are to forecast and diagnose battery issues in EVs (Zhang et al., 2024). Exact battery voltage prediction and anomaly detection are critical to EV safety, according to the research. With consideration for a number of vehicle and battery parameters, including driving style and charging condition, the study suggests a quick and accurate voltage forecast approach. A useful problem diagnosis method called iForest-Boxplot is also introduced. Utilizing actual EV data the algorithms are proven and demonstrate the capacity to precisely diagnose faults and forecast voltage abnormalities up to six minutes in advance. With applications extending to safer warning systems for EVs, the research attempts to improve BMS and EV performance. Table 4 depicts the non-model-based techniques comparative analysis in terms of accuracy, precision, computation cost, reliability and real-time implementation. The modified CNN outperforms other techniques across all the mentioned performance indices.
LIB systems, especially in electric vehicles and grid applications, pose significant challenges due to their complexity. With numerous interconnected cells, detecting early-stage faults like ISC and TR becomes increasingly difficult. These faults can propagate to neighboring cells, making early detection critical, yet technically challenging, due to the complexity of the systems.
Current fault detection methods primarily rely on sensors to monitor temperature, voltage, and current. However, these sensors often experience drift, scaling errors, and mechanical wear leading to inaccuracies in the fault diagnosis process. Moreover, the lack of real-time data from internal battery components, such as the electrode material, limits the ability to detect critical issues like ISC or over-discharge in their early stages. Since the impacts of latent faults like overcharging, over-discharging, and slow degradation take time to emerge, it is particularly challenging to diagnose them using current diagnostic models.
Additional challenges are presented by both model-based and non-model-based approaches. Though they are resource-intensive, model-based approaches seek to provide accurate physical models but necessitate thorough calibration and precise initial circumstances. Non-model-based methods, like machine learning, have potential, but their practical use is complicated by their high data requirements and noise susceptibility. These restrictions make fault detection systems less useful for early defect diagnosis and real-time monitoring in LIBs.
The future of LIB fault diagnosis is bright with sophisticated sensor technologies as well as a rich set of machine learning algorithms that can lead towards the early inception of anomalies and fault prediction. The strategic deployment of intelligent BMS and advanced thermal management enhances fault mitigation and provides more accurate diagnoses. Techniques for monitoring battery health open up exploration into fault-tolerant designs and integrated diagnostic platforms so that the overall system reliability and safety can be improved. Standardization and regulation initiatives yield better consistency of the testing protocol, thus facilitating continuous improvements to the methodology for fault diagnosis.
Future research projects can focus on the development of advanced techniques for fault diagnosis of LIBs to deal more effectively with present problems causing impairments in the safety levels of the entire battery. Hybrid fault diagnosis approaches may be developed, merging each of the strengths of model-based and non-model-based techniques. Integration of machine learning algorithms with physics-based models may be used to further accuracy and efficiency toward fault detection at minimal computational costs. New sensing technologies as well as diagnostics tools capable of real-time monitoring of health and performance in batteries are areas that need more research. Advanced sensor technologies, such as distributed fiber optic sensors, and novel electrode materials with sensors integrated inside for early fault detection and error prevention in LIBs, are just but a few examples.
In recent times, it has emerged as a promising field of investigation in the area of LIB fault diagnosis: multi-physics modeling techniques. This approach relates to the development of powerful computational models that are used for the simulation of various electrochemical, thermal, and mechanical processes occurring inside battery cells. In this way, multi-physics models lead to a holistic understanding of battery behavior under different operating conditions by taking into account a diverse variety of physical phenomena, such as ion transport, electrode kinetics, and heat generation. The models are applied by the researchers in simulating faults, predicting faults’ time-scales evolution, and also assessing the impact of faults on battery performance. Due to the inclusion of multi-physics modeling, the researchers can understand some complex interactions between the various determinants of battery faults, allowing them to achieve better diagnostics strategies.
Besides multi-physics modeling, researchers are also focusing on the application of advanced diagnostic techniques for LIB performance for real-time monitoring. Such techniques include observation of the behavior of the battery under real operating conditions so that researchers can capture dynamic changes during charge-discharge cycles. A number of advanced characterization techniques have pointed to the same internal state of a battery comprising electrode degradation, electrolyte decomposition, and SEI layers. At an early stage of application of these techniques, evidence of degradation or fault is realized; such proactive maintenance allows the extension of the service life of the battery.
Moreover, new fault-tolerant designs are also underway, making LIBs even more reliable and safe. These include different redundancies in individual components, self-healing materials or systems (Ustun and Ayyubi, 2019), or adaptive control strategies (Ustun et al., 2012) that may be necessary to reduce faults while allowing systems to continue operation. Designs focusing on faults, mainly suppress faults to a very minimal effect on battery performance, avoiding catastrophic failure mainly in EV and grid energy storage applications (Hussain S. M. S. et al., 2020). The aim of this study is to introduce fault tolerance into the battery system to enhance the system’s robustness and minimize potential dangers or failure events.
The future research in this area of LIB fault diagnosis will be to build a unified diagnostic platform, putting various diagnostic methods and algorithms under one framework, to integrate data from sensor sources with modeling simulations and in-situ measurements for holistic assessment of the condition and performance of batteries (Hashimoto et al., 2021). Integrated diagnostic platforms will use advanced data analytics and machine learning algorithms to generate actionable insights into the state of the batteries, allowing for informed decisions about their maintenance and operations, and safety protocols. Similar implementations in smartgrids can be found in Ustun et al. (2021a), Ustun et al. (2021b). In this sense, integrated diagnostic platforms would be a key way of strengthening the reliability, safety, and longevity of LIBs in all applications.
