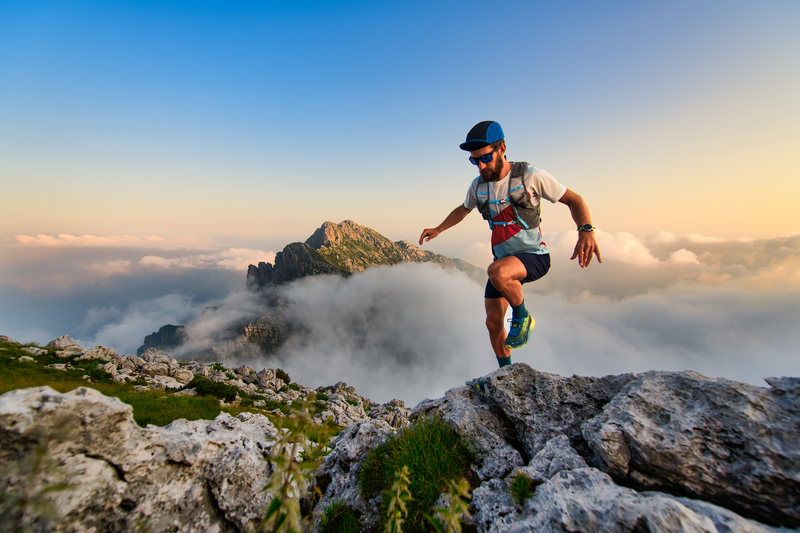
94% of researchers rate our articles as excellent or good
Learn more about the work of our research integrity team to safeguard the quality of each article we publish.
Find out more
ORIGINAL RESEARCH article
Front. Energy Res. , 17 February 2025
Sec. Sustainable Energy Systems
Volume 12 - 2024 | https://doi.org/10.3389/fenrg.2024.1384995
This article is part of the Research Topic Urban Energy System Planning, Operation, and Control with High Efficiency and Low Carbon Goals View all 29 articles
This study presents a Data-Enhanced Optimum Load Frequency Control (DEO-LFC) strategy for microgrids, targeting an optimal balance between generation costs and frequency stability amidst high renewable energy integration. By replacing traditional controls with agent-based systems and reinforcement learning, the DEO-LFC employs an optimal balance between generation costs and frequency stability amidst high renewable energy integration. By replacing traditional controls with agent-based systems and reinforcement learning, the DEO-LFC employs a Soft Graph Actor Critic (SGAC) algorithm, integrating deep reinforcement learning with graph sequence neural networks for effective frequency management. Proven effective in the China Southern Grid’s island microgrid model, DEO-LFC offers a sophisticated solution to the challenges posed by the island microgrid model. Proven effective in the China Southern Grid’s island microgrid model, DEO-LFC offers a sophisticated solution to the challenges posed by the variability of modern power grids.
In the context of escalating concerns over fossil fuel depletion, the importance of renewable energy in enhancing smart grid capabilities has surged. Renewable energy sources, inherently constrained by environmental conditions and geographical dispersion, necessitate integration into the power grid via sophisticated inverter technologies, leading to the development of Distributed Generation (DG) (Wang et al., 2013). This shift towards distributed generation presents a stark contrast to traditional centralized power generation systems, offering notable benefits in terms of energy efficiency, environmental sustainability, operational flexibility, reliability, and economic viability.
However, the integration of renewable energy into the power grid introduces challenges related to its unpredictable output, characterized by random intermittency and volatility (Mahboob Ul Hassan et al., 2022). Such unpredictability can compromise the power quality and jeopardize the stability of the grid system (Su et al., 2021). To address these issues and harness the full potential of distributed generation, the microgrid concept has been proposed. As an advanced technological solution predicated on renewable distributed power generation, microgrids are poised to play a pivotal role in the evolution of smart grid infrastructures. They facilitate the integration of diverse small-scale distributed energy resources and loads, ensuring safe and reliable operation both in grid-connected and islanded modes (Huang and Lv, 2023).
In grid-connected mode, microgrids complement the utility grid by supplying power to local loads and potentially exporting surplus energy back to the grid. Conversely, in scenarios of grid failure or disturbances, microgrids transition to islanded mode, independently powering local loads. This operational flexibility, however, necessitates robust control strategies to maintain system stability in the absence of grid support, given that islanded microgrids (IMGs) rely heavily on renewable energy sources (RESs) connected via power electronic converters. This configuration results in a diminished system inertia, posing challenges for frequency stability, reliable power supply, and efficient renewable energy utilization (Hosseini and Etemadi, 2008).
Load Frequency Control (LFC) emerges as a critical mechanism within power systems to balance frequency and active power demand across specific control areas (Bengiamin and Chan, 1982). Achieving optimal LFC performance in islanded microgrids requires a nuanced approach that not only improves frequency control but also minimizes the generation costs associated with distributed energy resources. Traditional LFC strategies, such as proportional-integral control (Mi et al., 2013), model predictive control (Mi et al., 2016), and adaptive control (Chen et al., 1991), often struggle to meet these dual objectives effectively.
Therefore, this discourse underscores the imperative for innovative control strategies that can adeptly manage the unique challenges posed by the integration of renewable energy sources into microgrids. The advancement of microgrid technology and the optimization of LFC mechanisms are essential for realizing the full potential of renewable energy within the smart grid paradigm, ensuring both environmental sustainability and grid stability.
Initial LFC studies stem from the Proportional-Integral (PI) control era, valued for their simplicity and computational ease (Wang et al., 1993). Integrated into LFC, PI controls split into steady-state integral and transient proportional parts. The PI control’s widespread use in LFC hinges on its non-differential regulation efficacy in fundamental power setups. However, as power systems grow and face more stochastic disruptions, PI’s static nature limits its dynamic stability, threatening frequency equilibrium and risking failures (Wang et al., 1994). Scholars have since sought to improve PI for LFC, with (Chen et al., 2022) incorporating sliding mode control for disturbance resilience. The rise of complex, nonlinear, and interconnected power systems demands control strategies that address these traits. Long et al. (2021) introduces a tri-layer LFC model for detailed power system dynamics, with a control strategy for nonlinear management. Yet, its reliance on specific parameters and a model-centric approach limits broad use. This shift from PI to adaptive, interconnected strategies reflects the ongoing effort to manage modern power systems’ complexities. The ongoing evolution of LFC methods highlights the necessity for flexible, robust, and efficient controls to maintain the stability and reliability of our increasingly intricate and interconnected power grids.
Model Predictive Control (MPC) uses dynamic models, typically linear empirical ones, to predict and optimize system behavior over a future time span, adjusting the present state with future constraints in mind (Peng et al., 2023). This allows for real-time feedback and corrections. A distributed MPC algorithm promotes collaborative LFC between wind and thermal plants, improving overall system performance through dynamic cooperation.
Adaptive Control (AC), on the other hand, adjusts its parameters and rules in response to changing system conditions, maintaining stability despite uncertainties and significant disturbances without needing known variability bounds (Yan et al., 2022). AC in LFC, via adaptive dynamic programming, minimizes frequency deviations in grids, requiring less reliance on prior knowledge than MPC. However, AC systems tend to be more complex and costly.
Traditional controls often lack adaptability, affecting the performance and cost of frequency control in islanded microgrids. AI algorithms offer a solution, handling nonlinear complexities and improving operational efficiency and stability. AI learns and adapts to changing conditions, refining LFC strategies, enhancing energy efficiency, and ensuring reliable power supply. AI’s predictive abilities also help prevent system failures, increasing microgrid resilience to uncertainties and disturbances.
In the modern era of islanded microgrids, which significantly integrate renewable energy resources, the complexity and interconnectedness of information flow across various regions necessitate a systematic approach for prioritizing the use of novel energy sources. Traditional LFC strategies face challenges in navigating the intricate decision-making processes required for efficient energy management. Within the realm of computer science, Artificial Intelligence (AI) emerges as a critical field, striving to emulate human cognitive abilities, including learning, decision-making, and problem-solving. The inherent capability of AI to engage with and learn from its environment independently positions it as a formidable tool for addressing complex challenges in energy systems.
