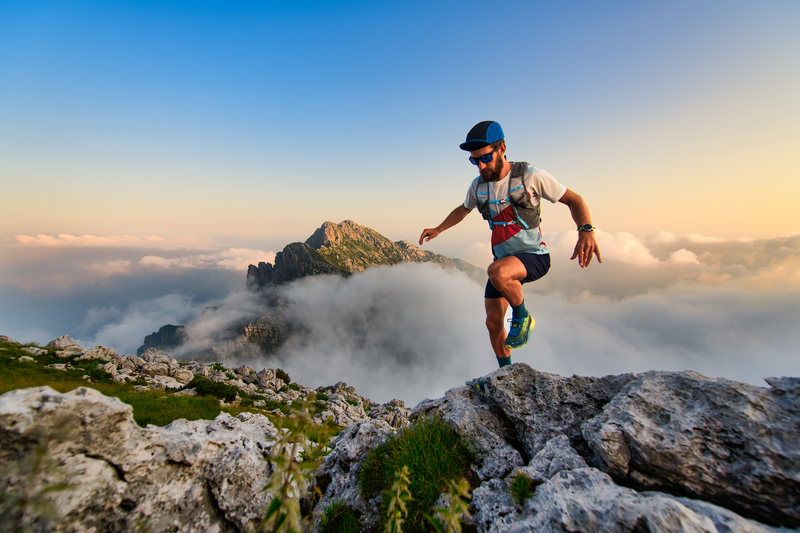
95% of researchers rate our articles as excellent or good
Learn more about the work of our research integrity team to safeguard the quality of each article we publish.
Find out more
ORIGINAL RESEARCH article
Front. Energy Res. , 27 March 2025
Sec. Smart Grids
Volume 13 - 2025 | https://doi.org/10.3389/fenrg.2025.1519053
This article is part of the Research Topic Advancing Demand Response in Renewable Smart Grid for a Sustainable Future View all 7 articles
Accurate forecasting of residential flexible load is imperative for effective demand-side management, ensuring efficient energy utilisation and power supply-demand stability. Conventional methods encounter challenges due to the uncertainty and volatility of residential flexible resources.This study proposes a meta-learning architecture based on Temporal Convolutional Networks (TCN). The proposed approach is comprised of three distinct stages. Firstly, preprocessing with Concatenated Fourier Features (CFF) is employed to accentuate periodicity. Secondly, a TCN base model is utilised to capture both long-term and short-term dependencies. Thirdly, a two-tiered learning process is implemented to adapt features from load disaggregation to forecasting.The efficacy of the proposed method is evaluated using public datasets, and the results demonstrate its superiority to baseline models in terms of forecasting accuracy for flexible loads. The enhanced performance of the proposed method is attributed to the integration of feature extraction and model adaptation within a meta-learning framework.Future research could explore the incorporation of contextual information to further enhance performance.
With the increasing penetration of diverse distributed renewable energy resources (DER), such as solar and wind power (Wang et al., 2023a), into the smart grid, their climate-dependent characteristics inject uncertainty and volatility into the power supply. To counteract the challenge, demand response (DR) has emerged as an essential mechanism to stabilize the power supply and demand by either incentivizing or directly controlling consumer power consumption patterns. However, effective implementation of demand response requires visibility of the proportion of demand-side controllable loads and their behavior characteristics, which cannot be discerned solely through direct smart meter measurements (Wang et al., 2023). Consequently, non-intrusive load monitoring (NILM) is gaining recognition as a critical technology for demand-side energy management, which disaggregates real-time household energy consumption into individual appliance usage without additional meters deployed for each appliance.
Starting from Hart in 1992, NILM can be roughly classified into steady-state and transient-state methods according to the feature engineering process. Transient-state NILM commonly detects the operation state changes according to the high-frequency features after event detection, including transient power changes (Figueiredo et al., 2013), Weighted Power Recurrence Graphs (Wang et al., 2023b), and frequency domain component features extracted by Fast Fourier Transform (FFT) (Wu X et al., 2017), Wavelet Transform (Chang et al., 2013) or Hilbert Transform (Heo and Kim, 2021). While steady-state features include active power (Liu et al., 2021), reactive power (Bonfigli et al., 2017), V-I trajectory (Liu et al., 2018; Du et al., 2023), or steady-state harmonics (Kang and Kim, 2020). Transient-state methods commonly achieve better load disaggregation performance due to the deep features extracted during the switching event. However, its performance highly depends on the accuracy of event detection and is more sensitive to noise signal and power signal peaks. Besides, transient-state methods require the sampling rate to be in the order of kHz, posing a significant burden on the device computational capability, sensor installation cost, and network communication bandwidth. Therefore, low-frequency NILM is highlighted as a significant steady-state NILM method due to its high versatility and low sampling rate requirements. Taking a slice of active and/or reactive power sampled lower than 1 Hz, low-frequency NILM commonly relies on machine learning or deep learning algorithms to extract deep features and compensate for the information loss caused by the sampling frequency (Zhou et al., 2020). proposes a convolutional-based sequence-to-sequence (S2S) model to disaggregate the aggregate power consumption sequence to individual load consumption sequences. Furthermore, sequence-to-point (S2P) methods (Jia et al., 2021) are proposed to predict a single point’s load consumption based on future and past power slices, achieving better efficiency in capturing temporal dependencies and patterns. On this basis (Luan et al., 2023), has deployed the S2P in edge devices with lightweight structures for online load monitoring, ensuring fast energy disaggregation while preserving user’s privacy. In summary, the explorations of low-frequency NILM have achieved remarkable outcomes, leading to satisfactory accuracy.
Although NILM can disaggregate household load consumption in real-time, it is more important for the demand response scheme to forecast flexible load consumption and thus prepare various response strategies for energy usage management. Initially, machine learning (ML) models for load forecasting excel in the sense that they can be individualized into arbitrarily fine-granular time slots and regions, thereby leading to much improved load forecasting accuracy performance (Faustine and Pereira 2022; Kalakova et al., 2021). In particular, from a large volume of data describing historical load fluctuations over time, the ML models exhibit enjoy a deep representation learning capability to capture the highly nonlinear and unstructured patterns underlying the raw inputs. Considering the limitations of offline techniques, an online adaptive recurrent neural network (RNN) technique is proposed. This model has continuous learning capacity, adapting itself from newly arriving data by updating the RNN weights to accommodate new patterns or changes in data types (Fekri et al., 2021). Furthermore, a deep bidirectional LSTM-based sequence-to-sequence regression approach is proposed in (Mughees et al., 2021). The framework has an adaptive structure with a window-based strategy to capture both historical data and current demand, enhancing the accuracy of peak demand forecasting in the residential sector. Although this methodology enhances system responsiveness, the considerable computational requirements of the bidirectional LSTM framework may limit the applicability in environments constrained by processing capacity.
