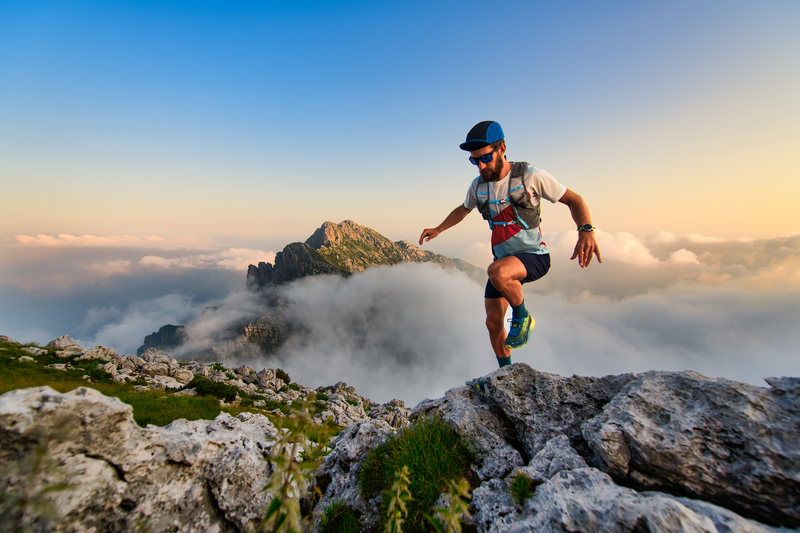
95% of researchers rate our articles as excellent or good
Learn more about the work of our research integrity team to safeguard the quality of each article we publish.
Find out more
ORIGINAL RESEARCH article
Front. Endocrinol. , 18 March 2025
Sec. Diabetes: Molecular Mechanisms
Volume 16 - 2025 | https://doi.org/10.3389/fendo.2025.1478139
This article is part of the Research Topic Cardiovascular Diseases Related to Diabetes and Obesity - Volume V View all 22 articles
Background: Diabetic cardiomyopathy (DC) is a serious complication in patients with type 1 diabetes mellitus and has become a growing public health problem worldwide. There is evidence that endoplasmic reticulum stress (ERS) is involved in the pathogenesis of DC, and related diagnostic markers have not been well-studied. Therefore, this study aimed to screen ERS-related genes (ERGs) with potential diagnostic value in DC.
Methods: Gene expression data on DC were downloaded from the GEO database, and ERGs were obtained from The Gene Ontology knowledgebase. Limma package analyzed differentially expressed genes (DEGs) in the DC and control groups, and then integrated with ERGs to identify ERS-related DEGs (ERDEGs). The ERDEGs diagnostic model was developed based on a combination of LASSO and Random Forest approaches, and the diagnostic performance was evaluated by the area under the receiver operating characteristic curve (ROC-AUC) and validated against external datasets. In addition, the association of the signature genes with immune infiltration was analyzed using the CIBERSORT algorithm and the Spearman correlation test.
Results: Gene expression data on DC were downloaded from the GEO database and ERGs were obtained from the Gene Ontology Knowledgebase. Limma package analysis identified 3100 DEGs between DC and control groups and then integrated with ERGs to identify 65 ERDEGs. Four diagnostic markers, Npm1, Jkamp, Get4, and Lpcat3, were obtained based on the combination of LASSO and random forest approach, and their ROC-AUCs were 0.9112, 0.9349, 0.8994, and 0.8639, respectively, which proved their diagnostic potential in DC. Meanwhile, Npm1, Jkamp, Get4, and Lpcat3 were validated by external datasets and a mouse model of type 1 DC. In addition, Npm1 was significantly negatively correlated with plasma cells, activated natural killer cells, or quiescent mast cells, whereas Get4 was significantly positively correlated with quiescent natural killer cells and significantly negatively correlated with activated natural killer cells (P < 0.05).
Conclusions: This study provides novel diagnostic biomarkers (Npm1, Jkamp, Get4, and Lpcat3) for DC from the perspective of ERS, which provides new insights into the development of new targets for individualized treatment of type 1 diabetic cardiomyopathy.
Type 1 diabetes mellitus is a chronic metabolic disease that threatens global health, with the latest epidemiology showing that it accounts for about two percent of the nearly 500 million total diabetes mellitus worldwide and is rising for some unknown reason (1, 2). Persuasive studies show that cardiovascular complications are the dominant cause of morbidity and mortality in Type 1 diabetes mellitus (3, 4). Diabetic cardiomyopathy (DC) is defined as structural and functional abnormalities of the heart in the absence of coronary artery disease, hypertension, and valvular heart disease, manifested by diastolic and systolic dysfunction, and ultimately progressing to heart failure, arrhythmias, and even sudden death from cardiogenic shock (5, 6). Potential mechanisms include oxidative stress, inflammation, and calcium impairment, as well as alterations in substrate metabolism/utilization, insulin signaling, gene regulation, mitochondrial dysfunction, endoplasmic reticulum stress (ERS), neurohumoral activation, and cell death (7). Currently, there is no specific treatment for DC, and a large number of patients irreversibly progress to heart failure. Therefore, it is crucial to identify effective biomarkers for early diagnosis and treatment.
