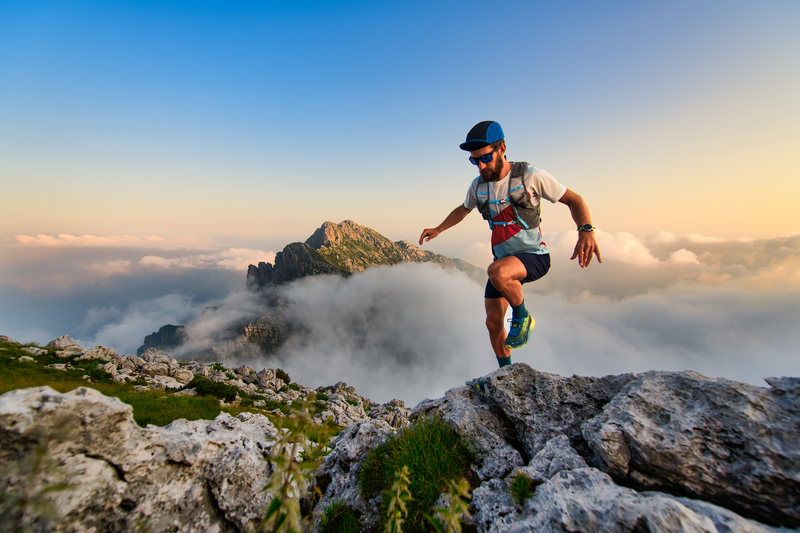
94% of researchers rate our articles as excellent or good
Learn more about the work of our research integrity team to safeguard the quality of each article we publish.
Find out more
SYSTEMATIC REVIEW article
Front. Endocrinol.
Sec. Clinical Diabetes
Volume 16 - 2025 | doi: 10.3389/fendo.2025.1485311
This article is part of the Research Topic Optical Coherence Tomography Angiography (OCTA) Applications in Ocular Complications of Diabetic Mellitus View all 4 articles
The final, formatted version of the article will be published soon.
You have multiple emails registered with Frontiers:
Please enter your email address:
If you already have an account, please login
You don't have a Frontiers account ? You can register here
Objective: To systematically review and meta-analyze the effectiveness of deep learning algorithms applied to optical coherence tomography (OCT) and retinal images for the detection of diabetic retinopathy (DR). Methods: We conducted a comprehensive literature search in multiple databases including PubMed, Cochrane library, Web of Science, Embase and IEEE Xplore up to July 2024. Studies that utilized deep learning techniques for the detection of DR using OCT and retinal images were included. Data extraction and quality assessment were performed independently by two reviewers. Meta-analysis was conducted to determine pooled sensitivity, specificity, and diagnostic odds ratios. Results: A total of 47 studies were included in the systematic review, 10 were meta-analyzed, encompassing a total of 188268 retinal images and OCT scans. The meta-analysis revealed a pooled sensitivity of 1.88 (95% CI: 1.45-2.44) and a pooled specificity of 1.33 (95% CI: 0.97-1.84) for the detection of DR using deep learning models. All of the outcome of deep learning-based optical coherence tomography ORs ≥0.785, indicating that all included studies with artificial intelligence assistance produced good boosting results. Conclusion: Deep learning-based approaches show high accuracy in detecting diabetic retinopathy from OCT and retinal images, supporting their potential as reliable tools in clinical settings. Future research should focus on standardizing datasets, improving model interpretability, and validating performance across diverse populations.
Keywords: meta analysis, deep learning, Diabetic Retinopathy, Image detection, Optical Coherence Tomography
Received: 23 Aug 2024; Accepted: 28 Feb 2025.
Copyright: © 2025 Bi, Li, Liu and Fang. This is an open-access article distributed under the terms of the Creative Commons Attribution License (CC BY). The use, distribution or reproduction in other forums is permitted, provided the original author(s) or licensor are credited and that the original publication in this journal is cited, in accordance with accepted academic practice. No use, distribution or reproduction is permitted which does not comply with these terms.
* Correspondence:
Zheng Bi, First Affiliated Hospital of Anhui University of Traditional Chinese Medicine, Hefei, Anhui Province, China
Zhaohui Fang, First Affiliated Hospital of Anhui University of Traditional Chinese Medicine, Hefei, Anhui Province, China
Disclaimer: All claims expressed in this article are solely those of the authors and do not necessarily represent those of their affiliated organizations, or those of the publisher, the editors and the reviewers. Any product that may be evaluated in this article or claim that may be made by its manufacturer is not guaranteed or endorsed by the publisher.
Research integrity at Frontiers
Learn more about the work of our research integrity team to safeguard the quality of each article we publish.