- 1State Grid Shandong Electric Power Research Institute, Jinan, China
- 2Shandong Smart Grid Technology Innovation Center, Jinan, China
With the growing integration of renewable energy into medium- and low-voltage distribution networks, the distribution substation area (DSA) has emerged, encompassing energy storage and loads. This paper introduces an energy interaction framework for multiple DSAs aimed at enhancing local renewable energy consumption. The energy interaction issue among various DSAs is modeled as a Nash bargaining problem to encourage energy exchanges. However, the variability in pricing and internal demand response may influence scheduling decisions, necessitating further investigation. To address price forecast errors, scenarios are developed using a stochastic programming approach to represent price uncertainties while adjusting the DSA’s load accordingly. Optimal power flow constraints are integrated into the model to bolster power system operation security. Additionally, the transmission capacity can impact scheduling outcomes and operational costs. The influence of transmission limitations on operational strategies is examined within the allowable capacity. To solve this issue, the bargaining model is divided into two subproblems, and an enhanced alternating direction multiplier method (ADMM) is used to maintain the privacy of DSAs. The simulation results obtained using the IEEE-33 bus system indicate that energy interaction among multiple DSAs significantly lowers operating costs and facilitates the integration of renewable energy.
1 Introduction
The integration of distributed renewable energy is a key challenge within distribution networks. To facilitate energy interaction, a distribution substation area (DSA), comprising a renewable power station, energy storage, and loads, can support local consumption and reduce disturbances in the network (Hirsch et al., 2018). Using energy storage, the DSA can adjust the load demand and better accommodate renewable generation. However, the inherent unpredictability of renewable sources may lead to energy shortages or surpluses. To optimize renewable energy efficiency, DSAs can interconnect with neighbors to facilitate energy exchanges (Kumar and Saravanan, 2017). Guided by the time-of-use (TOU) pricing set by the distribution network operator, energy interaction among multiple DSAs is encouraged, forming a small-scale interconnected DSA energy market (Vieira and Zhang, 2021). Given the shared interests, it is crucial to develop an interactive mechanism that incentivizes energy exchanges while maintaining economic viability and reliability within the region (Tushar et al., 2020).
A game theory-based mechanism is instrumental in studying and analyzing interactive strategies among multiple DSAs (Tushar et al., 2018). Generally, the game theory approach to interaction processes among participants can be categorized into non-cooperative and cooperative games (Tushar et al., 2019). In a non-cooperative game, buyers and sellers negotiate to establish interaction prices and quantities, achieving market clearing while maintaining the supply–demand balance (Paudel et al., 2019). A Stackelberg game-based negotiation process between buyers and sellers, which considers participant competition and achieves market clearing, is detailed by Jiang et al. (2022). Although Nash equilibrium solutions can be obtained in non-cooperative games, the decision-making processes are typically self-centered, and these solutions are not necessarily unique local optima (Chen et al., 2019).
To balance individual and collective interests, a cooperative game theory-based energy interaction model is proposed to achieve global optimization in energy sharing (Luo et al., 2022). The Nash bargaining game theory is well suited for energy interactions among multiple DSAs, ensuring equitable benefit allocation (Dehghanpour and Nehrir, 2017; Wang and Huang, 2016). Building on this cooperative model, optimal power flow constraints are incorporated into the system operation to enhance the model’s practicality (Li et al., 2018). However, these studies often overlook the impact of the demand response on energy interaction, which could potentially increase operational costs.
The demand response is an effective and promising approach that shifts electricity demand to periods when renewable generation is more abundant or the demand is lower. By leveraging load baselines, the demand response aids in the integration of renewable generation and reduces operational costs, thereby facilitating energy interaction (Sarker et al., 2020). A bi-level optimization model has been introduced for energy storage planning and operation, considering the electricity–heat demand response while utilizing Nash bargaining methods for benefit allocation (Alizadeh et al., 2024). However, these studies often distribute cooperative benefits equally among participants, which may lead to fairness concerns (Luo et al., 2022). To address this, a generalized Nash bargaining theory is adopted to incentivize energy interaction among multiple DSAs and allocate cooperative benefits based on the quantities of energy interaction (Kim et al., 2019).