As depicted in Figure 10, the future of fault diagnosis in LIBs suggests an integrated system based on IoT, ML, and Cloud for in-time monitoring and in-time detection of faults. It is built for large deployments and gives predictive maintenance, which prevents problems from happening in the first place. This approach can enhance cost efficiency through data-driven decision-making and operational optimization as well as remote monitoring and control of battery systems. Furthermore, enhancements realized due to the ML algorithm make for more response times and reliability of the systems.
The LIBs begin with the Battery Pack, which consists of an array of Li-ion cells, used in applications like electric vehicles and energy storage systems. Numerous sensors, including voltage, temperature, and current sensors, track important battery metrics to keep an eye on this pack and identify problems like thermal runaway or overcharging. The IoT Module (Edge Device), which receives this real-time data, aggregates the sensor data, processes it locally (filtering noise, does basic anomaly detection), and gets it ready to be transferred to the cloud. At this point, local data processing minimizes latency and bandwidth by calculating pertinent metrics prior to transferring data to the cloud.
After processing, data is sent to a cloud server via wireless communication technologies like Wi-Fi, LTE, or LoRaWAN, where it is centrally stored and accessible for analysis. Cloud-based machine learning analytics algorithms evaluate past and present data to identify patterns, forecast battery malfunctions, and determine the state of charge and SOH of the battery. Lastly, this data is displayed through a User Interface/Monitoring Dashboard, which gives users or operators access to a web or mobile interface for tracking battery health, receiving alarms, and visualizing performance patterns.
LIBs are essential to contemporary electronics and electric vehicles due to their high energy density, extended lifespan, low self-discharge rates, and environmental advantages. But there are serious safety issues with their extensive use, especially in some operational environments. To reduce risks like fires and explosions, accurate problem diagnosis is crucial. This study examines a variety of LIB flaws and divides diagnostic approaches into model-based and non-model-based approaches. While non-model-based approaches rely on a large amount of data and training without requiring intricate battery models, model-based approaches offer exact fault location and quantification but require accurate battery modeling.
Incorporating advanced sensor technologies and machine learning algorithms for precise diagnostics and early fault prediction is one of the future developments in LIB fault diagnosis. Advanced heat management techniques and intelligent BMS will enhance fault diagnosis and mitigation. The main focus of research should be on hybrid diagnostic techniques that maximize accuracy and efficiency while reducing computing costs by combining the advantages of model-based and non-model-based approaches. This research is novel in that it explores multi-physics modeling methodologies, providing detailed computer models to simulate different battery cell processes for improved diagnostic approaches.
In summary, improving LIB safety via efficient fault diagnostics is essential for wider implementation across a range of applications, guaranteeing a safer environment and a sustainable future. For researchers creating more effective defect diagnosis methods for LIB systems, this thorough overview is an invaluable resource. Battery systems’ safety and dependability can be greatly increased by implementing cutting-edge technology and creative strategies, which will increase user confidence.
KR: Writing–original draft, Writing–review and editing. NP: Writing–original draft, Writing–review and editing. MR: Writing–original draft, Writing–review and editing. CM: Writing–original draft, Writing–review and editing. SD: Writing–original draft, Writing–review and editing. TU: Writing–original draft, Writing–review and editing. AK: Writing–original draft, Writing–review and editing.
The author(s) declare that no financial support was received for the research, authorship, and/or publication of this article.
The authors declare that the research was conducted in the absence of any commercial or financial relationships that could be construed as a potential conflict of interest.
The authors declare that no Generative AI was used in the creation of this manuscript.
All claims expressed in this article are solely those of the authors and do not necessarily represent those of their affiliated organizations, or those of the publisher, the editors and the reviewers. Any product that may be evaluated in this article, or claim that may be made by its manufacturer, is not guaranteed or endorsed by the publisher.
Abu, S. M., Hannan, M. A., Hossain Lipu, M. S., Mannan, M., Ker, P. J., Hossain, M. J., et al. (2023). State of the art of lithium-ion battery material potentials: an analytical evaluations, issues and future research directions. J. Clean. Prod. 394, 136246. doi:10.1016/j.jclepro.2023.136246
Akhil, S. S., Rao, K. D., Mansa, T., Tharun, S., and Dilip, B. (2024). “A brief review of cyber attacks on electric vehicle battery management system,” in Innovations in energy management and renewable resources. IEMRE 2023. Lecture notes in electrical engineering, volume 1239. Editors M. Pal, J. M. Guerrero, P. Siano, D. Das, and S. Chowdhuri (Springer).
Alqarni, A. (2024). Investigating the performance and longevity of LIBs in grid-scale energy storage applications.
An, Z., Zhao, Y., Du, X., Shi, T., and Zhang, D. (2023). Experimental research on thermal-electrical behavior and mechanism during external short circuit for LiFePO4 Li-ion battery. Appl. Energy 332, 120519. doi:10.1016/j.apenergy.2022.120519
Bajagain, S. (2017). Mathematical modeling and capacity fading study in porous current collector based lithium ion battery.
