- Oak Ridge National Laboratory, Oak Ridge, TN, United States
Advanced manufacturing techniques and digital twin technology are rapidly transforming the nuclear industry, offering the potential to enhance productivity, safety, and cost-effectiveness. Customized parts are being produced using additive manufacturing, automation, and robotics, while digital twin technology enables the virtual modeling and optimization of complex systems. These advanced technologies can significantly improve operational efficiency, predict system behavior, and optimize maintenance schedules in the nuclear energy sector, leading to heightened safety and reduced downtime. However, the nuclear industry demands the highest levels of safety and security, as well as intricate manufacturing processes and operations. Thus, challenges such as data management and cybersecurity must be addressed to fully realize the potential of advanced manufacturing techniques and digital twin technology in the nuclear industry. This comprehensive review highlights the critical role of digital twin technology with advanced manufacturing toward nuclear energy to improve performance, minimize downtime, and heighten safety, ultimately contributing to the global energy mix by providing dependable and low-carbon electricity.
1 Introduction
Nuclear energy is a critical part of the world’s energy mix, providing reliable, low-carbon electricity (Lenzen, 2008; Shirizadeh and Quirion, 2021). However, the production of nuclear energy is complex and requires a high level of safety and security to prevent accidents and the release of radioactive materials (Hyvärinen et al., 2022). Advanced manufacturing and digital twin technologies offer new opportunities to improve the efficiency, reliability, and safety of nuclear energy production (Grieves, 2015; Kochunas and Huan, 2021; Pal et al., 2022). To meet the industry’s evolving needs, advanced manufacturing techniques and digital twin technology have emerged as transformative tools (Ostaševičius, 2022; Sleiti et al., 2022; Attaran et al., 2023). This section provides an overview of the significance and potential of these technologies in the context of nuclear energy. Advanced manufacturing in the nuclear industry uses cutting-edge technologies and processes to enhance the production, assembly, and maintenance of nuclear components. Key elements include additive manufacturing (3D printing), robotics, automation, advanced materials, digitalization, and stringent quality control measures. These elements work together to improve efficiency, precision, and safety in nuclear manufacturing. Figure 1 shows the key components involved in the nuclear energy sector.
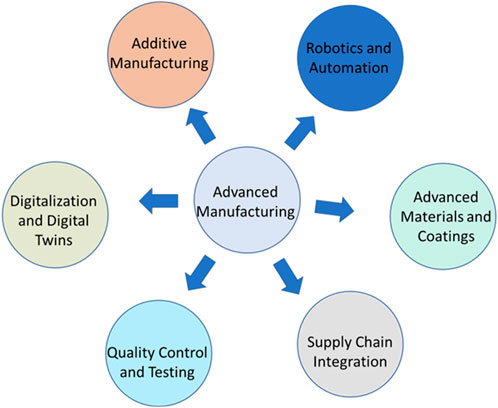
FIGURE 1. Components and elements integral to advanced manufacturing in the nuclear energy industry.
Advanced manufacturing techniques such as additive manufacturing, automation, and robotics can be used to produce complex nuclear components with reduced material waste, shorter lead times, and lower costs (Betzler et al., 2019; Balbaud et al., 2021; Li et al., 2022). For instance, additive manufacturing can be used to produce complex nuclear components with a high degree of accuracy and precision, thus reducing the risk of errors and defects that can compromise the safety of the nuclear energy systems (Lu et al., 2020; Van Rooyen and Morrell, 2020; Sun et al., 2021).
Furthermore, automation and robotics can be used to improve the speed and accuracy of manufacturing processes, resulting in increased production efficiency and lower costs (De Backer and DeStefano, 2021; Javaid et al., 2021). Additionally, they can be used to perform tasks that are hazardous or difficult for human operators, such as inspecting and maintaining the nuclear power plants (Moore, 1985). Digital twin technology provides a virtual replica of a physical system that enables real-time monitoring and control of the system’s performance (Haag and Anderl, 2018; Tao et al., 2018). Figure 2 shows the technological contributions to digital twin. This technology can be integrated with advanced manufacturing techniques to create a complete digital model of a nuclear energy system, including its physical components and their behavior (Zhang et al., 2020; Niu and Qin, 2021). For example, one can integrate sensor data with artificial intelligence, enabling the specialist care of different stages in additive manufacturing for the creation of designs or components. This digital model can be used to simulate different scenarios, test new designs, and optimize nuclear energy systems for improved performance, reduced downtime, and enhanced safety (Volodin and Tolokonskii, 2019; Singh et al., 2022; Song et al., 2022).
Advanced manufacturing and digital twin technologies have enormous potential to revolutionize the nuclear energy industry by improving the efficiency, reliability, and safety of nuclear energy production (Mandolla et al., 2019; Evangeline and Anandhakumar, 2020; Bartsch et al., 2021; Liu et al., 2022; Pantelidakis et al., 2022; Phua et al., 2022; Mu et al., 2023). This review provides an overview of the current state of these technologies and their potential applications and future scope in the nuclear energy industry.
2 Advanced manufacturing techniques
Additive manufacturing, automation, and robotics are revolutionizing the production of customized parts in the nuclear sector (French et al., 2020; Revuelta et al., 2021). This section investigates into the specific applications of these techniques, highlighting their benefits in terms of flexibility, cost-efficiency, and accelerated production cycles. It also discusses the challenges associated with adopting advanced manufacturing techniques in the nuclear industry and provides insights into how these challenges can be addressed.
2.1 Advanced manufacturing methods
Advanced manufacturing techniques, including additive manufacturing, automation, and robotics, have significant applications in the nuclear industry, revolutionizing the production of complex nuclear components (Patel and Kilic, 2021). Additive manufacturing, commonly known as 3D printing, offers numerous advantages when producing intricate parts with high precision and accuracy (Ngo et al., 2018). One of its key advantages lie in its capacity to create complex internal features with high tortuosity, minimizing waste. This process allows for rapid prototyping without the need for extensive retooling. Additionally, AM provides the flexibility to fabricate many components using a shared feedstock. Moreover, it can incorporate multiple materials seamlessly. This exclusive approach builds components layer by layer, a stark contrast to traditional manufacturing methods that entail extensive material removal from large stock blocks. By building components layer by layer, additive manufacturing reduces material waste, minimizes the risk of errors, and enhances the overall safety of nuclear energy systems.
Automation and robotics play crucial roles in streamlining manufacturing processes within the nuclear industry (Iqbal et al., 2012). These technologies enable the automation of repetitive tasks, leading to increased production speed, improved accuracy, and reduced costs. By employing robots in tasks such as welding, inspection, and material handling, the nuclear industry can achieve consistent quality and adhere to stringent safety standards (Savall et al., 1999). Automation and robotics also contribute to enhanced productivity and reduced labor requirements, resulting in cost savings (Schneider, 2014).
Moreover, advanced manufacturing techniques make it possible to perform tasks that are hazardous or challenging for human operators in nuclear power plants (Arinez et al., 2020). Robots equipped with specialized sensors and tools can efficiently inspect and maintain nuclear facilities, reducing the exposure of workers to radiation and other risks. Automation and robotics enhance safety measures and ensure the proper functioning of nuclear energy systems by performing critical tasks with precision and reliability (Domning et al., 1991).
By incorporating advanced manufacturing techniques such as additive manufacturing, automation, and robotics into the nuclear industry, manufacturers can benefit from reduced material waste, shorter lead times, improved production efficiency, and cost savings. These technologies not only enhance the manufacturing processes, but they also contribute to the overall safety and performance of nuclear energy systems by minimizing human errors and risks associated with manual operations.
Figure 3 shows the intricacies of advanced manufacturing techniques frequently used across diverse industries, including the nuclear sector. This comprehensive overview encompasses the essential details and characteristics of these advanced manufacturing methods, showcasing their widespread application and significance in various industrial domains.
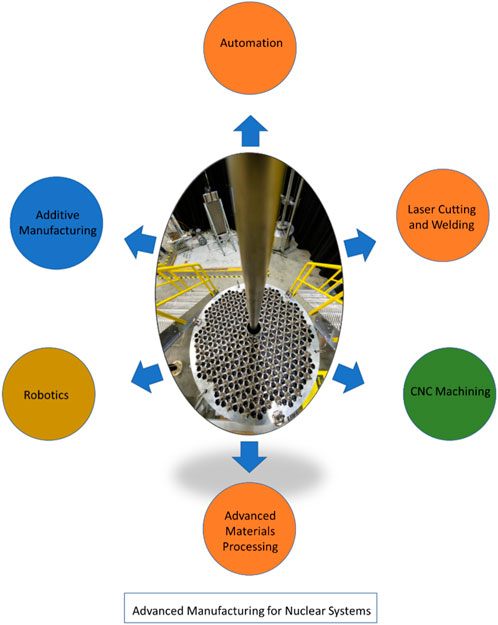
FIGURE 3. Advanced manufacturing techniques for nuclear industries. CNC: computer numerical control. Credit: ORNL.
2.1.1 Additive manufacturing or 3D printing
Additive manufacturing involves building 3D objects layer by layer using digital design data (Huang et al., 2015). It offers significant advantages when producing complex shapes, customizing components, and reducing material waste (Holshouser et al., 2013). Additive manufacturing can produce intricate parts with high precision and accuracy, offering design flexibility that traditional manufacturing methods often cannot achieve (Love, 2015).
Additive manufacturing encompasses various processes and uses various materials, including wires and powders of different sizes (Love et al., 2015). Accounting for environmental factors during the additive process necessitates numerous models because of the many possible combinations (Bourhis et al., 2013). Collecting and verifying the substantial amount of data required to train and validate these models becomes a formidable task. A comprehensive database of temperature-dependent thermophysical properties of commonly used engineering alloys would mitigate the computational burden. This database would streamline the modeling process and reduce the reliance on extensive data collection efforts. Figure 4 illustrates different types of metal additive manufacturing processes such as directed energy deposition (Svetlizky et al., 2021) and powder bed fusion (Bhavar et al., 2017; Kayacan et al., 2019), each employing distinct feedstock (powder or wire) and heat sources (laser, plasma, electron beam, or gas metal arc).
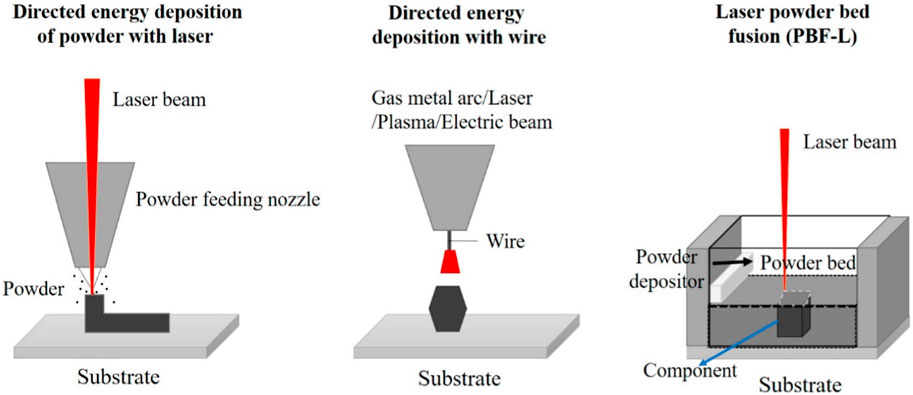
FIGURE 4. Primary processes of additive manufacturing. Reprinted with permission from (Zhang et al., 2020). Copyright 2020, Molle et al., licensed under MDPI, Basel, Switzerland.
For each commonly used additive manufacturing process, establishing standardized models under various conditions (including different materials, protective gases, and machines) is crucial (Bonnard et al., 2018; Bonnard et al., 2019a; Bonnard et al., 2019b). These models could then be employed to analyze parameter variations and environmental influences. Users would only be required to input relevant data to obtain a real-time digital twin of the additive manufacturing process. However, significant progress is still required to achieve this goal. Overcoming the challenges related to data acquisition, model validation, and standardization is essential for successfully implementing digital twins in additive manufacturing.
In the nuclear industry, additive manufacturing can be used to fabricate components with optimized designs, improved cooling capabilities, and enhanced performance. These components include nuclear cores, turbine blades, heat exchangers, and fuel assemblies (Watkins et al., 2013; Terrani et al., 2015; Hehr et al., 2017; Betzler et al., 2019; Betzler et al., 2019; Simpson et al., 2019). Figure 5 shows an example of additive manufacturing for nuclear applications; the components were printed at Oak Ridge National Laboratory (ORNL), which operates under the US Department of Energy (Jackson et al., 2016; Scime et al., 2020; Scime et al., 2021). These components were produced using AM, allowing the creation of complicated designs with features such as tube wall cooling channels and irregular geometry that flawlessly align with the specific necessities of the design, showcasing the outstanding versatility and precision of this technology.
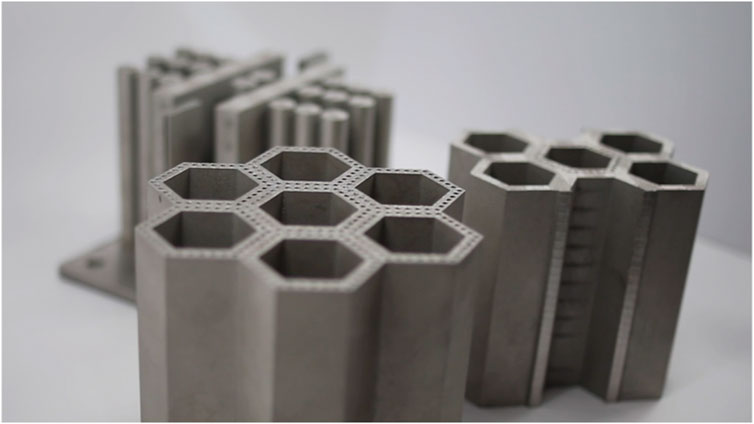
FIGURE 5. Nuclear components produced through additive manufacturing. Credit: ORNL (4 Major Opportunities for Additive Manufacturing in Nuclear Energy, 2019).
As part of the Transformational Challenge Reactor demonstration program, ORNL developed a prototype reactor core using 3D printing technology. This initiative aimed to address the challenges of high costs and lengthy deployment timelines that posed a threat to the future of nuclear energy. ORNL was entrusted to explore innovative solutions within the program to transform the landscape of nuclear energy production. Figure 6 displays 3D printed components for the prototype nuclear reactor.
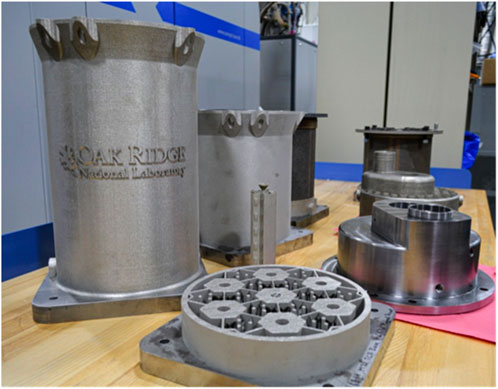
FIGURE 6. 3D printed components for the prototype reactor. Credit: B. Cramer, ORNL (Oak Ridge developing 3D-printed nuclear reactor core, 2020).
Figure 7 depicts a 3D printed fuel element showcased at ORNL (DANIEL, 2020). This exemplary fuel element is distinguished by its double-walled cladding and strategically integrated cooling channels, which incorporate helical guides. This intricate design enhances the surface area of the fuel element, leading to improved performance and functionality in its intended application.