Although the aforementioned methods have demonstrated certain feasibility in household load forecasting, it is crucial to obtain appliance-level load forecasting information for user-side flexibility resource management to assist with demand response. To address this issue (Lin et al., 2019), employs sparse coding algorithms to decompose short-term cooling loads of buildings into their respective components. It then applies Backpropagation Neural Networks (BPNN) and Auto-Regressive Integrated Moving Average (ARIMA) models to forecast each component separately, thus improving the overall cooling load forecasting accuracy (Pirbazari et al., 2020). focuses on decomposing total household load using Denoising Auto Encoder (DAE) to identify appliance-level load profiles. Based on the decomposition, a Gradient Boosting Regression Tree (GBRT) load forecasting model is constructed, demonstrating the potential benefit of load disaggregation in enhancing load forecasting accuracy. Furthermore (Welikala et al., 2017), improves total power demand forecasting accuracy by utilizing a Karhunen-Loeve Expansion (KLE)-based NILM decomposition method, incorporating appliance usage patterns and their priori probabilities. To refine existing methods (Langevin et al., 2023), develops a VAE-based NILM model to refine appliance load information. It then constructed TCN model based on the disaggregated results to improve short-term load forecasting accuracy for the entire household. However, the aforementioned studies predict appliance consumption after load disaggregation, meaning the disaggregation and forecasting models are trained independently, without sharing or transferring deep load features or important model parameters, thus leading to less precise forecasting outcomes.
Subsequently, a short-term residential load forecasting model using a TCN in emphasizes the impressive tracking properties of the TCN architecture for highly volatile residential loads (Peng and Liu 2020). Distinct from RNNs and LSTMs, TCNs obviate the issue of vanishing gradients and are intrinsically capable of capturing longer dependencies in data without extensive historical input requirements. This attribute renders TCNs particularly advantageous for scenarios where accuracy in real-time forecasting is crucial, and operational conditions are subject to frequent alterations (Ahajjam et al., 2022). However, the existing ML model often fail to perform well across diverse operational scenarios, exhibiting a lack of robustness when confronted with data or conditions not represented in the training set.
Addressing this practical problem illuminates fundamental questions: Can general principles of load forecasting be discerned and applied across diverse datasets? If so, what mechanisms could facilitate this knowledge transfer? Meta-learning excels by enabling the adaptation of learned representations across various load forecasting tasks, significantly enhancing model transferability and applicability in diverse environments (Raghu et al., 2020). In this paper, a meta-learning framework that integrates TCN with differentiable closed-form solution optimization is proposed, enabling the adaptation of feature learning from load disaggregation tasks to load forecasting. Initially, the extensive data preprocessing, including CFF transformations, enhances data quality and expressiveness. Taking the load disaggregation task as the training tasks and the load forecasting task as the testing task, the base TCN captures both long and short-term dependencies, optimized via a two-tiered learning process that rapidly adapts to new tasks. This approach not only improves prediction accuracy but also ensures robust generalization and adaptability across varying load conditions. The contribution of the proposed method for flexible load forecasting is as follows:
(1) The TCN-Meta framework enhances flexible load forecasting by integrating load disaggregation and forecasting tasks within a meta-learning structure. By sharing deep-layer features and parameters across tasks, it mitigates information loss compared to separated task training processes and thus improves load forecasting accuracy.
(2) The proposed model enables multiscale load feature extraction, including periodic and transient dynamics, across diverse load types through TCN blocks with Concatenated Fourier Features (CFF), demonstrating broad applicability across complex scenarios.
(3) A novel two-tiered parameters optimization process is embedded in the meta-learning framework, enabling rapid adaptation from load disaggregation to forecasting tasks. This strategy minimizes training complexity while ensuring synergistic task alignment.
(4) Extensive validation on public datasets proves that TCN-Meta outperforms state-of-the-art load forecasting models (Transformer, LSTM, etc.) across various flexible load types and diverse load behaviours, demonstrating its effectiveness and feasibility for flexible load forecasting through an integrated and adaptive mechanism.
The TCN-Meta model is a comprehensive framework designed for adaptive load forecasting. It integrates three crucial components: a data preprocessing module, a TCN-based base learner for capturing temporal dependencies, and a meta-learning approach utilizing differentiable closed-form solvers for efficient optimization. The framework is structured to ensure both high forecasting accuracy and adaptability through a dual-loop learning process, enabling it to quickly adapt to new forecasting tasks. The model’s architecture is illustrated in Figure 1, with each component contributing uniquely to the overall performance.
During the preprocessing phase, the input time-series dataset, denoted as X, comprises distinct electrical load types: vehicle load, heating load, and photovoltaic (PV) load. This dataset, which includes the aggregate power consumption and the individual consumption profiles of each appliance, undergoes a rigorous cleaning process. This entails addressing missing data points, rectifying anomalies, and ensuring overall data integrity. The dimensions of X are defined as a × b, where a, set to 20, represents the length of the time window to capture the sequential nature of power consumption and b denotes the number of input variables, corresponding to the different load types. To address the complexities introduced by high-dimensional data and to hasten the convergence of our analytical algorithms X is standardized. This normalization process aligns the scale of various features, thus enabling a more robust and expeditious analytical convergence. Lastly, a CFF transformation is carried out. This step involves capturing multiscale periodic features present in the data using Fourier basis functions of multiple scale parameters. This transformation not only enhances the expressiveness of the data but also improves the model’s capability to discern different frequency components. It provides a rich set of information for subsequent learning through TCN.The output Y of our model is structured to reflect the specific load types, with dimensions c × d. Here, c corresponds to the number of load types, which in this case is three—vehicle, heating, and PV loads. The dimension d represents the length of the prediction and disaggregation time window, which is set to 1, indicating that the model predicts the power consumption for the subsequent time point.
The Random Fourier Feature (RFF) approach provides a robust framework for capturing the inherent periodicity present in time-series data. This method is deeply anchored in Bochner’s theorem, which elegantly states that a continuous, shift-invariant kernel defined on Euclidean space is essentially the Fourier transform of a positive measure. The practical implementation of RFF begins by constructing a random frequency matrix B, where B is of size d × 1 and each element bi is independently drawn from a standard normal distribution N (0,1). The resultant RFF mapping, which can be explicitly defined, is:
Here, X represents the input data points, and the transformation aims to capture the underlying frequencies within these data points.
While the random Fourier features layer endows TCN with the ability to learn high-frequency patterns, one major drawback is the need to perform a hyperparameter sweep for each task and dataset to avoid over or underfitting.