The endoplasmic reticulum (ER) of mammalian cells serves as the primary site for protein folding and assembly, establishing its role as “the core organelle that ensures normal cell function” (8). Under normal conditions, misfolded proteins in the body trigger an unfolded protein response (UPR) within the endoplasmic reticulum lumen, effectively mitigating the adverse effects of misfolded proteins and potentially preventing disease onset (8, 9). However, it is important to note that in the presence of metabolic diseases such as diabetes, ocular conditions like age-related macular degeneration and retinitis pigmentosa, or cardiovascular diseases, liver disease and even cancer, factors such as oxidative stress, metabolic abnormalities, and Ca2+ dysregulation become widely activated (8, 10–13). These factors can lead to a significant increase in unfolded and misfolded proteins, resulting in the overactivation of the unfolded protein response and consequently inducing endoplasmic reticulum stress (8, 14–17). Following the occurrence of endoplasmic reticulum stress, downstream pathways are primarily activated through three signaling proteins: inositol-requiring protein-1α (IRE1α), protein kinase RNA-like ER kinase (PERK), and activating transcription factor 6 (ATF6) (18–20). These pathways ultimately inhibit protein synthesis, regulate gene expression, and determine cell fate, including processes such as apoptosis. In summary, endoplasmic reticulum stress serves as a sensitive sensor for the onset of disease and plays a crucial role in determining the final fate of cells. A significant number of studies have indicated that diabetic cardiomyopathy, a serious condition characterized by various risk factors including oxidative stress, metabolic abnormalities, and Ca2+ overload, is closely associated with endoplasmic reticulum stress (21–23). Furthermore, many investigations have highlighted that endoplasmic reticulum-related genes may serve as early markers of ischemic heart disease and play a crucial role in its pathophysiology (24, 25). Building on this foundation, we propose the scientific research hypothesis that endoplasmic reticulum-related genes may be involved in the progression of diabetic cardiomyopathy and could potentially serve as predictive markers for identifying intervention targets.
Many recent studies have emphasized that immune responses and ERS crosstalk with each other and are fundamentally and comprehensively intertwined (26–28). The effects of ERS include direct defense against microbial pathogens, production of pro-inflammatory cytokines, presentation of antigens to T cells, immunogenic cell death, metabolic homeostasis, and maintenance of immune tolerance (29). Effective immunity depends on endoplasmic reticulum homeostatic processes such as calcium signaling, glycosylation, lipid metabolism, and oxidative protein folding (30). During transient ER stress, all three signaling pathways of the UPR can crosstalk with inflammatory and stress signaling pathways, including nuclear factor-kappa B (NF-κB), a major transcriptional regulator of innate immunity (31). In view of this, ERGs and transcriptomic data on type 1 DC were collected from public databases, and ERS-related differentially expressed genes (ERDEGs) between DC and control samples were identified, which screened potential ERS-related diagnostic markers, providing new ideas for the diagnosis and treatment of DC.
To increase the sample size for enhanced confidence and reliability of the results, GSE155377, GSE210611, and GSE123975 were combined into one cohort, which ultimately consisted of 13 control samples and 13 DC samples. Box plot analysis (Figures 1A, C) and PCA (Figures 1B, D) indicated that batch effects were successfully eliminated. The batch-corrected PCA analysis showed that the data distribution tended to be uniform across the datasets, implying that normalization might be completed correctly. A total of 3100 DEGs were identified, of which 1662 were down-regulated and 1438 were up-regulated (Figure 1E). In addition, gene set enrichment analysis revealed that the biological processes (BP) involved in DC mainly consisted of complement activation, eosinophil migration, regulation of vascular endothelial growth factor production, response to cold and vascular endothelial growth factor production (Figure 1F). Cellular components (CC) involved in DC mainly consisted of collagen-containing extracellular matrix、external encapsulating structure, extracellular matrix, extracellular region and extracellular space (Figure 1G). molecular functions (MF) involved in DC mainly consisted of chemoattractant activity, endopeptidase inhibitor activity, endopeptidase regulator activity, peptidase inhibitor activity and thioester hydrolase activity (Figure 1H). The active Kyoto Encyclopedia of Genes and Genomes (KEGG) pathways were mainly biosynthesis of unsaturated fatty acids, fatty acid elongation, malaria, ovarian steroidogenesis, and PPAR signaling pathways (Figure 1I).
Figure 1. Identification and functional enrichment analysis of DEGs. (A-D) Boxplots and PCA were applied to visualize the batch correction effect before (A, B) and after (C, D) batch effect removal. (E) Volcano plot of DEGs. Blue and red dots indicate significantly up-and down-regulated genes, respectively, while gray dots indicate genes with no significant difference. (F) Top ten biological processes active in DC. (G) Top ten cellular components active in DC. (H) Top ten molecular functions active in DC. (I) Top ten KEGG pathways active in DC.
Sixty-five ERDEGs were obtained by crossing 345 ERGs with DEGs (Figure 2A). Of these, 35 were up-regulated and 30 were down-regulated (Figure 2B). Functional annotation analysis was applied to further explore the functions of ERDEGs, and as a result, many terms related to ERS were enriched. The BP was mainly enriched for response to ERS, cellular response to topologically incorrect protein, and cellular response to unfolded protein (Figure 2C), suggesting that their perturbation may mediate the pathogenesis of DC. The CC was mainly enriched for the endoplasmic reticulum lumen, organelle outer membrane, protein folding chaperone complex, etc. (Figure 2D). The MF was significantly enriched for unfolded protein binding, protein-folding chaperone binding, and misfolded protein binding (Figure 2E). Of particular note, KEGG analysis revealed that the top-ranked pathway was protein processing in the endoplasmic reticulum (Figure 2F).