Barik, A. K., Das, D. C., Latif, A., Hussain, S. M. S., and Ustun, T. S. (2021). Optimal voltage–frequency regulation in distributed sustainable energy-based hybrid microgrids with integrated resource planning. Energies 14, 2735. doi:10.3390/en14102735
Bharathraj, S., Adiga, S. P., Kaushik, A., Mayya, K. S., Lee, M., and Sung, Y. (2022). Towards in-situ detection of nascent short circuits and accurate estimation of state of short in Lithium-Ion Batteries. J. Power Sources 520, 230830. doi:10.1016/j.jpowsour.2021.230830
Bustos, R., Gadsden, S. A., Biglarbegian, M., AlShabi, M., and Mahmud, S. (2024). Battery state of health estimation using the sliding interacting multiple model strategy. Energies 17 (2), 536. doi:10.3390/en17020536
Cai, T., Mohtat, P., Stefanopoulou, A. G., and Siegel, J. B. (2020). Li-ion battery fault detection in large packs using force and gas sensors. IFAC-PapersOnLine 53 (2), 12491–12496. doi:10.1016/j.ifacol.2020.12.1763
Chang, C., Zhang, Z., Wang, Z., Tian, A., Jiang, Y., Wu, T., et al. (2023). Fault diagnosis of LIBs based on voltage dip behavior. Int. J. Green Energy, 1–13. doi:10.1080/15435075.2023.2260019
Chauhan, A., Upadhyay, S., Khan, M. T., Hussain, S. M. S., and Ustun, T. S. (2021). Performance investigation of a solar photovoltaic/diesel generator based hybrid system with cycle charging strategy using BBO algorithm. Sustainability 13, 8048. doi:10.3390/su13148048
Chen, S., Fan, G., Wang, Y., Zhou, B., Ye, S., Liu, Y., et al. (2024). The impact of intermittent overcharging on battery capacity and reliability: electrochemical performance analysis and failure prediction. J. Power Sources 591, 233800. doi:10.1016/j.jpowsour.2023.233800
Chen, S., Gao, Z., and Sun, T. (2021). Safety challenges and safety measures of Li-ion batteries. Energy Sci. and Eng. 9 (9), 1647–1672. doi:10.1002/ese3.895
Chen, T., Jin, Y., Lv, H., Yang, A., Liu, M., Chen, B., et al. (2020). Applications of lithium-ion batteries in grid-scale energy storage systems. Trans. Tianjin Univ. 26 (3), 208–217. doi:10.1007/s12209-020-00236-w
Chen, Z., Yildizbasi, A., Wang, Y., and Sarkis, J. (2022). Safety concerns for the management of end-of-life lithium-ion batteries. Glob. Challenges 6 (12), 2200049. doi:10.1002/gch2.202200049
Cheng, H., Shapter, J. G., Li, Y., and Gao, G. (2021). Recent progress of advanced anode materials of lithium-ion batteries. J. Energy Chem. 57, 451–468. doi:10.1016/j.jechem.2020.08.056
Choi, D., Shamim, N., Crawford, A., Huang, Q., Vartanian, C. K., Viswanathan, V. V., et al. (2021). Li-ion battery technology for grid application. J. Power Sources 511, 230419. doi:10.1016/j.jpowsour.2021.230419
Close, J., Barnard, J. E., Chew, Y. M. J., and Perera, S. (2024). A holistic approach to improving safety for battery energy storage systems. J. Energy Chem. 92, 422–439. doi:10.1016/j.jechem.2024.01.012
Das, A., Dawn, S., Gope, S., and Ustun, T. S. (2022). A risk curtailment strategy for solar PV-battery integrated competitive power system. Electronics 11, 1251. doi:10.3390/electronics11081251
Donati, S. (2024). “Thermal runaway experimental analysis on 18650 Lithium-ions batteries during nail penetration test,”. PhD diss. (Torino, Italy: Politecnico di Torino).
Du, H., Wang, Y., Kang, Y., Zhao, Y., Tian, Y., Wang, X., et al. (2024). Side reactions/changes in lithium-ion batteries: mechanisms and strategies for creating safer and better batteries. Adv. Mater. 36, 2401482. doi:10.1002/adma.202401482
Feng, Y., Zhu, X., Wang, Y., Wang, B.-C., Zhang, H., Wu, Z.-G., et al. (2024). PDE model-based on-line cell-level thermal fault localization framework for batteries. IEEE Trans. Syst. Man, Cybern. Syst. 54, 2507–2516. doi:10.1109/tsmc.2023.3344382
Gan, N., Sun, Z., Zhang, Z., Xu, S., Liu, P., and Qin, Z. (2021). Data-driven fault diagnosis of lithium-ion battery overdischarge in electric vehicles. IEEE Trans. Power Electron. 37 (4), 4575–4588. doi:10.1109/tpel.2021.3121701
Gao, W., Li, X., Ma, M., Fu, Y., Jiang, J., and Mi, C. (2020). Case study of an electric vehicle battery thermal runaway and online internal short-circuit detection. IEEE Trans. Power Electron. 36 (3), 2452–2455. doi:10.1109/tpel.2020.3013191
Giuliano, M. R., Advani, S. G., and Prasad, A. K. (2011). Thermal analysis and management of lithium–titanate batteries. J. Power Sources 196 (15), 6517–6524. doi:10.1016/j.jpowsour.2011.03.099
Guo, W., and He, M. (2022). An optimal relevance vector machine with a modified degradation model for remaining useful lifetime prediction of lithium-ion batteries. Appl. Soft Comput. 124, 108967. doi:10.1016/j.asoc.2022.108967
Hao, W. Z., Tang, X., and Zhou, Y. (2024). Recent progress on mechanism of mechanical abuse. SAE Technical Paper 2024-01-2405.