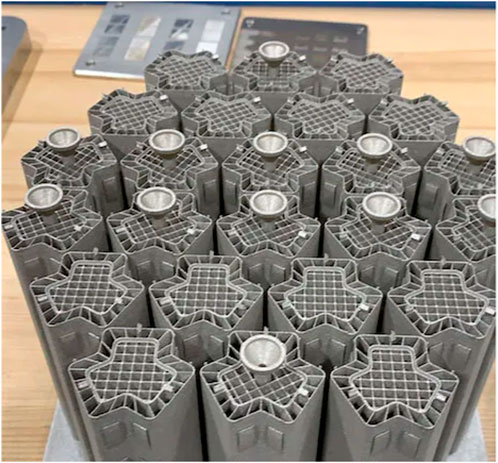
FIGURE 7. A fuel element featuring double-walled cladding and cooling channels that incorporate helical guides designed to enhance surface area. Credit: R. Dehoff, ORNL (DANIEL, 2020).
2.1.2 Automation
Automation involves using technology and machines to perform tasks with minimal or no human intervention (Muir, 1994; Kaber and Endsley, 2004; Nof, 2009). In advanced manufacturing, automation is a key factor in improving production efficiency, consistency, and quality (Rajawat et al., 2021). Robotic systems and automated machinery can perform repetitive tasks such as assembly, welding, material handling, and inspection with high accuracy and speed (Sandler, 1999). Automation not only increases productivity, but also reduces the risk of errors and enhances workplace safety (Crooks et al., 1994; Teizer et al., 2013; Dixit et al., 2019). In the nuclear industry, automation can help streamline manufacturing processes, optimize workflows, and ensure consistent quality control (Nelson and Haney, 1993; Crooks et al., 1994).
2.1.3 Robotics
Robotics refers to using mechanical devices or robots to perform tasks autonomously or under the control of human operators (Kofman et al., 2005; Losey et al., 2018). Robots are programmable machines that can carry out a wide range of tasks with precision and accuracy (Singh et al., 2013). In advanced manufacturing, robots are commonly used for tasks such as welding, printing, cutting, material handling, assembly, and inspection (Ding et al., 2015a; Djuric et al., 2016). They can operate in hazardous or challenging environments, reducing the risk to human operators (Meisel et al., 2022). In the nuclear industry, robots play a crucial role in handling hazardous or radioactive materials, performing maintenance tasks in radioactive environments, and supporting decommissioning activities (Sundar et al., 2012; Tsitsimpelis et al., 2019). In the field of advanced manufacturing for nuclear and aerospace industries, the adoption of robots for the production and 3D printing of components and parts has begun (French et al., 2019; Yuan et al., 2020; French et al., 2023). Utilizing simulated environments based on Digital Twins can assist in conducting needs assessments, refining route planning, and effectively preventing collisions among multiple robotic units engaged in concurrent operations on a shared workpiece.
2.1.4 Computer numerical control machining
Computer numerical control (CNC) machining involves using computer-controlled machines to shape and fabricate components from various materials (Rahmatullah et al., 2021). It uses computer-aided design (CAD) models to guide cutting, milling, drilling, and turning processes (Dubovska et al., 2014). CNC machines offer high precision and repeatability, enabling the production of complex and precise parts with tight tolerances (Jywe et al., 2012). CNC machining is vital to the nuclear industry for manufacturing precision components with high accuracy and quality. It is extensively used in fabricating critical parts such as reactor components, valves, and control system components, meeting stringent quality requirements. CNC machining ensures precise dimensions, intricate geometries, and smooth finishes for these components. It is also used to produce and maintain nuclear fuel elements such as fuel rods and spacers, meeting strict specifications with consistent precision (Wang et al., 2009; Hehr et al., 2017). Additionally, CNC machining is used to create customized tooling and equipment for nuclear research and development, enabling the production of fixtures, jigs, and prototypes for various applications. Overall, CNC machining contributes to the safety, reliability, and sustainability of nuclear power plants by delivering high-quality components with tight tolerances.
2.1.5 Laser cutting and welding
Laser cutting and welding use high-powered lasers to precisely cut or join materials together (Schuocker, 1989; Powell, 1993; Katayama, 2013). Laser cutting uses a focused laser beam to selectively remove material, thus enabling precise and intricate cuts (Yilbas, 2001). Additionally, with lower laser power, laser cutting can modify surface properties with precision (Mondal et al., 2020b). However, laser welding uses the heat generated by the laser beam to fuse materials (Sun and Ion, 1995; Zhang et al., 2020). These techniques offer high precision, minimal heat-affected zones, and reduced distortion, making them ideal for manufacturing nuclear components with complex geometries and tight tolerances.
Laser cutting and laser welding are integral aspects in various applications across the nuclear industry (Jain et al., 2010; Lopez et al., 2017; Francis et al., 2019). Laser cutting enables precise and intricate material removal, allowing for the creation of components with complex geometries. It is extensively used in nuclear power plants for cutting and shaping metals and alloys, thus facilitating the production of essential parts such as pressure vessels, piping systems, and fuel assemblies (Kirk et al., 2018). With advantages such as high precision, minimal heat-affected zones, and the ability to tune surface properties, laser cutting proves invaluable for manufacturing and maintenance operations in nuclear facilities (Tamura et al., 2016). However, laser welding is widely employed for joining and repairing components within nuclear power plants (Feng et al., 2016) and constructing and maintaining pressure vessels, piping systems, and containment structures. Laser welding offers precise control, minimal distortion, and reduced heat input, all of which are vital in ensuring the integrity and safety of nuclear facilities. Its ability to create high-quality hermetic seals in containment systems safeguards radioactive materials while supporting fabrication of nuclear fuel elements and assemblies. Laser welding’s precise and reliable welding capabilities are essential for maintaining fuel performance and safety. Both laser cutting and laser welding technologies greatly contribute to the efficient and effective operation of nuclear facilities, enabling manufacturing, maintenance, and repair processes while upholding high quality and safety standards.
2.1.6 Advanced materials processing
Advanced manufacturing techniques also include various processes for fabricating and modifying advanced materials (Mamalis, 2005). These processes include powder metallurgy, in which metal powders are compacted and sintered to produce components with superior mechanical properties (Panda et al., 2018). Additionally, laser cladding (Zhu et al., 2021) and thermal spraying techniques (Amin and Panchal, 2016) are used to deposit protective coatings onto surfaces, providing enhanced wear resistance, corrosion protection, or radiation shielding properties. These advanced materials processing techniques enable the production of materials with improved performance and durability, specifically tailored for nuclear applications.
These advanced manufacturing and engineering techniques offer numerous benefits, such as increased design flexibility, improved production efficiency, reduced material waste, and enhanced quality control (Mamalis, 2005). By leveraging these techniques, the nuclear industry can achieve higher productivity, improved safety, and the fabrication of customized components that meet the specific requirements of nuclear energy systems (Lou and Gandy, 2019). Advanced manufacturing and materials processing techniques are facilitating the exploration of an accelerated approach to constructing a high-performance nuclear system (Akinlabi et al., 2016). For example, ORNL progressed toward its goal of constructing a full-sized 3D printed nuclear reactor by 2023 (Oak Ridge developing 3D-printed nuclear reactor core, 2020).
2.2 Materials for advanced manufacturing
Materials for advanced manufacturing in the nuclear industry are critical components that enable the development and production of innovative technologies and processes (Babu et al., 2015). These materials are specifically engineered to meet the stringent requirements of nuclear applications, including resistance to radiation, extreme temperatures, corrosion, and mechanical stress (Zinkle and Was, 2013; Was et al., 2019). They encompass a wide range of materials, such as metals (Gorse-Pomonti and Russier, 2007; Harper et al., 2015), advanced alloys (Pickering et al., 2021; Beausoleil et al., 2022; Moschetti et al., 2022), ceramics (Marra, 2011; Cramer et al., 2022), composites (Hu et al., 2008; Kohyama and Kishimoto, 2013; Sauder, 2014; Mohee and Kamal, 2017), and coatings (Matějíček and Chráska, 2011; Mondal et al., 2021a; Mondal et al., 2021b), all designed to enhance the performance, safety, and longevity of nuclear systems. Through continuous research and development, the nuclear industry strives to push the boundaries of materials science and engineering, paving the way for advanced manufacturing techniques that revolutionize the field of nuclear energy (Bloom et al., 2007; Whittle, 2016).
Developing advanced materials specifically tailored for the nuclear industry is of utmost importance in advancing nuclear energy (Guérin et al., 2009; Allen et al., 2010). These materials must possess exceptional properties that can withstand the high levels of radiation and extreme temperatures encountered within nuclear energy systems (Little, 2006). Significant progress in materials science has led to the development of novel materials that exhibit remarkable resilience in harsh nuclear environments (Zinkle and Was, 2013; Konings and Stoller, 2020). One such example is the use of graphite and its composites, which offer enhanced resistance to radiation damage and possess excellent thermal properties (Burchell et al., 1991; Kanari et al., 1997). These composites can be employed in various applications, including the construction of reactor cores and fuel assemblies (Bonal et al., 2009).
Advanced ceramics have also emerged as promising materials for nuclear energy (Marra, 2011). These ceramics exhibit excellent thermal stability, corrosion resistance, and high melting points, making them ideal for applications in reactor components, fuel cladding, and containment systems (Yano and Matović, 2013). The use of ceramics enhances the overall safety and performance of nuclear reactors (Prakash et al., 2015).
High-temperature alloys have been another area of focus in materials development for the nuclear industry (Shirzadi and Jackson, 2014). These alloys can withstand extreme temperatures and maintain their mechanical integrity, making them suitable for use in critical components such as turbine blades and heat exchangers (Lai, 1990). The ability of high-temperature alloys to withstand the demanding conditions of nuclear energy systems ensures the reliability and longevity of these components (El-Genk and Tournier, 2005).
In addition to these specific materials, advancements in materials science have also led to the development of improved coatings and surface treatments (Mondal et al., 2021b). These coatings provide additional protection against corrosion, wear, and radiation damage, further enhancing the performance and durability of nuclear components (Kashkarov et al., 2021).
The continuous research and development of materials for advanced manufacturing in the nuclear industry are crucial for improving the efficiency, safety, and reliability of nuclear energy systems (Zinkle, 2005; Raj et al., 2008). By using materials such as graphite composites, advanced ceramics, high-temperature alloys, and specialized coatings, the nuclear industry can achieve optimal performance and meet the demanding requirements of nuclear energy generation (Aguiar et al., 2020).
2.2.1 Advanced materials for enhanced performance
Advanced manufacturing techniques enable the use of novel materials with improved properties in the nuclear industry (Nelson, 2023). For instance, materials with enhanced resistance to radiation, high-temperature capabilities, and corrosion resistance can be produced using techniques such as powder metallurgy and laser cladding (Kim et al., 2015; Wan et al., 2021). These advanced materials and technologies contribute to the longevity and safety of nuclear components, increasing their reliability and reducing the need for frequent maintenance and replacement (Bergeron and Crigger, 2018).
2.3 Applications
Advanced manufacturing techniques such as 3D printing and robotics improve the nuclear industry by producing customized parts, enhancing efficiency, handling hazardous materials, and reducing construction time and cost (Qing-yuan et al., 2022). These advancements ensure safety, performance, and cost-effectiveness while meeting global energy demands and promoting low-carbon electricity generation (Betzler, 2021). This section provides details about the applications of advanced manufacturing techniques in the nuclear energy industry.
2.3.1 Additive manufacturing for customized parts
Additive manufacturing, also known as 3D printing, offers significant advantages in producing customized parts for the nuclear industry (Koyanagi et al., 2021). It allows for the production of complex geometries and intricate designs that are challenging to manufacture using traditional methods (Christopher, 2015). Additive manufacturing enables the fabrication of components with improved functionality, reduced weight, and enhanced performance (Tang et al., 2016). Examples include the production of turbine blades, heat exchangers, and fuel assemblies with optimized designs and improved cooling capabilities (Kaur and Singh, 2021). For example, at the ORNL Manufacturing Demonstration Facility, four pioneering fuel assembly brackets were successfully 3D printed for the first time (Nuclear reactor components 3D printed by ORNL now installed at TVA Browns Ferry nuclear plant, 2021). These brackets—developed in collaboration with the Tennessee Valley Authority, Framatome, and the Transformational Challenge Reactor program funded by the US Department of Energy Office of Nuclear Energy—have been installed and are currently operating under routine conditions at Unit 2 of the Browns Ferry Nuclear Plant in Athens, Alabama. Figure 8 shows 3D printed channel fasteners for Framatome’s boiling water reactor fuel assembly. The simplicity of the channel fastener geometry, although asymmetrical, makes it an ideal candidate for the inaugural application of additive manufacturing within a nuclear reactor setting.
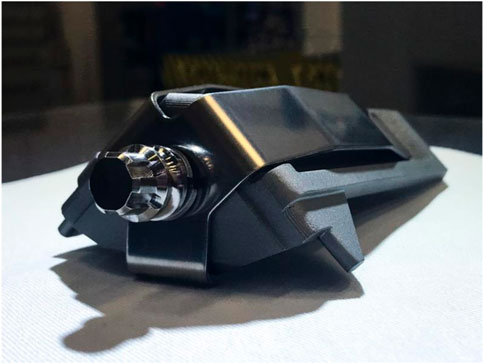
FIGURE 8. Using innovative additive manufacturing techniques, ORNL employed 3D printing to create channel fasteners specifically designed for Framatome’s boiling water reactor fuel assembly. At the Tennessee Valley Authority’s Browns Ferry Nuclear Plant, four components similar to that shown were successfully installed. Credit: Framatome (Nuclear reactor components 3D printed by ORNL now installed at TVA Browns Ferry nuclear plant, 2021).
2.3.2 Automation and robotics for efficiency
Automation and robotics are crucial for enhancing efficiency and safety in nuclear manufacturing processes (Rajagopalan and Venugopal, 2013). These technologies enable precise and repetitive tasks, reducing human error and minimizing the risk of accidents. Robotic systems can be used for tasks such as welding, inspection, and material handling, ensuring consistent quality and adherence to stringent safety standards (Koren and Koren, 1985). Automation and robotics also contribute to increased productivity and reduced production time by streamlining workflows and reducing manual labor requirements (Deb and Deb, 2010).
2.3.3 Remote handling and manipulation
In nuclear facilities, certain operations require handling hazardous or radioactive materials (Won et al., 1997). Advanced manufacturing techniques facilitate the development of remote handling systems and manipulators to perform these tasks (Ibarra et al., 2010). Remote handling systems use robotic arms, specialized grippers, and advanced sensors to safely handle radioactive materials, reducing the risk to human operators (Wilson, 1983). These systems are used for activities such as radioactive waste disposal, maintenance of nuclear reactors, and handling of nuclear fuel and spent fuel (McAffee et al., 1997).
2.3.4 Modular construction and prefabrication
Modular construction and prefabrication techniques are gaining traction in the nuclear industry because of their potential for reducing construction time, reducing cost, and enhancing quality control (Wrigley et al., 2021). Advanced manufacturing combined with automation enables the fabrication of modular components and structures off-site in controlled environments, ensuring precision and minimizing errors (Neelamkavil, 2009; Arashpour et al., 2018). These prefabricated modules can be transported to the nuclear site and assembled efficiently, reducing downtime during construction and maintenance activities.