Figure 2. Identification and functional annotation analysis of ERDEGs. (A) Venn diagram illustrating the overlap region between DEGs and ERGs in DC. (B) Heatmap demonstrating the expression levels of ERDEGs in each sample. (C-E) Dot plots of the top 10 highest enrichment levels for biological processes, cellular components, and molecular functions. (F) Dot plots of the top 10 pathway terms with the highest enrichment levels identified by KEGG analysis.
When LASSO was constructed based on 10-fold cross-validation, the minimum error value corresponded to 12 signature genes, including Pik3r1, Tomm20, Prkn, Rasgrf2, Sgta, Scamp5, Rcn3, and Pdia3 (Figures 3A, B). Using 0.25 as the importance score threshold, 18 signature genes were obtained, including Npm1, Jkamp, Get4, Lpcat3, Ppp2cb, Mkks, Clpx, Bcl2l1, Ube2g2, Flot1, Ppp1r15a, Nfe2l2, Rpap2, Srpx, Calr3, Serpinh1, Hspb1, and Herpud2 (Figure 3C). Npm1, Jkamp, Get4, and Lpcat3 are signature genes common to LASSO and RandomForest (Figure 3D). Among them, Jkamp was down-regulated in DC (Figure 3E) and all other genes were up-regulated (Figures 3F–H). Significant positive correlations were observed between Lpcat3 or Get4 and Npm1, and between Lpcat3 and Get4, whereas significant negative correlations were observed between Jkamp and the other three genes (Figure 3I). ROC-AUCs of the four signature genes in the combined dataset were all greater than 0.75, indicating excellent diagnostic capability (Figure 3J).
Figure 3. Selection of signature genes by machine learning algorithms. (A) Schematic diagram of the LASSO regression diagnostic model for ERDEGs. (B) Trajectory diagram describing the LASSO selection variables in the diagnostic model for ERDEGs. (C) Dotplot of relative importance ranking by RandomForest. (D) Venn diagram of common signature genes. (E-H) Boxplots depicting the expression levels of four signature genes in the constructed features. **P < 0.01, ***P < 0.001, ****P < 0.0001. (I) Chord plot illustrating the correlation of the characterized genes. (J) ROC analysis of the four characterized genes.
Cumulative histograms showed the relative proportions of 22 immune cells in DC and control samples, with B cells naive, Macrophages M2, T cells CD4 memory resting, and Plasma cells accounting for the majority (Figure 4A). Boxplots of differences in immune cell infiltration showed significantly more B cells naive in the DC group than in the control group (P < 0.05; Figure 4B). The heatmap displayed the correlation between the 22 immune cell types (Figure 4C). The correlation of Jkamp and Lpcat3 with 22 immune cell types was not statistically significant (Figures 4D, F). Npm1 showed a significant negative correlation with Plasma cells, natural killer cells activated, or Mast cells resting (Figure 4E). Get4 showed a significant positive correlation with natural killer cells resting and a significant negative correlation with natural killer cells activated (Figure 4G).
Figure 4. Correlation of signature genes with infiltrating immune cells. (A) Cumulative histograms of the distribution of 22 immune cells in the DC and control groups. (B) Box plots of immune cell expression in control and DC groups. *P < 0.05. (C) Heatmap of correlation between immune cells. (D-G) Lollipop plots of correlation between immune cells and signature genes.
In the validation dataset, the expression trends of the four signature genes were consistent with those in the training set (Figures 5A–D). All four ROC-AUCs in the validation dataset were greater than 0.75 (Figures 5E–H). Overall, these results suggest that ERS-related signature genes have a predictive ability in the diagnosis of DC.
Figure 5. Validation of the expression and diagnostic ability of the signature genes. (A-D) Box plots showing the expression levels of the four signature genes. (E-H) ROC curves of the four signature genes. *P < 0.05, **P < 0.01.
As shown in Figure 6A, the myocardium was significantly damaged in the DC group compared with the control group, as evidenced by disorganized cardiomyocyte arrangement, edema, and inflammatory cell infiltration. As shown in Figure 6B, there were more apoptotic cells in the DC group compared with the control group. As shown in Figures 6C and D, there were obvious fibrotic areas in the cardiac tissue in the DC group compared with the control group. As shown in Figures 6E–H, RT-qPCR was employed to verify the expression levels of the four signature genes in cardiac tissues, which was consistent with the results of bioinformatics analysis.
Figure 6. Validation of signature genes in a mouse model of type 1 DC. (A) Representative HE staining images to assess myocardial injury. The scale bar represents 200 and 100 μm.(n=3) (B) Representative Tunel staining to assess apoptosis. The scale bar represents 100 μm. (n=3) (C, D) Representative Masson staining images and Sirius red staining images to assess collagen fiber area. The scale bar represents 200 μm. (n=3) (E-H) Relative expression levels of mRNA of four signature genes in cardiac tissues by RT-qPCR. The data was presented as mean ± SD, ****P < 0.0001, n = 6.
