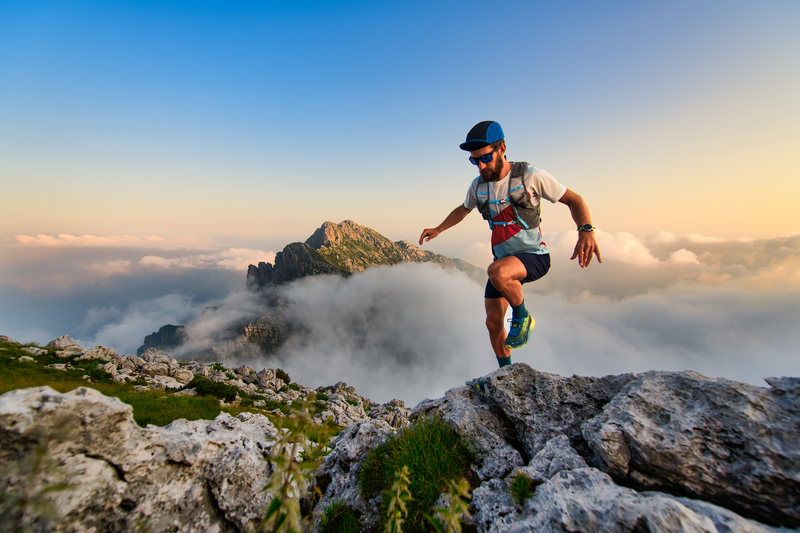
94% of researchers rate our articles as excellent or good
Learn more about the work of our research integrity team to safeguard the quality of each article we publish.
Find out more
ORIGINAL RESEARCH article
Front. Energy Res. , 18 February 2025
Sec. Sustainable Energy Systems
Volume 13 - 2025 | https://doi.org/10.3389/fenrg.2025.1454448
This article is part of the Research Topic Integrated Energy System Planning, Optimization, Trading and Benefit Assessment View all 11 articles
This article presents a novel particle swarm optimization (PSO)-based energy management system (EMS) designed for microgrids (MGs) to enhance operational efficiency, reduce dependency on the utility grid, and minimize electric vehicle (EV) charging costs. The proposed EMS integrates distributed energy sources, including photovoltaics (PVs), wind turbines (WTs), and battery energy storage systems (BESSs), alongside several EVs with variable loads to evaluate energy optimization. A comprehensive MG model is developed to assess multiple operational scenarios, including variations in EV demand, load, renewable energy production, and dynamic grid power prices. The study demonstrates the effectiveness of the PSO-based EMS in optimizing energy exchanges with the grid, leading to cost reductions of 14% and 21% with and without EV integration, respectively. Furthermore, the EMS facilitates efficient BESS charging/discharging schedules and implements proactive EV charging strategies to ensure uninterrupted electricity supply during peak and off-peak hours. These findings underscore the potential of PSO-based EMSs to provide a sustainable and cost-effective energy management solution in MGs by leveraging renewable resources and addressing the challenges posed by fluctuating energy demand and grid prices. Hence, the proposed model provides an optimized EMS and a proactive EV charging system.