The aforementioned studies formulate energy interaction models based on deterministic optimization, often overlooking forecast errors in TOU prices. Price uncertainty significantly impacts the economic and security aspects of an energy system. Generally, stochastic programming (Li et al., 2022) and robust optimization (Wei et al., 2021) are two prevalent methods used for addressing uncertainties. Considering the conservative nature of robust optimization, a stochastic optimization model is developed for unpredictable prices, aiming to achieve optimal scheduling (Baharvandi et al., 2019) and effective energy management (Chang et al., 2020). However, the influence of uncertain prices on energy interaction, as well as Nash bargaining-based operation decisions, is often neglected in these studies. Given the uncertain prices, DSAs schedule their demand to respond dynamically, which may alter their final decisions and operational costs. Considering the interdependent relationship between prices and demand response, the decisions of DSAs should integrate these influencing factors to devise optimal strategies.
Another aspect investigated in energy interaction is the consideration of physical constraints. Voltage fluctuations at each node (Jin et al., 2020) and power losses resulting from energy interaction (Khorasany et al., 2020) are modeled as costs paid to the operator. Although these factors are considered costs, further analysis is needed to understand strategy changes when transferring power to neighbors within the specified capacity limits. In other words, the congestion of transmission lines is directly addressed during the energy interaction process. Therefore, an energy interaction model is established that incorporates uncertain prices, demand response, and transmission capacity during energy interaction.
To sum up, the main contribution of this paper is to derive optimal operation strategies by considering the interdependent relationship between uncertain prices and the demand response of DSAs. Specifically, this paper investigates the effect of price uncertainty on the Nash bargaining theoretical model, analyzing both operation costs and the internal decision-making strategies of DSAs. To mitigate these adverse effects, the demand response combined with energy storage is proposed to enhance the flexibility of DSAs by shifting the load demand to periods of lower prices. The final operation strategies should account for the interconnected nature of price uncertainty and demand response. Additionally, optimal power flow is integrated into the optimization model to improve its practicality. Transmission limitations are also included to examine the impact of capacity restrictions on operation costs. Finally, the cooperative benefits are allocated based on the interaction of DSAs, ensuring a fair distribution that reflects the contribution of each participant to the energy interaction process.
2 Problem description
As illustrated in Figure 1, an energy interaction problem with
Given the price scenarios, DSAs negotiate with each other and respond to the prices by shifting load demands. To this end, the energy interaction problem is formulated as a generalized Nash bargaining game model to incentivize energy interaction and achieve fair benefit allocation. Additionally, a penalty for DSAs caused by the demand response is incorporated into the model to account for comfort levels. DSAs develop optimal operation strategies to maximize cooperative benefits and allocate these benefits based on their respective contributions. Congestion may occur during the process of energy transmission, especially considering the energy interaction among DSAs. To address this issue, we adjust the operation strategies of DSAs and analyze the impact on operation costs.
3 Energy interaction model
An independent operation model of a DSA and an energy interaction model among multiple DSAs are established for the comparative analysis of the operation costs of DSAs. Compared to independent operations, DSAs achieve cost savings through energy interaction with neighbors, which includes energy sharing, renewable generation integration, energy storage scheduling, and load shifting. Then, based on the generalized Nash bargaining theory, cooperative benefits are allocated by leveraging the bargaining power parameters.
3.1 Basic operation optimization model of the individual DSA
The objective function of a DSA is to minimize operational costs in the face of uncertain pricing. To achieve this, the DSA uses demand response strategies and manages the charging and discharging of energy storage systems. The model, accounting for various price scenarios, is structured as follows:
where the objective function (1a) represents the individual operation cost
3.2 Branch power-flow formulation
Following Farivar and Low (2013), the power flow model is established in a radial network using angle and conic relaxation. Additionally, quadratic terms in the power flow constraints are neglected since the branch powers
where the active and reactive powers in a branch are defined by constraints in Equations 2a, b, while the injection power of node
3.3 Operation cost for cooperative DSAs
Supported by the distribution network, DSAs engage in energy interaction with neighbors to share idle energy while reducing disturbance to the main grid. Taking into account the impact of uncertain prices and the demand response, their cooperative formulation is expressed as follows:
Unlike the individual operation in model (1), the interaction variable
3.4 General Nash bargaining game-based energy interaction
The general Nash bargaining game-based scheduling strategy is proposed to incentivize energy interaction among DSAs and ensure that benefits are allocated according to the contribution of each participant.