2.3.5 Waste management and decommissioning
Advanced manufacturing techniques also find applications in the field of nuclear waste management and decommissioning (Vitanov et al., 2021). Robotics and automation can be used for handling and processing radioactive waste, minimizing the risk to workers and the environment (Vitanov et al., 2021). Additive manufacturing techniques can also be used to produce shielding materials or encapsulation structures for safely storing radioactive waste (Beck et al., 2023). Additionally, advanced manufacturing enables the fabrication of cutting and dismantling tools required during the decommissioning of nuclear facilities (Huang et al., 2013).
Overall, advanced manufacturing techniques offer a wide range of applications in the nuclear energy industry (Mondal et al., 2023). From additive manufacturing for customized parts to automation and robotics for increased efficiency, these technologies contribute to improved safety, performance, and cost effectiveness. The adoption of advanced manufacturing in the nuclear industry is vital for advancing the sector and meeting the increasing global energy demands while ensuring low-carbon electricity generation.
2.4 Testing and validation
Testing and validation are critical steps in the manufacturing of nuclear components (Boring et al., 2015). Nondestructive testing techniques such as ultrasonic testing and x-ray inspection can be used to detect defects and ensure the quality of nuclear components (Casalta et al., 2003). Simulation and modeling can also be used to test the performance of nuclear components under different conditions, reducing the need for costly physical testing (Li et al., 2018).
However, the manufacturing of nuclear components is highly regulated to ensure safety and security (Findlay, 2010; Eom et al., 2023). Manufacturers must comply with strict quality assurance and quality control standards to ensure that nuclear components meet regulatory requirements. Advanced manufacturing techniques must also be validated and approved by regulatory bodies before they can be used in the production of nuclear components.
2.5 Challenges
Advanced manufacturing in the nuclear industry faces several challenges (Cannon et al., 2022). Strict safety regulations and compliance requirements necessitate careful evaluation and qualification processes for introducing advanced techniques (McMurtrey and Messner, 2021). Ensuring high-quality standards through robust quality assurance and certification processes is crucial when considering the complexities and variables introduced by advanced manufacturing. Selecting suitable materials with specific properties such as strength and resistance to radiation and then qualifying their performance under extreme operating conditions can pose challenges. Long-term material performance, including durability and aging, must be assessed to ensure component reliability. Covering initial investment costs and achieving cost-effective mass production while maintaining quality and safety present financial challenges. A skilled workforce proficient in advanced manufacturing technologies and providing continuous training must be developed and maintained. Robust cybersecurity measures are essential to protect sensitive data, intellectual property, and control systems from potential vulnerabilities. Continuous monitoring, incident response plans, and secure endpoints improve cyber resilience. Employee training is vital for averting security breaches, while integrating physical and digital safeguards warrants a comprehensive defense. Supply chain security, regulatory compliance, and a Zero Trust model underwrite to a cohesive cybersecurity framework. Regular updates are essential for sustained effectiveness in safeguarding nuclear systems (He et al., 2022). Addressing these challenges requires collaboration between industry, regulatory bodies, and research institutions to establish standards and protocols that prioritize safety, quality, and reliability in the application of advanced manufacturing in the nuclear energy sector.
3 Digital twin technology
Digital twin technology, with its ability to create virtual replicas of physical systems, offers unprecedented opportunities for optimizing nuclear operations (Liu et al., 2021). This section explores the concept of digital twin technology and its application in the nuclear industry. It discusses how digital twins can improve system monitoring, maintenance planning, and operational decision-making. The section also addresses the challenges of implementing digital twin technology in the nuclear sector and presents potential solutions.
3.1 Understanding digital twin technology: unleashing the potential of virtual replications
Digital twin technology is an innovative approach that involves creating a virtual model or replica of a physical system, whether it is a machine, building, industrial production process, or even an entire city. This virtual model aims to accurately mimic the characteristics, behavior, and functionality of its physical counterpart in real time. Harnessing the power of digital twins yields deeper insights into the performance, behavior, and life cycle of the physical system, enabling more effective monitoring, analysis, and optimization.
The term digital twin was first introduced in NASA’s draft version of the technological road map in 2010, and it was also known as the virtual digital fleet leader (Shafto et al., 2012). NASA played a pivotal role in defining the concept of the digital twin, characterizing it as an integrated simulation of a vehicle or system that combines various physical models, sensor updates, and fleet history to replicate the life and behavior of its real-world counterpart (Singh et al., 2021). NASA had previously employed a similar concept during the Apollo program, constructing two identical space vehicles to closely emulate each other (Boschert and Rosen, 2016). Inspired by NASA’s work, the US Air Force also adopted digital twin technology for aircraft design, maintenance, and prediction to simulate the physical and mechanical properties of aircraft to anticipate potential fatigue or structural issues and thereby extend their operational life spans (Tuegel et al., 2011). Researchers such as (Gockel et al., 2012; Tuegel, 2012) referred to the aircraft-specific version of the digital twin as the airframe digital twin, which served as a computational model for effectively managing the entire life cycle of an aircraft. But there are earlier mentions of DTs. The idea first came up as mirrored space models and information mirroring models in the context of managing product lifecycles (Grieves and Vickers, 2017a).
Furthermore, digital twin concepts were also proposed for sustainable space exploration and the advancement of future generations of aerospace vehicles (Glaessgen and Stargel, 2012). The evolution of digital twin technology spans from the conceptualization of “mirror worlds” in 1991 to the formalization of the term “digital twin” in 2010. Figure 9 shows a timeline depicting the evolution of digital twin technology (Singh et al., 2021). This historical journey not only refers to the origin of digital twin concepts but also aligns seamlessly with its application in advanced manufacturing. By outlining this progression, one can aim to illustrate how the foundational ideas evolved into a comprehensive paradigm, demonstrating the natural alignment of digital twin technology with the intricate needs of advanced manufacturing processes.
Real-time monitoring is a key advantage of digital twin technology because it allows for continuous monitoring of the physical system. By connecting sensors and data collection devices to the physical system, the digital twin receives a constant stream of data, reflecting the current state and conditions of the system. These real-time data facilitate proactive measures, enabling the identification of potential issues before they escalate. The feature significantly reduces downtime and enhances operational efficiency by empowering operators and maintenance personnel to address problems promptly and effectively.
Another valuable aspect of digital twins is their ability to simulate and optimize the performance of the physical system. When the virtual model is leveraged, different operating scenarios can be tested and analyzed without any effect on the actual physical system. This feature empowers organizations to experiment, optimize, and enhance performance, leading to improved efficiency and cost reduction.
Digital twins also span the entire life cycle of a system, including design, development, operation, and maintenance stages. They offer a comprehensive view of the system’s performance over time, enabling better decision-making at each stage. Moreover, digital twins can be continuously updated with new data and insights, ensuring their accuracy and relevance throughout the system’s life span.
Facilitating collaboration and data sharing is another significant benefit of digital twin technology. Various teams, including engineers, operators, and maintenance personnel, can access and interact with the digital twin. This feature promotes better communication, knowledge sharing, and collective problem-solving, resulting in more effective decision-making and improved outcomes.
In summary, digital twin technology revolutionizes how the performance of physical systems is simulated, monitored, and optimized. By creating virtual replicas and integrating real-time data, digital twins enable proactive maintenance, simulation-based optimization, and enhanced decision-making throughout the life cycle of the system. This technology is a powerful tool that drives efficiency, reduces downtime, and facilitates collaborative problem-solving across industries and domains.
3.2 Applications of digital twin in various industries
Digital twin technology can be applied in various areas of the nuclear energy industry, including reactor design and operation, waste management, and decommissioning (Varé and Morilhat, 2020; Bowman et al., 2022; Gong et al., 2023). In reactor design and operation, digital twins can be used to simulate different reactor scenarios that can be analyzed to optimize performance and safety. They can also be used to predict and prevent component failures, reducing the likelihood of accidents and unplanned outages. In waste management, digital twins can be used to simulate waste storage and transport scenarios, identify potential safety hazards, and optimize waste management practices. In decommissioning, digital twins can be used to simulate the dismantling process, identify potential risks, and optimize decommissioning strategies.
Additionally, digital twin technology offers diverse applications in the nuclear industry. It enables the creation of virtual models that replicate physical systems, enhancing operational efficiency and maintenance planning through insights into system performance and issue identification. Predictive maintenance and condition monitoring are facilitated by analyzing real-time data, detecting potential failures, optimizing maintenance schedules, and extending critical equipment life spans. In training and operator support, digital twins simulate realistic scenarios, improving operator skills, decision-making, and emergency response. By integrating data from multiple sources, digital twins optimize system performance, identify bottlenecks, and reduce operational costs. They aid in decommissioning by planning and simulating the dismantling process and contribute to waste management by providing insights into handling radioactive materials. Additionally, digital twins assess safety protocols, support risk analysis, and enhance safety culture in nuclear facilities. Leveraging digital twins enables the nuclear industry to achieve improved efficiency, enhanced maintenance, optimized performance, and heightened safety, contributing to dependable, low-carbon electricity generation.
3.3 Challenges
Integrating digital twin technology in the nuclear energy industry presents notable challenges that warrant careful consideration. Among these challenges, data management emerges as a significant concern. Digital twins rely heavily on accurate, timely data to ensure simulations fidelity. However, in the highly regulated environment of the nuclear energy sector, stringent security protocols may restrict data sharing. Striking a balance between data accessibility and security becomes a critical aspect in implementing digital twin solutions.
Modeling and Simulation (M&S) tools are critical in the field of Nuclear Engineering (NE), facilitating the analysis and virtual representation of complicated nuclear systems. Key tools encompass Monte Carlo N-Particle (MCNP) for simulating neutron transport, Standardized Computer Analysis for Licensing Evaluation (SCALE) for comprehensive safety analysis, including criticality safety, reactor physics, and radiation shielding modules. Additionally, Reactor Excursion and Leak Analysis Program (RELAP5) is laboring for thermal-hydraulic simulations, Transient Reactor Analysis Code (TRAC) for analyzing reactor behavior, and Methods of Estimation of Leakages and Consequences of Releases (MELCOR) for modeling severe accidents in nuclear power plants. Open-source Computational Fluid Dynamics (CFD) tool Open-source Field Operation and Manipulation (OpenFOAM) is utilized for simulating fluid flows and heat transfer in nuclear systems. Furthermore, Reactor Excursion and Leak Analysis Program/Source Term Code and Assessment Program Simulation (RELAP/SCDAPSIM) serves to simulate reactor transients, accidents, and severe core damage. These tools permit engineers and researchers to model and comprehend the behavior of nuclear systems under diverse conditions, thus contributing meaningfully to the design, safety, and optimization of nuclear power plants. When integrated with Digital Twin technology, these tools enhance the capability to predict and manage the real-time performance of nuclear systems.
The M&S tools at present working in NE vary significantly in scale and fidelity. System codes can simulate whole reactor systems but bargain lower fidelity owing to simplification and approximation. Predicting relevant quantities for different scenarios includes uncertainties. Component codes offer higher fidelity but are computationally expensive, limited to single physics or reactor components. Therefore, substantial resources and effort are obligatory for the development and assessment of these codes.
The level of expertise necessary to develop and maintain digital twins presents another key challenge. The creation and management of digital twins demand a profound understanding of nuclear energy systems and the intricacies of digital twin technology. The scarcity of experts possessing this dual proficiency poses a potential barrier to the widespread adoption of digital twin solutions in the industry.
To address these challenges, concerted efforts among industry stakeholders, regulatory bodies, and technology providers are imperative. Establishing robust frameworks for secure data sharing, ensuring compliance with regulatory requirements, and safeguarding confidentiality are paramount. Furthermore, investment in comprehensive training and educational programs to cultivate a skilled workforce equipped with the necessary multidisciplinary competencies is vital. By proactively addressing these challenges, the nuclear energy industry can unlock the full potential of digital twin technology, driving advancements in operational efficiency, safety, and overall performance.
4 Applications and challenges in advanced manufacturing and digital twin technology for nuclear energy
4.1 Manufacturing of nuclear components and result prediction
The combination of advanced manufacturing and digital twin technology offers new opportunities to improve the efficiency, reliability, and safety of nuclear energy production (Niu and Qin, 2021). One potential application is in the manufacturing of nuclear components (Kropaczek et al., 2023). Advanced manufacturing techniques like 3D printing can be used to produce complex geometries with high precision, reducing the need for assembly and reducing the number of components required. Digital twin technology can be used to simulate the manufacturing process, optimize the process, and reduce the risk of errors. The prediction of outcomes in additive manufacturing by using digital twins and precise models has the potential to revolutionize the field. Currently, additive manufacturing relies heavily on a trial-and-error approach involving extensive experimentation and destructive testing to evaluate microstructures and mechanical properties (Haslam et al., 2022). However, to produce site-specific parts, the ability to predict additive manufacturing outcomes becomes crucial. By accurately predicting results such as geometry, microstructures, and mechanical properties before the actual manufacturing process, additive manufacturing can become more efficient and cost effective.
The concept of the digital twin offers the potential to accurately predict results such as geometry, microstructures, and mechanical properties before the actual additive manufacturing process begins. This prediction relies on precise heat transfer and mechanical models, as illustrated in Figure 10 (Mukherjee and DebRoy, 2019). By employing these models, it becomes possible to efficiently identify optimal combinations, saving valuable time and resources.
Presently, the focus of additive manufacturing result prediction primarily lies in the areas of distortion (dimensional stability) and temperature distribution (Chen et al., 2022). These predictions are often based on dividing the part into discrete units or grids and computing the properties of each unit to obtain the overall properties of the part. Similar simulation approaches used in welding, which benefit from well-established knowledge in the field, can be leveraged. These approaches include predicting weld metal solidification (David and Vitek, 1989; Dye et al., 2001), solid-state transformations under various conditions (Kirkaldy, 1991), and static and dynamic mechanical properties (Ion et al., 1984; Kang et al., 2007; Yang et al., 2008).
For instance, Song et al. (Song et al., 2018) developed a robust numerical model to simulate convective fluid flow and surface tension forces at the air–fluid interface. They used an arbitrary Lagrangian–Eulerian moving mesh approach to calculate the physical interface of the free surface. Through this approach, they successfully predicted thermal gradient directions and solidified clad dimensions, and experimental validation demonstrated variations in clad height and melt-pool depth within a margin of less than 10%. Predicting crucial factors such as thermal history, part distortion, microstructure, and mechanical properties plays a vital role in the digital twin for additive manufacturing. It enables the optimization of parameters, process control, condition monitoring, and the attainment of expected results. However, the actual customization of final component properties based on predictive models still remains a distant goal.
Lately, researchers at Oak Ridge National Laboratory have developed Peregrine, an AI software for powder bed 3D printers (Scime et al., 2022). It estimates part quality in real time, eradicating the need for expensive characterization equipment. Peregrine also contributes to ORNL’s advanced manufacturing “digital thread,” which collects and analyzes data across the entire manufacturing process, spanning design, feedstock selection, print builds, and material testing.