The increasing number of smart grids has raised numerous obstacles in the preservation of power quality and consistency. Grid-connected energy management systems (EMSs) have experienced significant growth in recent years due to increasing energy usage. The EMS developed with a microgrid (MG) allows optimal scheduling of renewable energy sources (RESs), power storage, and charging for electric vehicles (EVs) to effectively manage the equilibrium between supply and demand while ensuring cost-effectiveness. There is a growing concern among urban populations regarding the escalating levels of atmospheric greenhouse gases (GHG) resulting from the rapid growth of urbanization and rising numbers of on-road vehicles. Developing a viable and enduring substitute for the transportation infrastructure is crucial to address this issue. Incorporating RESs into MGs presents a viable approach to addressing environmental concerns and providing a cost-effective EMS (Yong et al., 2015). MGs can mitigate the high costs of distributed RESs in their community by generating power during peak demand times and periods of excess supply on the grid. However, because these RESs are intermittent and unpredictable, integrating them into the grid has been problematic and challenging (Nienhueser and Qiu, 2016). Larger-scale RES integration needs the optimal combination of system management, operation, and enough energy flow. This study employed willingness-to-pay models to charge EVs with RESs. Recently, numerous cost-effective and ecologically sustainable EMSs for EVs have been successfully adopted. For instance, in a study by Qi et al. (2018) for the green energy local area network (ELAN), the collaborative energy management optimization (CEMO) technique was used. They considered economic, environmental, and safety issues, including a pollution treatment fee. Furthermore, a clever method for charging and unplugging plug-in hybrid electric cars (PHEVs) was suggested.
Two experimental modes have been developed to evaluate the model’s efficacy, and the simulation outcomes indicate that the energy management optimization model effectively distributes energy resources. Additionally, the innovative charging and discharging technique of the PHEV enhances economic benefits within the network. Based on the findings, it can be stated that recommended nonlinear energy management models can be used for an ELAN. Mehrasa et al. (2021) suggested a method for power management in an intelligent grid based on fuzzy logic. They put into place a system that considered both the cost of electricity and the plug-in electric vehicles’ (PEVs’) momentary state of charge (SOC). They projected actual photovoltaic (PV) generating data as the primary contributing variables to the grid system. As the expected outcome, the main goal of their study was to optimize PEV power output. To optimize power distribution among PEVs, distinct output regulations are allocated according to the rated power of each car. Furthermore, an additional heuristic approach was proposed to determine an SOC for PEVs upon their departure from the place of charging. The study indicated that intelligent charging and discharging methods for EVs at charging stations can optimize power generation, reduce expenses, and enhance overall system efficacy.
In another study, Sudhakar and Kumar (2022) propose reducing MG dependency and operating costs by coordinating RES and EV storage. The system works by forecasting RES generation and EV demand and then scheduling EV battery charging and discharging to ensure that the MG can operate independently from the grid as much as possible. Four decision variables (SOC, laxity, DOD, and transition probability) are used in an adaptive neuro-fuzzy inference system (ANFIS); the author used the prioritization-based EV technique. The authors show their method can significantly reduce grid dependency and operating costs while improving the reliability of MGs.
Misra et al. (2020) proposed a new battery management plan that engages an original concept of a distribution of dispersed generation based on the use of EVs to improve the intelligent chargers known as V2G. The article fills the gap concerning the key issues connected to energy management inside new charging systems, underlining the positive role of an EV as a distributed energy storage facility. Belkhier and Oubelaid (2024) proposed a fuzzy logic-based EMS for hybrid MG’s integrating tidal, WT, PV, and fuel cell systems with BESS. They claim that the efficiency and cost of their power systems were improved through their simulations to help prove the ability of the net zero energy systems. Guddanti and Illindala (2019) applied an economic optimization model with a real-time price structure for a DC MG EMS. The model was designed with the overall goal of making the operation cost of the MG as low as possible. This included optimizing how the power could be handed off between DGs, BESSs, and the utility efficiently. The model was implemented using two different algorithms. The proposed model includes two optimization algorithms: a genetic algorithm (GA) and a pattern search algorithm (PS). The results indicated that the proposed GA-based method optimized and surpassed the PS-based method by reducing the cost and time spent on computation. The GA-based method achieves cost savings of up to 20% compared to the conventional EMS. The proposed model can be used to develop a real-time EMS for DC MGs to operate economically and efficiently.
Another study by Fouladi et al. (2019) work focuses on CO2 reduction in MGs, integrating EVs and RESs. They use an improved whale optimization algorithm to optimize scheduling and resource utilization. This strategy aligns with the growing need for energy system decarbonization measures, with MG offering localized solutions. The study provides valuable insights for creating MG management plans to prioritize environmental sustainability and energy efficiency, building upon previous research on MG optimization and EV integration.