DSAs engage in energy interaction with neighbors to maximize social welfare, as expressed in the objective function (Equation 4a). The constraint in Equation 4b ensures that the cooperation cost does not exceed the cost of individual operation, thereby encouraging DSAs to participate in energy interactions. The purchasing cost of a DSA in an energy interaction must equal be to the selling income of neighbors, as represented by the constraint in Equation 4c. Additionally, the contribution of each participant is calculated using the coefficient
where the bargaining power
3.5 Decomposition and solution of the general Nash bargaining problem
According to the proposition put forward by Wang and Huang (2016), the optimal solution of the Nash bargaining problem is equivalent to the cost minimization of DSAs. By combining the individual rationality condition with the constraint in Equation 4b, a feasible payment allocation
where
According to Zhong et al. (2020), since the objective function
where
where
The iteration converges when the primal and dual residues satisfy the following conditions (
Algorithm 1.An improved ADMM algorithm for solving the energy interaction problem of the DSA.
Input: Set iteration index
1:repeat
2: Each DSA solves the local energy interaction problem (9).
3: Update the multipliers
4: Each DSA
5:until Stopping criteria (11) are satisfied,terminate.
4 Case study
Numerical simulations are conducted in a distribution network to evaluate the effect of the demand response and uncertain prices on energy interaction. The system aims to verify the performance of the operation strategy based on the general Nash bargaining game theory. In the case study, simulations are performed on an IEEE 33-bus system with three DSAs located at buses 11, 23, and 29. The operation data, including the forecast value of price, generation, and load demand, are obtained from the study by Chen et al. (2017) and Lu et al. (2020). All simulations are solved using the Gurobi solver (Gurobi Optimization, 2020) in the Python environment on an Intel Core i7 computer.
4.1 Operation cost considering the stochastic prices and demand response
The effect of stochastic prices on the operation strategy is analyzed by comparing the operation costs under multiple price scenarios with the forecast value. The fluctuation in the market prices is modeled using the given probability distribution function, which is assumed to obey the Gaussian distribution. As shown in Figure 2, the forecast values of TOU prices are selected as the mean value with a variance of
The deterministic and stochastic optimization results without/with the demand response are shown in Tables 1, 2, respectively. A negative value indicates the benefit that DSAs gain from selling energy to the distribution network or neighbors. It can be observed that DSAs reduce their operation cost when they participate in energy interaction. Considering the stochastic prices given in Table 1, the total operation cost of three DSAs is reduced by 24.20% under deterministic pricing, while the cost is reduced by 5.44% under uncertain pricing. In other words, compared with deterministic pricing, cooperative profit in energy interaction decreases by 16.98% in stochastic scenarios. The reason may be that, compared to deterministic prices, cooperative costs vary with the price scenarios, ultimately increasing the expected costs.
Considering the demand response, DSAs shift loads to periods of plentiful generation or lower demand through price adjustments or monetary incentives. They also enhance the efficiency of renewable energy utilization by coordinating the charging and discharging decisions of energy storage systems. As shown in Table 2, DSAs respond to prices through peak shaving and valley filling, which decreases the load demand during peak price periods. Consequently, operation costs—whether for individual or cooperative operations—significantly decrease compared to those given in Table 1. In other words, energy interaction among multiple DSAs enhances adaptable performance when considering the demand response.
The operation costs in energy interaction among DSAs will vary if the transmission lines have limitations within the distribution network. For simplicity, a maximum capacity of 100 kWh is considered for the line between nodes 2 and 3. This constraint causes decision changes for DSAs during the process of energy transactions with the distribution network and their neighbors. It can be observed that cooperative costs are always less than those of individual operations, and the demand response further reduces these operation costs.
Congestion impacts the operation decisions and, subsequently, the cooperative costs of DSAs. It restricts energy transmission for DSAs regardless of whether the demand response is considered. For deterministic prices, DSAs schedule battery usage to alleviate congestion, resulting in lower operation costs. However, stochastic prices compel DSAs to respond dynamically, leading to similar final operation costs with normal energy interaction since the total demand remains consistent.
4.2 Energy interaction with the distribution network
Figures 4, 5 illustrate the energy interaction results between the distribution network and DSAs over the course of a day. The positive/negative values represent the energy purchased/sold from/to the distribution network, respectively. We comparatively analyze the interaction quantities between the distribution network and multiple DSAs, regardless of their participation in energy interaction. Given the price scenarios, DSAs adjust their demand for purchasing/selling electricity to achieve cost minimization.