The integration of AI with LFC mechanisms represents a pioneering effort to transcend the limitations of conventional LFC methods. For example, the work of Jia et al. (2019) showcases the successful application of Q-learning to LFC, significantly enhancing system adaptability through the ongoing refinement of the state-action matrix for comprehensive power control in simplified models. Furthermore, Yu et al. (2012) have introduced an innovative imitation learning strategy that integrates eligibility traces into reinforcement learning, yielding improved LFC performance in islanded systems through faster convergence and enhanced dynamic capabilities. In another notable advancement, Yu et al. (2015) have developed a cooperative reinforcement learning strategy, employing multiple intelligent agents to devise an optimal unified control strategy, effectively addressing the challenges posed by the interconnectivity of disparate control regions.
Additionally, Zhang et al. (2023a) have constructed a tri-level architecture for a multi-agent system, enabling coordinated control over LFC and Automatic Voltage Control (AVC). This architecture leverages the autonomous, independent, and collaborative nature of intelligent agents to ensure logical consistency while decentralizing control functions physically. In a groundbreaking approach, Xi et al. (2018) propose the Evolutionary Population Cooperative Control (EPCC) strategy, utilizing a win-lose criterion and space-time tunneling concept to quickly achieve Nash equilibrium within a multi-agent system (MIS) framework. This strategy, rooted in the Multi-Agent System Stochastic Consensus Game (MAS-SCG), promotes frequent information exchanges among intelligent agents, demonstrating the potential of AI to enhance decision-making and operational efficiency in complex energy systems.
Reinforcement Learning (RL) is a key machine learning paradigm that focuses on devising strategies for agents to make optimal decisions to meet set objectives through interactions with environmental states. Utilizing a Markov decision process, RL entails recognizing states and selecting actions guided by rewards, leading to state transitions. Studies have investigated applying power system’s instantaneous frequency and transmission line power flow as RL environmental states, with power allocation directives as action decisions, tackling power allocation challenges effectively. (Yu et al., 2011; Shangguan et al., 2021; Zhang et al., 2023b).
Zhang et al. (2020) have considered the total profit of power generation companies, incorporating dispatch mileage compensation into power command allocation to enhance the economic efficiency of power generation. Li et al. (Zhang et al., 2021) introduced an adaptive distributed auction algorithm for optimizing LFC power command allocation, minimizing the deviation between total and allocated power commands. This method is praised for its rapid convergence and model-free nature, ensuring precise generator power control. Moreover, Li et al. (2021) proposed a double-delay deep deterministic policy gradient algorithm, augmented by a multi-experience pool probabilistic replay strategy, improving controller training efficiency, action instruction quality, and mitigating stochastic perturbations in systems incorporating new energy sources, highlighting the evolving applications of RL in power system optimization.
In the dynamically evolving context of islanded microgrids, enriched with a diverse array of renewable energy resources, the exploration of AI control strategies combined with RL distribution tactics is underway to realize an intelligently integrated LFC system across multiple regions. This research endeavor has led to the development of multi-regional, multi-layered distributed LFC frameworks, enabling intelligence dissemination from macro to micro levels (Xi et al., 2016a; Xi et al., 2016b). To address these limitations and enhance generalizability, Xi et al. (2021) replaced the traditional wolf climbing LFC algorithm with PDWoLF-PHC, proposing a VWPS-HDC method that offers improved performance through time-consistent climbing. Additionally, to overcome the drawbacks of the WPH algorithm, Xi et al. (2022) introduced a cost-consistent VWPC-HDC method, achieving faster dynamic optimization, enhanced robustness, and reduced generation costs.
However, the practicality of these methodologies, based on the wolf pack hunting principle, is constrained by their reliance on extensive knowledge systems. To mitigate these limitations.
The challenge of ensuring wide-ranging applicability in the domain of standalone microgrid Load Frequency Control (LFC) remains a critical issue. It necessitates the creation of control frameworks and algorithms that can effectively operate in diverse scenarios beyond the scope of their original design. This adaptability is essential for managing the dynamic operational landscapes and the variability in demand that are characteristic of isolated microgrids. The integration of a diverse set of techniques, alongside reinforcement learning, is vital for enhancing the robustness and adaptability required to navigate changes in the environment.
This paper introduces the Data-Enhanced Optimum Load Frequency Control (DEO-LFC) methodology, which is designed to achieve a harmonious balance between generation costs and frequency stability in microgrids with a substantial integration of renewable energy sources. The Soft Graph Actor Critic (SGAC) algorithm is presented as a groundbreaking fusion of deep reinforcement learning and graph sequence neural network models, tailored to manage the intricacies of adaptive frequency regulation. By employing a Markov decision process for system modeling and a graph to sequence neural network for policy function approximation, the DEO-LFC approach highlights its potential impact. Its application to the isolated island city microgrid model within the China Southern Grid serves as a testament to its effectiveness in modern electrical grid settings.
The main contributions of this paper are summarized as follows.
1) Introduction to the DEO-LFC Methodology: The DEO-LFC methodology signifies a paramount advancement in the realm of frequency stability enhancement and cost minimization in isolated microgrids, especially those with substantial renewable energy sources integration. This methodological shift toward employing agent-based systems, which utilize reinforcement learning algorithms, marks a departure from conventional control strategies. The DEO-LFC framework presents an innovative, adaptive approach to managing frequency control challenges in complex operational contexts. It specifically targets the issues arising from the fluctuating nature of renewable energy sources, thereby facilitating a more reliable and cost-effective energy management system.
2) Creation of the SGAC Algorithm: Central to the DEO-LFC methodology is the groundbreaking creation of the SGAC (Simultaneous Graph-based Actor-Critic) algorithm. This state-of-the-art algorithm fuses the sophistication of deep reinforcement learning with the nuanced processing capabilities of graph sequence neural networks, making it uniquely equipped to navigate the complexities of load frequency control. The algorithm employs a Markov decision process for comprehensive system modeling and is further enhanced by the integration of advanced iterative learning techniques. The SGAC algorithm’s design is purposefully crafted to devise an optimal strategy for frequency management, showcasing an innovative approach that elevates the performance and reliability of modern electrical power grids.
The organisation of this manuscript is as follows: Section 2 delineates the configuration of the islanded microgrid system. Subsequently, Section 3 introduces a groundbreaking approach, detailing its structural framework. Section 2 delineates the configuration of the islanded microgrid system. Section 4 is dedicated to the execution of case studies designed to evaluate the proposed method’s efficacy. Finally, Section 5 concludes the document by providing a comprehensive summary and discussing the principal outcomes derived from the research conducted. Finally, Section 5 concludes the document by providing a comprehensive summary and discussing the principal outcomes derived from the research conducted.
In microgrids, integration of Distributed Generation (DG) units such as Photovoltaic (PV), Wind Power (WP), and Energy Storage (ES) systems is achieved via grid-connected inverter interfaces, which allow these units to align with desired power outputs through specific control mechanisms. A simplified model for these inverters is used to explain the Load Frequency Control (LFC) framework, highlighting the role of traditional, renewable, and storage energy sources in frequency regulation.
Figure 1 illustrates an autonomous microgrid setup featuring diverse generation sources like diesel engines, micro gas turbines, fuel cells, PV, wind turbines, ES systems, and consumer loads. Here, diesel engines and ES systems play a crucial role in frequency regulation, while renewables focus on maximizing power output through Maximum Power Point Tracking (MPPT), offering limited frequency support. The control system of the microgrid dynamically distributes power to match demand, prioritizing efficiency, sustainability, and stability.
For independent operation, microgrids require self-adjusting power generation for voltage and frequency stability, incorporating Primary Frequency Control (PFC) and LFC mechanisms. PFC deals with immediate power output adjustments in response to frequency changes, whereas LFC involves coordinated efforts across multiple sources to correct frequency discrepancies, typically managed by centralized controllers and communication systems. ES and diesel generators are key to microgrid frequency stability, with PV and WP units focusing on MPPT due to their variable output.