Hashimoto, J., Suzuki, M., Sugahara, S., Hasegawa, M., and Otani, K. (2021). Advanced grid integration test platform for increased distributed renewable energy penetration in smart grids. IEEE Access 9, 34040–34053. doi:10.1109/access.2021.3061731
Hu, X., Gao, F., Xiao, Y., Wang, D., Gao, Z., Huang, Z., et al. (2024). Advancements in the safety of Lithium-Ion Battery: the trigger, consequence and mitigation method of thermal runaway. Chem. Eng. J. 481, 148450. doi:10.1016/j.cej.2023.148450
Hu, X., Zhang, K., Liu, K., Lin, X., Dey, S., and Onori, S. (2020). Advanced fault diagnosis for lithium-ion battery systems: a review of fault mechanisms, fault features, and diagnosis procedures. IEEE Ind. Electron. Mag. 14 (3), 65–91. doi:10.1109/mie.2020.2964814
Huang, H., Ghias, A. M. Y. M., Acuna, P., Dong, Z., Zhao, J., and Reza, Md S. (2024). A fast battery balance method for a modular-reconfigurable battery energy storage system. Appl. Energy 356, 122470. doi:10.1016/j.apenergy.2023.122470
Huang, W., Feng, X., Han, X., Zhang, W., and Jiang, F. (2021). Questions and answers relating to lithium-ion battery safety issues. Cell Rep. Phys. Sci. 2 (1), 100285. doi:10.1016/j.xcrp.2020.100285
Huria, T., Ceraolo, M., Gazzarri, J., and Jackey, R. (2012). “High fidelity electrical model with thermal dependence for characterization and simulation of high power lithium battery cells,” in 2012 IEEE International Electric Vehicle Conference, Greenville, SC, USA, 04-08 March 2012 (IEEE), 1–8.
Hussain, I., Das, D. C., Sinha, N., Latif, A., Hussain, S. M. S., and Ustun, T. S. (2020a). Performance assessment of an islanded hybrid power system with different storage combinations using an FPA-tuned two-degree-of-freedom (2DOF) controller. Energies 13, 5610. doi:10.3390/en13215610
Hussain, S. M. S., Aftab, M. A., Ali, I., and Ustun, T. S. (2020b). IEC 61850 based energy management system using plug-in electric vehicles and distributed generators during emergencies. Int. J. Electr. Power and Energy Syst. 119, 105873. doi:10.1016/j.ijepes.2020.105873
Juarez-Robles, D., Vyas, A. A., Fear, C., Jeevarajan, J. A., and Mukherjee, P. P. (2020). Overcharge and aging analytics of Li-ion cells. J. Electrochem. Soc. 167 (9), 090547. doi:10.1149/1945-7111/ab9569
Kaushik, S., Mehta, T., Chand, P., Sharma, S., and Kumar, G. (2024). Recent advancements in cathode materials for high-performance Li-ion batteries: progress and prospects. J. Energy Storage 97, 112818. doi:10.1016/j.est.2024.112818
Keshan, H., and Thornburg, J. (2016). “Comparison of lead-acid and lithium ion batteries for stationary storage in off-grid energy systems,” in 4th IET clean energy and technology conference (CEAT 2016) (Malaysia: Kuala Lumpur), 1–7.
Khosravi, N., Dowlatabadi, M., Abdelghany, M. B., Tostado-Véliz, M., and Jurado, F. (2024). Enhancing battery management for HEVs and EVs: a hybrid approach for parameter identification and voltage estimation in lithium-ion battery models. Appl. Energy 356, 122364. doi:10.1016/j.apenergy.2023.122364
Kim, H., Moon, J., Hong, D., Cha, E., and Yun, B. (2021). Prediction of critical heat flux for narrow rectangular channels in a steady state condition using machine learning. Nucl. Eng. Technol. 53 (6), 1796–1809. doi:10.1016/j.net.2020.12.007
Kim, J., and Kowal, J. (2022). A method for detecting the existence of an over-discharged cell in a lithium-ion battery pack via measuring total harmonic distortion. Batteries 8 (3), 26. doi:10.3390/batteries8030026
Kumar, R. (2024). “Lithium-ion battery for electric transportation: types, components, pack design, and technology,” in Energy efficient vehicles (Boca Raton, FL: CRC Press), 156–172.
Kumar, R. R., Bharatiraja, C., Udhayakumar, K., Devakirubakaran, S., Sathiya Sekar, K., and Mihet-Popa, L. (2023). Advances in batteries, battery modeling, battery management system, battery thermal management, SOC, SOH, and charge/discharge characteristics in EV applications. IEEE Access 11, 105761–105809. doi:10.1109/access.2023.3318121
Kurzweil, P., Frenzel, B., and Scheuerpflug, W. (2022). A novel evaluation criterion for the rapid estimation of the overcharge and deep discharge of lithium-ion batteries using differential capacity. Batteries 8 (8), 86. doi:10.3390/batteries8080086
Lai, X., Yi, W., Kong, X., Han, X., Zhou, L., Sun, T., et al. (2020). Online detection of early-stage internal short circuits in series-connected lithium-ion battery packs based on state-of-charge correlation. J. Energy Storage 30, 101514. doi:10.1016/j.est.2020.101514
Lalinde, I., Berrueta, A., Arza, J., Sanchis, P., and Ursúa, A. (2024). On the characterization of lithium-ion batteries under overtemperature and overcharge conditions: identification of abuse areas and experimental validation. Appl. Energy 354, 122205. doi:10.1016/j.apenergy.2023.122205
Lalinde, I., Berrueta, A., José Valera, J., Arza, J., Sanchis, P., and Ursúa, A. (2022). Perspective chapter: thermal runaway in LIBs.