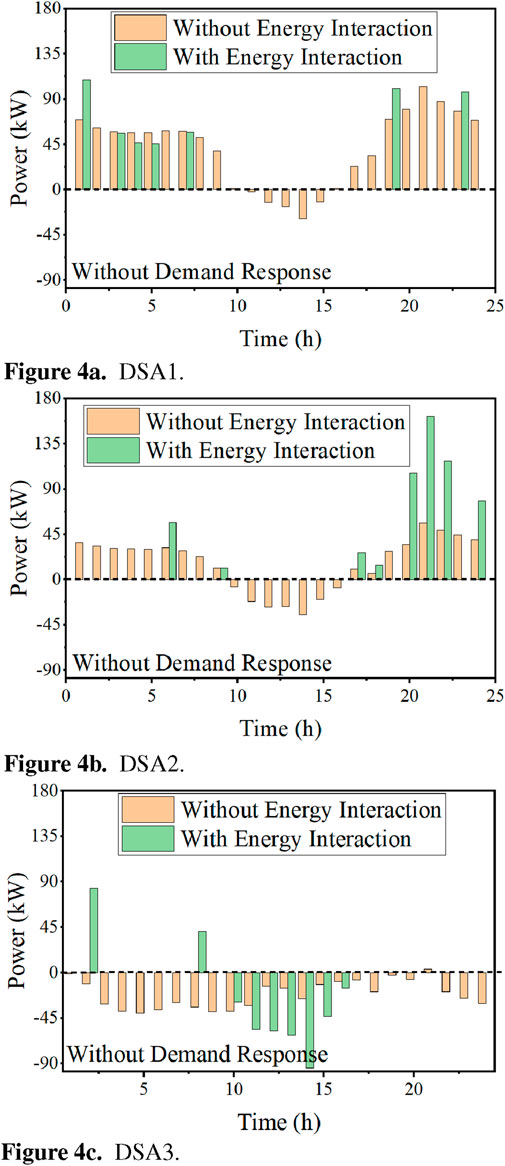
Figure 4. Interaction between the distribution network and DSAs ignoring the demand response. (A) DSA 1, (B) DSA 2, and (C) DSA 3.
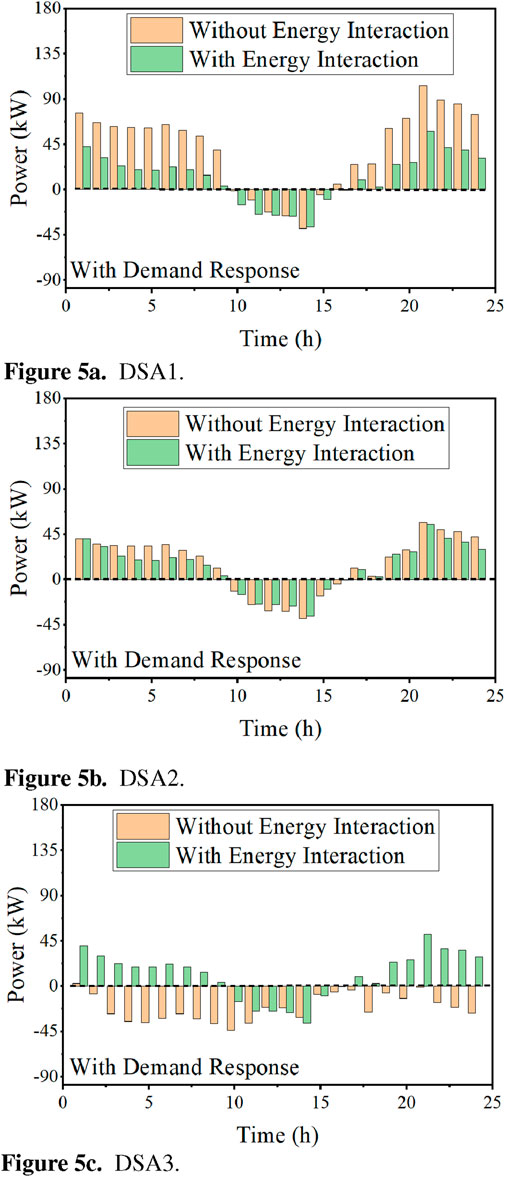
Figure 5. Interaction between the distribution network and DSAs considering the demand response. (A) DSA 1, (B) DSA 2, and (C) DSA 3.