Recent research suggests strategies for integrating wind and solar into frequency regulation by reserving part of their output to improve system response. Yet, the focus remains on PFC. This study explores how diesel and ES significantly contribute to frequency stability, managing variances in power supply. Wind and solar, despite their fluctuating nature, are considered less reliable for maintaining balance and stability in microgrids.
This paper introduces a DEO-LFC method designed to optimize generation costs while ensuring frequency stability in microgrids rich in renewables. The DEO-LFC strategy balances cost-efficiency with the critical need for frequency stability, addressing the challenges posed by high renewable energy integration in isolated microgrids.
The framework employs advanced algorithms for adaptive frequency regulation, adept at navigating the complex dynamics characteristic of such systems. It promises improved performance, especially in mitigating the unpredictability associated with renewable energy sources. By integrating data-driven insights and knowledge-based control, the DEO-LFC approach enhances the reliability and efficiency of frequency management in microgrids, aligning operational expenditures with the overarching goal of frequency stabilization. This methodological innovation stands to significantly advance the operational robustness of isolated microgrids, ensuring stability amidst the fluctuating nature of renewable energy contributions.
Diesel generators (DGs) serve as pivotal controllable Distributed Generation (DG) units within microgrids, offering low operational costs and high reliability but posing environmental concerns. They are particularly crucial in islanded microgrid systems (Su et al., 2021), where they significantly contribute to maintaining the equilibrium between power supply and demand. However, DGs exhibit minimum operational power thresholds, leading to inefficiencies under low-load conditions. Consequently, optimizing the usage of diesel generators necessitates minimizing their operation at low loads while prioritizing their deployment for higher load demands. This strategy ensures efficient energy production and enhances the overall operational efficacy of microgrid systems, aligning with the objectives of balancing energy supply with demand while addressing the inherent limitations of DGs. The relationship between diesel generator fuel and power is given as follows.
where Q is the fuel per hour of the diesel generator, P is the current power of the diesel generator, P0 is the rated power, α and β are the fuel consumption factors.
In the early stage of invention of micro gas turbine, due to the immaturity of related technology, which resulted in low power generation efficiency, the usage rate of micro gas turbine was not very high at first, but with the development of power generation technology, the power generation efficiency has been gradually improved, and the practicality has been enhanced after the size is reduced. As a kind of controllable distributed power generation unit, when the renewable energy power generation equipment becomes unstable due to the natural environment, the micro gas turbine can be adjusted to coordinate the microgrid to achieve the optimal operation state.
MGTs operate as rotary heat engines utilizing fuel and air, emerging as viable, energy-efficient, and eco-friendly power solutions suitable for urban, rural, and remote applications. The MGT system comprises components such as a gas bath wheel, combustion chamber, reheater, and compressor. The process involves air being drawn in and pressurized by the compressor, then preheated and mixed with fuel in the combustion chamber. The resultant high-temperature, high-pressure gas drives an electric motor to produce electricity. The output power of MGTs is directly proportional to fuel consumption, necessitating a mathematical model to optimize cost efficiency. This technological evolution underscores the MGT’s significance in enhancing microgrid resilience and sustainability across diverse geographical locales. The magnitude of the generation power of the micro gas turbine is determined by the consumption of the fuel, and the mathematical model of its cost is as follows (Hosseini and Etemadi, 2008):
where CMT is the fuel cost of the micro gas turbine, C is the unit price of the fuel gas, PMT is the power generated by the micro gas turbine at t, LHV is the low calorific value of natural gas.
The power output of Fuel Cells (FCs) is directly correlated with the quantity of fuel supplied, allowing for modulation of power levels through the adjustment of fuel flow rates. FCs are characterized by their superior dynamic response capabilities, enabling rapid adjustments to power output in response to varying operational demands. This attribute not only enhances the adaptability of FCs within energy systems but also underscores their potential in applications requiring quick response times and flexible power generation.
where
Wind Turbines (WTs) serve as devices for transforming airflow kinetic energy into mechanical energy, subsequently converted into electrical energy. The primary components of a WT include blades, a gearbox, and a generator. The process entails wind propelling the blades, thereby converting the wind’s kinetic energy into mechanical energy. This mechanical energy is then amplified through gearbox-mediated blade acceleration, which powers the generator to convert mechanical energy into magnetic field energy, and ultimately into electrical energy. The correlation between a WT’s output power and wind speed is mathematically represented in Equation 5, illustrating the efficiency of energy conversion under varying wind conditions:
where
Photovoltaic (PV) power generation system is a new type of power generation model that uses the photovoltaic effect of semiconductor materials to directly convert solar radiation energy into electricity. The photovoltaic effect refers to the change of carrier distribution state and concentration of semiconductor materials after they are exposed to light, thus generating electric current and electric potential. Photovoltaic power generation system consists of photovoltaic panel module, controller, inverter and transformer, as shown in Figures 2, 3, the photovoltaic panel module is easily affected by external conditions, making its output energy is not stable enough, the battery as an energy storage device, can be converted to solar panels to store solar energy, so as to meet the needs of continuous load operation. The temperature of the PV array at a certain moment is related to the current solar radiation intensity and the warming coefficient of the PV array, and the temperature of the PV array at a certain moment is given by the following equation.
where
where
The formulation of generation cost within the electricity production sphere is articulated via a sophisticated mathematical model, meticulously capturing the comprehensive economic obligations of power generation firms. This model is all-encompassing, amalgamating crucial operational cost elements integral to the generation process. It assimilates direct costs, such as fuel expenses encompassing a spectrum from fossil to renewable sources, and extends to cover the wide range of maintenance demands for generation infrastructure, encompassing routine checks, parts replacement, and emergency repairs. Furthermore, the model includes labor expenses covering wages, training, and health and safety measures for personnel, along with various indirect costs. These indirect expenses encompass regulatory compliance charges, environmental levies, and investments in technological innovation, essential for the electricity generation continuum.
Crafted with precision, the model reflects the intricate dynamics prevalent in the energy sector by integrating both variable costs, which alter with production levels and operational intensity, and fixed costs, which are invariant to output volume. This dual approach offers a comprehensive perspective on the economic terrain of electricity generation, covering the spectrum from initial capital outlay to incremental operating expenses.
Merging such varied economic components into a unified model provides stakeholders with an in-depth view of the critical economic considerations vital for prudent and sustainable power generation management. It facilitates a detailed comprehension of the interplay between different cost determinants and their collective influence on the cost-efficiency and -effectiveness of power plants. Ultimately, this model transcends being a mere cost inventory, evolving into a dynamic tool that underpins strategic decision-making and future-oriented planning, pivotal for the advancement of the power generation industry. The cost of power generation is as follows:
where PGi is the output of the ith unit, ai, bi,ci are constants, and Ci is the cost of the ith unit.
where ΔPGi is the output of ith unit, PGi,actual is the output of ith unit,αi, βi, γi are coefficients.
The Differential Evolution Optimization for Load Frequency Control (DEO-LFC) framework represents a vanguard methodology in the domain of electrical grid management, specifically engineered to fortify frequency stability across power networks—a critical factor for ensuring uninterrupted service and superior power quality within microgrid configurations. Achieving and maintaining an exact frequency balance is of paramount importance, as deviations from the established frequency spectrum can lead to detrimental effects, such as the deterioration of infrastructure, compromised quality of electricity, and a heightened risk of grid instability. Within the realm of microgrid management, the economic aspects of power generation take on a significant role, deeply influencing the operational dynamics and the economic viability of these systems. The implementation of efficacious frequency control measures is indispensable for reducing superfluous energy consumption and operational expenses, thereby enhancing the economic efficiency of microgrids, optimizing the use of resources, and improving the cost-efficiency of power generation initiatives.