Emerging evidence and ongoing research suggest the feasibility of developing a preliminary version of a digital twin for additive manufacturing. However, this technology is currently in its early stages and encounters several research obstacles. The essential components for constructing a digital twin of additive manufacturing, including hardware, software, and associated technologies, are still undergoing development, necessitating further efforts to fully realize its capabilities (Debroy et al., 2017). DebRoy, Yang, and their colleagues have made significant strides in the field, pioneering this domain (Debroy et al., 2017; Knapp et al., 2017; Mukherjee and DebRoy, 2019). Their extensive work has laid the foundation for implementing digital twin technology and has provided valuable insights within the additive manufacturing research community. They have presented an overarching framework for the realization of digital twins in additive manufacturing, conducting exploratory studies to showcase the concept’s applicability. Furthermore, they have outlined the fundamental elements necessary for constructing a first-generation digital twin of additive manufacturing, encompassing a mechanistic model, a sensing and control model, a statistical model, and the integration of big data and machine learning techniques. Figure 11 depicts the comprehensive structure proposed by their research (Knapp et al., 2017; Mukherjee and DebRoy, 2019).
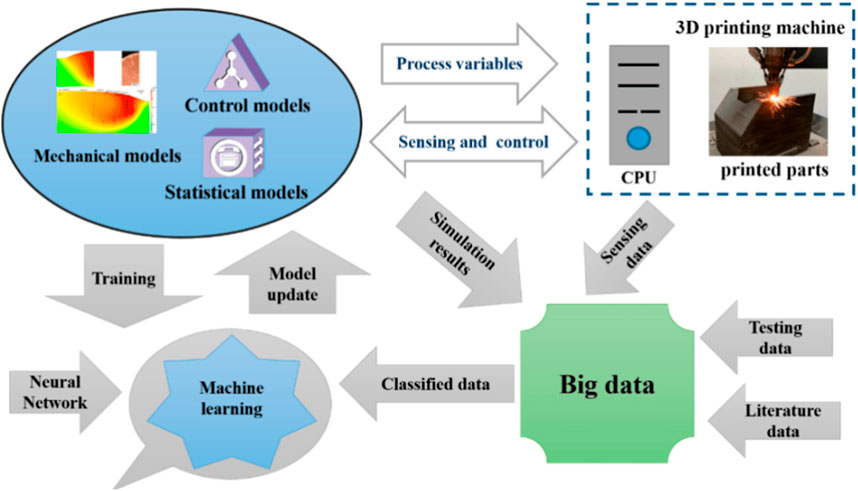
FIGURE 11. Conceptual framework of the digital twin for additive manufacturing. Reprinted with permission from (Song et al., 2018; Mukherjee and DebRoy, 2019; Zhang et al., 2020). Copyright 2020, licensed under MDPI, Basel, Switzerland.
4.2 Operation of nuclear power plants
Another application of integrated advanced manufacturing and digital twin is in the operation of nuclear power plants (Ayo-Imoru et al., 2021; Gong et al., 2023). Digital twins can be used to simulate different operating scenarios, optimizing the plant’s performance and reducing the likelihood of accidents and unplanned outages (Lee et al., 2019). Advanced manufacturing techniques can also be used to produce replacement parts more quickly and efficiently, reducing the downtime of the plant (Milewski and Milewski, 2017; Mondal et al., 2020a; Mondal and McMurtrey, 2020; Free et al., 2021; Mondal and Tripathy, 2021).
Using advanced manufacturing and digital twin technology in the nuclear energy industry is not without challenges (Fuller et al., 2020; Roy et al., 2020; Jharko et al., 2021; Kochunas and Huan, 2021; Yadav et al., 2023). One of the significant challenges is data management. Digital twins require accurate and up-to-date data to ensure the accuracy of simulations. The nuclear energy industry is highly regulated, and data sharing can be restricted because of security concerns. Another challenge is the high level of expertise required to develop and maintain digital twins, which can be a significant barrier to adoption.
4.3 Enhancing safety and efficiency
The integration of advanced manufacturing techniques and digital twin technology could revolutionize safety and efficiency in the field of nuclear energy (Eckhart and Ekelhart, 2019; Ghenai et al., 2022; Zheng et al., 2022). By combining these technologies, various aspects of nuclear operations can be enhanced, resulting in significant advantages. This section delves into the specific areas in which these advancements can bring about positive changes, including operational efficiency, system behavior prediction, and maintenance schedule optimization. Additionally, it explores the broader implications of improved safety and reduced downtime within the nuclear industry, emphasizing the benefits derived from sustainable and reliable electricity generation.
Operational efficiency stands out as a crucial aspect that can be bolstered by integrating advanced manufacturing and digital twin technology (Gunasegaram et al., 2021; Wang et al., 2021). By using advanced manufacturing techniques such as additive manufacturing, components can be fabricated with increased precision and reduced lead times (Ding et al., 2015b). This effort enables more efficient operations, streamlined supply chains, and reduced costs. Moreover, digital twin technology allows for real-time monitoring and analysis of nuclear systems, enabling proactive identification of potential issues, optimization of processes, and continuous improvement (Ritter et al., 2022; Xu et al., 2022).
System behavior prediction is another key area in which these technologies prove valuable. Digital twins can simulate the behavior of nuclear systems, considering various factors such as operational conditions, material properties, and external influences (Grieves and Vickers, 2017b; Brockhoff et al., 2021). This capability allows operators to forecast the performance and behavior of the system, enabling better decision-making and risk management. By accurately predicting system behavior, potential anomalies or failures can be addressed in a timely manner, mitigating risks and ensuring the safety and reliability of nuclear operations.
Furthermore, the integration of advanced manufacturing techniques and digital twin technology facilitates optimized maintenance schedules (Aivaliotis et al., 2019; Park et al., 2020). With real-time data and predictive capabilities, digital twins can identify optimal maintenance intervals, reducing unnecessary downtime and maximizing the availability of nuclear systems. By implementing condition-based maintenance approaches—in which maintenance activities are scheduled based on the actual condition of the components rather than predefined time intervals—resources can be used more efficiently, resulting in cost savings and improved operational continuity.
The implications of enhanced safety and reduced downtime in the nuclear industry are profound (Wright and Davidson, 2020). Improved safety measures facilitated by advanced manufacturing techniques and digital twins lead to enhanced risk mitigation and accident prevention (Xiong et al., 2021). This improved safety ensures the protection of both the workers and the environment. Additionally, reduced downtime contributes to increased availability and productivity, leading to higher electricity generation efficiency and reliability. These benefits are particularly significant for nuclear power, which plays a vital role in providing sustainable and low-carbon electricity (Borowski, 2021).
The convergence of advanced manufacturing techniques and digital twin technology offers a transformative opportunity to enhance safety and efficiency in the nuclear energy sector. Through the integration of these technologies, operational efficiency can be optimized, enabling accurate predictions of system behavior and the effective scheduling of maintenance activities. The resulting advantages encompass heightened safety measures, reduced downtime, and enhanced sustainability, all of which collectively contribute to the reliable and sustainable generation of nuclear power. This integration represents a significant leap toward creating a safer and more efficient nuclear energy landscape for the future.
4.4 Addressing challenges: data management and cyber security
Using advanced manufacturing techniques and digital twin technology in the nuclear industry presents significant benefits, but it also poses a set of unique challenges that must be effectively addressed (Varé and Morilhat, 2020). This section delves into two critical areas of concern: data management and cybersecurity, both of which play pivotal roles in maintaining the integrity and safety of nuclear industry systems.
Data management is a supreme challenge, given the requirement for accurate and up-to-date data to fuel the simulations and analyses conducted by digital twins (Kobayashi et al., 2022). The nuclear energy industry functions in a tightly regulated environment with firm data sharing protocols due to security considerations. Ensuring secure, reliable, and efficient data acquisition, storage, and processing becomes a vital task. Robust data management protocols are essential to manage the vast amounts of data generated by digital twins, ensuring their accuracy, and enabling informed decision-making for the safety and efficiency of nuclear operations.
Additionally, cybersecurity emerges as a crucial aspect of deploying advanced manufacturing and digital twin technology within the nuclear industry (Guo et al., 2021). Nuclear facilities house sensitive and critical information that must be safeguarded against cyber threats. The potential consequences of a cyberattack on nuclear systems are severe, including the compromise of operational integrity, safety risks, and potential disruptions to the energy supply. Thus, implementing comprehensive cybersecurity measures, including robust network security, data encryption, access control, and continuous monitoring, becomes imperative to protect against cyber threats and preserve the security of nuclear operations.
By undertaking these challenges, the nuclear industry guarantees operational integrity and safety while unlocking the full potential of advanced manufacturing and digital twin technology. The foundation stone of a secure and resilient nuclear energy landscape lies in robust data management protocols and a comprehensive cybersecurity strategy. This includes advanced network security, encryption for data in transit and at rest, strict access controls, nonstop monitoring for real-time threat detection, and a streamlined incident response plan. Additional defense layers comprise regular data backups, endpoint security, employee training on cybersecurity best practices, and attentions for physical security, supply chain, and regulatory compliance standards. Implementing a Zero Trust security model further strengthens this protective framework, jointly safeguarding sensitive data, intellectual property, and control systems, ensuring the complete integrity and security of nuclear operations while minimizing vulnerabilities (He et al., 2022).
5 Future scope for integrated advanced manufacturing and digital twin in nuclear industries
The future scope for integrated advanced manufacturing and digital twins in the nuclear industry is promising and holds immense potential for further advancements (Cimino et al., 2019; Bong Kim et al., 2022). One area of focus is the development of advanced materials specifically tailored for nuclear applications. Advanced manufacturing techniques such as additive manufacturing can be leveraged to fabricate components with improved properties, including enhanced radiation resistance, corrosion protection, and increased durability. The use of modeling and simulation approaches shows highly advantageous for advancing additive manufacturing, facilitating a deeper understanding of process control and optimizing parameters (Stournaras et al., 2009; Bikas et al., 2016; Foteinopoulos et al., 2018; Foteinopoulos et al., 2020). Digital twin technology can simulate the behavior and performance of these advanced materials under various operating conditions, thus improving material selection and qualification processes.
Another aspect of future scope lies in the optimization of nuclear plant operations can be achieved by integrating advanced manufacturing and digital twin technology (Rahman et al., 2022). By using digital twins to create virtual models of the plant, operators can gain valuable insights into system behavior, identify potential bottlenecks, and optimize operational parameters (Gong et al., 2022; Baniqued et al., 2023). Advanced manufacturing techniques can aid in the rapid prototyping and production of replacement components, reducing downtime and improving plant reliability. This integration can also enhance predictive maintenance strategies, allowing for timely interventions and minimizing the risk of equipment failures.
Furthermore, the future scope extends to the field of decommissioning and sustainable waste management (Barni et al., 2018). Digital twins can assist in planning and optimizing decommissioning activities, providing insights into dismantling processes, waste handling, and disposal strategies. Advanced manufacturing techniques can contribute to the fabrication of the specialized tools and equipment needed for decommissioning, reducing costs, and improving overall efficiency.
To realize this future scope, collaborative efforts between industry stakeholders, research institutions, and regulatory bodies are crucial. Developing standardized protocols, guidelines, and qualification processes for advanced manufacturing and digital twin technologies in the nuclear industry will help ensure their successful implementation. Additionally, investment in research and development will drive innovation and advancements in materials, processes, and digital twin capabilities specific to nuclear applications.
By harnessing the potential of integrated advanced manufacturing and digital twin technology, the nuclear industry can further enhance safety, efficiency, and sustainability. These technologies pave the way for continuous improvement, enabling the nuclear energy sector to meet the growing global energy demands while ensuring the production of low-carbon electricity.
Advanced Manufacturing and Digital Twin Technology are transformative methodologies that have potential to revolutionized industries, each offering unique advantages and facing distinct challenges. Table 1 below provides a comprehensive comparison of these technologies, outlining their respective pros and cons and detailing the improvements facilitated by their application in various industrial contexts.
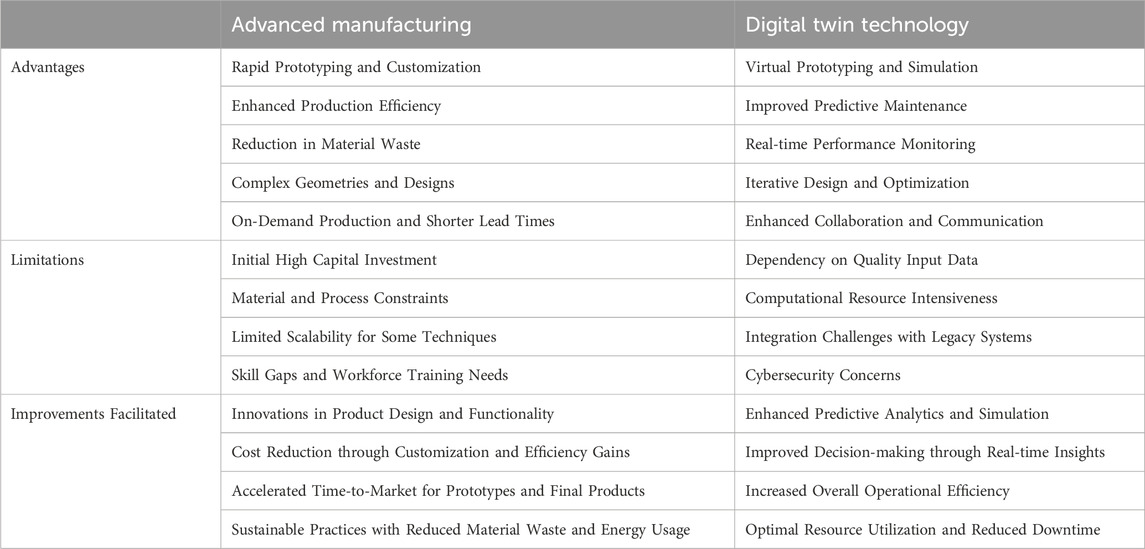
TABLE 1. Comparison of advanced manufacturing and digital twin technology (Stornelli et al., 2021; Thelen et al., 2022).
In the context of advanced manufacturing within the nuclear energy sector, it is imperative to extend the evaluation to include Hybrid Manufacturing, a fabrication process that integrates both additive and subtractive techniques. Traditional machining methods such as milling, turning, and drilling, as well as advanced processes like laser machining, grinding, electrical discharge machining (EDM), and abrasive jet machining, play an essential role in post-processing and finishing stages. These subtractive techniques contribute to attain precise geometries, tight tolerances, and desired surface finishes, synergizing with the design flexibility obtainable by additive manufacturing. The incorporation of Hybrid Manufacturing embraces substantial relevance as it offers a distinct approach to fabricating complex components, contribute distinct advantages and also subsequent challenges. By probing Hybrid Manufacturing alongside other advanced manufacturing processes and digital twin technologies, a more comprehensive understanding of the evolving landscape in the nuclear industry develops (Stavropoulos et al., 2018; Stavropoulos et al., 2020). This consolidative analysis can unveil synergies, potential optimizations, and novel applications that arise from the combination of Advanced manufacturing, Hybrid Manufacturing, and digital twin technologies, thus enriching the complete understanding on the transformative potential of these innovations within the nuclear sector (Langlotz et al., 2022; Huang et al., 2023).