Diabetic cardiomyopathy represents a significant complication of diabetes, with affected patients being 2 to 4 times more likely to develop heart failure or succumb to mortality compared to the general population (23, 32). Given that diabetes is recognized as the most prevalent disease globally, alongside the aging population and shifts in contemporary dietary patterns, the incidence of diabetic cardiomyopathy has shown a concerning upward trend (7, 23, 24, 33, 34). Consequently, it is imperative to investigate early diagnosis and prevention strategies for this condition. In this study, DC samples and ERGs obtained from public datasets identified 65 ERDEGs, from which four signature genes of type 1 DC, including Jkamp, Npm1, Lpcat3, and Get4, were identified by LASSO and Random Forest analyses. Four characteristic genes of diagnostic significance have been discovered to be closely associated with immune cells. Although the four genes we screened currently lack relevant literature confirming directly their relationship with DC, they are significant in the progression or repair of various complications associated with diabetes and cardiovascular disease. This indicates their considerable research potential in the context of DC progression. We will discuss each gene in detail below.
JNK1/mapk8-associated membrane protein (JKAMP/JAMP) is a seven-transmembrane protein situated in the cytoplasmic membrane. It interacts with JNK1 via its C-terminal domain, enhancing and prolonging JNK1 activity, which in turn increases the incidence of JNK-dependent apoptosis (35). This apoptotic process has been established as a critical factor in DC and is recognized as a consequence of ERS (36–39). Furthermore, studies examining other systemic complications of diabetes, such as diabetic osteoporosis, have confirmed that the overexpression of JKAMP appears to mitigate the adverse effects of hyperglycemia through the activation of the Wnt signaling pathway (40). These phenomena suggest that JKAMP may influence the progression of DC by modulating ERS. Nucleophosmin 1 (NPM1) is a multifunctional nucleophosmin with shuttling properties involved in a variety of cellular functions, including participation in liquid-liquid phase separation, ribosome biogenesis, and histone chaperoning and transcriptional regulation (41). Although a direct link between NPM1 and DC has not yet been established, existing evidence suggests that NPM1 can significantly influence macrophage polarization, thereby impacting the repair processes of cardiomyocytes (42). During the progression of DC, which is characterized as a typical metabolic and immune-related cardiovascular disease, the polarization of macrophages by NPM1 may represent a critical mechanism (43). LPCAT3, a gene widely expressed in key metabolic tissues such as the liver, small intestine, skeletal muscle, macrophages, and adipocytes, has been shown to directly enhance the activation of IRE1α and PERK by altering the phospholipid composition of the endoplasmic reticulum membrane, thereby triggering ERS (44, 45). These processes are regarded as potential targets for the treatment of metabolic diseases (46, 47). Interestingly, several studies have indicated that lpcat3 can significantly influence the expression levels of GPX4, thereby regulating the extent of ferroptosis and ultimately impacting the progression of DC (48, 49). Golgi to ER traffic protein 4 (GET4) and Get5 form a complex that competitively binds ribosomes with SRP and directs tail-anchored proteolytic delivery to the endoplasmic reticulum (50). Mutants with GET dysfunction are more susceptible to ERS (51). DC, whether its rate of progression is believed to be significantly influenced by ERS (36–39) or regarded as a classic metabolic disease (23, 43), appears to be inextricably linked to the four aforementioned molecules. Unfortunately, there are currently no definitive studies confirming the relationship between these four molecules and DC; however, this also suggests the research potential of these molecules.
The present study further analyzed immune cell infiltration and revealed that there were significantly more B cells naive in the DC group than in the control group, so it was hypothesized that DC was associated with abnormalities in the immune system. Inhibited activation of the NF-κB signaling pathway in activated B cells decelerated the progression of type 1 DC (52). In addition, this study demonstrated the correlation of Npm1 and Get4 with immune cells, suggesting that they may influence the development of DC by affecting immune cells. Therefore, ameliorating the abnormal immune status may also be a promising therapeutic strategy for DC.
Numerous prior studies on DC have sought to identify potential biomarkers and therapeutic targets for intervention. These investigations frequently emphasize aspects such as immune metabolism, with some specifically addressing the regulation of the immune microenvironment (53–56). ERS is the earliest pathophysiological process to be fully activated in DC (36–38, 57). Molecular markers derived from related molecules appear to possess superior properties for early diagnosis. Furthermore, ERS and the consequent apoptosis of cardiomyocytes represent critical factors influencing the progression rate of DC (36–38, 57). Consequently, the molecular markers identified from these related molecules are more effective as therapeutic targets. However, some existing studies utilize high-throughput technical methods, such as metabolomics and liquid chromatography-mass spectrometry (LC-MS), which appear to play a more significant role in identifying key pathways and molecules involved in DC (58).
Additionally, there are some limitations of this study. Although some studies have indicated that mice are the only mammals that can provide such a rich resource of genetic diversity while also allowing for extensive genome manipulation, making them a powerful tool for modeling human cardiovascular diseases, particularly diabetic cardiomyopathy (23, 59–61). In these models, the trends of various physiological indicators in mice, such as left ventricular contractility and left ventricular ejection fraction, align with those observed in humans, establishing the mouse diabetic cardiomyopathy model as a primary technology for studying this disease (23, 60, 62). However, it is important to note that numerous studies have highlighted significant deviations in conclusions derived from mouse models when translated to clinical and technical applications (23, 63). Furthermore, intervention methods used in humans cannot be fully replicated in diabetic mice (23, 62). Therefore, our study requires further supplementation and validation through clinically relevant research in the future. In addition to this limitation, we also face several shortcomings, including the need for further investigation into the expression and diagnostic value of these four genes at the protein level, as well as the absence of additional experiments to validate the effects of these characteristic genes on immune cells and heart function.