Figure 4 shows the comparison of the effects of individual versus cooperative operation among DSAs on energy interactions with the distribution network. An individual DSA satisfies power balance by leveraging the distribution network and energy storage. Due to the scheduling limitation of the battery, the individual DSA may need to purchase electricity at a high price, increasing the operation costs. In contrast, cooperative DSAs form a group and share idle energy to balance their energy needs. DSAs purchase energy at 0:00–5:00 and 20:00–24:00 while selling energy at 10:00–15:00 to maximize their cooperative benefits during energy interactions. The load regulation capability of DSAs is enhanced when considering the demand response. As shown in Figure 5, DSAs achieve peak shaving and valley filling by leveraging the demand response and energy interactions.
To further analyze the energy interaction, the total interaction quantity between DSAs and the distribution network is summarized in Table 3. The data show that the interaction between DSAs and the distribution network is influenced by both the interactive behavior of DSAs and demand response. We observed that the energy interaction among DSAs can reduce their dependence on the distribution network, enhancing their ability to cope with price uncertainty. To maintain energy balance, they purchase less energy from the distribution network and reduce the quantity of energy sold. Since the demand response is closely tied to electricity prices, DSAs increase their demand when the prices are lower, effectively responding to price uncertainties.
4.3 Analysis of optimal results of the energy storage
The scheduling results of energy storage without/with the demand response are given in Figures 6, 7, respectively. The positive values represent storage discharging, while the negative values indicate storage charging. These figures demonstrate that energy storage can be utilized to satisfy the supply–demand balance through an internal scheduling strategy. As shown in Figure 6, the depth of charge/discharge is higher for the DSA operating individually without a demand response as energy storage is the primary means of shifting energy demand to other periods. This is particularly evident for DSA 1 at 10:00 and DSA 3 at 19:00. Although cooperative DSAs reduce the depth of charging/discharging, energy storage still works in conjunction with energy interaction to maintain energy balance.
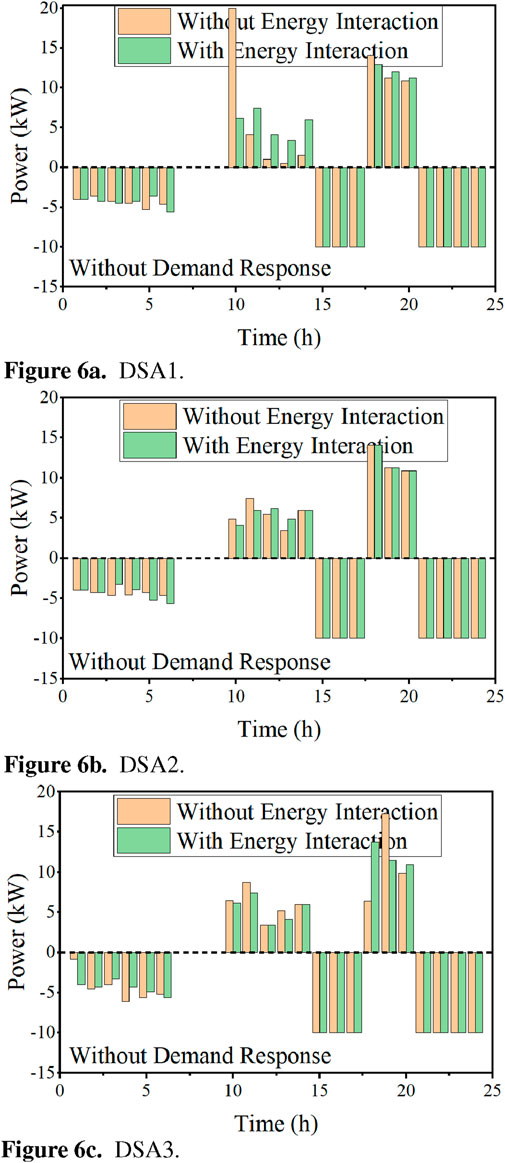
Figure 6. Scheduling result of the energy storage without the demand response. (A) DSA 1, (B) DSA 2, and (C) DSA 3.