Islanded microgrids, characterized by their compact scale and increased susceptibility to fluctuations in load demand, encounter unique challenges in achieving consistent frequency control. These standalone power systems require intricate and flexible management strategies that can effectively align the twin goals of cost minimization and optimization of system performance. The DEO-LFC approach addresses these challenges by deploying an innovative multi-objective optimization framework, carefully crafted to strike a balance between cost-effectiveness and dependable system performance. This framework is designed to minimize the adverse effects of operational constraints while preserving the integrity of economic and performance objectives, thus embodying a holistic strategy that caters to both economic and operational performance imperatives.
Incorporating multi-objective optimization techniques, the DEO-LFC methodology skillfully manages the intricate interplay between grid stability and economic factors in power generation. It delivers a refined solution that adeptly adjusts the equilibrium between ensuring grid stability and contemplating the economic dimensions of power generation. This versatile and comprehensive approach is uniquely suited to address the fluctuating demands of microgrid settings, guaranteeing frequency stability alongside a commitment to operational efficiency and fiscal judiciousness. The strategic formulation of objective functions and constraints under this methodology underscores its capacity to navigate the complexities of modern power systems, providing a robust framework for the sustainable and efficient management of energy resources in microgrids. Through the meticulous design of its optimization processes, the DEO-LFC strategy exemplifies an advanced paradigm in grid management, advocating for a harmonious integration of technical and economic considerations to foster resilient and economically viable microgrid ecosystems. The objective functions and constraints are as follows.
where ΔPorder-∑ is the total command, ΔPimax and ΔPimin are the limits of the ith unit, ΔPiin is the command of the ith unit.
In the realm of Artificial Intelligence (AI), rapid advancements have necessitated the adaptation to complex task deployment, with edge computing environments emerging as a pivotal solution due to their low latency, high throughput, and energy-efficient characteristics. These environments are increasingly applied across various sectors including intelligent Internet of Things (IoT), transportation, and healthcare, demanding efficient task deployment to maximize computing performance and resource allocation. Yet, task deployment poses a complex combinatorial optimization challenge, entangled with inter-task dependencies and multifaceted constraints. To navigate these complexities, the scholarly and industrial sectors have proposed innovative approaches, notably Graph Neural Networks (GNNs) and Deep Reinforcement Learning (DRL) methodologies.
GNNs offer a graphical framework to encapsulate inter-task dependencies, typically represented by a Directed Acyclic Graph (DAG) in LFC scenarios. The adoption of a graph structure transmutes the LFC challenge into a graph combinatorial optimization problem, thereby enhancing LFC’s efficiency and robustness. GNNs, as a subset of artificial neural networks adept at processing graph data, can discern and manage task dependencies, facilitating superior task deployment outcomes.
Conversely, DRL, a subset of reinforcement learning that derives optimal strategies through environmental interaction, is instrumental in refining LFC strategies. DRL optimizes LFC policies to augment efficiency and precision, assimilating task dependencies and constraints to advance LFC performance.
This section delves into the synthesis of GNNs and DRL to tackle the LFC dilemma, particularly in isolated microgrid contexts. It envisages employing GNNs for delineating task interdependencies and optimizing these relationships. Concurrently, DRL will be leveraged to formulate and implement optimal LFC policies, aiming to address the intricate LFC issues inherent in islanded microgrids. This integrative approach signifies a promising direction for enhancing task deployment and LFC efficacy in complex computational landscapes.
In Reinforcement Learning (RL), the dynamic interaction between an agent and its environment is conceptualized through a Markov Decision Process (MDP), serving as both the mathematical foundation and a key modeling instrument for RL challenges. An MDP framework typically encapsulates a state space, action space, state transition probabilities, and a reward function. Within this structure, the agent selects actions in accordance with the present state at each discrete time step, while the environment responds by presenting a subsequent state and associated reward, as dictated by the state transition probability function and the reward function. The MDP framework posits that state transitions in the decision-making process adhere to the Markov property—meaning the forthcoming state is contingent solely on the current state and the executed action, devoid of any historical influence. The quintessential components of an MDP include.
1) State space: the set of all possible states.
2) Action space: The set of all possible actions.
3) State transfer probability: describes the probability distribution from one state and one action to the next state.
4) Reward function: describes the immediate reward obtained after performing an action in a state.
In MDP, the goal of an agent is to find an optimal strategy, i.e., to choose an optimal action in each state to maximize the expected cumulative reward.
In the sphere of sophisticated power grid management, the imperative for an advanced control system is pronounced. Such a system is essential for the generation and distribution of precise control directives to every unit within specified sectors, highlighting the need for a comprehensive action space for the supervisory entity. This action space is crucial, crafted to encompass a full array of commands vital for the seamless operation of each unit. The contemplated action space is intricate, mirroring the wide array of decisions the controlling agent must implement. These decisions span various operational aspects, from adjusting power output levels to fine-tuning for system equilibrium and reliability. The complexity inherent in this space reflects the diverse nature of the required tasks, emphasizing the necessity for the agent to exhibit exceptional precision and adaptability.
Furthermore, the action space illustrates the complex coordination and synergy required among different units to attain collective operational efficacy. It establishes a structure that not only facilitates task execution at the individual unit level but also integrates these activities within the broader grid management goals. This integration demands a degree of interaction and cooperation that goes beyond mere directive issuance, necessitating a unified approach that aligns with overarching performance objectives.
Thus, the agent must adeptly navigate this action space, informed by the dynamic interrelations within the power grid, to make decisions that are both cognizant of the current context and anticipatory of future grid conditions. Such advanced decision-making capability is crucial for ensuring optimal grid performance, reducing operational interruptions, and enabling a resilient adaptation to fluctuating demand and supply scenarios. The meticulously designed action space is a fundamental element of the control architecture, endowed with the complexity and strategic insight necessary to meet the rigorous demands of contemporary grid operation and management. The action space is as follows:
where
The autonomous control agent plays a pivotal role in diligently managing a comprehensive database of operational metrics for the standalone microgrid. Its core function is to meticulously implement decisions that adjust for frequency deviations, leveraging an extensive collection of real-time and historical data. This role is crucial for the continuous monitoring and adjustment of the power output from each turbine unit, especially critical in environments lacking rapid-response mechanisms to counteract significant power fluctuations.
Ultimately, this meticulous and strategic methodology endows the autonomous agent with the capabilities required to uphold the operational integrity of the microgrid. It underscores the agent’s critical contribution to maintaining the resilience, efficiency, and stability of the energy system, navigating the intricate dynamics of managing standalone power grids effectively. The state space is as follows:
where
Within the domain of power system operational efficiency optimization, reinforcement learning algorithms frequently utilize two paramount metrics as reward functions: frequency deviation and generation cost. These metrics are integral for assessing system performance and economic viability, respectively. To bolster the training efficacy and mitigate the risk of frequency tuning errors during the exploration phase, a penalty factor is strategically implemented. This factor is aimed at accelerating the learning curve by imposing penalties for actions resulting in non-ideal outcomes, such as deviations from the desired frequency levels.
The incorporation of a penalty factor serves to guide the learning algorithm towards optimal actions by introducing a cost for inaccuracies, thereby enhancing the training process’s efficiency and dependability. This approach addresses the exploration-exploitation dilemma in reinforcement learning, necessitating a balance between investigating novel actions and leveraging established strategies. This equilibrium is crucial, especially in intricate systems where the ramifications of less-than-optimal decisions can significantly impact system stability and operational expenses.
By embedding a penalty factor focused on rectifying frequency tuning discrepancies, the training methodology is refined to emphasize system stability and cost efficiency. Consequently, this adjustment improves the power system’s operational performance, aligning with the objectives of maintaining system reliability and economic efficiency. The reward is as follows:
where r is the reward and Ci is the punishment function.