Le, P.-A., Vuong, D. T., Natsuki, J., and Natsuki, T. (2023). Overview of the thermal runaway in lithium-ion batteries with application in electric vehicles: working principles, early warning, and future outlooks. Energy and Fuels 37 (22), 17052–17074. doi:10.1021/acs.energyfuels.3c02548
Li, Z., Gao, M., Zhao, X., Cai, X., and Zhang, Y. (2024). Heat generation effect and failure mechanism of pouch-type lithium-ion battery under over-discharge for electric vehicle. J. Energy Storage 76, 109759. doi:10.1016/j.est.2023.109759
Liao, H., Huang, B., Cui, Y., Qin, H., Liu, X., and Xu, H. (2022). Research on a fast detection method of self-discharge of lithium battery. J. Energy Storage 55, 105431. doi:10.1016/j.est.2022.105431
Linja-Aho, V. (2024). Assessing the electrical risks in electric vehicle repair: results for use in developing safe working practices and regulations. IEEE Ind. Appl. Mag. 30, 32–41. doi:10.1109/mias.2024.3387142
Liu, B., Jia, Y., Yuan, C., Wang, L., Gao, X., Yin, S., et al. (2020). Safety issues and mechanisms of lithium-ion battery cell upon mechanical abusive loading: a review. Energy Storage Mater. 24, 85–112. doi:10.1016/j.ensm.2019.06.036
Liu, Q., Ma, J., Zhao, X., Zhang, K., Xiangli, K., Meng, D., et al. (2023b). Voltage fault diagnosis and misdiagnosis analysis of battery systems using the modified Shannon entropy in real-world electric vehicles. J. Energy Storage 73, 109287. doi:10.1016/j.est.2023.109287
Liu, S., and Kang, L. (2023). Thermal monitoring of lithium-ion batteries based on machine learning and fibre Bragg grating sensors. Trans. Inst. Meas. Control 45 (8), 1570–1578. doi:10.1177/01423312221143776
Liu, S., Nie, Y., Tang, A., Li, J., Yu, Q., and Wang, C. (2023a). Online health prognosis for lithium-ion batteries under dynamic discharge conditions over wide temperature range. ETransportation 18, 100296. doi:10.1016/j.etran.2023.100296
Mago, H. (2024). “Application of active balancing strategies for heterogeneous battery cells,”. PhD diss. (Ingolstadt, Germany: Technische Hochschule Ingolstadt).
Menale, C., Constà, S., Sglavo, V., Della Seta, L., and Bubbico, R. (2022). Experimental investigation of overdischarge effects on commercial Li-ion cells. Energies 15 (22), 8440. doi:10.3390/en15228440
Meng, J., Boukhnifer, M., Delpha, C., and Diallo, D. (2020). Incipient short-circuit fault diagnosis of lithium-ion batteries. J. Energy Storage 31, 101658. doi:10.1016/j.est.2020.101658
Mishra, R., Anne, M., Das, S., Chavva, T., Shelke, M. V., and Pol, V. G. (2024). Glory of fire retardants in Li-ion batteries: could they Be intrinsically safer? Adv. Sustain. Syst., 2400273. doi:10.1002/adsu.202400273
Mitra, D., and Mukhopadhyay, S. (2024). Detection and isolation of faults in a lithium-ion battery pack using a switched architecture of equivalent cell diagnosers. J. Energy Storage 90, 111811. doi:10.1016/j.est.2024.111811
Obrien, V., Rao, V. S., and Trevizan, R. D. (2023). Detection of false data injection attacks in battery stacks using input noise-aware nonlinear state estimation and cumulative sum algorithms. IEEE Trans. Industry Appl. 59, 7800–7812. doi:10.1109/tia.2023.3308548
Olabi, A. G., Abbas, Q., Shinde, P. A., and Abdelkareem, M. A. (2023). Rechargeable batteries: technological advancement, challenges, current and emerging applications. Energy 266, 126408. doi:10.1016/j.energy.2022.126408
Ouyang, D., Weng, J., Chen, M., Wang, J., and Wang, Z. (2023). Investigation on topographic, electrochemical and thermal features of aging lithium-ion cells induced by overcharge/over-discharge cycling. J. Energy Storage 68, 107799. doi:10.1016/j.est.2023.107799
Parekh, M. H., Li, B., Palanisamy, M., Adams, T. E., Tomar, V., and Pol, V. G. (2020). In situ thermal runaway detection in lithium-ion batteries with an integrated internal sensor. ACS Appl. Energy Mater. 3 (8), 7997–8008. doi:10.1021/acsaem.0c01392
Park, S., Song, Y., and Kim, S. W. (2024). Simultaneous diagnosis of cell aging and internal short circuit faults in lithium-ion batteries using average leakage interval. Energy 290, 130220. doi:10.1016/j.energy.2023.130220
Patel, A. (2023). “Numerical investigation on the thermal behavior of Li-ion batteries under various cooling methods,”. Master’s thesis (Enschede: University of Twente).