This paper overcomes this limitation with a simple scheme of concatenating multiple Fourier basis functions with diverse scale parameters, called Concatenated Fourier Features. Unlike the standard RFF where a single random matrix B is used, CFF leverages a sequence of such matrices, denoted B1, B2, … , Bs, to capture a wider range of frequency responses. The variable s denotes the total count of unique frequency matrices that are harnessed to create a comprehensive multi-frequency representation of the input data. Each matrix corresponds to a different scale hyperparameter σ2 S, and is used to sample from a normal distribution N (0, σ2 S). This leads to a multi-frequency representation of the input data:
In this transformation, each set of sine and cosine functions expands the input feature space to capture periodicities at different scales. For a TCN that processes time-series data, the CFF provides a detailed frequency domain picture, enabling the network to identify and exploit patterns across various temporal resolutions. This property is particularly beneficial for applications like load forecasting, where input signals may contain overlapping cycles of activity ranging from minutes to seasons. By implementing CFF, we also circumvent the need for meticulous hyperparameter tuning for each dataset and task. The combination of features at multiple scales offers robustness to the model, making it less prone to overfitting or underfitting. The TCN, augmented with CFF, is capable of adapting to diverse datasets and potentially reducing the requirement for iterative hyperparameter optimization, thus streamlining the training process.
Within the meta-learning framework, the optimization and updating of model parameters make use of differentiable closed-form solutions, such as ridge regression. This replaces traditional iterative optimization methods like gradient descent, thereby substantially enhancing the efficiency of the optimization process. This approach allows the model to quickly adapt to new prediction tasks. Both the base parameters and meta-parameters undergo a two-tiered learning process under this framework. The inner-loop optimization hones in on optimizing the base parameters for individual specific tasks, while the outer-loop optimization is responsible for meta-parameter optimization across tasks. This meta-learning framework ensures that the model not only exhibits sound generalization capabilities but also infers high flexibility and adaptability when confronted with new load conditions.
On the basis of one-dimensional causal convolution, expansion convolution and residual convolution are added to the TCN model, and convolution is used to process data in parallel so as to extract characteristics across time steps. Its complete structure is shown in Figure 2. Causal dilative convolution is composed of causal convolution and dilative convolution, and the structure is shown in Figure 3. The causal convolution is composed of one-dimensional convolution, whose output is obtained by the combination of the input at this time and the input at an earlier time in the previous layer. It is a strict time-constraint model, which is suitable for mining the potential features of time series data. Expansive convolution was initially proposed to solve the problem of information loss in the sampling process of the image domain. Unlike the traditional convolution layer, which only focuses on the characteristics of the local neighborhood, expansive convolution can obtain information in a larger receptive field so as to better capture the long-term dependencies in the sequence. Its expression is as follows:
Where i is the ith element in the sequence, j is the size of the filter, f(i) is the input sequence, F(i) is the output sequence, h(j) is the filter of length j, and d is the expansion factor.
Subsequent to the application of expansive convolution, which is instrumental in capturing long-term dependencies within the sequence, the network incorporates 1 × 1 convolutions—commonly referred to as pointwise convolutions—to enhance feature extraction. These convolutions operate independently on each channel of the input, thereby enabling the model to learn complex transformations specific to each channel. Furthermore, they facilitate the integration of features across different channels, effectively reducing the dimensionality of the input data and mitigating computational complexity. Consequently, this architectural choice enhances the overall efficiency of the network while preserving its capacity for rich and nuanced feature representation.
Skip connections are a widely used technique in deep learning that helps to maintain or improve network performance without compromising computational efficiency. Typically, the input to a deep neural network model undergoes a transformation through multiple layers. For instance, if x represents the input to a layer and (x) represents the transformed output of that layer, then in a TCN, a skip connection adds (x) to x, thus combining the input and transformed output. This helps to mitigate the vanishing gradient problem by allowing gradients to flow directly through the network. The process can be mathematically expressed as:
This formula denotes the iterative process across K layers of the network, where xk is the input to the kth layer, F represents the function performed by the layer, typically consisting of convolutions, and Activation represents a non-linear activation function.
In TCNs, modules such as expansion convolution, causal convolution, and residual connections, including skip connections and Dropout, form a residual block. This architectural choice aims to prevent gradient issues while enabling the network to learn deeper representations and facilitate the transfer of information across layers, thus enhancing the model’s generalization capability.
It is important to note that although TCN convolution blocks are adept at extracting features, they might not align perfectly with the task’s output space, especially when the task involves predictions over different domains or requires output in varied dimensions. To address this and improve the model’s adaptability, an additional linear layer is introduced at the end of the TCN. This layer provides a direct, learnable mapping to the desired output space and can be easily adapted for different tasks.
The output o of the entire network, after it has processed through K layers and passed through the final linear layer, can be represented as:
where xK is the output of the last residual block, and W and b are the weights and biases of the final linear layer, respectively. This final linear transformation ensures that the multidimensional features learned by the TCN are mapped onto the output space relevant to the specific task at hand.
In the meta-learning framework, tasks are divided into training tasks and testing tasks. In this paper, the training task is load disaggregation, while the testing task is load forecasting. As the training task, load disaggregation aims to extract the power consumption of individual appliances from total energy consumption data. It focuses on identifying the temporal patterns in the total load to disaggregate the appliance-level consumption at a specific historical timestamp. This task enables the model to capture key features of energy usage over time, allowing it to distinguish between the consumption profiles of different appliances. In contrast, our load forecasting task focuses on predicting the future power consumption of different appliances based on the existing power measurement.
The input of the meta-learning framework is a slice of the total power consumption Pt(t) over W timestamps (where W is odd). For the load disaggregation task, the output is the power consumption of each appliance Pa(t) at the (W+1)/2 timestamp. For the load forecasting task, the output is the power consumption of each appliance Pa(t) at the W+1 timestamp. The output of the load forecasting task is the power consumption of each appliance Pa(t) in the W+1 timestamps. That is to say, the load disaggregation task focuses on past data, while the load forecasting task focuses on predicting future data. However, both tasks share the same input Pt(t) over W timestamps, and the outputs are related by their temporal dependencies on the past and future timestamps respectively. Through sequences slicing, the two tasks compose two sets {Xd, Yd} and {Xp, Yp}, which denotes the load disaggregation task for training and the load forecasting task for testing respectively. f, fd, and fp are the meta-learning model, the load disaggregation model and the load predicting model separately. θd and θp are specific parameters for load disaggregation and load predicting models. ϕ* is the meta-parameter for the entire meta-learning model. The bi-level optimization problem can be formalized as:
In the above equations, the first optimization problem aims to find the best meta-parameters ϕ* that minimize the sum of the task-specific loss functions across all tasks, which encompasses both load forecasting and load monitoring tasks. The task-specific loss function,
For every individual task, such as load disaggregation and load forecasting, we start by optimizing the base parameters θd and θp over the support set {Xd, Yd} and {Xp, Yp} to obtain the optimal set of specific parameters θ *d and θ *p. This step leverages the loss function
The meta-learning framework is instrumental in not only bolstering the generalization capabilities of the model but also ensuring its adaptability and flexibility when confronted with novel scenarios of load conditions. This adaptive approach is especially crucial in the dynamic realm of power system load data, enabling the model to deliver fast and accurate predictions and monitoring in the face of constantly evolving conditions.