Ahmad et al. (2019) proposed an ideal foundation for a community MG-integrated EMS for EV charging stations for the public. The foundation of the suggested structure is a switching mechanism that enables the EMS to select the energy source that will charge EVs. The most affordable framework is designed to fulfill community load demand, minimize the cost of energy to charge EVs, and maximize the selling cost of excess energy from PV systems and EVs. It is formulated as a mixed-integer, nonlinear issue under numerous trading locations. The article presents a case study of a 100-house community in India and demonstrates that the proposed EMS can significantly reduce energy costs and improve grid stability. A study by Ahmad et al. (2019) proposed reducing the energy cost of the station by optimizing the battery-swapping process and utilizing RESs. The system’s performance was evaluated on a small-scale battery-swapping station, and the results showed reduced energy costs and GHG emissions.
A novel hybrid GA/MOPSO EMS for grid-connected MGs is presented in another study by Tabrizi (2022). With PV energy, WTs, microturbines, diesel generators, and a BESS, the system tries to satisfy operational needs while lowering operating costs.
Zadeh et al. (2023) proposed an energy scheduling model for intelligent buildings with EVs. Demand response (DR) programs optimize energy costs by considering the building’s energy consumption profile, EV charging and discharging requirements, and DR incentives. Demand-side management (DSM) and techno-economic indices are used to minimize total energy costs. The model’s performance in a real-world intelligent building case study significantly reduced energy costs. Another approach by Ghasemi-Marzbali et al. (2023) integrates DR, BESSs, and RES into EV rapid charging stations. The fuzzy logic approach reduces the cost and environmental impact of EV charging, considering factors like WTs, BESSs, PV capacity, and RES. The hybrid algorithm predicts wind speed variations. Attou et al. (2021) suggested a unified EMS for a hybrid energy storage system (HESS) and connected MG. The primary aim was to mitigate fluctuations in demand as well as production patterns while simultaneously improving the use of RESs and managing the transfer of energy with the utility grid. The application of a rule-based EMS (RB EMS) was proposed by the author as a means to implement long-term EMSs, including the day-ahead schedule of distributed energy resources (DERs), in a household multi-source grid-connected MG. The distributed controller simultaneously supervises the management of short-term electricity. The EMS enables the efficient control of the MG’s operational requirements by predicting and scheduling DERs for service to meet the needed load in conformity with several conditions. Based on the implementation and assessment of the proposed EMS in a simulated hybrid MG, the outcomes reveal that this system is beneficial in the minimization of operation costs and improvement of the MG’s resilience.
Rule-based systems, while offering straightforward solutions using discrete algorithms, often struggle to accommodate the dynamic and non-linear complexities of modern EMS. Their inherent rigidity limits adaptability to evolving user preferences, system constraints, and environmental variations, frequently resulting in sub-optimal solutions. This limitation is particularly pronounced in scenarios involving multi-objective optimization and highly variable energy demands, where a broader exploration of possibilities is essential. To overcome these challenges, advanced optimization techniques like PSO offer significant advantages. PSO is adept at addressing complex constraints, processing real-time data, and achieving multiple objectives, making it a powerful tool for optimizing EMS performance in MG’s. The primary objective of this study is to integrate PSO into an EMS framework to enhance the cost-effectiveness and operational efficiency of managing EV energy within MGs. PSO’s robust optimization capabilities enable effective scheduling for battery charging and discharging by prioritizing RESs and dynamically responding to electricity price fluctuations. For instance, the EMS ensures battery charging during low-cost periods and discharging during high-cost periods, thereby reducing overall energy expenses. By leveraging PSO, this research offers a comprehensive and adaptable EMS solution to address the dynamic challenges of modern energy management while promoting the sustainable integration of EVs in MGs.