Upon reviewing the trio of DRL algorithms delineated in the preceding section, it becomes apparent that the challenges confronting the DRL domain are fundamentally consistent. These challenges include the intricacies of exploration and decision-making within high-dimensional state spaces, alongside the convergence dilemmas encountered in function optimization. The former challenge is attributed to the exponential growth in the number of states within extensive state spaces, which significantly escalates computational time and resource allocation, thereby complicating the identification of optimal policies within constrained temporal and spatial parameters. The latter challenge pertains to the non-convex optimization issues inherent in algorithmic exploration and decision-making processes. This complication arises from the prevalence of numerous local optima within the deep neural network’s parameter space, impeding the algorithm’s progression towards a global optimum due to entrapment in local optima during optimization phases.
It is crucial to acknowledge that these two predominant challenges are not mutually exclusive but are interlinked, necessitating concurrent resolution. Thus, addressing these issues collectively is paramount. This paper introduces a novel approach through the development of a flexible actor-critic algorithm, which leverages the maximum entropy framework, diverging from traditional DRL algorithms that solely prioritize maximizing long-term rewards. The Soft Actor-Critic (SAC) algorithm innovates by incorporating an action’s maximum entropy estimation into its action selection strategy, aiming to enhance decision-making robustness and algorithmic convergence. The adoption of a maximum entropy-based strategy for the objective function, as depicted in Equation 15, signifies a strategic pivot designed to mitigate the aforementioned challenges by promoting a more explorative and globally informed optimization process.
where α denotes the temperature control factor, which is used to regulate the importance of the entropy term
Incorporating the maximum entropy function into the Soft Actor-Critic (SAC) algorithm fundamentally alters the probabilistic landscape of action selection. This approach ensures a distribution mechanism that mitigates the likelihood of the agent persistently favoring actions with disproportionately high probabilities. The primary advantage of integrating the maximum entropy principle lies in its capacity to randomize the strategic optimization pathway. This randomness acts as a catalyst for enhanced exploration during the initial training phases, enabling the algorithm to evaluate and learn from a broader spectrum of action outcomes. Such a mechanism not only accelerates the training process by enriching the exploration domain but also prevents the convergence on suboptimal policies in later stages by discouraging repetitive action selection.
By diminishing the repetitive selection of identical actions, the strategy effectively minimizes the perturbation induced by noise, thereby streamlining the algorithm’s path to convergence. This reduction in noise influence is crucial for achieving a more stable and efficient learning trajectory. The strategic application of the maximum entropy function, therefore, plays a critical role in balancing exploration with exploitation, optimizing training velocity, and facilitating smoother algorithmic convergence by alleviating the impact of stochastic behaviors on the learning process.
In order to make the algorithm work in the continuous domain, the SAC algorithm sets up function approximators for the value function and the policy function that can assist the function to be updated for optimization. There are three main types of objective optimization functions in the SAC algorithm, namely, the state value function (
where
In order to avoid over-estimation of the Q values of certain actions by the action value function, the SGAC algorithm adopts the cropping dual Q value learning technique, i.e., it uses two identical Q networks, Q1 and Q2, and achieves the purpose of reducing the training bias and improving the stability and robustness of the algorithm by selecting the smaller of the largest Q values obtained from the Q1 and Q2 networks. In order to simplify the block diagram structure. For the current Q network, due to the deletion of the V network, the gradient update formula of the corresponding action value function
where
In the deep reinforcement learning-based LFC scenario studied in this paper, the training process of the recommended intelligences model can also be represented as a serialisation process based on a Markov decision process.
For the policy function of the system, the policy gradient algorithm is particularly suitable for long-term interactive recommendation scenarios because of its iterative optimization feature, which can gradually accumulate experience through the continuous interaction between the agent and the environment. In this paper, we use the classic algorithm in the policy gradient algorithm to optimise the policy, and the policy function of this algorithm is shown below.
Among them, B denotes the experience pool, which is used to store the current state, target state, action and the instant reward obtained after interacting with the environment of each recommending agent,
This study introduces an advanced policy gradient algorithm featuring a self-adjusting temperature control factor, designed to enhance system robustness against interference and to accelerate convergence rates. A distinctive aspect of this approach is the incorporation of an entropy-based action selection term into the policy objective function, as detailed in Equation 21. This modification aims to optimize the policy function’s performance by leveraging the value function for reward assessment, thereby minimizing bias throughout the training phase. The formulation of the actor component’s policy function within the SGAC algorithm is elucidated in Equation 22. This innovative mechanism facilitates a more dynamic adaptation process, significantly improving the algorithm’s efficiency in navigating complex environments and achieving optimal decision-making strategies.
where
Deep reinforcement learning algorithms based on the Actor-Critic architecture usually choose the state value function or action value function as an important basis for policy optimisation. In the SAC algorithm, the action value function, as a direct influence on the policy function update, is particularly important in the design. The algorithm adopted in this paper contains one actor network and four critic networks. The actor network is a strategy network, while the critic network contains two identical current action value networks and two identical target action value networks. The structure of the current action value network and the target action value network is basically the same, the only difference is that the parameter
As can be seen from Equation 23, the current action value function in the SGAC algorithm contains two terms related to the value of Q.
Since the SGAC algorithm deletes the state value network, the target state value is represented as a target action value term containing a decay factor and an entropy term containing a self-renewal temperature control factor. The parameter updating method of the target action value function is a flexible updating method based on the smoothing factor τ, and the specific updating formula is shown in Equation 25.
From Equation 25, it can be seen that the real action value function including the target state value term is a major innovation of the SGAC algorithm compared with the traditional Q-learning algorithm, i.e., the introduction of the operation term based on the entropy of the action selection is used to balance the relationship between exploration and exploitation, so as to improve the stability of the algorithm and the convergence speed. In addition, the current action value network and the target action value network in the SGAC algorithm contain two identical network structures, and the reward evaluation of the strategy function is performed by selecting a smaller Q value from the same network structure, which effectively reduces the training bias caused by overestimation.
The SGAC algorithm has a significant improvement over the original SAC algorithm in terms of updating the temperature control factors. This improvement is mainly reflected in the fact that the SGAC algorithm uses the constrained optimisation method to split the entropy term into the strategy entropy related to the strategy and the minimum entropy unrelated to the strategy. The self-renewal temperature control factor
Compared with the original SAC algorithm, the self-updating temperature control factor
The GRL algorithm proposed in this paper consists of graph convolutional neural network (GCN) and deep deterministic policy gradient (DDPG), which includes graph policy network and graph value network, its overall structure is shown in Figure 2. GRL algorithm consists of graph policy network and graph value network.
1) The input of the graph strategy network is the state diagram of an islanded microgrid considering the knowledge of strong nonlinear currents, which contains the adjacency matrix
2) The initial input of the graph value network is the same as that of the graph strategy network, the input of the first fully connected layer (FC) after the graph convolution layer is composed of the features output from the graph convolution layer and the actions generated by the graph strategy network, and the final output of the graph value network is a one-dimensional data, i.e., the value of Q of the actions applied in the environment state, which is used to evaluate the actions.
In the graph strategy network
where
Therefore, the rules for transferring feature information between layers of the GRL algorithm are shown in Equations 28, 29.
where
where
Within the ambit of this research, the efficacy of the DEO-LFC architecture, employing the SGAC algorithm, underwent a stringent assessment against a backdrop of a sophisticated CSG microgrid LFC paradigm, as explicated in the seminal work of (Yousef et al., 2014), with the deployment of parameters extrapolated from verifiable empirical datasets as expounded in (Bengiamin and Chan, 1982). The microgrid subject to this study operates at a nominal voltage of 10 kV and integrates a multifaceted energy portfolio, including a 1.04 MWp solar photovoltaic array, a 50 kW wind power installation, a 1220 kW diesel power generator, a 2000 kWh energy storage system, and a 300 kW facility for electric This eclectic mix of energy sources and storage capabilities facilitates a fluid and efficacious transition across various strata of grid integration, from micro-energy to energy storage. This eclectic mix of energy sources and storage capabilities facilitates a fluid and efficacious transition across various strata of grid integration, from micro-energy production and load management to the incorporation of renewable energy sources and the execution of energy control systems both locally and remotely.