6 Conclusion
This comprehensive review highlights the critical role of integrating digital twin technology with advanced manufacturing techniques in advancing the nuclear industry. It emphasizes the potential of these synergistic technologies to enhance performance, minimize downtime, and bolster safety within nuclear energy ecosystems. In spite of these possibilities, the imperative to address challenges in data management and cybersecurity is underlined for the full realization of these benefits. In core, the review expresses the indispensable nature of digital twin technology alongside advanced manufacturing in propelling the nuclear industry forward. It provides a shade in understanding of their collective potential, not only in optimizing performance and reducing downtime but also in fundamentally fortifying safety protocols. However, the transformative impact of these technological developments is contingent upon effectively tackling the substantial challenges posed by data management and cybersecurity. Steering these challenges underlines the strategic necessity of embracing the synergy between advanced manufacturing and digital twin technology in the nuclear industry. This integration positions the sector to make substantial contributions to the global energy landscape, presenting an opportunity to deliver reliable, low-carbon electricity and playing a key role in fostering a sustainable and resilient energy future.
Author contributions
KM: Conceptualization, Investigation, Supervision, Visualization, Writing–original draft, Writing–review and editing. OM: Conceptualization, Funding acquisition, Resources, Writing–original draft, Writing–review and editing. PJ: Supervision, Writing–review and editing.
Funding
The author(s) declare financial support was received for the research, authorship, and/or publication of this article. This work was supported by the US Department of Energy Office of Nuclear Energy, Office of Spent Fuel and Waste Disposition.
Acknowledgments
The authors gratefully acknowledge the support of the ORNL Nuclear Energy and Fuel Cycle Division.
Conflict of interest
The authors declare that the research was conducted in the absence of any commercial or financial relationships that could be construed as a potential conflict of interest.
The author(s) declared that they were an editorial board member of Frontiers, at the time of submission. This had no impact on the peer review process and the final decision.
Publisher’s note
All claims expressed in this article are solely those of the authors and do not necessarily represent those of their affiliated organizations, or those of the publisher, the editors and the reviewers. Any product that may be evaluated in this article, or claim that may be made by its manufacturer, is not guaranteed or endorsed by the publisher.
Footnotes
*Notice: This manuscript has been authored by UT-Battelle LLC under contract DE-AC05-00OR22725 with the US Department of Energy (DOE). The US government retains and the publisher, by accepting the article for publication, acknowledges that the US government retains a nonexclusive, paid-up, irrevocable, worldwide license to publish or reproduce the published form of this manuscript, or allow others to do so, for US government purposes. DOE will provide public access to these results of federally sponsored research in accordance with the DOE Public Access Plan (http://energy.gov/downloads/doe-public-access-plan).
References
4 Major Opportunities for Additive Manufacturing in Nuclear Energy, 2019 4 Major Opportunities for Additive Manufacturing in Nuclear Energy (2019). 4 major opportunities for additive manufacturing in nuclear energy. Available at: https://www.energy.gov/ne/articles/4-major-opportunities-additive-manufacturing-nuclear-energy.
Aguiar, J. A., Jokisaari, A. M., Kerr, M., and Allen Roach, R. (2020). Bringing nuclear materials discovery and qualification into the 21st century. Nat. Commun. 11, 2556. doi:10.1038/s41467-020-16406-2
Aivaliotis, P., Georgoulias, K., Arkouli, Z., and Makris, S. (2019). Methodology for enabling digital twin using advanced physics-based modelling in predictive maintenance. Procedia Cirp 81, 417–422. doi:10.1016/j.procir.2019.03.072
Akinlabi, E. T., Mahamood, R. M., and Akinlabi, S. A. (2016). Advanced manufacturing techniques using laser material processing. IGI Glob.
Allen, T., Busby, J., Meyer, M., and Petti, D. (2010). Materials challenges for nuclear systems. Mat. Today 13, 14–23. doi:10.1016/S1369-7021(10)70220-0
Arashpour, M., Miletic, M., Williams, N., and Fang, Y. (2018). Design for manufacture and assembly in off-site construction: advanced production of modular façade systems. Lyon, France: IAARC Publications, 1–6.
Arinez, J. F., Chang, Q., Gao, R. X., Xu, C., and Zhang, J. (2020). Artificial intelligence in advanced manufacturing: current status and future outlook. J. Manuf. Sci. Eng. 142. doi:10.1115/1.4047855
Attaran, M., Attaran, S., and Celik, B. G. (2023). The impact of digital twins on the evolution of intelligent manufacturing and Industry 4.0. Adv. Comput. Intell. 3, 11. doi:10.1007/s43674-023-00058-y
Ayo-Imoru, R. M., Ali, A. A., and Bokoro, P. N. (2021). “An enhanced fault diagnosis in nuclear power plants for a digital twin framework,” in 2021 International Conference on Electrical, Computer and Energy Technologies (ICECET), Cape Town, South Africa, 09-10 December, 2021, 1. doi:10.1109/ICECET52533.2021.9698715
Babu, S. S., Love, L., Dehoff, R., Peter, W., Watkins, T. R., and Pannala, S. (2015). Additive manufacturing of materials: opportunities and challenges. MRS Bull. 40, 1154–1161. doi:10.1557/mrs.2015.234
Balbaud, F., Cabet, C., Cornet, S., Dai, Y., Gan, J., Hernández Mayoral, M., et al. (2021). A NEA review on innovative structural materials solutions, including advanced manufacturing processes for nuclear applications based on technology readiness assessment. Nucl. Mat. Energy 27, 101006. doi:10.1016/j.nme.2021.101006
Baniqued, P. D., Bremner, P., Sandisson, M., Harper, S., Agrawal, S., Bolarinwa, J., et al. (2023). Multimodal immersive digital twin platform for cyber-physical robot fleets in nuclear environments. Preprints. doi:10.22541/au.169046495.53097561/v1
Barni, A., Fontana, A., Menato, S., Sorlini, M., and Canetta, L. (2018). “Exploiting the digital twin in the assessment and optimization of sustainability performances,” in 2018 International Conference on Intelligent Systems (IS), Funchal - Madeira, Portugal, 25-27 September 2018. 706–713. doi:10.1109/IS.2018.8710554
Bartsch, K., Pettke, A., Hübert, A., Lakämper, J., and Lange, F. (2021). On the digital twin application and the role of artificial intelligence in additive manufacturing: a systematic review. J. Phys. Mat. 4, 032005. doi:10.1088/2515-7639/abf3cf
Beausoleil, G. L., Parry, M. E., Mondal, K., Kwon, S., Gomez-Hurtado, L. R., Kaoumi, D., et al. (2022). Spark plasma sintered, MoNbTi-based multi-principal element alloys with Cr, V, and Zr. J. Alloys Compd. 927, 167083. doi:10.1016/j.jallcom.2022.167083
Beck, D., Bickus, J., Klein, E., Miller, P., Di Cecca, S., Benz, R., et al. (2023). Additive manufacturing of multimaterial composites for radiation shielding and thermal management. ACS Appl. Mat. Interfaces 15, 35400–35410. doi:10.1021/acsami.2c22478
Bergeron, A., and Crigger, J. (2018). Early progress on additive manufacturing of nuclear fuel materials. J. Nucl. Mat. 508, 344–347. doi:10.1016/j.jnucmat.2018.05.058
Betzler, B. (2021). Additive manufacturing in the nuclear reactor industry. Oak Ridge, TN (United States): Oak Ridge National Lab.
Betzler, B. R., Ade, B. J., Wysocki, A., Greenwood, M. S., Heineman, J., Chesser, P., et al. (2019). Advanced manufacturing for nuclear core design. Oak Ridge, TN (United States): Oak Ridge National Lab.
Bhavar, V., Kattire, P., Patil, V., Khot, S., Gujar, K., and Singh, R. (2017). A review on powder bed fusion technology of metal additive manufacturing. Addit. Manuf. Handb. 251–253.
Bikas, H., Stavropoulos, P., and Chryssolouris, G. (2016). Additive manufacturing methods and modelling approaches: a critical review. Int. J. Adv. Manuf. Technol. 83, 389–405. doi:10.1007/s00170-015-7576-2
Bloom, E. E., Busby, J. T., Duty, C. E., Maziasz, P. J., McGreevy, T. E., Nelson, B. E., et al. (2007). Critical questions in materials science and engineering for successful development of fusion power. Proc. Twelfth Int. Conf. Fusion React. Mat. ICFRM-12 367-370, 1–10. doi:10.1016/j.jnucmat.2007.02.007
Bonal, J.-P., Kohyama, A., van der Laan, J., and Snead, L. L. (2009). Graphite, ceramics, and ceramic composites for high-temperature nuclear power systems. MRS Bull. 34, 28–34. doi:10.1557/mrs2009.9
Bong Kim, D., Shao, G., and Jo, G. (2022). A digital twin implementation architecture for wire + arc additive manufacturing based on ISO 23247. Manuf. Lett. 34, 1–5. doi:10.1016/j.mfglet.2022.08.008
Bonnard, R., Hascoët, J.-Y., and Mognol, P. (2019a). Data model for additive manufacturing digital thread: state of the art and perspectives. Int. J. Comput. Integr. Manuf. 32, 1170–1191. doi:10.1080/0951192X.2019.1690681
Bonnard, R., Hascoët, J.-Y., Mognol, P., and Stroud, I. (2018). STEP-NC digital thread for additive manufacturing: data model, implementation and validation. Int. J. Comput. Integr. Manuf. 31, 1141–1160. doi:10.1080/0951192X.2018.1509130
Bonnard, R., Hascoët, J.-Y., Mognol, P., Zancul, E., and Alvares, A. J. (2019b). Hierarchical object-oriented model (HOOM) for additive manufacturing digital thread. J. Manuf. Syst. 50, 36–52. doi:10.1016/j.jmsy.2018.11.003
Boring, R. L., Ulrich, T. A., Joe, J. C., and Lew, R. T. (2015). Guideline for operational nuclear usability and knowledge elicitation (GONUKE). 6th Int. Conf. Appl. Hum. Factors Ergon. AHFE 2015 Affil. Conf. AHFE 3, 1327–1334. doi:10.1016/j.promfg.2015.07.287
Borowski, P. F. (2021). Digitization, digital twins, blockchain, and industry 4.0 as elements of management process in enterprises in the energy sector. Energies 14, 1885. doi:10.3390/en14071885
Boschert, S., and Rosen, R. (2016). Digital twin—the simulation aspect. Mechatron. Futur. Chall. Solut. Mechatron. Syst. Their Des. 59–74.
Bourhis, F. L., Kerbrat, O., Hascoet, J.-Y., and Mognol, P. (2013). Sustainable manufacturing: evaluation and modeling of environmental impacts in additive manufacturing. Int. J. Adv. Manuf. Technol. 69, 1927–1939. doi:10.1007/s00170-013-5151-2
Bowman, D., Dwyer, L., Levers, A., Patterson, E. A., Purdie, S., and Vikhorev, K. (2022). A unified approach to digital twin architecture—proof-of-concept activity in the nuclear sector. IEEE Access 10, 44691–44709. doi:10.1109/access.2022.3161626
Brockhoff, T., Heithoff, M., Koren, I., Michael, J., Pfeiffer, J., Rumpe, B., et al. (2021). “Process prediction with digital twins,” in 2021 ACM/IEEE International Conference on Model Driven Engineering Languages and Systems Companion (MODELS-C), Fukuoka, Japan, 10-15 October 2021, 182–187.
Burchell, T., Fuller, E., Romanoski, G., and Strizak, J. (1991). Graphite for the nuclear industry. TN (USA): Oak Ridge National Lab.
Cannon, N., Biegalski, S., and Erickson, A. (2022). Additive manufacturing: a challenge to nuclear nonproliferation. J. Radioanal. Nucl. Chem. 331, 4997–5002. doi:10.1007/s10967-022-08599-1
Casalta, S., Daquino, G., Metten, L., Oudaert, J., and Van de Sande, A. (2003). Digital image analysis of X-ray and neutron radiography for the inspection and the monitoring of nuclear materials. Ndt E Int. 36, 349–355. doi:10.1016/s0963-8695(03)00008-2
Chen, S., Gao, H., Zhang, Y., Wu, Q., Gao, Z., and Zhou, X. (2022). Review on residual stresses in metal additive manufacturing: formation mechanisms, parameter dependencies, prediction and control approaches. J. Mat. Res. Technol. 17, 2950–2974. doi:10.1016/j.jmrt.2022.02.054
Christopher, G. (2015). 3D printing: a challenge to nuclear export controls. Strateg. Trade Rev. 1, 18–25.
Cimino, C., Negri, E., and Fumagalli, L. (2019). Review of digital twin applications in manufacturing. Comput. Ind. 113, 103130. doi:10.1016/j.compind.2019.103130
Cramer, C. L., Ionescu, E., Graczyk-Zajac, M., Nelson, A. T., Katoh, Y., Haslam, J. J., et al. (2022). Additive manufacturing of ceramic materials for energy applications: road map and opportunities. J. Eur. Ceram. Soc. 42, 3049–3088. doi:10.1016/j.jeurceramsoc.2022.01.058
Crooks, C., Evans, S., and Macchietto, S. (1994). An application of automated operating procedure synthesis in the nuclear industry. Comput. Chem. Eng. 18, S385–S389. doi:10.1016/0098-1354(94)80063-4
Daniel, O. (2020). Coming soon: a nuclear reactor—with a 3D-printed core. Available at: https://www.wired.com/story/coming-soon-a-nuclear-reactor-with-a-3d-printed-core/.
David, S., and Vitek, J. (1989). Correlation between solidification parameters and weld microstructures. Int. Mat. Rev. 34, 213–245. doi:10.1179/imr.1989.34.1.213
Deb, S. R., and Deb, S. (2010). Robotics technology and flexible automation. United States: McGraw-Hill Education.
De Backer, K., and DeStefano, T. (2021). “Robotics and the global organisation of production,” in Robotics, AI, and humanity. Editors J. Von Braun, M. S. Archer, G. M. Reichberg, and M. Sánchez Sorondo (Cham: Springer International Publishing), 71–84. doi:10.1007/978-3-030-54173-6_6
Debroy, T., Zhang, W., Turner, J., and Babu, S. S. (2017). Building digital twins of 3D printing machines. Scr. Mat. 135, 119–124. doi:10.1016/j.scriptamat.2016.12.005
Ding, D., Pan, Z., Cuiuri, D., and Li, H. (2015a). A multi-bead overlapping model for robotic wire and arc additive manufacturing (WAAM). Robot. Comput.-Integr. Manuf. 31, 101–110. doi:10.1016/j.rcim.2014.08.008
Ding, D., Pan, Z., Cuiuri, D., and Li, H. (2015b). Wire-feed additive manufacturing of metal components: technologies, developments and future interests. Int. J. Adv. Manuf. Technol. 81, 465–481. doi:10.1007/s00170-015-7077-3
Dixit, V., Xiong, Z., Jian, S., and Saxena, N. (2019). Risk of automated driving: implications on safety acceptability and productivity. Accid. Anal. Prev. 125, 257–266. doi:10.1016/j.aap.2019.02.005
Djuric, A. M., Urbanic, R., and Rickli, J. (2016). A framework for collaborative robot (CoBot) integration in advanced manufacturing systems. SAE Int. J. Mat. Manuf. 9, 457–464. doi:10.4271/2016-01-0337
Domning, E. E., McMahon, T. T., and Sievers, R. H. (1991). Robotic and nuclear safety for an automated/teleoperated glove box system. Livermore, California: Lawrence Livermore National Lab.