Raijmakers, L. H. J., Danilov, D. L., Eichel, R.-A., and Notten, P. H. L. (2019). A review on various temperature-indication methods for Li-ion batteries. Appl. energy 240, 918–945. doi:10.1016/j.apenergy.2019.02.078
Ralls, A. M., Leong, K., Clayton, J., Fuelling, P., Mercer, C., Vincent, N., et al. (2023). The role of lithium-ion batteries in the growing trend of electric vehicles. Materials 16 (17), 6063. doi:10.3390/ma16176063
Rana, S., Kumar, R., and Bharj, R. S. (2023). Current trends, challenges, and prospects in material advances for improving the overall safety of lithium-ion battery pack. Chem. Eng. J. 463, 142336. doi:10.1016/j.cej.2023.142336
Ranga, M. R., Aduru, V. R., Krishna, N. V., Rao, K. D., Alsaif, F., Alsulamy, S., et al. (2023). An unscented kalman filter-based robust state of health prediction technique for lithium ion batteries. Batteries 9, 376. doi:10.3390/batteries9070376
Rangarajan, S. S., Poyyamani Sunddararaj, S., Sudhakar, A. V. V., Kumar Shiva, C., Subramaniam, U., Randolph Collins, E., et al. (2022). Lithium-ion batteries—the crux of electric vehicles with opportunities and challenges. Clean. Technol. 4 (4), 908–930. doi:10.3390/cleantechnol4040056
Rao, C. K., Sahoo, S. K., and Yanine, F. F. (2024c). “Smart energy management and monitoring system for electric vehicles with IoT integration,” in Electric vehicle design: design, simulation and applications, 57–80.
Rao, K. D., Anand, N. V., Pandraju, T. K. S., Alsaif, F., and Ustun, T. S. (2024b). Optimally tuned gated recurrent unit neural network-based state of health estimation scheme for lithium ion batteries. IEEE Access 12, 58597–58607. doi:10.1109/access.2024.3392432
Rao, K. D., Chander, A. H., and Ghosh, S. (2021). Robust observer design for mitigating the impact of unknown disturbances on state of charge estimation of lithium iron phosphate batteries using fractional calculus. IEEE Trans. Veh. Technol. 70 (4), 3218–3231. doi:10.1109/tvt.2021.3066249
Rao, K. D., Chappa, A., Chaitanya, S., Hemachander, A., Teja, B. P., Dawn, S., et al. (2024a). Fractional order modeling based optimal multistage constant current charging strategy for lithium iron phosphate batteries. Energy Storage 6 (2), e593. doi:10.1002/est2.593
Rao, K. D., Ramakrishna, A., Ramesh, M., Koushik, P., Dawn, S., Pavani, P., et al. (2024d). A hyperparameter-tuned LSTM technique-based battery remaining useful life estimation considering incremental capacity curves. IEEE Access 12, 127259–127271. doi:10.1109/access.2024.3450871
Rao, K. D., Reddy, K. K., Koushik, P., Avinash, B., and Madhavi, D. L. (2023b). “Performance analysis of lithium-ion battery considering round trip efficiency,” in 2023 IEEE 2nd International Conference on Industrial Electronics: Developments and Applications (ICIDeA), Imphal, India, 29-30 September 2023 (IEEE), 555–559.
Rao, K. D., Srinivas, K. Y. S., Sucharita, L., Vineela, T., and Daveed, A. (2023c). “Lithium ion battery modeling and state of charge estimation using kalman filter based observer,” in 2023 2nd International Conference for Innovation in Technology (INOCON), Bangalore, India, 03-05 March 2023 (IEEE), 1–6.
Rao, K. D., Taddi, M., Sriramula, T., Kumar Baliga, D., Simhadri, A., and Panigrahy, P. S. (2023a). “Detection of cyber attacks on wireless BMS of electric vehicles using long short-term memory networks,” in 2023 7th International Conference on Computation System and Information Technology for Sustainable Solutions (CSITSS), Bangalore, India, 02-04 November 2023 (IEEE), 1–6.
Roth, T., Streck, L., Graule, A., Niehoff, P., and Jossen, A. (2023). Relaxation effects in self-discharge measurements of lithium-ion batteries. J. Electrochem. Soc. 170 (2), 020502. doi:10.1149/1945-7111/acb669
Sadhukhan, C., Mitra, S. K., Bhattacharyya, S., Almatrafi, E., Saleh, B., and Naskar, M. K. (2022). Modeling and simulation of high energy density lithium-ion battery for multiple fault detection. Sci. Rep. 12 (1), 9800. doi:10.1038/s41598-022-13771-4
Schmid, M., Gebauer, E., and Endisch, C. (2021). Structural analysis in reconfigurable battery systems for active fault diagnosis. IEEE Trans. Power Electron. 36 (8), 8672–8684. doi:10.1109/tpel.2021.3049573
Seba, A. M., Gemeda, K. A., and Ramulu, P. J. (2024). Prediction and classification of IoT sensor faults using hybrid deep learning model. Discov. Appl. Sci. 6 (1), 9. doi:10.1007/s42452-024-05633-7
Shahid, S., and Agelin-Chaab, M. (2022). A review of thermal runaway prevention and mitigation strategies for LIBs. Energy Convers. Manag. X, 100310. doi:10.1016/j.ecmx.2022.100310
She, C., Bin, G., Wang, Z., and Zhang, L. (2024). Influencing factor-decoupled battery ageing assessment for real-world electric vehicles based on fusion of fuzzy logic and neural network. IEEE Trans. Transp. Electrification 1, 3405184. doi:10.1109/tte.2024.3405184