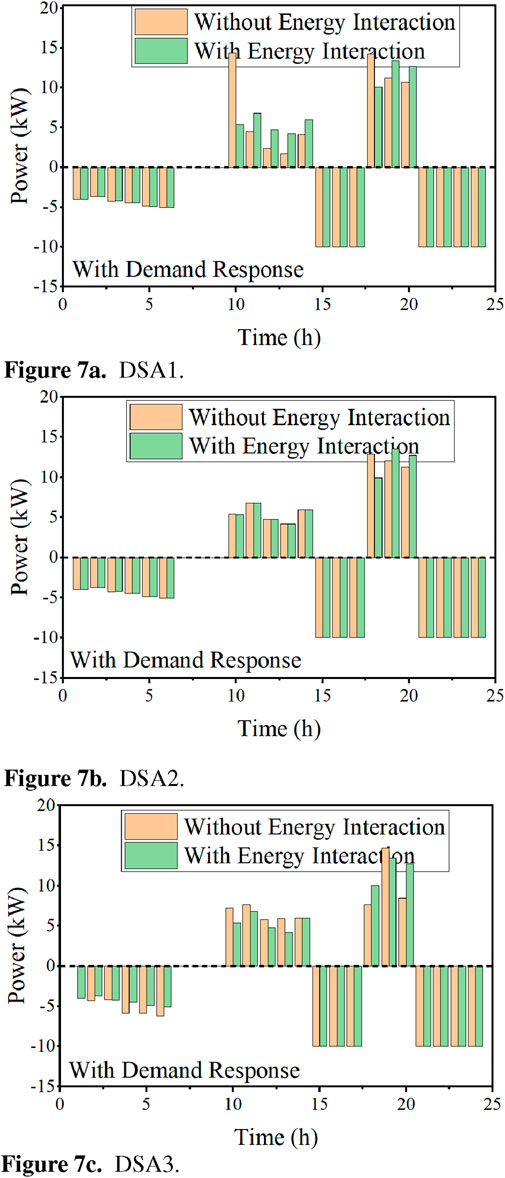
Figure 7. Scheduling result of the energy storage with the demand response. (A) DSA 1, (B) DSA 2, and (C) DSA 3.
The scheduling results of the energy storage considering the demand response are shown in Figure 7. We can observe that the reliance on energy storage decreases as supply–demand balance can be achieved through load shifting. Consequently, the charging and discharging depth of the energy storage for DSA 1 and DSA 3 is reduced, which, in turn, lowers the degradation costs in the objective function.
4.4 Analysis of energy interaction among DSAs
The energy interaction quantities among DSAs are given in Figure 8 when they cooperate with neighbors to share idle energy. The positive values indicate DSA
4.5 Distribution of the node voltage
The node voltage varies with the energy interaction and demand response. Nodes 11, 23, and 29, connected to DSAs, serve as examples, and their voltages are shown in Figure 9. Although the voltage fluctuates across various simulation scenarios and periods, it always remains within the boundary limits. DSA 1, which has the highest load demand, experiences the largest voltage fluctuation, ranging from 0.986 p.u. to 1.001 p.u. Conversely, DSA 2, with the smallest load demand, exhibits relatively smooth voltage fluctuations. The demand response also leads to significant voltage fluctuations at different times. As shown in Figures 9A, C, DSAs shift loads to maintain the supply–demand balance, thereby altering the voltage distribution within the network.
5 Conclusion
This paper presents an energy interaction framework for DSAs to enhance the local consumption of renewable generation. A general Nash bargaining theoretic model is established, taking into account the effect of uncertain prices and demand response. The typical price scenarios are depicted via Monte Carlo sampling and clustering. Given the price scenarios, DSAs make the optimal decisions by shifting loads to the plentiful generation or lower demand time through prices or monetary incentives. To solve the energy interaction model, it is decomposed and transformed into a traceable problem by leveraging the logarithmic transformation. In addition, the optimal power flow constraints are incorporated to improve the model’s practicality. The limitation of transmission capacity alters the operation strategies, which affects the operation costs. It decreases the energy exchange with the distribution network and increases the energy interaction among DSAs. An improved ADMM is proposed to solve the energy interaction problem using local information. Numerical simulations are conducted on an IEEE-33 bus system, demonstrating that uncertain prices may increase the total operation costs, while the demand response improves scheduling flexibility. Future work will focus on addressing energy transmission insufficiency due to capacity limitations during energy interactions.
Data availability statement
The original contributions presented in the study are included in the article/Supplementary Material; further inquiries can be directed to the corresponding author.
Author contributions
FW: investigation and writing–original draft. XW: conceptualization and writing–original draft. JL: software and writing–original draft. YL: methodology and writing–original draft. HY: conceptualization and writing–original draft.
Funding
The author(s) declare that financial support was received for the research, authorship, and/or publication of this article. This work was supported by the Research on key technologies to improve application capability of distribution automation in new power system (2023A-016).
Conflict of interest
The authors declare that the research was conducted in the absence of any commercial or financial relationships that could be construed as a potential conflict of interest.