The Gene Ontology (GO) knowledgebase (http://geneontology.org/) was searched to collect ERGs, including regulation of response to endoplasmic reticulum stress (GO:1905897), unfolded protein binding (GO:0051082), response to endoplasmic reticulum stress (GO:0034976), and endoplasmic reticulum unfolded protein response (GO:0030968), and 345 ERGs were obtained after removing duplicates. Gene expression profiling of type 1 DC was obtained from the GEO (http://www.ncbi.nlm.nih.gov/geo) database, including GSE210611, GSE155377, GSE123975, and GSE215979, working according to the flowchart in Figure 7. Among them, GSE210611 (3 CON and 3 DC), GSE155377 (4 CON and 4 DC), and GSE123975 (4 CON and 4 DC) were constructed as a multichip dataset containing 13 CON and 13 DC by R (version 4.3.1) software, and the remove batch effect function of the limma (version 3.60.2) package could be applied to remove batch effects. Principal Component Analysis (PCA) was applied to assess whether the batch effect had been removed. GSE215979 (3 CON and 3 DC) was set as the external validation dataset.
Figure 7. Workflow diagram of this study. The training set acquires ERS-related signature genes and performs immune infiltration analysis. In addition, the validation set judges the diagnostic performance of the signature genes.
DEGs were analyzed between the DC and control groups using the R package “limma” (version 3.60.2) for the multi-chip dataset. Genes filtered according to the threshold |log2fold change(FC)|> 0 and P < 0.05 were selected as DEGs, and volcano plots were generated to visualize the results. In addition, gene set enrichment analysis was utilized to identify the most significant functional terms between the DC and control groups (64).
Draw Venn Diagram (https://bioinformatics.psb.ugent.be/webtools/Venn/) intersected ERGs with DEGs in the multichip dataset to obtain ERDEGs, whose gene expression was demonstrated by heatmaps created with the “pheatmap” package (version 1.0.12). To further elucidate the biological functions of ERDEGs, GO enrichment analysis and KEGG pathway analysis were performed via “ClusterProfiler” (version 4.12.0), whose results were plotted using the “ggplot2” package (version 3.5.1). Screening for BP, CC, MF, and KEGG pathways with P <0.05 (65, 66).
The R package “glmnet” (version 4.1.8) was applied to obtain ERS-related signature genes by the LASSO algorithm with 10-fold cross-validation to determine the optimal value of the penalty parameter λ. LASSO algorithms are often interpreted by cross-validation graphs and regression coefficient path graphs. First, the cross-validation curve is applied to select the optimal lambda value. The X-axis is the logλ of the penalty coefficient, and the Y-axis is the likelihood deviation. The smaller the Y-axis is, the better the fitting effect of the equation is. The top number is the number of variables left in the equation for different λ. There are usually two dotted lines, the lambda with the smallest deviation but the highest model fit (lambda. min) on the left, and the lambda value with the smallest deviation but the more concise model (lambda.1se) on the right. Based on the best prediction performance and feature selection capability, lambda. min is selected in this paper. Second, regression coefficient path graph, each line in the graph represents a variable, the ordinate is the coefficient, and the upper abscissa is the number of non-zero coefficients in the model under different regularization parameters. The lower horizontal coordinate is the normalized normalized parameter. The figure shows the variation trajectories of variable coefficients under different regularization parameters. When the regularization is larger, the complexity of the model is lower, so most parameters will approach 0. We can know which features contribute more or less to the prediction of the model through this figure, and we can initially use the features with greater contributions in subsequent analysis.
Random Forest is an integrated learning method based on decision trees, where multiple decision trees are constructed and their predictions are aggregated for classification or regression tasks to improve overall accuracy and stability (67). One of the most important results of Random Forests in machine learning is the assessment of significance based on the Gini index and the final identification of the featured genes, which can be done with the “RandomForest” (version 4.7.1.1) package of the R software and visualized by drawing dotplots with varImpPlot. The crossover genes of LASSO and Random Forest were then identified as the optimal ERS-associated signature genes for the next step of the study.
ROC analysis was performed to determine the diagnostic validity of the four signature genes with the “pROC” package (version 1.18.5). ROC curve is a curve obtained by plotting the true positive rate and false positive rate, which can reflect the relationship between sensitivity and specificity. The horizontal axis represents the false positive rate (1- specificity) and the vertical axis represents the true positive rate (sensitivity). ROC-AUC reflects the value of diagnostic tests. The larger the area, the closer to 1.0, the higher the diagnostic authenticity. The closer it is to 0.5, the lower the accuracy of the diagnosis. When it is equal to 0.5, it has no diagnostic value. ROC-AUC was applied to estimate the diagnostic ability to differentiate DC from the control group.
Immune cell subtypes in the multichip dataset were evaluated by the CIBERSORT algorithm with the LM22 gene feature matrix, and values with P < 0.05 were considered statistically different (68). Correlations between immune cells and between immune cells and ERS-related signature genes were analyzed using Spearson analysis.