Within the meta-learning framework, the optimization and updating of model parameters make use of differentiable closed-form solutions, such as ridge regression. This replaces traditional iterative optimization methods like gradient descent, thereby substantially enhancing the efficiency of the optimization process. This approach allows the model to quickly adapt to new prediction tasks. Both the base parameters and meta-parameters undergo a two-tiered learning process under this framework. The inner-loop optimization hones in on optimizing the base parameters for individual specific tasks, while the outer-loop optimization is responsible for meta-parameter optimization across tasks. This meta-learning framework ensures that the model not only exhibits sound generalization capabilities but also infers high flexibility and adaptability when confronted with new load conditions.
In the realm of meta-learning, where the focus is on rapidly adapting models to a variety of tasks, the efficiency of the optimization process is paramount. The inner loop optimization is a critical component that directly influences the adaptability of the model. Originally, optimization-based meta-learning methods involved a computationally intensive bi-level optimization procedure, where gradients are backpropagated through the inner optimization steps, typically requiring second-order optimization methods.
However, the inner loop optimization within our meta-learning model simplifies this process. By leveraging a differentiable closed-form solution—specifically, a ridge regressor in the case of a mean squared error loss—this paper streamlines the optimization process, making it much more efficient and competitive with traditional learning methods that optimize on large datasets.
The inner loop optimization is described for a K-layered model parameterized by a set of meta parameters ϕ and base parameters θ. Then let gϕ: R→Rd be the meta learner where gϕ(x) = xk. The support set features obtained from the meta learner are denoted Xk. The inner loop thus solves the optimization problem:
This closed-from solution is differentiable, which enables gradient updates on the parameters of the meta-learner, ϕ. A bias term can be included for the closed-form ridge regressor by appending a scalar 1 to the feature vector gϕ. The result of training on a dataset is the restricted hypothesis class
Building upon the structural overview of the TCN-Meta model, this section provides a detailed description of the model’s training procedure, which is critical for ensuring both its adaptability and accuracy. The training process is composed of several phases, each essential for the model’s effective learning and ability to generalize across different tasks. These phases include data preprocessing, feature transformation, forward propagation through the network, loss calculation, backpropagation, and meta-learning optimization. The training process for both load disaggregation and load forecasting tasks follows a bi-level optimization approach, wherein the model learns task-specific parameters during the inner loop and updates shared meta-parameters during the outer loop. A comprehensive explanation of this process is outlined in Algorithm 1, shown in Algorithm 1.
The training process begins with data preprocessing, where the input time-series data x is standardized. Standardization ensures that the features share a similar scale, preventing any feature with a larger numerical range from dominating the learning process. This step is fundamental for stabilizing training and ensuring that the model learns efficiently. Following standardization, CFF are generated, transforming the data into a multi-frequency feature space. This transformation uses random frequency matrices B1, B2, … , Bs, drawn from a standard normal distribution, capturing periodic patterns across multiple frequency scales. The CFF process greatly enhances the model’s ability to detect complex temporal dependencies, which is especially valuable in load forecasting tasks where periodicity and long-term dependencies are prevalent.
Algorithm 1. The training process of the TCN-Meta architecture. TCN-Meta Model for Flexible Load Forecasting.
Once the data has been preprocessed and transformed, it is fed into the TCN model. The TCN is designed to capture both short-term and long-term dependencies by using causal convolutions. This ensures that the network only uses past information when predicting future values, preserving the temporal structure of the data. To further capture long-range dependencies, the model incorporates dilated convolutions and skip connections. Each convolutional layer is followed by an activation function, processing the input from the previous layer. The resulting feature maps are progressively refined through the network, leading to a final feature representation z, which is then passed to the next stages of the model. The final output is computed through a linear layer that maps the extracted features to the desired prediction space, generating the model’s output. In the inner loop of the training process, the base parameters θT for each task T (either disaggregation or forecasting) are optimized using the support set. This is where the model adapts to each task. The closed-form solution for updating θT is applied using the feature matrix
The gradients Gϕ of the meta-parameters ϕ are accumulated across all tasks, as shown in Step 29 of Algorithm 1. Once the gradients have been calculated, the meta-parameters are updated by gradient descent as step 32. After iterating through the support and query sets, the model’s parameters are optimized. The final meta-parameters ϕ* and the task-specific base parameters θd* and θq*are returned, as shown in Step 34 of Algorithm 1. These optimized parameters are then used to make predictions for future load consumption in load forecasting or load disaggregation tasks. By leveraging both the base parameters and meta-parameters, the model is able to achieve high performance on both tasks, making it suitable for dynamic energy consumption prediction.
The training process of the TCN-Meta model is characterized by a bi-level optimization strategy that enables quick adaptation to new tasks. In the inner loop, the base parameters θT are updated on the support set using a closed-form solution. In the outer loop, the meta-parameters ϕ are updated by accumulating gradients from the query set, allowing the model to generalize across tasks. This two-stage process ensures that the model is both accurate and adaptable, allowing it to effectively handle load disaggregation and load forecasting tasks. By utilizing a closed-form solution for base parameter optimization, the model achieves efficient training while maintaining the flexibility required to adapt to different forecasting tasks.
In the experiment section, the Austin area data from the Pecan Street Dataport dataset (Parson et al., 2015) is used as the evaluation dataset for both load disaggregation and load forecasting. This dataset includes 25 homes in Austin, Texas, with a recording period of 1 year and a sampling resolution of 1 min. The disaggregated and forecasted flexible components are categorized into vehicle load, heating load, and photovoltaic (PV) load. The vehicle load includes electric vehicles, the heating load consists of air compressors, air conditioners, water heaters, and furnaces, and the PV load represents the power generation from photovoltaic systems.