Shen, D., Yang, D., Lyu, C., Ma, J., Hinds, G., Sun, Q., et al. (2024b). Multi-sensor multi-mode fault diagnosis for lithium-ion battery packs with time series and discriminative features. Energy 290, 130151. doi:10.1016/j.energy.2023.130151
Shen, R., Quan, Y., McIntosh, J. D., Salem, A., and Wang, Q. (2024a). Fire safety of battery electric vehicles: hazard identification, detection, and mitigation. SAE Int. J. Electrified Veh. 13. doi:10.4271/14-13-03-0024
Simpa, P., Solomon, N. O., Adenekan, O. A., and Obasi, S. C. (2024). The safety and environmental impacts of battery storage systems in renewable energy. World J. Adv. Res. Rev. 22 (2), 564–580. doi:10.30574/wjarr.2024.22.2.1398
Sun, T., Chen, J., Wang, S., Chen, Q., Han, X., and Zheng, Y. (2023). Aging mechanism analysis and capacity estimation of lithium-ion battery pack based on electric vehicle charging data. Energy 283, 128457. doi:10.1016/j.energy.2023.128457
Svetlík, J., Tancos, Z., Tancos, P., Markova, I., and Slastan, K. (2024). Large-scale fire tests of battery electric vehicle (BEV): Slovak case study. Appl. Sci. 14 (10), 4013. doi:10.3390/app14104013
Tappeta, V. S. R., Appasani, B., Patnaik, S., and Ustun, T. S. (2022). A review on emerging communication and computational technologies for increased use of plug-in electric vehicles. Energies 15, 6580. doi:10.3390/en15186580
Tian, L., Dong, C., Mu, Y., Yu, X., and Jia, H. (2024). Online lithium-ion battery intelligent perception for thermal fault detection and localization. Heliyon 10 (4), e25298. doi:10.1016/j.heliyon.2024.e25298
Tran, M.-K., and Fowler, M. (2020). A review of lithium-ion battery fault diagnostic algorithms: current progress and future challenges. Algorithms 13 (3), 62. doi:10.3390/a13030062
Ulutas, A., Altas, I. H., Onen, A., and Ustun, T. S. (2020). Neuro-fuzzy-based model predictive energy management for grid connected microgrids. Electronics 9, 900. doi:10.3390/electronics9060900
Ustun, T., Ozansoy, C., and Zayegh, A. (2012). “Simulation of communication infrastructure of a centralized microgrid protection system based on IEC 61850-7-420,” in 2012 IEEE Third International Conference on Smart Grid Communications (SmartGridComm), Tainan, Taiwan, 05-08 November 2012 (IEEE), 492–497.
Ustun, T. S., and Ayyubi, S. (2019). Automated network topology extraction based on graph theory for distributed microgrid protection in dynamic power systems. Electronics 8, 655. doi:10.3390/electronics8060655
Ustun, T. S., Hussain, S. M. S., Ulutas, A., Onen, A., Roomi, M. M., and Mashima, D. (2021b). Machine learning-based intrusion detection for achieving cybersecurity in smart grids using IEC 61850 GOOSE messages. Symmetry 13, 826. doi:10.3390/sym13050826
Ustun, T. S., Hussain, S. M. S., Yavuz, L., and Onen, A. (2021a). Artificial intelligence based intrusion detection system for IEC 61850 sampled values under symmetric and asymmetric faults. IEEE Access 9, 56486–56495. doi:10.1109/access.2021.3071141
Wang, J., Zhang, S., and Hu, X. (2021). A fault diagnosis method for lithium-ion battery packs using improved RBF neural network. Front. Energy Res. 9, 702139. doi:10.3389/fenrg.2021.702139
Wang, X.-X., Qiu-Tong, L., Zhou, X.-Y., Hu, Y.-M., and Guo, X. (2024). Monitoring thermal runaway of LIBs by means of gas sensors. Sensors Actuators B Chem., 135703. doi:10.1016/j.snb.2024.135703
Wu, M., Du, W., Zhang, F., Zhao, N., Wang, J., Wang, L., et al. (2022). Fault diagnosis method for lithium-ion battery packs in real-world electric vehicles based on K-means and the fréchet algorithm. ACS omega 7 (44), 40145–40162. doi:10.1021/acsomega.2c04991
Wu, X., Zhixin, W., Wen, T., Du, J., Sun, J., and Shtang, A. A. (2023). Research on short-circuit fault-diagnosis strategy of lithium-ion battery in an energy-storage system based on voltage cosine similarity. J. Energy Storage 71, 108012. doi:10.1016/j.est.2023.108012
Xiong, R., Sun, X., Meng, X., Shen, W., and Sun, F. (2024). Advancing fault diagnosis in next-generation smart battery with multidimensional sensors. Appl. Energy 364, 123202. doi:10.1016/j.apenergy.2024.123202
Xu, J., Cai, X., Cai, S., Shao, Y., Hu, C., Lu, S., et al. (2023). High-energy lithium-ion batteries: recent progress and a promising future in applications. Energy and Environ. Mater. 6 (5), e12450. doi:10.1002/eem2.12450
Xu, X., Wang, H., Zhang, N., Liu, Z., and Wang, X. (2017). Review of the fault mechanism and diagnostic techniques for the range extender hybrid electric vehicle. IEEE Access 5, 14234–14244. doi:10.1109/access.2017.2725298
Xu, Y., Ge, X., and Shen, W. (2024). Multi-objective nonlinear observer design for multi-fault detection of lithium-ion battery in electric vehicles. Appl. Energy 362, 122989. doi:10.1016/j.apenergy.2024.122989