Dubovska, R., Jambor, J., and Majerik, J. (2014). Implementation of CAD/CAM system CATIA V5 in simulation of CNC machining process. Procedia Eng. 69, 638–645. doi:10.1016/j.proeng.2014.03.037
Dye, D., Hunziker, O., and Reed, R. (2001). Numerical analysis of the weldability of superalloys. Acta Mater 49, 683–697. doi:10.1016/s1359-6454(00)00361-x
Eckhart, M., and Ekelhart, A. (2019). Digital twins for cyber-physical systems security: state of the art and outlook. Secur. Qual. Cyber-Phys. Syst. Eng. Forewords Robert M. Lee Tom Gilb, 383–412.
El-Genk, M. S., and Tournier, J.-M. (2005). A review of refractory metal alloys and mechanically alloyed-oxide dispersion strengthened steels for space nuclear power systems. J. Nucl. Mat. 340, 93–112. doi:10.1016/j.jnucmat.2004.10.118
Eom, S., Riznic, J., Nitheanandan, T., and Kirkhope, K. (2023). “Regulatory and licensing challenges with Generation-IV nuclear energy systems,” in Handbook of generation IV nuclear reactors (Amsterdam, Netherlands: Elsevier), 837–864.
Evangeline, P., and Anandhakumar, (2020). “Digital twin technology for smart manufacturing,” in Advances in computers (Amsterdam, Netherlands: Elsevier), 35–49. doi:10.1016/bs.adcom.2019.10.009
Feng, J., Guo, W., Francis, J., Irvine, N., and Li, L. (2016). Narrow gap laser welding for potential nuclear pressure vessel manufacture. J. Laser Appl. 28, 022421. doi:10.2351/1.4943905
Findlay, T. (2010). Nuclear energy and global governance: ensuring safety, security and non-proliferation. England, UK: Routledge.
Foteinopoulos, P., Papacharalampopoulos, A., Angelopoulos, K., and Stavropoulos, P. (2020). Development of a simulation approach for laser powder bed fusion based on scanning strategy selection. Int. J. Adv. Manuf. Technol. 108, 3085–3100. doi:10.1007/s00170-020-05603-4
Foteinopoulos, P., Papacharalampopoulos, A., and Stavropoulos, P. (2018). On thermal modeling of Additive Manufacturing processes. CIRP J. Manuf. Sci. Technol. 20, 66–83. doi:10.1016/j.cirpj.2017.09.007
Francis, J., Holtum, N., Olschok, S., Roy, M., Vasileiou, A., Jakobs, S., et al. (2019). Vacuum laser welding of SA508 steel. J. Mat. Process. Technol. 274, 116269. doi:10.1016/j.jmatprotec.2019.116269
Free, Z., Hernandez, M., Mashal, M., and Mondal, K. (2021). A review on advanced manufacturing for hydrogen storage applications. Energies 14, 8513. doi:10.3390/en14248513
French, R., Marin-Reyes, H., and Benakis, M. (2019). “Transfer analysis of human engineering skills for adaptive robotic additive manufacturing in the aerospace repair and overhaul industry,” in Advances in manufacturing, production Management and process control advances in intelligent systems and computing. Editors W. Karwowski, S. Trzcielinski, B. Mrugalska, M. Di Nicolantonio, and E. Rossi (Cham: Springer International Publishing), 3–12. doi:10.1007/978-3-319-94196-7_1
French, R., Marin-Reyes, H., Kapellmann-Zafra, G., and Abrego-Hernandez, S. (2020). Development of an intelligent robotic additive manufacturing cell for the nuclear industry. Berlin, Germany: Springer, 3–13.
French, R., Marin-Reyes, H., Kapellmann-Zafra, G., and Abrego-Hernandez, S. (2023). Robotic Additive Manufacturing system for nuclear industry applications. Available at: https://www.shadowrobot.com/wp-content/uploads/2022/04/Robotic-Additive-Manufacturing-system-for-nuclear-industry-applications-Shadows-Modular-Grasper-Mentioned-2019.pdf.
Fuller, A., Fan, Z., Day, C., and Barlow, C. (2020). Digital twin: enabling technologies, challenges and open research. IEEE Access 8, 108952–108971. doi:10.1109/ACCESS.2020.2998358
Ghenai, C., Husein, L. A., Al Nahlawi, M., Hamid, A. K., and Bettayeb, M. (2022). Recent trends of digital twin technologies in the energy sector: a comprehensive review. Sustain. Energy Technol. Assess. 54, 102837. doi:10.1016/j.seta.2022.102837
Glaessgen, E., and Stargel, D. (2012). “The digital twin paradigm for future NASA and US Air Force vehicles,” in Paper for the 53rd Structures, Structural Dynamics, and Materials Conference, Honolulu, Hawaii, USA, 23-26 April 2012.
Gockel, B., Tudor, A., Brandyberry, M., Penmetsa, R., and Tuegel, E. (2012). “Challenges with structural life forecasting using realistic mission profiles,”, Honolulu, Hawaii, USA, 23-26 April 2012.
Gong, H., Cheng, S., Chen, Z., and Li, Q. (2022). Data-Enabled physics-informed machine learning for reduced-order modeling digital twin: application to nuclear reactor physics. Nucl. Sci. Eng. 196, 668–693. doi:10.1080/00295639.2021.2014752
Gong, H., Zhu, T., Chen, Z., Wan, Y., and Li, Q. (2023). Parameter identification and state estimation for nuclear reactor operation digital twin. Ann. Nucl. Energy 180, 109497. doi:10.1016/j.anucene.2022.109497
Gorse-Pomonti, D., and Russier, V. (2007). Liquid metals for nuclear applications. Liq. Amorph. Mater. XII 353, 3600–3614. doi:10.1016/j.jnoncrysol.2007.05.175
Grieves, M. (2015). Digital twin: manufacturing excellence through virtual factory replication. Cocoa Beach, FL, United States: Michael W. Grieves, LLC.
Grieves, M., and Vickers, J. (2017a). “Digital twin: mitigating unpredictable, undesirable emergent behavior in complex systems,” in Transdisciplinary perspectives on complex systems. Editors F.-J. Kahlen, S. Flumerfelt, and A. Alves (Cham: Springer International Publishing), 85–113. doi:10.1007/978-3-319-38756-7_4
Grieves, M., and Vickers, J. (2017b). Digital twin: mitigating unpredictable, undesirable emergent behavior in complex systems. Transdiscipl. Perspect. Complex Syst. New Find. Approaches, 85–113.
Guérin, Y., Was, G. S., and Zinkle, S. J. (2009). Materials challenges for advanced nuclear energy systems. Mrs Bull. 34, 10–19.
Gunasegaram, D. R., Murphy, A. B., Matthews, M., and DebRoy, T. (2021). The case for digital twins in metal additive manufacturing. J. Phys. Mat. 4, 040401. doi:10.1088/2515-7639/ac09fb
Guo, Y., Yan, A., and Wang, J. (2021). “Cyber security RiskAnalysis of physical protection systems of nuclear power plants and research on the cyber security test platform using digital twin technology,” in 2021 International Conference on Power System Technology (POWERCON), Haikou, China, 08-09 December 2021, 1889–1892.
Haag, S., and Anderl, R. (2018). Digital twin – proof of concept. Ind. 40 Smart Manuf. 15, 64–66. doi:10.1016/j.mfglet.2018.02.006
Harper, E. M., Diao, Z., Panousi, S., Nuss, P., Eckelman, M. J., and Graedel, T. E. (2015). The criticality of four nuclear energy metals. Resour. Conserv. Recycl. 95, 193–201. doi:10.1016/j.resconrec.2014.12.009
Haslam, J., Kelly, J., and Harris, R. (2022). Predictive models for ceramic additive manufacturing, CRADA No. TC02251. Livermore, CA (United States): Lawrence Livermore National Lab.
He, Y., Huang, D., Chen, L., Ni, Y., and Ma, X. (2022). A survey on Zero trust architecture: challenges and future trends. Wirel. Commun. Mob. Comput. 2022, 1–13. doi:10.1155/2022/6476274
Hehr, A., Wenning, J., Terrani, K., Babu, S. S., and Norfolk, M. (2017). Five-axis ultrasonic additive manufacturing for nuclear component manufacture. Jom 69, 485–490. doi:10.1007/s11837-016-2205-6
Holshouser, C., Newell, C., Palas, S., Duty, C., Love, L., Kunc, V., et al. (2013). Out of bounds additive manufacturing. AMP Tech. Artic. 171, 15–17. doi:10.31399/asm.amp.2013-03.p015
Hu, H., Wang, Q., Qin, J., Wu, Y., Zhang, T., Xie, Z., et al. (2008). Study on composite material for shielding mixed neutron and $\gamma$-Rays. IEEE Trans. Nucl. Sci. 55, 2376–2384. doi:10.1109/TNS.2008.2000800
Huang, S. H., Liu, P., Mokasdar, A., and Hou, L. (2013). Additive manufacturing and its societal impact: a literature review. Int. J. Adv. Manuf. Technol. 67, 1191–1203. doi:10.1007/s00170-012-4558-5
Huang, Y., Leu, M. C., Mazumder, J., and Donmez, A. (2015). Additive manufacturing: current state, future potential, gaps and needs, and recommendations. J. Manuf. Sci. Eng. 137. doi:10.1115/1.4028725
Huang, Z., Fey, M., Liu, C., Beysel, E., Xu, X., and Brecher, C. (2023). Hybrid learning-based digital twin for manufacturing process: modeling framework and implementation. Robot. Comput.-Integr. Manuf. 82, 102545. doi:10.1016/j.rcim.2023.102545
Hyvärinen, J., Vihavainen, J., Ylönen, M., and Valkonen, J. (2022). An overall safety concept for nuclear power plants. Ann. Nucl. Energy 178, 109353. doi:10.1016/j.anucene.2022.109353
Ibarra, A., Perlado, M., Aracil, R., Blanco, D., Ferre, M., García-Cortés, I., et al. (2010). TechnoFusión, a relevant facility for fusion technologies: the remote handling area. Proc. Ninth Int. Symp. Fusion Nucl. Technol. 85, 1659–1663. doi:10.1016/j.fusengdes.2010.05.010
Ion, J. C., Easterling, K. E., and Ashby, M. F. (1984). A second report on diagrams of microstructure and hardness for heat-affected zones in welds. Acta Metall. 32, 1949–1962. doi:10.1016/0001-6160(84)90176-7
Iqbal, J., Tahir, A. M., and ul Islam, R. (2012). “Robotics for nuclear power plants—challenges and future perspectives,” in 2012 2nd International Conference on Applied Robotics for the Power Industry (CARPI), Zurich, Switzerland, 11-13 September 2012, 151–156.
Jackson, R., Curran, S., Chambon, P., Post, B., Love, L., Wagner, R., et al. (2016). Overview of the Oak Ridge National Laboratory Advanced Manufacturing Integrated Energy Demonstration Project: case study of additive manufacturing as a tool to enable rapid innovation in integrated energy systems. New York City, U.S: American Society of Mechanical Engineers.
Jain, R., Agrawal, D., Vishwakarma, S., Choubey, A., Upadhyaya, B., and Oak, S. (2010). Development of underwater laser cutting technique for steel and zircaloy for nuclear applications. Pramana 75, 1253–1258. doi:10.1007/s12043-010-0214-5
Javaid, M., Haleem, A., Singh, R. P., and Suman, R. (2021). Substantial capabilities of robotics in enhancing industry 4.0 implementation. Cogn. Robot. 1, 58–75. doi:10.1016/j.cogr.2021.06.001
Jharko, E., Meshcheryakov, R., and Promyslov, V. (2021). “Aspects of nuclear power plant digital decommissioning,” in 2021 International Siberian Conference on Control and Communications (SIBCON), Kazan, Russia, November 17 – 19, 2022. doi:10.1109/SIBCON50419.2021.9438885
Jywe, W., Hsu, T.-H., and Liu, C.-H. (2012). Non-bar, an optical calibration system for five-axis CNC machine tools. Int. J. Mach. Tools Manuf. 59, 16–23. doi:10.1016/j.ijmachtools.2012.01.004
Kaber, D. B., and Endsley, M. R. (2004). The effects of level of automation and adaptive automation on human performance, situation awareness and workload in a dynamic control task. Theor. Issues Ergon. Sci. 5, 113–153. doi:10.1080/1463922021000054335
Kanari, M., Tanaka, K., Baba, S., and Eto, M. (1997). Nanoindentation behavior of a two-dimensional carbon-carbon composite for nuclear applications. Carbon 35, 1429–1437. doi:10.1016/s0008-6223(97)00042-0
Kang, H. T., Dong, P., and Hong, J. K. (2007). Fatigue analysis of spot welds using a mesh-insensitive structural stress approach. Int. J. Fatigue 29, 1546–1553. doi:10.1016/j.ijfatigue.2006.10.025
Kashkarov, E., Afornu, B., Sidelev, D., Krinitcyn, M., Gouws, V., and Lider, A. (2021). Recent advances in protective coatings for accident tolerant Zr-based fuel claddings. Coatings 11, 557. doi:10.3390/coatings11050557
Kaur, I., and Singh, P. (2021). State-of-the-art in heat exchanger additive manufacturing. Int. J. Heat. Mass Transf. 178, 121600. doi:10.1016/j.ijheatmasstransfer.2021.121600
Kayacan, M. Y., Özsoy, K., Duman, B., Yilmaz, N., and Kayacan, M. C. (2019). A study on elimination of failures resulting from layering and internal stresses in Powder Bed Fusion (PBF) additive manufacturing. Mat. Manuf. Process. 34, 1467–1475. doi:10.1080/10426914.2019.1655151
Kim, H.-G., Kim, I.-H., Jung, Y.-I., Park, D.-J., Park, J.-Y., and Koo, Y.-H. (2015). Adhesion property and high-temperature oxidation behavior of Cr-coated Zircaloy-4 cladding tube prepared by 3D laser coating. J. Nucl. Mat. 465, 531–539. doi:10.1016/j.jnucmat.2015.06.030
Kirk, S., Keogh, K., Suder, W., Tremethick, T., Allen, C., and Farquhar, I. (2018). “Remote in-bore laser cutting and welding tools for use in future nuclear fusion reactors,” in Waste Management Symposia, 18–22.
Kirkaldy, J. (1991). Diffusion-controlled phase transformations in steels Theory and applications. Scand. J. Metall. 20, 50–61.
Knapp, G., Mukherjee, T., Zuback, J., Wei, H., Palmer, T., De, A., et al. (2017). Building blocks for a digital twin of additive manufacturing. Acta Mater 135, 390–399. doi:10.1016/j.actamat.2017.06.039
Kobayashi, K., Daniell, J., Usman, S., Kumar, D., and Alam, S. (2022). Surrogate modeling-driven physics-informed multi-fidelity kriging: path forward to digital twin enabling simulation for accident tolerant fuel. ArXiv Prepr. ArXiv221007164.
Kochunas, B., and Huan, X. (2021). Digital twin concepts with uncertainty for nuclear power applications. Energies 14, 4235. doi:10.3390/en14144235
Kofman, J., Wu, X., Luu, T. J., and Verma, S. (2005). Teleoperation of a robot manipulator using a vision-based human-robot interface. IEEE Trans. Ind. Electron. 52, 1206–1219. doi:10.1109/tie.2005.855696
Kohyama, A., and Kishimoto, H. (2013). SiC/SiC composite materials for nuclear applications. Int. Electron. J. Nucl. Saf. Simul. 4.