C57BL/6j male mice (20-25 g) were raised in the standard barrier environment of the Animal Experiment Center at Renmin Hospital of Wuhan University, maintained at a temperature of 21-24°C and a humidity of 50-60%, with a light and dark cycle of 12 hours. This experiment received approval from the Wuhan University Committee on Animal Care and Utilization (IACUC Issue No. 20230805C). Upon reaching 6 to 8 weeks of age, the mice were randomly assigned to either a control group or a diabetes group, with a minimum of six mice in each group. The diabetic group received intraperitoneal injections of streptozotocin (STZ, Boagang, China) dissolved in a citric acid buffer (pH 4.2-4.5) at a dose of 50 mg/kg for five consecutive days. In contrast, the control group was administered an equal volume of STZ-free citrate buffer. Fasting blood glucose levels were measured on days three and seven following the injections; mice exhibiting fasting blood glucose levels of 11.1 mM or higher were classified as diabetic. Subsequently, the mice were maintained on a continuous feeding regimen for 12 weeks, during which blood glucose levels were assessed every four weeks to confirm that the blood glucose levels of the diabetic mice remained consistently above 11.1 mM. At the conclusion of the 12th week, the mice were euthanized in accordance with ethical guidelines, and their hearts were excised. Some hearts were designated for immediate mRNA extraction, while others were fixed in 4% paraformaldehyde for subsequent pathological analysis. This allocation was performed randomly. Mice that did not develop the disease model were euthanized following the protocols outlined in the ethics manual.
The fixed heart tissues were embedded in paraffin and cut into 4 μm thick sections. They were then stained with hematoxylin and eosin (HE), Tunel, Masson, and Sirius red which are shown below.
The paraffin sections were dewaxed in a series of solutions: Dewaxing Clear Solution I for 20 minutes, Dewaxing Clear Solution II for 20 minutes, Absolute Ethanol I for 5 minutes, Absolute Ethanol II for 5 minutes, and 75% Alcohol for 5 minutes. Subsequently, the sections were immersed in a hematoxylin staining solution for 5 minutes, followed by a wash with double-distilled water. The sections were then differentiated using hematoxylin differentiation solution for a few seconds, rinsed with double-distilled water, and treated with hematoxylin blue-returning solution to restore the blue color, followed by another rinse with double-distilled water. Next, the sections were dehydrated in 85% Alcohol and 95% Alcohol for 5 minutes each, stained with eosin stain for 5 minutes, and then dehydrated and clarified in absolute ethanol and dewaxing clear solution. Finally, the sections were sealed with neutral gum. The structure and morphology of the myocardial fibers were observed using optical microscopes.
Terminal deoxynucleotidyl transferase-mediated dUTP nick-end labeling (TUNEL) was used to examine the myocardial cell apoptosis with an in situ cell death detection kit (Nanjing Jiancheng Bioengineering Institute, China) according to the manufacturer’s instructions. Briefly, the heart tissues of each group were embedded in paraffin and cut into 5 μm thick sections. Then the sections were stained with TUNEL reaction mixture for 60 min and immersed into 4’,6-diamidino2-phenylindole (DAPI) to stain nuclei for 30 min. Apoptotic cells were observed under a light microscope with an excitation wavelength of 585–600 nm.
After dewaxing the paraffin sections to water using the aforementioned method, perform the following steps in sequence: (1) Immerse in potassium dichromate (Servicebio, G3326, China) overnight; (2) Stain with iron hematoxylin (Servicebio, G3326, China) for 10 minutes, followed by thorough washing with water; (3) Return to blue using Masson blue solution (Servicebio, G3326, China) for 5 minutes, and wash well with water; (4) Stain with Ponceau magenta (Servicebio, G3326, China) for 10 minutes; (5) Wash with phosphomolybdic acid solution (Servicebio, G3326, China) for 2 minutes; (6) Wash with weak acid working solution for 1 minute; (7) Stain with aniline blue dyeing solution (Servicebio, G3326, China) for 1 minute; (8) Wash with weak acid working solution for 1 minute; (9) Sequentially immerse in 95% alcohol, 100% alcohol, and xylene to dehydrate and render the sections transparent; (10) Seal the slide with neutral gum. Finally, use an optical microscope to observe the deposition of collagen fibers in the myocardium, where collagen fibers appear blue, while muscle fibers, cytoplasm, and cutin are red.
After removing the paraffin sections and dewaxing them in water, proceed with the following steps in sequence: (1) stain with Sirius scarlet for 8 minutes; (2) dehydrate using absolute ethanol for 5 minutes; (3) seal with neutral gum. Utilize a 400× optical microscope to observe the deposition of collagen fibers in the myocardium, where collagen fibers appear red and other tissue components are displayed in yellow.
Total RNA was extracted from cardiac tissues using an RNA extraction kit (RC113-01; Vazyme, China). Reverse transcription and quantification were performed using SweScript All-in-One RT SuperMix for qPCR (One-Step gDNA Remover) (Servicebio, G3337, China) and 2×Universal Blue SYBR Green qPCR Master Mix (Servicebio, G3326, China). Finally, RT-qPCR was performed on a Lightcycler 480II Real-Time Fluorescence Quantitative PCR System (Roche, Germany). β-actin was used for expression normalization. The primers are shown in Table 1, and the relative expression of the genes was determined by the 2-ΔΔCT method.