The analytic scrutiny of the DEO-LFC model, predicated on the SGAC algorithm, was conducted in juxtaposition with a spectrum of alternative This encompassed DEO-LFC frameworks predicated on algorithms such as Soft Q-Learning (Mi et al., 2016), Proximal Policy Optimisation (PPO) (Chen et al., 1991), and the SGAC algorithm (Wang et al., 1993). Proximal Policy Optimisation (PPO) (Yan et al., 2022), Trust Region Policy Optimization (TRPO) (Mahboob Ul Hassan et al., 2022), Distributed Distributional Deterministic Policy Gradients (D4PG) (Yu et al., 2012), Asynchronous Actor-Critic Agents (A3C) (Yu et al., 2015), Twin Delayed Deep Deterministic Policy Gradient (TD3) (Shangguan et al., 2021), Deep Deterministic Policy Gradient (DDPG) (Li et al., 2021), Double Deep Q-Network (DDQN) (Chen et al., 2022), Deep Q-Network (DQN) (Zhang et al., 2023b), Distributed Model Predictive Control (DMPC) (Su et al., 2021), Model Predictive Control (MPC) (Hosseini and Etemadi, 2008), Fuzzy Fractional Order Proportional Integral (Fuzzy-FOPI) (Wang et al., 2013), Fuzzy Proportional Integral (Fuzzy-PI) (Chen et al., 2022), and Particle Swarm Optimisation Proportional Integral (PSO-PI) (Peng et al., 2023) for LFC purposes.
This extensive comparative review was meticulously designed to evaluate the DEO-LFC framework, undergirded by the SGAC algorithm, in terms of efficiency, reliability, and adaptability within the operational milieu of advanced microgrid systems. The evaluative criteria were centred on the system’s proficiency in maintaining voltage stability, enhancing the integration and The evaluative criteria were centred on the system’s proficiency in maintaining voltage stability, enhancing the integration and exploitation of renewable energy resources, securing The evaluative criteria were centred on the system’s proficiency in maintaining voltage stability, enhancing the integration and exploitation of renewable energy resources, securing dependable energy storage and retrieval mechanisms, and orchestrating efficacious load management protocols. Research aims to shed light on the transformative potential of cutting-edge deep learning algorithms in augmenting the operational efficiency of smart This, in turn, is envisaged to catalyze the evolution towards energy infrastructures that are not only more sustainable but also markedly more resilient.
In the present investigation, step disturbances were systematically introduced into the Case study to meticulously evaluate the system’s response and resilience under perturbed conditions. The outcomes of this experimental setup are comprehensively documented and presented through a series of visual representations and quantitative data analyses, spanning Figure 4. The outcomes of this experimental setup are comprehensively documented and presented through a series of visual representations and quantitative data analyses, spanning Figure 4 and including the detailed numerical results compiled in Table 1. This approach was deliberately chosen to facilitate a nuanced understanding of the system’s dynamics and its capability to maintain stability or adapt to sudden changes in operating conditions. This approach was deliberately chosen to facilitate a nuanced understanding of the system’s dynamics and its capability to maintain stability or adapt to sudden changes in operating conditions.
The inclusion of step disturbances serves as a critical methodological tool to simulate real-world operational challenges, providing insights into The inclusion of step disturbances serves as a critical methodological tool to simulate real-world operational challenges, providing insights into the system’s robustness and the efficacy of implemented control strategies. This structured presentation of findings, leveraging both graphical and This structured presentation of findings, leveraging both graphical and tabular formats, is designed to provide a comprehensive overview of the experimental results, fostering an in-depth analysis of the system’s response patterns. The empirical evidence gathered through this methodology is instrumental in validating the theoretical models and hypotheses posited in the study, thereby contributing to the advancement of the system. The empirical evidence gathered through this methodology is instrumental in validating the theoretical models and hypotheses posited in the study, thereby contributing to the advancement of knowledge in the field. Furthermore, the detailed exposition of results in this manner adheres to rigorous scientific communication standards, ensuring clarity, precision, and replicability of the research findings.
Table 1’s analysis provides a detailed comparison of the SGAC algorithm against other algorithmic models, focusing on frequency deviation and generation cost metrics. The findings reveal that the frequency deviations with other strategies were 1.089–4.155 times greater than with the SGAC algorithm. Additionally, the SGAC algorithm demonstrated a reduction in generation costs by 0.31%–1.28% over its counterparts, underscoring its superior efficiency and control in microgrid management. A deeper investigation into frequency response and diesel generator outputs across different control strategies highlights the variance in performance and the efficacy of each control mechanism. The SGAC algorithm emerges as the top performer, with soft Q-learning noted as a strong alternative.
The standout performance of both the SGAC and soft Q-learning algorithms is linked to their use of maximum entropy exploration mechanisms, enabling precise adjustments in learning rates and importance weighting through an updated experience-sharing framework. This adaptability allows for customized control strategies in various zones, enhancing operational flexibility. Particularly, the SGAC algorithm excels in making decisions based on dynamic joint trajectories and historical data, bypassing traditional policy evaluation methods and improving its responsiveness to learning adjustments.
The SGAC algorithm’s adaptability and control effectiveness across diverse system conditions firmly establish its role as a leader in the field of reinforcement learning, distinguished by its straightforward and universally applicable parameters. However, applying reinforcement learning broadly faces challenges, such as setting a shared exploration goal for multiple agents in complex tasks and dealing with the instability from agents needing to respond to each other.
The introduction of multi-agent reinforcement learning approaches, focusing on collective characteristics, marks a significant advancement in overcoming these challenges, steering reinforcement learning towards achieving dynamic tasks through autonomous decision-making and agent exploration.
Further examination of operational dynamics, as shown in Figure 3B, reveals the system’s ability to closely follow load disturbances, including negative and square wave perturbations. The LFC units’ power outputs adjust to address unpredictable power changes effectively. An in-depth look at the regulation curves of various LFC units in Figure 3A shows a strategic allocation based on regulation costs and disturbance types, leading to optimized frequency control. The uniform micro-increment rate principle guides power distribution among LFC units, resulting in an economically efficient power output, contrasting with other DRL models that lack refinement mechanisms and heavily rely on theoretical models, thus limiting their control accuracy.
In the research delineated within this document, a sophisticated smart distribution grid model, replete with a plethora of renewable energy sources, is meticulously constructed to facilitate an in-depth analysis of the SGAC algorithm’s operational performance amidst a highly stochastic environment. The model is enriched with an array of renewable energy sources, encompassing electric vehicles, wind turbines, small-scale hydropower plants, micro-gas turbines, fuel cells, photovoltaic systems, and other energy sources. The model is enriched with an array of renewable energy sources, encompassing electric vehicles, wind turbines, small-scale hydropower plants, micro gas turbines, fuel cells, photovoltaic systems, and biomass energy solutions. Notably, certain renewable energy sources such as electric vehicles, wind power, and photovoltaic power generation exhibit considerable variability and unpredictability in their output. Consequently, these sources are modelled as sources of random load disturbances, their power outputs being incorporated into the system without contributing to the frequency Consequently, these sources are modelled as sources of random load disturbances, their power outputs being incorporated into the system without contributing to the frequency regulation mechanisms.
The variability inherent in wind power generation is captured through the application of finite bandwidth white noise as the input signal for the wind turbine model, thereby replicating the stochastic nature of wind speeds. The variability inherent in wind power generation is captured through the application of finite bandwidth white noise as the input signal for the wind turbine model, thereby replicating the stochastic nature of wind speeds. Similarly, the active power output for the photovoltaic generation model is derived by emulating the diurnal variations in solar irradiance. Characteristic of these renewable energy sources, thereby providing a robust framework for evaluating the efficacy of the SGAC algorithm under conditions that closely mimic real-world operational challenges.