The suggested power management technique is formulated and provided in this section. Reducing dependency on the MG and offer reasonably priced EV charging entails gradually increasing the PHEVs’ charging power for a day. The primary aim of the recommended optimization approach is to obtain the most economical configuration for the MG system while meeting the system’s overall requirements. Energy optimization management aims to decrease overall expenses, including pollution and power generation costs while satisfying network load needs. Equation 1 shows a mathematical expression of an objective function.
where PP v(t) is the PV output, PW ind(t) is the wind output, PBESS(t) is the battery power, PGrid (t, c) is the grid generation, PH. Load(t) is the residential load, PEV. Load(t) is the EV load, and the time t is in hours (0–24).
The PV array’s output power is directly correlated with the sun irradiation, and the generated power is given by Equation 2.
To evaluate the generated PV power, see Figure 1, which is contingent upon solar radiation at time G(t). Ppv(t) is the produced power of PV arrays (W) at each hour, ηpv is the PV module efficiency, Pmpv is the rated power of each PV (W), Npv is the optimum size of PV, and G0 is 1,000(W/m2) Tooryan et al. (2022). This dataset is from the city of Burlington, USA. Latitude is 44.47, and longitude is −73.21 Stackhouse (2023).
The generated output power of the WT by Equation 3 is shown in Figure 2 and is related to wind speed. This dataset is from the city of Burlington, USA; latitude is 44.47 and longitude is −73.21 Stackhouse (2023).
where Pw,ra and Pw,co are the WT’s output power levels at its rated and cut-out speeds, represented by kW, respectively, Vw is the measured wind speed in m/s, Vra is the rated wind speed in m/s, Vco is the cut-out wind speed in m/s, and Vci is the cut-in wind speed in m/s (Fouladi et al., 2020).
In this work, the stochastic load profile of PHEV and the intermittent output power of RESs are reduced using a lead-acid BESS. Several conditions must be fulfilled to charge and discharge the BESS. These conditions are described in Equations 4, 5.
where SOCmax and SOCmin are the BESS’s maximum and minimum SOC boundaries, respectively. To increase the lifespan of the BESS and prevent damage, the SOC of the BESS needs to fulfill the above requirements (Fouladi et al., 2020). Equation 6 represents the difference between the BESS’s charging and discharging powers, which can be referred to as its output power, and Equation 7 provided that it stays within its permitted bounds.
The utility grid, WTs, PVs, BESSs, and other DERs are all included in the proposed MG system. Obtaining the cost-optimal MG system while meeting the overall system demand is the main objective of the suggested optimization process. The MG’s electricity demand is simultaneously met every hour during a specific timeframe. The input data include a range of technical and economic factors and changeable hourly load profiles for various needs over a year, such as electricity demand, solar radiation, and wind speed. The PSO method, which is a meta-heuristic optimization approach to identify the time for EV charging, is used. According to the suggested energy management strategy for meeting electrical demand, the resulting optimal outcomes can thus provide the system’s whole demands for all hours within a given time. The EMS receives power from PVs, WTs, BESSs, and utility grids. The EMS gives power to the community and EV loads, as shown in Figure 3. The EMS depends on load demand and cost according to time. The aim is to address the optimization of a cost-efficient EMS for EVs in a residential setting.
The goal is to balance the use of locally generated renewable energy, grid energy, and BESSs to minimize costs and maximize the utilization of sustainable resources. PSO will be explored as a potential optimization technique to achieve this goal. The primary energy sources used to power the loads are PVs and WTs. When the price of electricity is low, the grid or excess renewable energy power charges the BESS (when the output is higher than the loads). When there is insufficient power from the PV system and WTs, and the cost of grid electricity is high, the BESSs release energy. EVs are charged by excess PV and WT energy when the price of grid power is low. During a surge in demand or a variation in renewable output, EVs give power to the grid (V2G). In that case, if the PV, WT, and BESS systems are insufficient, the grid will supply the load. If output outpaces demand and the BESS is fully charged, surplus renewable energy will be sent straight into the grid.