All statistical analyses were performed by R software (version 4.3.1). Student’s t-test or one-way ANOVA was applied to assess differences between the two groups. P < 0.05 indicated statistical significance.
In summary, four signature genes (Npm1, Jkamp, Get4, and Lpcat3) have been tentatively identified as potential diagnostic markers of type 1 DC, which may be influenced by controlling ERS and immune cells. The results of this study provide new insights into the development of new targets for the diagnosis and treatment of DC.
The datasets presented in this study can be found in online repositories. The names of the repository/repositories and accession number(s) can be found in the article/supplementary material.
This experiment received approval from the Wuhan University Committee on Animal Care and Utilization (IACUC Issue No. 20230805C). The study was conducted in accordance with the local legislation and institutional requirements.
QT: Conceptualization, Data curation, Methodology, Visualization, Writing – original draft. YJ: Data curation, Investigation, Methodology, Validation, Writing – original draft. ZX: Funding acquisition, Project administration, Supervision, Writing – review & editing. YZ: Formal analysis, Investigation, Writing – review & editing, Validation. CD: Funding acquisition, Project administration, Writing – review & editing, Visualization. QS: Funding acquisition, Investigation, Project administration, Resources, Writing – review & editing. SL: Conceptualization, Funding acquisition, Project administration, Supervision, Writing – review & editing.
The author(s) declare that financial support was received for the research and/or publication of this article. This work is supported by the National Natural Science Foundation of China (82072140), Hubei Province Natural Science Foundation of China (No. 2023AFB821), Fundamental Research Funds for the Central Universities (2042023kf0033), and Tianjin Science and Technology Fund Planning Project (21JCYBJC01130).
The authors declare that the research was conducted in the absence of any commercial or financial relationships that could be construed as a potential conflict of interest.
All claims expressed in this article are solely those of the authors and do not necessarily represent those of their affiliated organizations, or those of the publisher, the editors and the reviewers. Any product that may be evaluated in this article, or claim that may be made by its manufacturer, is not guaranteed or endorsed by the publisher.
1. Saeedi P, Petersohn I, Salpea P, Malanda B, Karuranga S, Unwin N, et al. Global and regional diabetes prevalence estimates for 2019 and projections for 2030 and 2045: Results from the International Diabetes Federation Diabetes Atlas, 9(th) edition. Diabetes Res Clin Pract. (2019) 157:107843. doi: 10.1016/j.diabres.2019.107843
2. Green A, Hede SM, Patterson CC, Wild SH, Imperatore G, Roglic G, et al. Type 1 diabetes in 2017: global estimates of incident and prevalent cases in children and adults. Diabetologia. (2021) 64:2741–50. doi: 10.1007/s00125-021-05571-8
3. Mozaffarian D, Benjamin EJ, Go AS, Arnett DK, Blaha MJ, Cushman M, et al. Heart disease and stroke statistics–2015 update: a report from the American Heart Association. Circulation. (2015) 131:e29–322. doi: 10.1161/cir.0000000000000152
4. Konduracka E, Cieslik G, Galicka-Latala D, Rostoff P, Pietrucha A, Latacz P, et al. Myocardial dysfunction and chronic heart failure in patients with long-lasting type 1 diabetes: a 7-year prospective cohort study. Acta diabetologica. (2013) 50:597–606. doi: 10.1007/s00592-013-0455-0
5. Julián MT, Pérez-Montes de Oca A, Julve J, Alonso N. The double burden: type 1 diabetes and heart failure-a comprehensive review. Cardiovasc Diabetol. (2024) 23:65. doi: 10.1186/s12933-024-02136-y
6. Tan Y, Zhang Z, Zheng C, Wintergerst KA, Keller BB, Cai L. Mechanisms of diabetic cardiomyopathy and potential therapeutic strategies: preclinical and clinical evidence. Nat Rev Cardiol. (2020) 17:585–607. doi: 10.1038/s41569-020-0339-2
7. Ritchie RH, Abel ED. Basic mechanisms of diabetic heart disease. Circ Res. (2020) 126:1501–25. doi: 10.1161/circresaha.120.315913
8. Chen X, Shi C, He M, Xiong S, Xia X. Endoplasmic reticulum stress: molecular mechanism and therapeutic targets. Signal transduction targeted Ther. (2023) 8:352. doi: 10.1038/s41392-023-01570-w
9. Xu J, Zhou Q, Xu W, Cai L. Endoplasmic reticulum stress and diabetic cardiomyopathy. Exp Diabetes Res. (2012) 2012:827971. doi: 10.1155/2012/827971