Comprehensive details pertaining to the specifications and operational parameters of each energy unit incorporated within this model can be found in This extensive cataloguing of parameters is instrumental in underpinning the simulation exercises with a high degree of accuracy and relevance. This extensive cataloguing of parameters is instrumental in underpinning the simulation exercises with a high degree of accuracy and relevance, ensuring that the insights gleaned from this study are both valid and applicable to the design. This extensive cataloguing of parameters is instrumental in underpinning the simulation exercises with a high degree of accuracy and relevance, ensuring that the insights gleaned from this study are both valid and applicable to the design and optimization of future smart distribution grids. Through this meticulously constructed model, the paper aims to shed light on the adaptability and control capabilities of the SGAC algorithm, particularly in managing the complexities introduced by the SGAC algorithm. Particularly in managing the complexities introduced by the integration of a diverse mix of renewable energy sources within smart grid infrastructures.
This paper presents a detailed examination of the integration of random white noise as a proxy for load disturbances within a sophisticated smart distribution network model. This model is intentionally crafted to mimic the erratic load fluctuations commonly observed in power systems that are extensively integrated with novel energy resources. The primary objective of this research is to conduct a comprehensive evaluation of the Soft Graph Actor Critic (SGAC)’s efficacy and resilience when faced with environments characterized by substantial stochastic disturbances. A key component of this investigation involves conducting simulations that introduce 24-h cycles of random white noise disturbances, thereby allowing for an in-depth analysis of the SGAC algorithm’s robustness and long-term operational integrity under scenarios of severe random load fluctuations.
Figure 4 delineates the proficiency of the SGAC algorithm in accurately and promptly tracking random disturbances, thereby highlighting its precision in real-time disturbance management. The outcomes of these simulations, systematically compiled in Table 2, offer a quantitative assessment of the generation costs incurred, representing a cumulative analysis of the total regulatory expenses accumulated by all generating units over a 24-h period. A comparative evaluation reveals that the frequency deviation experienced with alternative control algorithms ranges from 1.388 to 3.711 times higher than that encountered when employing the SGAC algorithm. Moreover, the generation costs associated with the SGAC algorithm exhibit a nominal decrease, spanning from 0.0006% to 0.019%. These statistics underscore the SGAC algorithm’s superior economic efficiency, advanced self-adaptive capabilities, and its distinguished performance in executing coordinated optimal control compared to other intelligent algorithms.
To further substantiate the SGAC algorithm’s performance efficacy, an assortment of disturbances, including step waves, square waves, and random waves, were systematically introduced into the system. The resulting data underscore the SGAC’s exceptional convergence properties and its elevated learning efficiency, underscoring its unmatched adaptability and robustness within stochastic operational environments. The algorithm’s prowess in attenuating random disturbances and enhancing dynamic control effectiveness across interconnected grid landscapes is emphatically validated through these findings.
Figure 4 provides insight into how the output power from various units aligns with load demand across a 24-h cycle, showcasing the system’s adeptness at matching load fluctuations and achieving optimal operational states through the synchronized management of multiple energy sources under a cohesive power command strategy. The Energy Storage System (ESS) is identified as a pivotal element, demonstrating its capability to swiftly and accurately modulate power output, thereby contributing significantly to the balance of supply and demand through its flexible charging and discharging functionalities. The real-time optimization conducted by the system controller enables a smoother and more stable regulation process, facilitating rapid and efficient cooperative responses to abrupt load changes within the power system. This, in turn, validates the SGAC algorithm’s capacity to support swift and optimal cooperative operations amidst fluctuating system conditions, thereby enhancing the overall operational efficiency and reliability of the power system in managing dynamic and unpredictable environments.
In conclusion, this study presents a comprehensive evaluation of the integration challenges posed by emerging energy sources within electrical grids, specifically highlighting the resultant variability that complicates traditional Load Frequency Control (LFC) mechanisms. Specifically highlighting the resultant variability that complicates traditional LFC mechanisms. The key findings of this research can be summarised as follows. The key findings of this research can be summarised as follows.
Challenge Identification: This study highlights the complexities introduced by renewable energy integration into electrical grids, specifically the variability leading to frequency fluctuations and increased generation costs.
Innovative Approach: Introduction of the Data-Enhanced Optimum Load Frequency Control (DEO-LFC) approach and the Soft Graph Actor Critic (SGAC) algorithm, utilising deep reinforcement learning and graph sequence neural networks for adaptive frequency regulation. Algorithm, utilising deep reinforcement learning and graph sequence neural networks for adaptive frequency regulation.
Methodological Shift: Transition from traditional control mechanisms to agent-based frameworks within DEO-LFC, aiming to enhance grid stability and optimise generation costs amidst high renewable energy penetration.
Validation and Impact: Application of DEO-LFC to the China Southern Grid’s isolated island city microgrid model, showcasing its effectiveness in managing grid stability and reducing generation costs in environments with substantial renewable energy sources.
The study underscores the importance of advanced LFC strategies and algorithmic innovations for addressing the challenges of renewable energy integration into electrical grids, offering a pathway towards more stable and cost-efficient grid operations. The study underscores the importance of advanced LFC strategies and algorithmic innovations for addressing the challenges of renewable energy integration into electrical grids, offering a pathway towards more stable and cost-efficient grid operations.
Our future work will enhance the robustness of the algorithm and apply it to the power grid.
The author(s) declared no potential conflicts of interest with respect to the research, authorship, and/or publication of this article.
The original contributions presented in the study are included in the article/Supplementary material, further inquiries can be directed to the corresponding author.
MW: Writing–original draft, Writing–review and editing. DM: Writing–original draft, Writing–review and editing. KX: Writing–original draft, Writing–review and editing. LY: Writing–original draft, Writing–review and editing.
The author(s) declare that financial support was received for the research, authorship, and/or publication of this article. This work was supported by Science and Technology Project of China Southern Power Grid Corporation, under Grant No. GDKJXM20220183.
Authors MW, KX, and LY were employed by Dongfang Electronics Corporation. Author DM was employed by Guangzhou Power Supply Bureau of Guangdong Power Grid Co., Ltd.
All claims expressed in this article are solely those of the authors and do not necessarily represent those of their affiliated organizations, or those of the publisher, the editors and the reviewers. Any product that may be evaluated in this article, or claim that may be made by its manufacturer, is not guaranteed or endorsed by the publisher.