Yuan, H., Cui, N., Li, C., Cui, Z., and Chang, L. (2023). Early stage internal short circuit fault diagnosis for lithium-ion batteries based on local-outlier detection. J. Energy Storage 57, 106196. doi:10.1016/j.est.2022.106196
Zhang, K., Jiang, L., Deng, Z., Xie, Y., Couture, J., Lin, X., et al. (2023c). An early soft internal short-circuit fault diagnosis method for lithium-ion battery packs in electric vehicles. IEEE/ASME Trans. Mechatronics 28 (2), 644–655. doi:10.1109/tmech.2023.3234770
Zhang, X., Chen, S., Zhu, J., and Gao, Y. (2023b). A critical review of thermal runaway prediction and early-warning methods for lithium-ion batteries. Energy Mater. Adv. 4, 0008. doi:10.34133/energymatadv.0008
Zhang, Y., Jiang, M., Zhou, Y., Zhao, S., and Yuan, Y. (2023a). Towards high-safety lithium-ion battery diagnosis methods. Batteries 9 (1), 63. doi:10.3390/batteries9010063
Zhang, Z., Dong, S., Li, D., Liu, P., and Wang, Z. (2024). Prediction and diagnosis of electric vehicle battery fault based on abnormal voltage: using decision tree algorithm theories and isolated forest. Processes 12 (1), 136. doi:10.3390/pr12010136
Zhao, H., Chen, Z., Xing, S., Xiao, R., Shen, J., Liu, Y., et al. (2024c). Online surface temperature prediction and abnormal diagnosis of LIBs based on hybrid neural network and fault threshold optimization. Reliab. Eng. Syst. Saf. 243, 109798. doi:10.1016/j.ress.2023.109798
Zhao, J., Feng, X., Pang, Q., Fowler, M., Lian, Y., Ouyang, M., et al. (2024b). Battery safety: machine learning-based prognostics. Prog. Energy Combust. Sci. 102, 101142. doi:10.1016/j.pecs.2023.101142
Zhao, J., Feng, X., Tran, M.-K., Fowler, M., Ouyang, M., and Burke, A. F. (2024a). Battery safety: fault diagnosis from laboratory to real world. J. Power Sources 598, 234111. doi:10.1016/j.jpowsour.2024.234111
Zou, B., Zhang, L., Xue, X., Tan, R., Jiang, P., Ma, B., et al. (2023). A review on the fault and defect diagnosis of lithium-ion battery for electric vehicles. Energies 16 (14), 5507. doi:10.3390/en16145507
SD Self-discharge
ECM Equivalent Circuit Model
LIBs Lithium-ion Batteries
FDI Fault Detection and Isolation
EVs Electric Vehicles
EKF Extended Kalman Filter
BMS Battery Management Systems
RBF Radial Basis Function
ISC Internal Short Circuit
PNN Probabilistic Neural Network
TR Thermal Runaway
GRNN General Regression Neural Network
NiMH Nickel-Metal Hydride
SF Sensitivity Factor
NiCd Nickel-Cadmium
ICC Incremental Capacity Curves
SOC State of Charge
LOF Local Outlier Factor
SOH State of Health
DKF Dual Kalman Filter
ESC External Short Circuits
KF Kalman Filter
SEI Solid Electrolyte Interface
HNN Hybrid Neural Network
OCV Open Circuit Voltage
CNN Convolutional Neural Networks
HT High-temperature
LSTM Long-Short Term Memory
NT Normal-temperature
UKF Unscented Kalman Filter
EOL End of Line
OLS Ordinary Least Squares
ORI Ohmic Resistance Increase
GBDT Gradient Boosting Decision Tree
MAE Mean Absolute Error
GPR Gaussian Process Regression
SD Self-discharge
ECM Equivalent Circuit Model
Keywords: lithium-ion batteries, electric vehicles, thermal runaway, fault diagnosis, battery management system
Citation: Rao KD, Lakshmi Pujitha NN, Rao Ranga M, Manaswi C, Dawn S, Ustun TS and Kalam A (2025) Fault mitigation and diagnosis for lithium-ion batteries: a review. Front. Energy Res. 13:1529608. doi: 10.3389/fenrg.2025.1529608
Received: 17 November 2024; Accepted: 17 January 2025;
Published: 19 February 2025.
Edited by:
Ahmad Arabkoohsar, Technical University of Denmark, DenmarkReviewed by:
Wei Zuo, Wuhan University of Science and Technology, ChinaCopyright © 2025 Rao, Lakshmi Pujitha, Rao Ranga, Manaswi, Dawn, Ustun and Kalam. This is an open-access article distributed under the terms of the Creative Commons Attribution License (CC BY). The use, distribution or reproduction in other forums is permitted, provided the original author(s) and the copyright owner(s) are credited and that the original publication in this journal is cited, in accordance with accepted academic practice. No use, distribution or reproduction is permitted which does not comply with these terms.
*Correspondence: K. Dhananjay Rao, kdhananjayrao@gmail.com; Taha Selim Ustun, selim.ustun@aist.go.jp; Akhtar Kalam, akhtar.kalam@vu.edu.au
Disclaimer: All claims expressed in this article are solely those of the authors and do not necessarily represent those of their affiliated organizations, or those of the publisher, the editors and the reviewers. Any product that may be evaluated in this article or claim that may be made by its manufacturer is not guaranteed or endorsed by the publisher.
Research integrity at Frontiers
Learn more about the work of our research integrity team to safeguard the quality of each article we publish.