Konings, R., and Stoller, R. E. (2020). Comprehensive nuclear materials. Amsterdam, Netherlands: Elsevier.
Koyanagi, T., Terrani, K., Harrison, S., Liu, J., and Katoh, Y. (2021). Additive manufacturing of silicon carbide for nuclear applications. J. Nucl. Mat. 543, 152577. doi:10.1016/j.jnucmat.2020.152577
Kropaczek, D. J., Badalassi, V., Jain, P. K., Ramuhalli, P., and Pointer, W. D. (2023). “Digital twins for nuclear power plants and facilities,” in The digital twin (Berlin, Germany: Springer), 971–1022.
Lai, G. Y. (1990). High temperature corrosion of engineering alloys. United States. Available at: https://www.osti.gov/biblio/5465166.
Langlotz, P., Klar, M., Yi, L., Hussong, M., Sousa, F. J. P., and Aurich, J. C. (2022). Concept of hybrid modeled digital twins and its application for an energy management of manufacturing systems. Procedia CIRP 112, 549–554. doi:10.1016/j.procir.2022.09.098
Lee, J., Cameron, I., and Hassall, M. (2019). Improving process safety: what roles for Digitalization and Industry 4.0? Process Saf. Environ. Prot. 132, 325–339. doi:10.1016/j.psep.2019.10.021
Lenzen, M. (2008). Life cycle energy and greenhouse gas emissions of nuclear energy: a review. Energy Convers. Manag. 49, 2178–2199. doi:10.1016/j.enconman.2008.01.033
Li, H., Fu, X., Zhang, L., Zhang, Y., Jiang, L., and Pu, Z. (2022). A review of the latest developments in the field of additive manufacturing techniques for nuclear reactors. Crystals 12, 918. doi:10.3390/cryst12070918
Li, W., Peng, M., Liu, Y., Jiang, N., Wang, H., and Duan, Z. (2018). Fault detection, identification and reconstruction of sensors in nuclear power plant with optimized PCA method. Ann. Nucl. Energy 113, 105–117. doi:10.1016/j.anucene.2017.11.009
Little, E. A. (2006). Development of radiation resistant materials for advanced nuclear power plant. Mat. Sci. Technol. 22, 491–518. doi:10.1179/174328406X90998
Liu, C., Le Roux, L., Körner, C., Tabaste, O., Lacan, F., and Bigot, S. (2022). Digital twin-enabled collaborative data management for metal additive manufacturing systems. J. Manuf. Syst. 62, 857–874. doi:10.1016/j.jmsy.2020.05.010
Liu, M., Fang, S., Dong, H., and Xu, C. (2021). Review of digital twin about concepts, technologies, and industrial applications. J. Manuf. Syst. 58, 346–361. doi:10.1016/j.jmsy.2020.06.017
Lopez, A. B., Assuncao, E., Quintino, L., Blackburn, J., and Khan, A. (2017). High-power fiber laser cutting parameter optimization for nuclear decommissioning. Nucl. Eng. Technol. 49, 865–872. doi:10.1016/j.net.2017.02.004
Losey, D. P., McDonald, C. G., Battaglia, E., and O’Malley, M. K. (2018). A review of intent detection, arbitration, and communication aspects of shared control for physical human–robot interaction. Appl. Mech. Rev. 70. doi:10.1115/1.4039145
Lou, X., and Gandy, D. (2019). Advanced manufacturing for nuclear energy. JOM 71, 2834–2836. doi:10.1007/s11837-019-03607-4
Love, L. (2015). Utility of big area additive manufacturing (BAAM) for the rapid manufacture of customized electric vehicles. Oak Ridge, TN (United States): Oak Ridge National Lab. doi:10.2172/1209199
Love, L. J., Duty, C. E., Post, B. K., Lind, R. F., Lloyd, P. D., Kunc, V., et al. (2015). Breaking barriers in polymer additive manufacturing. Oak Ridge, TN (United States): Oak Ridge National Lab.
Lu, J., Sabharwall, P., Heidrich, B., and Christensen, R. (2020). Advanced manufacturing and instrumentation for neutron chopper design. J. Young Investig. 38.
Mamalis, A. (2005a). Advanced manufacturing of advanced materials. Berlin, Germany: Springer, 63–80.
Mamalis, A. G. (2005b). Advanced manufacturing engineering. J. Mat. Process. Technol. 161, 1–9. doi:10.1016/j.jmatprotec.2004.07.055
Mandolla, C., Petruzzelli, A. M., Percoco, G., and Urbinati, A. (2019). Building a digital twin for additive manufacturing through the exploitation of blockchain: a case analysis of the aircraft industry. Comput. Ind. 109, 134–152. doi:10.1016/j.compind.2019.04.011
Marra, J. (2011). Advanced ceramic materials for next-generation nuclear applications. United Kingdom: IOP Publishing.162001
Matějíček, J., and Chráska, P. (2011). Development of advanced coatings for ITER and future fusion devices. Adv. Sci. Technol. 66, 47–65.
McAffee, D., Raczka, N., and Schwartztrauber, K. (1997). Robotics and remote handling concepts for disposal of high-level nuclear waste. Las Vegas, NV. Yucca Mountain Project (US): Morrison Knudsen Corp.
McMurtrey, M., and Messner, M. (2021). “Qualification challenges for additive manufacturing in high temperature nuclear applications,” in Materials and fabrication (New York City, U.S: American Society of Mechanical Engineers), Vol. 4. doi:10.1115/PVP2021-62331
Meisel, N. A., Watson, N., Bilén, S. G., Duarte, J. P., and Nazarian, S. (2022). Design and system considerations for construction-scale concrete additive manufacturing in remote environments via robotic arm deposition. 3D Print. Addit. Manuf. 9, 35–45. doi:10.1089/3dp.2020.0335
Milewski, J. O., and Milewski, J. O. (2017). Additive manufacturing metal, the art of the possible. Berlin, Germany: Springer.
Mohee, F., and Kamal, F. (2017). Application of high-strength composite materials for the nuclear waste containers and flasks. in.
Mondal, K., Fujimoto, K., and McMurtrey, M. D. (2020a). Advanced manufacturing of printed melt wire chips for cheap, compact passive in-pile temperature sensors. JOM 72, 4196–4201. doi:10.1007/s11837-020-04426-8
Mondal, K., Karkkainen, R., Martinez, O., Sikkema, I., Mathur, M., Hollifield, S., et al. (2023). Advanced manufacturing technologies for enhancing security in nuclear and radiological materials transport. Int. J. Adv. Nucl. React. Des. Technol. 5, 144–150. doi:10.1016/j.jandt.2024.01.001
Mondal, K., Liu, Y., Shay, T., Genzer, J., and Dickey, M. D. (2020b). Application of a laser cutter to pattern wrinkles on polymer films. ACS Appl. Polym. Mat. 2, 1848–1855. doi:10.1021/acsapm.0c00061
Mondal, K., and McMurtrey, M. D. (2020). Present status of the functional advanced micro-nano-printings – a mini review. Mat. Today Chem. 17, 100328. doi:10.1016/j.mtchem.2020.100328
Mondal, K., Nuñez, L., Downey, C. M., and van Rooyen, I. J. (2021b). Recent advances in the thermal barrier coatings for extreme environments. Mat. Sci. Energy Technol. 4, 208–210. doi:10.1016/j.mset.2021.06.006
Mondal, K., Nuñez, L., Downey, C. M., and Van Rooyen, I. J. (2021a). Thermal barrier coatings overview: design, manufacturing, and applications in high-temperature industries. Ind. Eng. Chem. Res. 60, 6061–6077. doi:10.1021/acs.iecr.1c00788
Mondal, K., and Tripathy, P. K. (2021). Preparation of smart materials by additive manufacturing technologies: a review. Materials 14, 6442. doi:10.3390/ma14216442
Moschetti, M., Burr, P. A., Obbard, E., Kruzic, J. J., Hosemann, P., and Gludovatz, B. (2022). Design considerations for high entropy alloys in advanced nuclear applications. J. Nucl. Mat. 567, 153814. doi:10.1016/j.jnucmat.2022.153814
Mu, H., He, F., Yuan, L., Commins, P., Wang, H., and Pan, Z. (2023). Toward a smart wire arc additive manufacturing system: a review on current developments and a framework of digital twin. J. Manuf. Syst. 67, 174–189. doi:10.1016/j.jmsy.2023.01.012
Muir, B. M. (1994). Trust in automation: Part I. Theoretical issues in the study of trust and human intervention in automated systems. Ergonomics 37, 1905–1922. doi:10.1080/00140139408964957
Mukherjee, T., and DebRoy, T. (2019). A digital twin for rapid qualification of 3D printed metallic components. Appl. Mat. Today 14, 59–65. doi:10.1016/j.apmt.2018.11.003
Neelamkavil, J. (2009). “Automation in the prefab and modular construction industry,” in 26th International Symposium on Automation and Robotics in Construction (ISARC 2009), Austin, Texas, United States, June 24-27, 2009.
Nelson, A. T. (2023). Prospects for additive manufacturing of nuclear fuel forms. Prog. Nucl. Energy 155, 104493. doi:10.1016/j.pnucene.2022.104493
Nelson, W., and Haney, L. (1993). Development of a framework of human-centered automation for the nuclear industry. Idaho Falls, ID (United States): EG and G Idaho, Inc.
Ngo, T. D., Kashani, A., Imbalzano, G., Nguyen, K. T., and Hui, D. (2018). Additive manufacturing (3D printing): a review of materials, methods, applications and challenges. Compos. Part B Eng. 143, 172–196. doi:10.1016/j.compositesb.2018.02.012
Niu, X., and Qin, S. (2021). Integrating crowd-/service-sourcing into digital twin for advanced manufacturing service innovation. Adv. Eng. Inf. 50, 101422. doi:10.1016/j.aei.2021.101422
Nof, S. Y. (2009). Automation: what it means to us around the world. Cham: Springer Handb. Autom., 13–52.
Nuclear reactor components 3D printed by ORNL now installed at TVA Browns Ferry nuclear plant (2021). Nuclear reactor components 3D printed by ORNL now installed at TVA Browns Ferry nuclear plant. Available at: https://www.ornl.gov/news/nuclear-reactor-components-3d-printed-ornl-now-installed-tva-browns-ferry-nuclear-plant.
Oak Ridge developing 3D-printed nuclear reactor core (2020). Oak Ridge developing 3D-printed nuclear reactor core. Available at: https://www.ans.org/news/article-179/oak-ridge-developing-3dprinted-nuclear-reactor-core/.
Ostaševičius, V. (2022). Digital twins in manufacturing: virtual and physical twins for advanced manufacturing. Cham: Springer International Publishing. doi:10.1007/978-3-030-98275-1
Pal, S. K., Mishra, D., Pal, A., Dutta, S., Chakravarty, D., and Pal, S. (2022). Digital twin – fundamental concepts to applications in advanced manufacturing. Cham: Springer International Publishing. doi:10.1007/978-3-030-81815-9
Panda, A., Dobránsky, J., Jančík, M., Pandova, I., and Kačalová, M. (2018). Advantages and effectiveness of the powder metallurgy in manufacturing technologies. Metalurgija 57, 353–356.
Pantelidakis, M., Mykoniatis, K., Liu, J., and Harris, G. (2022). A digital twin ecosystem for additive manufacturing using a real-time development platform. Int. J. Adv. Manuf. Technol. 120, 6547–6563. doi:10.1007/s00170-022-09164-6
Park, K. T., Lee, J., Kim, H.-J., and Noh, S. D. (2020). Digital twin-based cyber physical production system architectural framework for personalized production. Int. J. Adv. Manuf. Technol. 106, 1787–1810. doi:10.1007/s00170-019-04653-7
Patel, J., and Kilic, G. (2021). “A review on advanced manufacturing techniques and their applications,” in Computational optimization techniques and applications. Editors M. Sarfraz,, and S. Ariffin Abdul Karim (London, UK: IntechOpen). doi:10.5772/intechopen.97702
Phua, A., Davies, C. H. J., and Delaney, G. W. (2022). A digital twin hierarchy for metal additive manufacturing. Comput. Ind. 140, 103667. doi:10.1016/j.compind.2022.103667
Pickering, E. J., Carruthers, A. W., Barron, P. J., Middleburgh, S. C., Armstrong, D. E. J., and Gandy, A. S. (2021). High-Entropy alloys for advanced nuclear applications. Entropy 23, 98. doi:10.3390/e23010098
Prakash, D., Purohit, R., and Sinha, P. (2015). Advanced ceramics for nuclear heat utilization and energy harvesting. in.
Qing-yuan, M., Pei-nan, D., Ying-bo, P., Rui-qian, Z., and Wei, Z. (2022). Application and development of metal additive manufacturing technology in the field of nuclear industry. 粉末冶金技术 40, 86–94.
Rahman, M., Khan, A., Anowar, S., Al-Imran, M., Verma, R., Kumar, D., et al. (2022). Leveraging industry 4.0--deep learning, surrogate model and transfer learning with uncertainty quantification incorporated into digital twin for nuclear system. ArXiv Prepr. ArXiv221000074.
Rahmatullah, R., Amiruddin, A., and Lubis, S. (2021). Effectiveness of CNC turning and CNC milling in machining process. Int. J. Econ. Technol. Soc. Sci. Injects 2, 575–583. doi:10.53695/injects.v2i2.610
Raj, B., Vijayalakshmi, M., Rao, P. V., and Rao, K. (2008). Challenges in materials research for sustainable nuclear energy. Mrs Bull. 33, 327–337. doi:10.1557/mrs2008.67
Rajagopalan, C., and Venugopal, S. (2013). Automation, robotics and remote handling technology in the nuclear fuel cycle. Kovilpatti (India): Society for Manufacturing Engineers, National Engineering College.
Rajawat, A. S., Rawat, R., Barhanpurkar, K., Shaw, R. N., and Ghosh, A. (2021). “Robotic process automation with increasing productivity and improving product quality using artificial intelligence and machine learning,” in Artificial intelligence for future generation robotics (Amsterdam, Netherlands: Elsevier), 1–13.
Revuelta, A., Riipinen, T., Siren, M., Vaajoki, A., Que, Z., Jayaprakash, S., et al. (2021). “Additive manufacturing in nuclear power plants (AM-NPP),” in SAFIR2022-The Finnish research programme on nuclear power plant safety 2019-2022 (VTT), 318–328. doi:10.32040/2242-122X.2021.T383
Ritter, C., Hays, R., Browning, J., Stewart, R., Bays, S., Reyes, G., et al. (2022). Digital twin to detect nuclear proliferation: a case study. J. Energy Resour. Technol. 144, 102108. doi:10.1115/1.4053979
Roy, R. B., Mishra, D., Pal, S. K., Chakravarty, T., Panda, S., Chandra, M. G., et al. (2020). Digital twin: current scenario and a case study on a manufacturing process. Int. J. Adv. Manuf. Technol. 107, 3691–3714. doi:10.1007/s00170-020-05306-w
Sandler, B.-Z. (1999). Robotics: designing the mechanisms for automated machinery. United States: Academic Press.