In load disaggregation and load forecasting, we mainly concerned about how close the estimates are to the ground truths. To this end, root mean squared Error (RMSE) and absolute percentage error (MAPE) are often chosen as the evaluation metrics. RMSE and MAE reflect the forecasting error ratio and the absolute forecasting error, respectively. They can be computed as follows:
In this study, we selected several commonly used time series prediction models as baselines to evaluate the performance of the proposed TCN-Meta model. These baseline models include TCN, CNN, LSTM, GRU, RNN, and Transformer. The input of the proposed TCN-Meta and baseline methods is only the aggregated power consumption. Table 1 gives their brief descriptions.
The performance of load disaggregation significantly impacts that of load forecasting in meta-learning structures. Figures 4–6 illustrate the disaggregation results for vehicle, heat, and PV loads. Despite the rapid slope and sharp edges in the power consumption patterns of vehicle loads, the base TCN-Meta model successfully tracks the transient changes, achieving satisfactory results with an RMSE of 0.05789 kW and an MAE of 0.04897 kW. For heat loads, the frequent and random transient variations in power consumption increase the difficulty of disaggregation. However, the proposed structure effectively tracks these changes, yielding an RMSE of 0.08862 kW and an MAE of 0.06423 kW. Although PV loads exhibit clear periodicity, the severe fluctuations in peak power generation degrade disaggregation accuracy. Nonetheless, the model achieves a low RMSE of 0.19823 kW and an MAE of 0.15482 kW.
In summary, the meta-learning structure delivers satisfactory disaggregation results for three typical flexible resources, establishing a strong foundation for subsequent load forecasting tasks.
Tables 2, 3 present a detailed comparison of the RMSE and MAE results between TCN-Meta and other baseline models, including TCN, CNN, LSTM, GRU, RNN, and Transformer, across three different types of loads: Vehicle-Load, PV-Load, and Heat-Load. TCN-Meta consistently outperformed all the baseline models, demonstrating its superior ability to handle diverse time series forecasting tasks.
For Vehicle-Load, TCN-Meta achieved the lowest RMSE of 0.04839 kW and the lowest MAE of 0.03858 kW, outperforming all other models by a significant margin. The TCN baseline, which shares the core architecture of TCN-Meta but without the meta-learning enhancements, also performed well with an RMSE of 0.06395 kW and an MAE of 0.05785 kW. This result highlights the strength of TCN for load disaggregation tasks, though the addition of meta-learning in TCN-Meta clearly provides a substantial improvement in accuracy. The Transformer model also showed competitive performance, achieving an RMSE of 0.06966 kW and an MAE of 0.05558 kW. However, other models, such as CNN and LSTM, with RMSEs of 0.18286 kW and 0.19423 kW respectively, showed less favorable results, indicating their relatively lower performance in Vehicle-Load prediction.
For PV-Load, TCN-Meta again outperformed the baseline models, recording an RMSE of 0.24991 kW and an MAE of 0.19989 kW, maintaining its superiority across the different load types. Interestingly, the performance of the TCN baseline was quite close to that of TCN-Meta, with an RMSE of 0.25436 kW and an MAE of 0.25136 kW, suggesting that the meta-learning component has less impact for PV-Load compared to other load types. The Transformer model followed closely, with an RMSE of 0.28742 kW and an MAE of 0.23812 kW, further validating its effectiveness in handling more complex load patterns. Meanwhile, models such as CNN, LSTM, and RNN displayed higher error rates, with CNN showing the highest RMSE of 0.38952 kW, indicating that convolutional models without temporal enhancements struggle more with this type of load forecasting.
In terms of Heat-Load, TCN-Meta again demonstrated the most accurate performance, achieving the lowest RMSE of 0.10081 kW and an exceptionally low MAE of 0.07990 kW. This highlights the model’s capability in predicting heat loads, which are often influenced by external factors such as weather conditions. The Transformer model also performed well in this category, with an RMSE of 0.12944 kW and an MAE of 0.11868 kW, making it the second-best performer. TCN, though not as effective as TCN-Meta, still recorded respectable results with an RMSE of 0.22355 kW and an MAE of 0.18971 kW. Other models, such as GRU, performed reasonably well with an RMSE of 0.17338 kW, while RNN and LSTM showed higher error rates, further emphasizing the limitations of recurrent architectures when applied to Heat-Load forecasting.
Overall, TCN-Meta consistently outperformed the baseline models across all load types, particularly excelling in minimizing MAE, which measures the average magnitude of prediction errors. This suggests that TCN-Meta is particularly suited for applications where precision is critical, such as in energy management and load forecasting for smart grids. Among the baseline models, TCN and Transformer stood out for their consistent performance across the board, validating the effectiveness of temporal convolution and attention mechanisms in time series tasks. GRU also performed well, particularly for PV-Load and Heat-Load, demonstrating the usefulness of its gating mechanisms for capturing complex dependencies in time series data. In contrast, RNN showed the highest RMSE and MAE values across all categories, highlighting its relative inefficiency in capturing the intricate patterns present in load time series data. This underscores the importance of selecting appropriate architectures for different forecasting tasks, with newer models like TCN and Transformer clearly providing more robust solutions for energy load prediction.
These results collectively highlight the superior performance of TCN-Meta, particularly in time series forecasting tasks where accuracy is crucial. The model’s ability to outperform both its base TCN architecture and other widely used models like Transformer and LSTM underscores the value of incorporating meta-learning into temporal convolutional networks. While Transformer showed competitive results and could be considered a strong baseline for future research, TCN-Meta consistently achieved the best results across all load types, solidifying its position as the optimal model for load disaggregation and similar forecasting applications.
In this study, we applied the TCN-Meta model to predict three different types of loads: Vehicle Load, Heat Load, and PV Load. By comparing the predictive performance of the TCN-Meta model with the actual load curves shown in Figures 7–9. We were able to identify both the strengths and challenges of the model under different load conditions.
For Vehicle Load, the TCN-Meta model generally captured the overall trend, but some inconsistencies were observed when compared to the actual load data. These discrepancies can be attributed to the inherent variability of Vehicle loads, which are significantly influenced by user behaviors in electric vehicle charging. Factors such as variations in charging times, the frequency of vehicle use, and differing charging speeds among users create a dynamic and unstable load profile. This results in occasional misalignments between the predicted and observed values, particularly during periods of peak demand. While the model was able to follow the broader trajectory of Vehicle load, the irregular nature of electric vehicle charging presents a unique challenge that may require additional model refinement to improve prediction accuracy.
When forecasting Heat Load, the TCN-Meta model demonstrated a high level of accuracy. Heat loads tend to be more stable and consistent, which allowed the model to closely track the actual load curves with minimal deviation. The relatively low variability in Heat loads, influenced largely by external factors such as weather and heating system cycles, provides a more predictable pattern that the model handled effectively. The near-perfect alignment of the predicted and actual Heat loads suggests that the TCN-Meta model is particularly well-suited for load types that exhibit lower volatility and are more stable over time.