Publisher’s note
All claims expressed in this article are solely those of the authors and do not necessarily represent those of their affiliated organizations, or those of the publisher, the editors, and the reviewers. Any product that may be evaluated in this article, or claim that may be made by its manufacturer, is not guaranteed or endorsed by the publisher.
References
Alizadeh, A., Esfahani, M., Dinar, F., Kamwa, I., Moeini, A., Mohseni-Bonab, S. M., et al. (2024). A cooperative transactive multi-carrier energy control mechanism with p2p energy+ reserve trading using nash bargaining game theory under renewables uncertainty. Appl. Energy 353, 122162. doi:10.1016/j.apenergy.2023.122162
Baharvandi, A., Aghaei, J., Nikoobakht, A., Niknam, T., Vahidinasab, V., Giaouris, D., et al. (2019). Linearized hybrid stochastic/robust scheduling of active distribution networks encompassing pvs. IEEE Trans. Smart Grid 11, 357–367. doi:10.1109/tsg.2019.2922355
Chang, X., Xu, Y., Gu, W., Sun, H., Chow, M.-Y., and Yi, Z. (2020). Accelerated distributed hybrid stochastic/robust energy management of smart grids. IEEE Trans. Industrial Inf. 17, 5335–5347. doi:10.1109/tii.2020.3022412
Chen, S., Wei, Z., Sun, G., Cheung, K. W., Wang, D., and Zang, H. (2017). Adaptive robust day-ahead dispatch for urban energy systems. IEEE Trans. Industrial Electron. 66, 1379–1390. doi:10.1109/tie.2017.2787605
Chen, Y., Mei, S., Zhou, F., Low, S. H., Wei, W., and Liu, F. (2019). An energy sharing game with generalized demand bidding: model and properties. IEEE Trans. Smart Grid 11, 2055–2066. doi:10.1109/tsg.2019.2946602
Dehghanpour, K., and Nehrir, H. (2017). Real-time multiobjective microgrid power management using distributed optimization in an agent-based bargaining framework. IEEE Trans. Smart Grid 9, 6318–6327. doi:10.1109/tsg.2017.2708686
Farivar, M., and Low, S. H. (2013). Branch flow model: relaxations and convexification-part i. IEEE Trans. Power Syst. 28, 2554–2564. doi:10.1109/tpwrs.2013.2255317
Hirsch, A., Parag, Y., and Guerrero, J. (2018). Microgrids: a review of technologies, key drivers, and outstanding issues. Renew. Sustain. Energy Rev. 90, 402–411. doi:10.1016/j.rser.2018.03.040
Jiang, Q., Mu, Y., Jia, H., Cao, Y., Wang, Z., Wei, W., et al. (2022). A stackelberg game-based planning approach for integrated community energy system considering multiple participants. Energy 258, 124802. doi:10.1016/j.energy.2022.124802
Jin, Y., Choi, J., and Won, D. (2020). Pricing and operation strategy for peer-to-peer energy trading using distribution system usage charge and game theoretic model. Ieee Access 8, 137720–137730. doi:10.1109/access.2020.3011400
Khorasany, M., Paudel, A., Razzaghi, R., and Siano, P. (2020). A new method for peer matching and negotiation of prosumers in peer-to-peer energy markets. IEEE Trans. Smart Grid 12, 2472–2483. doi:10.1109/tsg.2020.3048397
Kim, H., Lee, J., Bahrami, S., and Wong, V. W. (2019). Direct energy trading of microgrids in distribution energy market. IEEE Trans. Power Syst. 35, 639–651. doi:10.1109/tpwrs.2019.2926305
Kumar, K. P., and Saravanan, B. (2017). Recent techniques to model uncertainties in power generation from renewable energy sources and loads in microgrids–a review. Renew. Sustain. Energy Rev. 71, 348–358. doi:10.1016/j.rser.2016.12.063
Li, G., Li, Q., Liu, Y., Liu, H., Song, W., and Ding, R. (2022). A cooperative stackelberg game based energy management considering price discrimination and risk assessment. Int. J. Electr. Power and Energy Syst. 135, 107461. doi:10.1016/j.ijepes.2021.107461
Li, J., Zhang, C., Xu, Z., Wang, J., Zhao, J., and Zhang, Y.-J. A. (2018). Distributed transactive energy trading framework in distribution networks. IEEE Trans. Power Syst. 33, 7215–7227. doi:10.1109/tpwrs.2018.2854649