To ensure the efficient use of RESs, the BESS strategic approach was used, as shown in Figure 4. According to the proposed BESS strategy, if the generation of renewable energy is greater than the demand, then the system will check the battery SOC. In the case that the battery SOC is less than 100%, the battery will be charged; otherwise, the remaining power will be sold to the grid at minimum cost. On the other hand, if the generation of renewable energy is less than the demand, then the algorithm will check the utility grid price according to time. If the grid price t is less than the price t+1, the system will charge the battery until SOC is 100%. If the electricity price t is greater than t+1, then the system will check the battery SOC, and if it is greater than 50%, the battery will discharge until SOC is 20%. On the other hand, if the battery SOC is less than 50%, then the system will buy the power from the grid, and the battery will be charged.
The PSO can be utilized to dynamically optimize the charging schedules of electric vehicles by iteratively adjusting the charging rates based on the community’s energy consumption patterns. This feature of PSO helps utilize the available renewable energy from PV and WT sources while considering the charging requirements of the EVs.
This study presents the PSO technique for the best scheduling mode for EV car charging, aiming to produce a more optimized EMS. The suggested MG EMS, which integrates RESs, including PVs, WTs, BESSs, EVs, and the main grid, is evaluated using a MATLAB algorithm simulation.
Several scenarios are implemented to see how the EMS behaves in power-sharing across various sources in all operational modes (electricity pricing, BESS SOC, residential load, and electric car charging time frames). For this simulation, we took 24 h to test the different scenarios. This operation is divided into three modes.
Mode 1: PV, wind, and grid.
Mode 2: PV, wind, BESS, and Grid.
Mode 3: Proactive EV charging.
In all modes, the SOCmin and SOCmax are 20% and 80%, respectively. Depending on the amount of solar irradiation, the PV system operates in all modes with a regular distribution over the day, peaking in power at noon.
Throughout the day, there are peaks and troughs in the WT generation due to the wind speed, which varies randomly. The daily load curve displays a 23 kW maximum power output for the residential and EV loads. The load profile fluctuates throughout the day and weather, with strong demand periods in the morning and evening and low demand throughout the night.
When the MG runs in Mode 1 (without a BESS), WTs and the main grid supply load in the morning when energy prices are low. Then, the PV system produces energy to satisfy the residential load demand. At the same time, the power produced by the grid decreases, as shown in Figure 5. The grid works with the WTs to supply electricity as PV production decreases. The energy demand is low at midnight and evening, and the WTs provide the load at this time. All the energy needed to meet the electricity demand is obtained from the grid, resulting in extremely high purchasing costs.
Figure 6 shows that when the EV load is added to the residential load, the amount of power imported from the grid increases. When operating in this mode, the system becomes less affordable outside of periods of renewable production when all demand is met by purchasing electricity from the grid due to the lack of storage mechanisms (stationary BESSs).
In Mode 2, PVs, WTs, BESSs, and the utility grid were used. Initially, the analysis was performed without implementing optimization techniques, as shown in Figure 7. The total amounts of power received and returned are 88.01 kW and 6.2 kW, respectively. The per-day cost was also calculated (6.69 $ without optimization) as presented in Table 1.
Figure 8 shows that with optimization to take advantage of the cost-effective energy pricing, the grid and WTs work together to meet the demand and enable the charging of batteries before sunrise. This method aims to ensure that the SOC remains between 20% and 80%, with a minimum limit of SOCmin at 20%.
In Equation 8 when more electricity is produced than consumed, PV and WT systems are used to meet the demand, giving preference to using excess power from renewable sources. On the other hand, in the optimized case, surplus energy is used to raise the BESS SOC to its SOCmax limit at a reasonable cost when the BESS is not fully charged. The remaining surplus energy will be used as a second priority, which is power return to the grid. Figure 8 shows the optimized power received and returned from the grid as 75.1 kW and 8.1 kW, respectively. The per-day cost for this case can be noted as 5.25 $. It can be observed that optimization results in a 21% reduction in cost.