In the case of PV Load, the TCN-Meta model successfully captured the primary periodic fluctuations associated with solar energy generation. While the overall prediction followed the periodic nature of PV loads, there were minor errors in terms of timing and amplitude, particularly at peak moments of energy generation. These errors can be attributed to the inherent variability of PV loads, which are subject to environmental factors like sunlight intensity and weather changes. Despite these challenges, the model performed well in recognizing the cyclical nature of PV loads and responded effectively to their variability, though further improvements could be made to enhance its precision during peak generation times.
The results from this study not only confirm the efficacy of the TCN-Meta model across various load types but also shed light on the specific challenges the model faces when predicting highly dynamic and unstable loads. This insight is valuable for guiding future optimizations of the model, especially in improving its capacity to handle unpredictable load patterns such as those from electric vehicle charging and solar power generation. Future enhancements to the model could involve integrating more contextual data, such as real-time user behavior or environmental conditions, to further improve forecasting accuracy in these complex scenarios.
The computational efficiency of the proposed TCN-Meta model is crucial for its applicability in real-time load forecasting scenarios, particularly within the constraints of smart grid operations. To assess the practicality of our model, we conducted a thorough analysis of the computation time on a robust hardware setup and optimized software environment.
The experiments were executed on a high-performance computing setup comprising an AMD Ryzen ThreadRipper 3970 × 3.8 GHz CPU, 128 GB DDR4 RAM, and an Nvidia GeForce RTX 3090 GPU. This configuration ensures that the computational demands of our model are adequately met. The model was implemented using PyTorch 3.7, leveraging the NVIDIA CUDA 10 environment to harness the GPU’s parallel computing capabilities, which is essential for handling the intensive matrix operations inherent in deep learning models.
The total training time for our model, including data preprocessing and model convergence, was measured to be approximately 14 min. This duration includes the preprocessing of 31,532 samples through the Fourier Feature transformation and the subsequent training of the TCN layers. The testing phase, which involved evaluating the model on 3404 samples, was completed in approximately 0.3 s. These timings underscore the model’s efficiency in both learning from historical data and making predictions on new data.
To further enhance the computational efficiency, our model employs a meta-learning framework that utilizes differentiable closed-form solutions for optimization. This approach significantly reduces the need for iterative optimization, thereby cutting down the computation time. Additionally, the use of GPU acceleration ensures that the model can leverage parallel processing for both training and inference, making it suitable for deployment in edge computing environments where rapid decision-making is required.
The TCN-Meta model demonstrates a commendable balance between computational efficiency and forecasting accuracy. Its training and testing times are within acceptable limits for practical applications, and its computational complexity is manageable given the current computational capabilities.
To evaluate the impact of different components on model performance, we conducted an ablation study to investigate the effects of removing Concatenated Fourier Features (CFF) and replacing the base model with various architectures, including GRU, LSTM, CNN, RNN, and Transformer. This study provides valuable insights into the contributions of CFF and the choice of base model to the overall performance of the meta-learning framework.
The first experiment in the ablation study investigates the effect of removing CFF from the TCN-Meta model. CFF was introduced as an innovative preprocessing step to capture periodic and frequency-domain features, which are often crucial for time series tasks involving seasonal or cyclical patterns. The results from Tables 4, 5 illustrate that the TCN-Meta/CFF model outperforms the TCN-Meta model (without CFF) across most evaluation metrics, suggesting that CFF contributes significantly to the model’s ability to capture complex temporal dependencies. In particular, the TCN-Meta/CFF model shows lower RMSE and MAE values in the Vehicle-Load and Heat-Load categories. As shown in Table 4, TCN-Meta/CFF achieves RMSE values of 0.06966 and 0.12944, respectively, compared to 0.04839 and 0.10081 for TCN-Meta. Similarly, in Table 5, the TCN-Meta/CFF model outperforms the version without CFF in terms of MAE for both Vehicle-Load and Heat-Load, with MAE values of 0.0387 and 0.0807, respectively, compared to 0.0386 and 0.0799 for TCN-Meta. These results demonstrate that the inclusion of CFF helps improve the model’s ability to capture frequency-based patterns, which are particularly important for tasks involving cyclical or periodic data.
While the performance improvement with CFF is evident, it is also important to recognize that the effectiveness of CFF may depend on the specific characteristics of the data. In some cases, removing CFF leads to slight improvements, particularly in Vehicle-Load, where the model benefits from a simpler architecture. Nevertheless, the overall results underscore the value of CFF in enhancing the model’s ability to learn from frequency-domain features, thus supporting its role as a key innovation in this study.
In addition to investigating the effect of CFF, the ablation study also compares the performance of different base models within the meta-learning framework. This comparison includes CNN, GRU, LSTM, RNN, and Transformer, all of which were tested with and without CFF. The results, presented in Tables 4, 5, reveal considerable variation in performance across these models. Among the base models, CNN-meta achieved the best performance in terms of RMSE and MAE across all load types, particularly in Vehicle-Load and PV-Load. However, TCN-Meta with CFF outperformed CNN-meta in Heat-Load and showed competitive performance in Vehicle-Load and PV-Load. This suggests that TCN, when enhanced with CFF, is more effective at modeling the complex temporal patterns inherent in time series data compared to other models like CNN.
Furthermore, GRU-meta and LSTM-meta demonstrated relatively weaker performance in all categories, particularly in PV-Load, where GRU-meta and LSTM-meta achieved higher RMSE values (0.32680 and 0.38952, respectively) and MAE values (0.2932 and 0.2315). These results emphasize the need for careful selection of base models that are capable of capturing the temporal dependencies critical to time series forecasting tasks. The TCN model, with or without CFF, demonstrated superior ability to handle such dependencies, particularly in more complex tasks like Heat-Load.
The results from this ablation study clearly demonstrate the effectiveness of CFF in enhancing the performance of the TCN-Meta model for time series forecasting. While removing CFF resulted in minor improvements in certain cases, the overall performance of the TCN-Meta/CFF model was consistently superior, particularly in Vehicle-Load and Heat-Load. These findings highlight the importance of CFF as a key feature engineering step, as it enables the model to better capture periodic and frequency-domain patterns, which are essential for accurate forecasting in many real-world applications.Moreover, the comparison of base models further reinforces the value of TCN as the most suitable architecture for this task, particularly when paired with CFF. TCN demonstrated the ability to effectively capture long-range temporal dependencies, making it a strong candidate for time series forecasting tasks. The superior performance of TCN-Meta with CFF supports the hypothesis that integrating frequency-domain features improves the model’s capability to handle complex and cyclical patterns in time series data.