Lu, S., Gu, W., Zhang, C., Meng, K., and Dong, Z. (2020). Hydraulic-thermal cooperative optimization of integrated energy systems: a convex optimization approach. IEEE Trans. Smart Grid 11, 4818–4832. doi:10.1109/tsg.2020.3003399
Luo, C., Zhou, X., and Lev, B. (2022). Core, shapley value, nucleolus and nash bargaining solution: a survey of recent developments and applications in operations management. Omega 110, 102638. doi:10.1016/j.omega.2022.102638
Paudel, A., Chaudhari, K., Long, C., and Gooi, H. B. (2019). Peer-to-peer energy trading in a prosumer-based community microgrid: a game-theoretic model. IEEE Trans. Industrial Electron. 66, 6087–6097. doi:10.1109/tie.2018.2874578
Sarker, E., Seyedmahmoudian, M., Jamei, E., Horan, B., and Stojcevski, A. (2020). Optimal management of home loads with renewable energy integration and demand response strategy. Energy 210, 118602. doi:10.1016/j.energy.2020.118602
Tushar, W., Saha, T. K., Yuen, C., Morstyn, T., McCulloch, M. D., Poor, H. V., et al. (2019). A motivational game-theoretic approach for peer-to-peer energy trading in the smart grid. Appl. energy 243, 10–20. doi:10.1016/j.apenergy.2019.03.111
Tushar, W., Saha, T. K., Yuen, C., Smith, D., and Poor, H. V. (2020). Peer-to-peer trading in electricity networks: an overview. IEEE Trans. Smart Grid 11, 3185–3200. doi:10.1109/tsg.2020.2969657
Tushar, W., Yuen, C., Mohsenian-Rad, H., Saha, T., Poor, H. V., and Wood, K. L. (2018). Transforming energy networks via peer-to-peer energy trading: the potential of game-theoretic approaches. IEEE Signal Process. Mag. 35, 90–111. doi:10.1109/msp.2018.2818327
Vieira, G., and Zhang, J. (2021). Peer-to-peer energy trading in a microgrid leveraged by smart contracts. Renew. Sustain. Energy Rev. 143, 110900. doi:10.1016/j.rser.2021.110900
Wang, H., and Huang, J. (2016). Incentivizing energy trading for interconnected microgrids. IEEE Trans. Smart Grid 9, 2647–2657. doi:10.1109/tsg.2016.2614988
Wang, L., Zhang, Y., Song, W., and Li, Q. (2021). Stochastic cooperative bidding strategy for multiple microgrids with peer-to-peer energy trading. IEEE Trans. industrial Inf. 18, 1447–1457. doi:10.1109/tii.2021.3094274
Wei, C., Shen, Z., Xiao, D., Wang, L., Bai, X., and Chen, H. (2021). An optimal scheduling strategy for peer-to-peer trading in interconnected microgrids based on ro and nash bargaining. Appl. Energy 295, 117024. doi:10.1016/j.apenergy.2021.117024
Zhong, W., Xie, S., Xie, K., Yang, Q., and Xie, L. (2020). Cooperative p2p energy trading in active distribution networks: an milp-based nash bargaining solution. IEEE Trans. Smart Grid 12, 1–1276. doi:10.1109/tsg.2020.3031013
Nomenclature
Keywords: multiple distribution substation areas, energy interaction, uncertain prices, demand response, generalized Nash bargaining
Citation: Wang F, Wen X, Li J, Liu Y and Yu H (2024) Cooperative energy interaction for neighboring multiple distribution substation areas considering demand response. Front. Energy Res. 12:1413769. doi: 10.3389/fenrg.2024.1413769
Received: 07 April 2024; Accepted: 26 August 2024;
Published: 19 September 2024.
Edited by:
Lorenzo Ferrari, University of Pisa, ItalyReviewed by:
Yunting Yao, Nanjing Normal University, ChinaDuong Nguyen, Arizona State University, United States
Copyright © 2024 Wang, Wen, Li, Liu and Yu. This is an open-access article distributed under the terms of the Creative Commons Attribution License (CC BY). The use, distribution or reproduction in other forums is permitted, provided the original author(s) and the copyright owner(s) are credited and that the original publication in this journal is cited, in accordance with accepted academic practice. No use, distribution or reproduction is permitted which does not comply with these terms.
*Correspondence: Feng Wang, d2Zfc2N1QDE2My5jb20mI3gwMjAwYTs=