In Equation 9 when less electricity is produced than consumed, and EV is available without optimization, the total received power from the grid is 125.3 kW, the total returned power to the grid is 4.67 kW, and the day cost is 9.82 $, as shown in Figure 9 and Table 1, respectively.
Figure 9. Residential, EV and renewable, BESS, and grid import and return power without optimization.
If an EV is available after charging the BESS, the remaining power is initially used to charge the EV battery, and any excess power will be returned to the grid. The BESS begins to discharge to make up the power deficit when the demand exceeds consumption. The BESS and the WTs feed the load to its ultimate SOCmin limit. Then, importing the power from the grid fulfills the load demand requirements, shown in Figure 6, resulting in a significant financial benefit.
In Equation 10 through dynamic adjustments to BESS pricing in response to hourly electricity rates, the system makes the best use of renewable energy during low-cost periods. Figure 10 shows the optimized total power received and returned from the grid as 112.4 kW, 6.51 kW, and the 1-day cost as 8.38 $. It can be seen that the optimization reduces the total cost by 14%, as shown in Table 1. The system stays off the grid during periods of high cost, as shown in Figure 11. This strategy is to improve the operating conditions, together with a concurrent decrease in the cost of fuel, grid energy purchases, and CO2 emissions. Figure 12 describes the optimized time for EV charging based on cost and time. The provided method shows how EV car charging may be optimized by carefully timing charging sessions to coincide with times when power is most affordable. In addition to guaranteeing economical energy use, this enhances the MG system’s overall sustainability. Incorporating a BESS is essential for reducing excessive power bills, improving energy use flexibility, bills, improving energy use flexibility, and encouraging eco-friendly EV charging habits. In Mode 3, a proactive EV charging system was used, which is an innovative technique for managing electric car charging that optimizes energy use, lowers costs, and has a low effect on the power grid, unlike standard charging systems, which allow EV owners to plug-in their vehicles and start charging instantly from the grid (EV CharFG). Figure 13 shows the EV without considering the proactive algorithm, while Figure 14 presents EV charging with implementing proactive algorithms. Proactive charging systems employ powerful algorithms and real-time data to intelligently manage and regulate the charging process. It can be seen in Figure 14 that the system checks the renewable energy and BESS charging if any is available and then checks the EV charging by RESs (EV CharFRew). When renewables and BESSs are unavailable, the system checks the electricity price, tries to charge the EV at the lowest cost, and optimizes the EV charging hours.
This study proposed an EMS in a grid connected to an MG that consists of EVs with variable loads, a PV system, a wind farm, and a BESS. The PSO optimization technique has been developed to determine the best configuration of energy sources to minimize operating costs and grid dependence while maximizing renewable energy and electricity exchange. The simulation’s findings showed the system’s ability to generate electricity during varying times, following the power system’s demands, and offer a more adaptable and effective service. Additionally, the energy exchange mechanism between the load and various sources illustrates the effectiveness of smart grids in maximizing energy exchanges with the main grid, optimizing the use of renewable resources, and improving energy management and consumption prices. The suggested scheduling technique lowers the energy cost of EVs. The scheduling problem is using PSO with certain modifications based on the condition that batteries will be charged if electricity rates are low and discharged when prices rise. Moreover, the results showed that the implementation of PSO-based optimization resulted in a cost reduction of 14% and 21% in cases with and without EV consideration, respectively. EV charging also uses a proactive technique and saves cost and power. The simulation results validate the approach and method.
The original contributions presented in the study are included in the article/Supplementary Material, further inquiries can be directed to the corresponding author.