The ablation study also highlights the limitations of other base models in handling long-range dependencies. CNN performed well in tasks dominated by short-range dependencies, such as Vehicle-Load and PV-Load, but struggled with tasks like Heat-Load, which require capturing extended temporal relationships. This limitation is due to CNN constrained receptive fields. In contrast, TCN with CFF demonstrated a clear advantage in modeling long-range dependencies and cyclical patterns, making it particularly effective for more complex tasks. While Transformer and RNN-based models, including GRU and LSTM, can capture temporal dependencies, they were less efficient than TCN, particularly in tasks with intricate patterns. Transformers, relying on attention mechanisms, and RNNs, despite their sequential nature, both struggled to capture long-term dependencies, as evidenced by their higher RMSE and MAE values, particularly in tasks like PV-Load.
Finally, the study emphasizes the benefits of meta-learning in improving model adaptability across different forecasting tasks. The TCN-Meta framework, leveraging prior knowledge from related tasks, can effectively generalize to new tasks, reducing the need for extensive retraining. This ability to adapt to diverse temporal patterns is essential for real-world forecasting applications. The integration of CFF enhances this process by enabling the model to better capture frequency-domain features, improving its performance not only on simpler tasks but also on more complex ones. The results suggest that the combination of TCN, CFF, and meta-learning offers a robust solution for handling the diverse and complex temporal dependencies in time series forecasting.
In this paper, a comprehensive study improving the performance of flexible load forecasting by leveraging feature extraction from a preliminary load disaggregation task is investigated. Initially, during the data pre-processing stage, CFF is employed to capture the multiscale periodicity features within the power consumption data. Additionally, the TCN base learner is utilized with stacked causal convolutional layers for perception field extending to extract high-dimensional periodicity features. Finally, the meta-learning framework is built with differentiable closed-form solutions to optimize and update model parameters from load disaggregation task to flexible load forecasting task through a two-tiered learning process. After experiment on the public residential power consumption dataset, the proposed method outperforms state-of-the-art algorithms, proving its robustness and effectiveness across various flexible load types. Future work will explore the integration of more contextual factors, such as real-time user behavior or environmental conditions, to further improve load forecasting accuracy in complex scenarios.
The original contributions presented in the study are included in the article/supplementary material, further inquiries can be directed to the corresponding authors.
YZ: Writing–original draft. QS: Data curation, Writing–review and editing. FD: Data curation, Writing–review and editing. FL: Validation, Writing–review and editing. SJ: Formal Analysis, Writing–review and editing. WW: Methodology, Supervision, Writing–review and editing.
The author(s) declare that financial support was received for the research and/or publication of this article. This work was supported by the Science and Technology Project of State Grid Electric Power Co., Ltd. (J2023175). The funder was not involved in the study design, collection, analysis, interpretation of data, the writing of this article, or the decision to submit it for publication.
Authors YZ, QS, FD, FL, SJ, and WW were employed by State Grid Jiangsu Electric Power Co., Ltd., Yancheng Power Supply Company.
The author(s) declare that no Generative AI was used in the creation of this manuscript.
All claims expressed in this article are solely those of the authors and do not necessarily represent those of their affiliated organizations, or those of the publisher, the editors and the reviewers. Any product that may be evaluated in this article, or claim that may be made by its manufacturer, is not guaranteed or endorsed by the publisher.
Ahajjam, M. A., Licea, D. B., Ghogho, M., and Kobbane, A. (2022). Experimental investigation of variational mode decomposition and deep learning for short-term multi-horizon residential electric load forecasting. Appl. Energy 326 (November), 119963. doi:10.1016/j.apenergy.2022.119963
Bonfigli, R., Principi, E., Fagiani, M., Severini, M., Squartini, S., and Piazza, F. (2017). Non-intrusive load monitoring by using active and reactive power in additive Factorial Hidden Markov Models. Appl. Energy 208, 1590–1607. doi:10.1016/j.apenergy.2017.08.203
Chang, H. H., Lian, K. L., Su, Y. C., and Lee, W. J. (2013). Power-spectrum-based wavelet transform for nonintrusive demand monitoring and load identification. IEEE Trans. Industry Appl. 50 (3), 2081–2089. doi:10.1109/tia.2013.2283318
Du, Z., Yin, B., Zhu, Y., Huang, X., and Xu, J. (2023). A NILM load identification method based on structured VI mapping. Sci. Rep. 13 (1), 21276. doi:10.1038/s41598-023-48736-8
Faustine, A., and Pereira, L. (2022). FPSeq2Q: fully parameterized sequence to quantile regression for net-load forecasting with uncertainty estimates. IEEE Trans. Smart Grid 13 (3), 2440–2451. doi:10.1109/TSG.2022.3148699
Fekri, M. N., Patel, H., Grolinger, K., and Sharma, V. (2021). Deep learning for load forecasting with smart meter data: online adaptive recurrent neural network. Appl. Energy 282 (January), 116177. doi:10.1016/j.apenergy.2020.116177
Figueiredo, M., Ribeiro, B., and de Almeida, A. (2013). Electrical signal source separation via nonnegative tensor factorization using on site measurements in a smart home. IEEE Trans. Instrum. Meas. 63 (2), 364–373. doi:10.1109/tim.2013.2278596
Gao, X., Li, X., Zhao, B., Ji, W., Jing, X., and He, Y. (2019). Short-term electricity load forecasting model based on EMD-GRU with feature selection. Energies 12 (6), 1140. doi:10.3390/en12061140
Heo, S., and Kim, H. (2021). Toward load identification based on the Hilbert transform and sequence to sequence long short-term memory. IEEE Trans. Smart Grid 12 (4), 3252–3264. doi:10.1109/tsg.2021.3066570
Heureux, L., Grolinger, K., and Capretz, M. A. M. (2022). Transformer-based model for electrical load forecasting. Energies 15 (14), 4993. doi:10.3390/en15144993