Sauder, C. (2014). “Ceramic matrix composites: nuclear applications,” in Ceramic matrix composites. Editors N. P. Bansal,, and J. Lamon (Hoboken, NJ, USA: John Wiley and Sons, Inc.), 609–646. doi:10.1002/9781118832998.ch22
Savall, J., Avello, A., and Briones, L. (1999). “Two compact robots for remote inspection of hazardous areas in nuclear power plants,” in Proceedings 1999 IEEE International Conference on Robotics and Automation, Detroit, MI, USA, 10-15 May 1999, 1993–1998.
Schneider, R. (2014). Robotics and automation can save your factory. Available at: https://www.productivity.com/wp-content/uploads/2015/04/Article-Robotics-and-Automation-Can-Save-Your-Factory.pdf.
Scime, L., Haley, J., Halsey, W., Singh, A., Sprayberry, M., Ziabari, A., et al. (2020). Report on Progress of correlation of in-situ and ex-situ data and the use of artificial intelligence to predict defects. Oak Ridge, TN (United States): Oak Ridge National Lab. doi:10.2172/1684671
Scime, L., Singh, A., and Paquit, V. (2022). A scalable digital platform for the use of digital twins in additive manufacturing. Manuf. Lett. 31, 28–32. doi:10.1016/j.mfglet.2021.05.007
Scime, L., Sprayberry, M., Collins, D., Singh, A., Joslin, C., Duncan, R., et al. (2021). Diagnostic and predictive capabilities of the TCR digital platform. Oak Ridge, TN (United States): Oak Ridge National Lab. doi:10.2172/1831630
Shafto, M., Conroy, M., Doyle, R., Glaessgen, E., Kemp, C., LeMoigne, J., et al. (2012). Modeling, simulation, information technology and processing roadmap. Natl. Aeronaut. Space Adm. 32, 1–38.
Shirizadeh, B., and Quirion, P. (2021). Low-carbon options for the French power sector: what role for renewables, nuclear energy and carbon capture and storage? Energy Econ. 95, 105004. doi:10.1016/j.eneco.2020.105004
Shirzadi, A., and Jackson, S. (eds) (2014). Structural alloys for power plants: operational challenges and high-temperature materials. doi:10.1016/C2013-0-16201-0
Simpson, J., Haley, J., Cramer, C., Shafer, O., Elliott, A., Peter, W., et al. (2019). Considerations for application of additive manufacturing to nuclear reactor core components. Oak Ridge, TN (United States): Oak Ridge National Lab.
Singh, B., Sellappan, N., and Kumaradhas, P. (2013). Evolution of industrial robots and their applications. Int. J. Emerg. Technol. Adv. Eng. 3, 763–768.
Singh, M., Fuenmayor, E., Hinchy, E., Qiao, Y., Murray, N., and Devine, D. (2021). Digital twin: origin to future. Appl. Syst. Innov. 4, 36. doi:10.3390/asi4020036
Singh, M., Srivastava, R., Fuenmayor, E., Kuts, V., Qiao, Y., Murray, N., et al. (2022). Applications of digital twin across industries: a review. Appl. Sci. 12, 5727. doi:10.3390/app12115727
Sleiti, A. K., Kapat, J. S., and Vesely, L. (2022). Digital twin in energy industry: proposed robust digital twin for power plant and other complex capital-intensive large engineering systems. Energy Rep. 8, 3704–3726. doi:10.1016/j.egyr.2022.02.305
Song, H., Song, M., and Liu, X. (2022). Online autonomous calibration of digital twins using machine learning with application to nuclear power plants. Appl. Energy 326, 119995. doi:10.1016/j.apenergy.2022.119995
Song, J., Chew, Y., Bi, G., Yao, X., Zhang, B., Bai, J., et al. (2018). Numerical and experimental study of laser aided additive manufacturing for melt-pool profile and grain orientation analysis. Mat. Des. 137, 286–297. doi:10.1016/j.matdes.2017.10.033
Stavropoulos, P., Bikas, H., Avram, O., Valente, A., and Chryssolouris, G. (2020). Hybrid subtractive–additive manufacturing processes for high value-added metal components. Int. J. Adv. Manuf. Technol. 111, 645–655. doi:10.1007/s00170-020-06099-8
Stavropoulos, P., Foteinopoulos, P., Papacharalampopoulos, A., and Bikas, H. (2018). Addressing the challenges for the industrial application of additive manufacturing: towards a hybrid solution. Int. J. Lightweight Mat. Manuf. 1, 157–168. doi:10.1016/j.ijlmm.2018.07.002
Stornelli, A., Ozcan, S., and Simms, C. (2021). Advanced manufacturing technology adoption and innovation: a systematic literature review on barriers, enablers, and innovation types. Res. Policy 50, 104229. doi:10.1016/j.respol.2021.104229
Stournaras, A., Stavropoulos, P., Salonitis, K., and Chryssolouris, G. (2009). An investigation of quality in CO2 laser cutting of aluminum. CIRP J. Manuf. Sci. Technol. 2, 61–69. doi:10.1016/j.cirpj.2009.08.005
Sun, C., Wang, Y., McMurtrey, M. D., Jerred, N. D., Liou, F., and Li, J. (2021). Additive manufacturing for energy: a review. Appl. Energy 282, 116041. doi:10.1016/j.apenergy.2020.116041
Sun, Z., and Ion, J. (1995). Laser welding of dissimilar metal combinations. J. Mat. Sci. 30, 4205–4214. doi:10.1007/bf00361499
Sundar, G. S., Sivaramakrishnan, R., and Venugopal, S. (2012). Design and developments of inspection robots in nuclear environment: a review. Int. J. Mech. Eng. Rob. Res. 1, 400–409.
Svetlizky, D., Das, M., Zheng, B., Vyatskikh, A. L., Bose, S., Bandyopadhyay, A., et al. (2021). Directed energy deposition (DED) additive manufacturing: physical characteristics, defects, challenges and applications. Mat. Today 49, 271–295. doi:10.1016/j.mattod.2021.03.020
Tamura, K., Ishigami, R., and Yamagishi, R. (2016). Laser cutting of thick steel plates and simulated steel components using a 30 kW fiber laser. J. Nucl. Sci. Technol. 53, 916–920. doi:10.1080/00223131.2015.1080633
Tang, Y., Yang, S., and Zhao, Y. F. (2016). Sustainable design for additive manufacturing through functionality integration and part consolidation. Handb. Sustain. Addit. Manuf. 1, 101–144.
Tao, F., Zhang, H., Liu, A., and Nee, A. Y. (2018). Digital twin in industry: state-of-the-art. IEEE Trans. Ind. Inf. 15, 2405–2415. doi:10.1109/tii.2018.2873186
Teizer, J., Cheng, T., and Fang, Y. (2013). Location tracking and data visualization technology to advance construction ironworkers’ education and training in safety and productivity. Autom. Constr. 35, 53–68. doi:10.1016/j.autcon.2013.03.004
Terrani, K. A., Babu, S. S., Bryan, C., Kiggans, J. O., Pinkston, D. L., Sridharan, N., et al. (2015). Demonstration of advanced manufacturing techniques for production of nuclear core structures: ultrasonic additive manufacturing of hybrid structures resembling HFIR control plates. Oak Ridge, TN (United States): Oak Ridge National Lab.
Thelen, A., Zhang, X., Fink, O., Lu, Y., Ghosh, S., Youn, B. D., et al. (2022). A comprehensive review of digital twin — part 1: modeling and twinning enabling technologies. Struct. Multidiscip. Optim. 65, 354. doi:10.1007/s00158-022-03425-4
Tsitsimpelis, I., Taylor, C. J., Lennox, B., and Joyce, M. J. (2019). A review of ground-based robotic systems for the characterization of nuclear environments. Prog. Nucl. Energy 111, 109–124. doi:10.1016/j.pnucene.2018.10.023
Tuegel, E. (2012). “The airframe digital twin: some challenges to realization,” in 54th AIAA/ASME/ASCE/AHS/ASC Structures, Structural Dynamics, and Materials Conference, Boston, Massachusetts, April 8-11, 2013.
Tuegel, E. J., Ingraffea, A. R., Eason, T. G., and Spottswood, S. M. (2011). Reengineering aircraft structural life prediction using a digital twin. Int. J. Aerosp. Eng. 2011, 1–14. doi:10.1155/2011/154798
Van Rooyen, I. J., and Morrell, S. R. (2020). Methods of forming structures and fissile fuel materials by additive manufacturing. Idaho Falls, ID (United States): Idaho National Laboratory.
Varé, C., and Morilhat, P. (2020). Digital twins, a new step for long term operation of nuclear power plants. Berlin, Germany: Springer, 96–103.
Vitanov, I., Farkhatdinov, I., Denoun, B., Palermo, F., Otaran, A., Brown, J., et al. (2021). A suite of robotic solutions for nuclear waste decommissioning. Robotics 10, 112. doi:10.3390/robotics10040112
Volodin, V. S., and Tolokonskii, A. O. (2019). Concept of instrumentation of digital twins of nuclear power plants units as observers for digital NPP I&C system. J. Phys. Conf. Ser. 1391, 012083. doi:10.1088/1742-6596/1391/1/012083
Wan, H., Song, D., Shi, X., Cai, Y., Li, T., and Chen, C. (2021). Corrosion behavior of Al0. 4CoCu0. 6NiSi0. 2Ti0. 25 high-entropy alloy coating via 3D printing laser cladding in a sulphur environment. J. Mat. Sci. Technol. 60, 197–205. doi:10.1016/j.jmst.2020.07.001
Wang, L. P., Huang, Y., Huang, Z., and Zhang, W. W. (2009). The development of large high efficient CNC gantry grinder for vessel head in nuclear power. Key Eng. Mat. 416, 421–425. doi:10.4028/www.scientific.net/KEM.416.421
Wang, Y., Ren, W., Li, Y., and Zhang, C. (2021). Complex product manufacturing and operation and maintenance integration based on digital twin. Int. J. Adv. Manuf. Technol. 117, 361–381. doi:10.1007/s00170-021-07350-6
Was, G. S., Petti, D., Ukai, S., and Zinkle, S. (2019). Materials for future nuclear energy systems. J. Nucl. Mat. 527, 151837. doi:10.1016/j.jnucmat.2019.151837
Watkins, T., Bilheux, H., An, K., Payzant, A., Dehoff, R., Duty, C., et al. (2013). Neutron characterization for additive manufacturing. Adv. Mat. 171, 23–27. doi:10.31399/asm.amp.2013-03.p023
Whittle, K. (2016). Nuclear materials science. United States: IOP Publishing. doi:10.1088/978-0-7503-1104-5
Wilson, M. T. (1983). Remote handling and accelerators. IEEE Trans. Nucl. Sci. 30, 2138–2141. doi:10.1109/tns.1983.4332741
Won, K., Han, J., and Bonne, A. (1997). Radioactive waste disposal: global experience and challenges. Iaea Bull. 39, 33–41.
Wright, L., and Davidson, S. (2020). How to tell the difference between a model and a digital twin. Adv. Model. Simul. Eng. Sci. 7, 13. doi:10.1186/s40323-020-00147-4
Wrigley, P., Wood, P., O’Neill, S., Hall, R., and Robertson, D. (2021). Off-site modular construction and design in nuclear power: a systematic literature review. Prog. Nucl. Energy 134, 103664. doi:10.1016/j.pnucene.2021.103664
Xiong, M., Wang, H., Fu, Q., and Xu, Y. (2021). Digital twin–driven aero-engine intelligent predictive maintenance. Int. J. Adv. Manuf. Technol. 114, 3751–3761. doi:10.1007/s00170-021-06976-w
Xu, Q., Zhou, G., Zhang, C., Chang, F., Huang, Q., Zhang, M., et al. (2022). Digital twin-driven intelligent maintenance decision-making system and key-enabling technologies for nuclear power equipment. Digit. Twin 2, 14. doi:10.12688/digitaltwin.17695.1
Yadav, V., Agarwal, V., Jain, P., Ramuhalli, P., Zhao, X., Ulmer, C., et al. (2023). Technical challenges and gaps in integration of advanced sensors, instrumentation, and communication technologies with digital twins for nuclear application. Oak Ridge, TN, United States: Oak Ridge National Laboratory (ORNL). doi:10.13182/NPICHMIT23-41192
Yang, Y., Babu, S., Orth, F., and Peterson, W. (2008). Integrated computational model to predict mechanical behaviour of spot weld. Sci. Technol. Weld. Join. 13, 232–239. doi:10.1179/174329308x283901
Yano, T., and Matović, B. (2013). “Handbook of advanced ceramics,” in Advanced ceramics for nuclear applications (Amsterdam, Netherlands: Elsevier Inc.). Chapter 4.3.
Yilbas, B. (2001). Effect of process parameters on the kerf width during the laser cutting process. Proc. Inst. Mech. Eng. Part B J. Eng. Manuf. 215, 1357–1365. doi:10.1243/0954405011519132
Yuan, L., Ding, D., Pan, Z., Yu, Z., Wu, B., Van Duin, S., et al. (2020). Application of multidirectional robotic wire arc additive manufacturing process for the fabrication of complex metallic parts. IEEE Trans. Ind. Inf. 16, 454–464. doi:10.1109/TII.2019.2935233
Zhang, L., Chen, X., Zhou, W., Cheng, T., Chen, L., Guo, Z., et al. (2020a). Digital twins for additive manufacturing: a state-of-the-art review. Appl. Sci. 10, 8350. doi:10.3390/app10238350
Zhang, Y. M., Yang, Y.-P., Zhang, W., and Na, S.-J. (2020b). Advanced welding manufacturing: a brief analysis and review of challenges and solutions. J. Manuf. Sci. Eng. 142. doi:10.1115/1.4047947
Zheng, T., Liu, M., Puthal, D., Yi, P., Wu, Y., and He, X. (2022). Smart grid: cyber attacks, critical defense approaches, and digital twin. ArXiv Prepr. ArXiv220511783.
Zhu, L., Xue, P., Lan, Q., Meng, G., Ren, Y., Yang, Z., et al. (2021). Recent research and development status of laser cladding: a review. Opt. Laser Technol. 138, 106915. doi:10.1016/j.optlastec.2021.106915
Zinkle, S. J. (2005). Advanced materials for fusion technology. Fusion Eng. Des. 74, 31–40. doi:10.1016/j.fusengdes.2005.08.008
Keywords: advanced manufacturing, additive manufacturing, automation, robotics, digital twin technology, cost-effectiveness, nuclear energy
Citation: Mondal K, Martinez O and Jain P (2024) Advanced manufacturing and digital twin technology for nuclear energy*. Front. Energy Res. 12:1339836. doi: 10.3389/fenrg.2024.1339836
Received: 16 November 2023; Accepted: 05 February 2024;
Published: 16 February 2024.
Edited by:
Wenzhong Zhou, Sun Yat-sen University, ChinaReviewed by:
Rosa Lo Frano, University of Pisa, ItalyParidhi Athe, North Carolina State University, United States
Panagiotis Stavropoulos, University of Patras, Greece
Copyright © 2024 Mondal, Martinez and Jain. This is an open-access article distributed under the terms of the Creative Commons Attribution License (CC BY). The use, distribution or reproduction in other forums is permitted, provided the original author(s) and the copyright owner(s) are credited and that the original publication in this journal is cited, in accordance with accepted academic practice. No use, distribution or reproduction is permitted which does not comply with these terms.
*Correspondence: Kunal Mondal, mondalk@ornl.gov