AK: conceptualization, data curation, formal analysis, funding acquisition, investigation, methodology, project administration, resources, software, supervision, validation, visualization, writing–original draft, and writing–review and editing. AH: conceptualization, data curation, formal analysis, investigation, methodology, project administration, resources, supervision, validation, writing–original draft, and writing–review and editing. MB: conceptualization, data curation, formal analysis, funding acquisition, investigation, methodology, project administration, resources, software, supervision, validation, visualization, writing–original draft, and writing–review and editing.
The author(s) declare that financial support was received for the research, authorship, and/or publication of this article. This work was supported in part by the Collaborative Research Project (CRP), Nazarbayev University, under Grant 211123CRP1604, and in part by the Faculty Development Competitive Research Grant (FDCRG), Nazarbayev University, under Grant 201223FD8811.
Author AH was employed by Revterra Company.
The remaining authors declare that the research was conducted in the absence of any commercial or financial relationships that could be construed as a potential conflict of interest.
The author(s) declared that they were an editorial board member of Frontiers, at the time of submission. This had no impact on the peer review process and the final decision.
All claims expressed in this article are solely those of the authors and do not necessarily represent those of their affiliated organizations, or those of the publisher, the editors and the reviewers. Any product that may be evaluated in this article, or claim that may be made by its manufacturer, is not guaranteed or endorsed by the publisher.
Ahmad, F., Alam, M. S., Shariff, S. M., and Krishnamurthy, M. (2019). A cost-efficient approach to ev charging station integrated community microgrid: a case study of indian power market. IEEE Trans. Transp. Electrification 5, 200–214. doi:10.1109/tte.2019.2893766
Attou, N., Zidi, S.-A., Khatir, M., and Hadjeri, S. (2021). Energy management system for hybrid microgrids. Electroteh. Electron. Autom. 69, 21–30. doi:10.46904/eea.21.69.2.1108003
Belkhier, Y., and Oubelaid, A. (2024). Novel design and adaptive coordinated energy management of hybrid fuel-cells/tidal/wind/pv array energy systems with battery storage for microgrids. Energy Storage 6. doi:10.1002/est2.556
Fouladi, E., Baghaee, H. R., Bagheri, M., and Gharehpetian, G. (2019). “A charging strategy for phevs based on maximum employment of renewable energy resources in microgrid,” in 2019 IEEE international conference on environment and electrical engineering and 2019 IEEE industrial and commercial power systems europe (EEEIC/I&CPS Europe), Genova, Italy, 11-14 June 2019, (IEEE), 1–5.
Fouladi, E., Baghaee, H. R., Bagheri, M., Lu, M., and Gharehpetian, G. (2020). “Bess sizing in an isolated microgrid including phevs and rers,” in 2020 IEEE International Conference on Environment and Electrical Engineering and 2020 IEEE Industrial and Commercial Power Systems Europe (EEEIC/I&CPS Europe) Madrid, Spain, 09-12 June 2020, (IEEE), 1–5.
Ghasemi-Marzbali, A., and Shafiei, M. (2023). Electric vehicle fast charging station design by considering probabilistic model of renewable energy source and demand response. Energy 267, 126545. doi:10.1016/j.energy.2022.126545
Guddanti, B., and Illindala, M. S. (2019). “Cost saving optimization model for energy management system of a dc microgrid under real time pricing,” in 2019 IEEE Industry Applications Society Annual Meeting, Baltimore, United States, 29 September 2019–03 October 2019, (IEEE), 1–8.
Mehrasa, M., Razi, R., Hajar, K., Labbone, A., Hably, A., and Bacha, S. (2021). “Power management of a smart vehicle-to-grid (v2g) system using fuzzy logic approach,” in IECON 2021–47th Annual Conference of the IEEE Industrial Electronics Society, Toronto, ON, Canada, 13-16 October 2021, (IEEE), 1–6.
Misra, S., Panigrahi, P. K., and Ghosh, S. (2020). “Smart battery management scheme for v2g based ev smart charger–a better approach of allocation of ev based distributed generation,” in 2020 IEEE International Symposium on Sustainable Energy, Signal Processing and Cyber Security (iSSSC), Gunupur Odisha, India, 16-17 December 2020, (IEEE), 1–6.