Imani, M. (2021). Electrical load-temperature CNN for residential load forecasting. Energy 227. doi:10.1016/j.energy.2021.120480
Jia, Z., Yang, L., Zhang, Z., Liu, H., and Kong, F. (2021). Sequence to point learning based on bidirectional dilated residual network for non-intrusive load monitoring. Int. J. Electr. Power and Energy Syst. 129, 106837. doi:10.1016/j.ijepes.2021.106837
Kalakova, A., Kumar Nunna, H. S. V. S., Jamwal, P. K., and Doolla, S. (2021). A novel genetic algorithm based dynamic economic dispatch with short-term load forecasting. IEEE Trans. Industry Appl. 57 (3), 2972–2982. doi:10.1109/TIA.2021.3065895
Kang, H., and Kim, H. (2020). Household appliance classification using lower odd-numbered harmonics and the bagging decision tree. IEEE Access 8, 55937–55952. doi:10.1109/access.2020.2981969
Kong, W., Dong, Z. Y., Jia, Y., Hill, D. J., Xu, Y., and Zhang, Y. (2019). Short-term residential load forecasting based on LSTM recurrent neural network. IEEE Trans. Smart Grid 10 (1), 841–851. doi:10.1109/TSG.2017.2753802
Langevin, A., Mohamed, C., and Gagnon, G. (2023). Efficient deep generative model for short-term household load forecasting using non-intrusive load monitoring. Sustain. Energy, Grids Netw. 34, 101006. doi:10.1016/j.segan.2023.101006
Lin, X., Tian, Z., Lu, Y., Zhang, H., and Niu, J. (2019). Short-term forecast model of cooling load using load component disaggregation. Appl. Therm. Eng. 157. doi:10.1016/j.applthermaleng.2019.04.040
Liu, M., Qin, H., Cao, R., and Deng, S. (2022). Short-term load forecasting based on improved TCN and DenseNet. IEEE Access 10, 115945–115957. doi:10.1109/ACCESS.2022.3218374
Liu, Y., Liu, W., Shen, Y., Zhao, X., and Gao, S. (2021). Toward smart energy user: real time non-intrusive load monitoring with simultaneous switching operations. Appl. Energy 287. doi:10.1016/j.apenergy.2021.116616
Liu, Y., Wang, X., and You, W. (2018). Non-intrusive load monitoring by voltage–current trajectory enabled transfer learning. IEEE Trans. Smart Grid 10 (5), 5609–5619. doi:10.1109/tsg.2018.2888581
Luan, W., Zhang, R., Liu, B., Zhao, B., and Yu, Y. (2023). Leveraging sequence-to-sequence learning for online non-intrusive load monitoring in edge device. Int. J. Electr. Power and Energy Syst. 148. doi:10.1016/j.ijepes.2022.108910
Mughees, N., Ali Mohsin, S., Mughees, A., and Mughees, A. (2021). Deep sequence to sequence Bi-LSTM neural networks for day-ahead peak load forecasting. Expert Syst. Appl. 175 (August), 114844. doi:10.1016/j.eswa.2021.114844
Parson, O., Fisher, G., Hersey, A., Batra, N., Kelly, J., Singh, A., et al. (2015). “Dataport and NILMTK: a building data set designed for non-intrusive load monitoring,” in 2015 ieee global conference on signal and information processing (globalsip) (IEEE), 210–214.
Peng, Q., and Liu, Z.-W. (2020). “Short-term residential load forecasting based on smart meter data using temporal convolutional networks,” in 2020 39th Chinese control conference (CCC), 5423–5428. doi:10.23919/CCC50068.2020.9188453
Pirbazari, A. M., Farmanbar, M., Chakravorty, A., and Rong, C. (2020). “Improving load forecast accuracy of households using load disaggregation techniques,” in 2020 international conferences on internet of things (iThings) and IEEE green computing and communications (GreenCom) and IEEE cyber, physical and social computing (CPSCom) and IEEE smart data (SmartData) and IEEE congress on cybermatics (cybermatics) (IEEE), 843–851.
Raghu, A., Raghu, M., Bengio, S., and Vinyals, O. (2020). Rapid learning or feature reuse? Towards understanding the effectiveness of MAML. arXiv. Available online at: http://arxiv.org/abs/1909.09157.
Shi, H., Xu, M., and Ran, Li (2018). Deep learning for household load forecasting—a novel pooling deep RNN. IEEE Trans. Smart Grid 9 (5), 5271–5280. doi:10.1109/TSG.2017.2686012
Wang, H., Ma, J., and Zhu, J. (2023). Identifying household EV models via weighted power recurrence graphs. Electr. Power Syst. Res. 217, 109121. doi:10.1016/j.epsr.2023.109121
Wang, J., Zhu, H., Cheng, F., Zhou, C., Zhang, Y., Xu, H., et al. (2023b). A novel wind power prediction model improved with feature enhancement and autoregressive error compensation. J. Clean. Prod. 420, 138386. doi:10.1016/j.jclepro.2023.138386
Wang, J., Zhu, H., Zhang, Y., Cheng, F., and Zhou, C. (2023a). A novel prediction model for wind power based on improved long short-term memory neural network. Energy 265, 126283. doi:10.1016/j.energy.2022.126283
Welikala, S., Dinesh, C., Ekanayake, M. P. B., Godaliyadda, R. I., and Ekanayake, J. (2017). Incorporating appliance usage patterns for non-intrusive load monitoring and load forecasting. IEEE Trans. Smart Grid 10 (1), 448–461. doi:10.1109/tsg.2017.2743760
Wu, X., Han, L., Wang, Z., and Qi, B. (2017). A nonintrusive fast residential load identification algorithm based on frequency-domain template filtering. IEEJ Trans. Electr. Electron. Eng. 12, S125–S133. doi:10.1002/tee.22425
Keywords: meta-learning, non-intrusive load monitoring, load forecasting, temporal convolutional network, concatenated Fourier Features
Citation: Zhang Y, Shu Q, Ding F, Liu F, Jiang S and Wu W (2025) Incorporated flexible load forecasting based on non-intrusive load monitoring: a TCN-based meta learning approach. Front. Energy Res. 13:1519053. doi: 10.3389/fenrg.2025.1519053
Received: 29 October 2024; Accepted: 24 February 2025;
Published: 27 March 2025.
Edited by:
Shuqing Zhang, Tsinghua University, ChinaReviewed by:
Ziming Yan, Nanyang Technological University, SingaporeCopyright © 2025 Zhang, Shu, Ding, Liu, Jiang and Wu. This is an open-access article distributed under the terms of the Creative Commons Attribution License (CC BY). The use, distribution or reproduction in other forums is permitted, provided the original author(s) and the copyright owner(s) are credited and that the original publication in this journal is cited, in accordance with accepted academic practice. No use, distribution or reproduction is permitted which does not comply with these terms.
*Correspondence: Yun Zhang, YTE4MzEwMDI4NDcwQGdtYWlsLmNvbQ==
Disclaimer: All claims expressed in this article are solely those of the authors and do not necessarily represent those of their affiliated organizations, or those of the publisher, the editors and the reviewers. Any product that may be evaluated in this article or claim that may be made by its manufacturer is not guaranteed or endorsed by the publisher.
Research integrity at Frontiers
Learn more about the work of our research integrity team to safeguard the quality of each article we publish.