Bengiamin, N. N., and Chan, W. C. (1982). Variable structure control of electric power generation. IEEE Trans. Power Appar. Syst. PAS-101, 376–380. doi:10.1109/TPAS.1982.317117
Chen, Y. H., Leitmann, G., and Kai, X. Z. (1991). Robust control design for interconnected systems with time-varying uncertainties. Int. J. Control 54, 1119–1142. doi:10.1080/00207179108934201
Chen, Z., Zhu, J., Li, S., Liu, Y., and Luo, T. (2022). Detection of false data injection attacks on load frequency control system with renewable energy based on fuzzy logic and neural networks. J. Mod. Power Syst. Clean. Energy 10, 1576–1587. doi:10.35833/MPCE.2021.000546
Hosseini, S. H., and Etemadi, A. H. (2008). Adaptive neuro-fuzzy inference system based automatic generation control. Electr. Power Syst. Res. 78, 1230–1239. doi:10.1016/j.epsr.2007.10.007
Huang, T., and Lv, X. (2023). Load frequency control of power system based on improved AFSA-PSO event-triggering scheme. Front. Energy Res. 11, 1235467. doi:10.3389/fenrg.2023.1235467
Jia, Y., Dong, Z. Y., Sun, C., and Meng, K. (2019). Cooperation-based distributed economic MPC for economic load dispatch and load frequency control of interconnected power systems. IEEE Trans. Power Syst. 34, 3964–3966. doi:10.1109/TPWRS.2019.2917632
Li, J., Yu, T., Zhang, X., Li, F., Lin, D., and Zhu, H. (2021). Efficient experience replay based deep deterministic policy gradient for AGC dispatch in integrated energy system. Appl. Energy 285, 116386. doi:10.1016/j.apenergy.2020.116386
Long, B., Liao, Y., Chong, K. T., Rodríguez, J., and Guerrero, J. M. (2021). Enhancement of frequency regulation in AC microgrid: a fuzzy-MPC controlled virtual synchronous generator. IEEE Trans. Smart Grid 12, 3138–3149. doi:10.1109/TSG.2021.3060780
Mahboob Ul Hassan, S., Ramli, M. A. M., and Milyani, A. H. (2022). Robust load frequency control of hybrid solar power systems using optimization techniques. Front. Energy Res. 10, 902776. doi:10.3389/fenrg.2022.902776
Mi, Y., Fu, Y., Li, D., Wang, C., Loh, P. C., and Wang, P. (2016). The sliding mode load frequency control for hybrid power system based on disturbance observer. Int. J. Electr. Power Energy Syst. 74, 446–452. doi:10.1016/j.ijepes.2015.07.014
Mi, Y., Fu, Y., Wang, C., and Wang, P. (2013). Decentralized sliding mode load frequency control for multi-area power systems. IEEE Trans. Power Syst. 28, 4301–4309. doi:10.1109/TPWRS.2013.2277131
Peng, B., Ma, X., Ma, X., Tian, C., and Sun, Y. (2023). Coordinated AGC control strategy for an interconnected multi-source power system based on distributed model predictive control algorithm. Front. Energy Res. 10, 1019464. doi:10.3389/fenrg.2022.1019464
Shangguan, X. C., Zhang, C. K., He, Y., Jin, L., Jiang, L., Spencer, J. W., et al. (2021). Robust load frequency control for power system considering transmission delay and sampling period. IEEE Trans. Ind. Inf. 17, 5292–5303. doi:10.1109/TII.2020.3026336
Su, K., Li, Y., Chen, J., and Duan, W. (2021). Optimization and H∞ performance analysis for load frequency control of power systems with time-varying delays. Front. Energy Res. 9, 762480. doi:10.3389/fenrg.2021.762480
Wang, Y., Delille, G., Bayem, H., Guillaud, X., and Francois, B. (2013). High wind power penetration in isolated power systems—assessment of wind inertial and primary frequency responses. IEEE Trans. Power Syst. 28, 2412–2420. doi:10.1109/TPWRS.2013.2240466
Wang, Y., Zhou, R., and Wen, C. (1993). Robust load-frequency controller design for power systems. IEE Proc. C-Generation, Transm. Distribution 140, 11–16. doi:10.1049/ip-c.1993.0003
Wang, Y., Zhou, R., and Wen, C. (1994). New robust adaptive load-frequency control with system parametric uncertainties. IEE Gener. Transm. Dis. 141, 184–190. doi:10.1049/ip-gtd:19949757
Xi, L., Li, Y., Huang, Y., Lu, L., and Chen, J. (2018). A novel automatic generation control method based on the ecological population cooperative control for the islanded smart grid. Complexity 2018, 1–17. doi:10.1155/2018/2456963
Xi, L., Wu, J., Xu, Y., and Sun, H. (2021). Automatic generation control based on multiple neural networks with actor-critic strategy. IEEE Trans. Neural Netw. Learn. Syst. 32, 2483–2493. doi:10.1109/TNNLS.2020.3006080
Xi, L., Yu, T., Yang, B., Zhang, X., and Qiu, X. (2016a). A wolf pack hunting strategy based virtual tribes control for automatic generation control of smart grid. Appl. Energy 178, 198–211. doi:10.1016/j.apenergy.2016.06.041
Xi, L., Zhang, L., Xu, Y., Wang, S., and Yang, C. (2022). Automatic generation control based on multiple-step greedy attribute and multiple-level allocation strategy. CSEE J. Power Energy Syst. 8, 281–292. doi:10.17775/CSEEJPES.2020.02650
Xi, L., Zhang, Z., Yang, B., Huang, L., and Yu, T. (2016b). Wolf pack hunting strategy for automatic generation control of an islanding smart distribution network. Energy Convers. manage. 122, 10–24. doi:10.1016/j.enconman.2016.05.039
Yan, C. H., Liu, B., Xiao, P., and Zhang, C. (2022). Stabilization of load frequency control system via event-triggered intermittent control. IEEE T. Circuits-II 69, 4934–4938. doi:10.1109/TCSII.2022.3197460
Yousef, H. A., Kharusi, K. A.-, Albadi, M. H., and Hosseinzadeh, N. (2014). Load frequency control of a multi-area power system: an adaptive fuzzy logic approach. IEEE Trans. Power Syst. 29, 1822–1830. doi:10.1109/TPWRS.2013.2297432
Yu, T., Wang, H. Z., Zhou, B., Chan, K. W., and Tang, J. (2015). Multi-agent correlated equilibrium Q(λ) learning for coordinated smart generation control of interconnected power grids. IEEE Trans. Power Syst. 30, 1669–1679. doi:10.1109/TPWRS.2014.2357079
Yu, T., Wang, Y. M., Ye, W. J., Zhou, B., and Chan, K. W. (2011). Stochastic optimal generation command dispatch based on improved hierarchical reinforcement learning approach. IET Gener. Transm. Dis. 5, 789–797. doi:10.1049/iet-gtd.2010.0600
Yu, T., Zhou, B., Chan, K. W., Yuan, Y., Yang, B., and Wu, Q. H. (2012). R (λ) imitation learning for automatic generation control of interconnected power grids. Automatica 48, 2130–2136. doi:10.1016/j.automatica.2012.05.043
Zhang, G., Li, J., Bamisile, O., Xing, Y., Cai, D., and Huang, Q. (2023a). An ${H_\infty }$ load frequency control scheme for multi-area power system under cyber-attacks and time-varying delays. IEEE Trans. Power Syst. 38, 1336–1349. doi:10.1109/TPWRS.2022.3171101
Zhang, M., Dong, S., Wu, Z. G., Chen, G., and Guan, X. (2023b). Reliable event-triggered load frequency control of uncertain multiarea power systems with actuator failures. IEEE Trans. Autom. Sci. Eng. 20, 2516–2526. doi:10.1109/TASE.2022.3205176
Zhang, X., Tan, T., Zhou, B., Yu, T., Yang, B., and Huang, X. (2021). Adaptive distributed auction-based algorithm for optimal mileage based AGC dispatch with high participation of renewable energy. Int. J. Electr. Power Energy Syst. 124, 106371. doi:10.1016/j.ijepes.2020.106371
Keywords: load frequency control, deep graph reinforcement learning, isolated island city microgrid, soft graph actor critic, data-enhanced
Citation: Wu M, Ma D, Xiong K and Yuan L (2025) Optimizing load frequency control in isolated island city microgrids: a deep graph reinforcement learning approach with data enhancement across extensive scenarios. Front. Energy Res. 12:1384995. doi: 10.3389/fenrg.2024.1384995
Received: 11 February 2024; Accepted: 15 April 2024;
Published: 17 February 2025.
Edited by:
Yunqi Wang, Monash University, AustraliaReviewed by:
Cheng Yang, Shanghai University of Electric Power, ChinaCopyright © 2025 Wu, Ma, Xiong and Yuan. This is an open-access article distributed under the terms of the Creative Commons Attribution License (CC BY). The use, distribution or reproduction in other forums is permitted, provided the original author(s) and the copyright owner(s) are credited and that the original publication in this journal is cited, in accordance with accepted academic practice. No use, distribution or reproduction is permitted which does not comply with these terms.
*Correspondence: Dakui Ma, bWFkYWt1aV9jc2dAZ2Rjc2cuY29t
Disclaimer: All claims expressed in this article are solely those of the authors and do not necessarily represent those of their affiliated organizations, or those of the publisher, the editors and the reviewers. Any product that may be evaluated in this article or claim that may be made by its manufacturer is not guaranteed or endorsed by the publisher.
Research integrity at Frontiers
Learn more about the work of our research integrity team to safeguard the quality of each article we publish.