Nienhueser, I. A., and Qiu, Y. (2016). Economic and environmental impacts of providing renewable energy for electric vehicle charging–a choice experiment study. Appl. energy 180, 256–268. doi:10.1016/j.apenergy.2016.07.121
Qi, J., Lai, C., Xu, B., Sun, Y., and Leung, K.-S. (2018). Collaborative energy management optimization toward a green energy local area network. IEEE Trans. Industrial Inf. 14, 5410–5418. doi:10.1109/tii.2018.2796021
Stackhouse, P. (2023). NASA POWER | Data Access Viewer. NASA. Available at: https://power.larc.nasa.gov/data-access-viewer/.
Sudhakar, A., and Kumar, B. M. (2022). “Reducing grid dependency and operating cost of micro grids with effective coordination of res and ev storage,” in 2022 International Virtual Conference on Power Engineering Computing and Control: Developments in Electric Vehicles and Energy Sector for Sustainable Future (PECCON), Chennai, India, 05-06 May 2022, (IEEE), 1–6.
Tabrizi, A. R. (2022). “Energy management system optimization for grid connected microgrids in presence of energy storage,” in 2022 International Congress on Human-Computer Interaction, Optimization and Robotic Applications (HORA), Ankara, Turkey, 09-11 June 2022, (IEEE), 1–7.
Tooryan, F., HassanzadehFard, H., Dargahi, V., and Jin, S. (2022). A cost-effective approach for optimal energy management of a hybrid cchp microgrid with different hydrogen production considering load growth analysis. Int. J. Hydrogen Energy 47, 6569–6585. doi:10.1016/j.ijhydene.2021.12.036
Yong, J. Y., Ramachandaramurthy, V. K., Tan, K. M., and Mithulananthan, N. (2015). A review on the state-of-the-art technologies of electric vehicle, its impacts and prospects. Renew. Sustain. energy Rev. 49, 365–385. doi:10.1016/j.rser.2015.04.130
Zadeh, D. R., Derkhshan, G., Hakimi, S. M., and Abdi, B. (2023). Optimal energy scheduling in smart buildings with electric vehicle and demand response. Int. J. Integr. Eng. 15, 301–310. doi:10.30880/ijie.2023.15.04.026
Keywords: energy management system, electric vehicle charging, microgrid, particle swarm optimization, renewable energy
Citation: Khan AM, Hekmati A and Bagheri M (2025) Enhancing cost-effectiveness in residential microgrids: an optimization for energy management with proactive electric vehicle charging. Front. Energy Res. 13:1454448. doi: 10.3389/fenrg.2025.1454448
Received: 25 June 2024; Accepted: 15 January 2025;
Published: 18 February 2025.
Edited by:
Zhong Ge, Yunnan University, ChinaReviewed by:
Youcef Belkhier, Université de Bretagne Occidentale, FranceCopyright © 2025 Khan, Hekmati and Bagheri. This is an open-access article distributed under the terms of the Creative Commons Attribution License (CC BY). The use, distribution or reproduction in other forums is permitted, provided the original author(s) and the copyright owner(s) are credited and that the original publication in this journal is cited, in accordance with accepted academic practice. No use, distribution or reproduction is permitted which does not comply with these terms.
*Correspondence: Abdul Moeed Khan, YWJkdWxtb2VlZC5raGFuQG51LmVkdS5reg==
Disclaimer: All claims expressed in this article are solely those of the authors and do not necessarily represent those of their affiliated organizations, or those of the publisher, the editors and the reviewers. Any product that may be evaluated in this article or claim that may be made by its manufacturer is not guaranteed or endorsed by the publisher.
Research integrity at Frontiers
Learn more about the work of our research integrity team to safeguard the quality of each article we publish.