10. Chen X, Cubillos-Ruiz JR. Endoplasmic reticulum stress signals in the tumour and its microenvironment. Nat Rev Cancer. (2021) 21:71–88. doi: 10.1038/s41568-020-00312-2
11. Lebeaupin C, Vallée D, Hazari Y, Hetz C, Chevet E, Bailly-Maitre B. Endoplasmic reticulum stress signalling and the pathogenesis of non-alcoholic fatty liver disease. J Hepatol. (2018) 69:927–47. doi: 10.1016/j.jhep.2018.06.008
12. Ajoolabady A, Kaplowitz N, Lebeaupin C, Kroemer G, Kaufman RJ, Malhi H, et al. Endoplasmic reticulum stress in liver diseases. Hepatol (Baltimore Md.). (2023) 77:619–39. doi: 10.1002/hep.32562
13. Ren J, Bi Y, Sowers JR, Hetz C, Zhang Y. Endoplasmic reticulum stress and unfolded protein response in cardiovascular diseases. Nat Rev Cardiol. (2021) 18:499–521. doi: 10.1038/s41569-021-00511-w
14. Yang L, Zhao D, Ren J, Yang J. Endoplasmic reticulum stress and protein quality control in diabetic cardiomyopathy. Biochim Biophys Acta. (2015) 1852:209–18. doi: 10.1016/j.bbadis.2014.05.006
15. Jin Z, Ji Y, Su W, Zhou L, Wu X, Gao L, et al. The role of circadian clock-controlled mitochondrial dynamics in diabetic cardiomyopathy. Front Immunol. (2023) 14:1142512. doi: 10.3389/fimmu.2023.1142512
16. Wang M, Kaufman RJ. Protein misfolding in the endoplasmic reticulum as a conduit to human disease. Nature. (2016) 529:326–35. doi: 10.1038/nature17041
17. Frakes AE, Dillin A. The UPR(ER): sensor and coordinator of organismal homeostasis. Mol Cell. (2017) 66:761–71. doi: 10.1016/j.molcel.2017.05.031
18. Li C, Li S, Zhang G, Li Q, Song W, Wang X, et al. IRE1α Mediates the hypertrophic growth of cardiomyocytes through facilitating the formation of initiation complex to promote the translation of TOP-motif transcripts. Circulation. (2024) 150:1010–29. doi: 10.1161/circulationaha.123.067606
19. Liu ZW, Zhu HT, Chen KL, Dong X, Wei J, Qiu C, et al. Protein kinase RNA-like endoplasmic reticulum kinase (PERK) signaling pathway plays a major role in reactive oxygen species (ROS)-mediated endoplasmic reticulum stress-induced apoptosis in diabetic cardiomyopathy. Cardiovasc Diabetol. (2013) 12:158. doi: 10.1186/1475-2840-12-158
20. Han X, Zhou W, Zhang J, Tu Y, Wei J, Zheng R, et al. Linderalactone mitigates diabetic cardiomyopathy in mice via suppressing the MAPK/ATF6 pathway. Int Immunopharmacol. (2023) 124:110984. doi: 10.1016/j.intimp.2023.110984
21. Li J, Zhu H, Shen E, Wan L, Arnold JM, Peng T. Deficiency of rac1 blocks NADPH oxidase activation, inhibits endoplasmic reticulum stress, and reduces myocardial remodeling in a mouse model of type 1 diabetes. Diabetes. (2010) 59:2033–42. doi: 10.2337/db09-1800
22. Wu T, Dong Z, Geng J, Sun Y, Liu G, Kang W, et al. Valsartan protects against ER stress-induced myocardial apoptosis via CHOP/Puma signaling pathway in streptozotocin-induced diabetic rats. Eur J Pharm sciences: Off J Eur Fed Pharm Sci. (2011) 42:496–502. doi: 10.1016/j.ejps.2011.02.005
23. Dillmann WH. Diabetic cardiomyopathy. Circ Res. (2019) 124:1160–2. doi: 10.1161/circresaha.118.314665
24. Xue A, Lin J, Que C, Yu Y, Tu C, Chen H, et al. Aberrant endoplasmic reticulum stress mediates coronary artery spasm through regulating MLCK/MLC2 pathway. Exp Cell Res. (2018) 363:321–31. doi: 10.1016/j.yexcr.2018.01.032
25. Yu B, Xu C, Tang X, Liu Z, Lin X, Meng H, et al. Endoplasmic reticulum stress-related secretory proteins as biomarkers of early myocardial ischemia-induced sudden cardiac deaths. Int J Legal Med. (2022) 136:159–68. doi: 10.1007/s00414-021-02702-z
26. Zhao Y, Guo H, Li Q, Wang N, Yan C, Zhang S, et al. TREM1 induces microglial ferroptosis through the PERK pathway in diabetic-associated cognitive impairment. Exp Neurol. (2025) 383:115031. doi: 10.1016/j.expneurol.2024.115031
27. LaMarche NM, Kane H, Kohlgruber AC, Dong H, Lynch L, Brenner MB. Distinct iNKT cell populations use IFNγ or ER stress-induced IL-10 to control adipose tissue homeostasis. Cell Metab. (2020) 32:243–258.e246. doi: 10.1016/j.cmet.2020.05.017
28. Keestra-Gounder AM, Byndloss MX, Seyffert N, Young BM, Chávez-Arroyo A, Tsai AY, et al. NOD1 and NOD2 signalling links ER stress with inflammation. Nature. (2016) 532:394–7. doi: 10.1038/nature17631
29. Bettigole SE, Glimcher LH. Endoplasmic reticulum stress in immunity. Annu Rev Immunol. (2015) 33:107–38. doi: 10.1146/annurev-immunol-032414-112116
30. Di Conza G, Ho PC. ER stress responses: an emerging modulator for innate immunity. Cells. (2020) 9. doi: 10.3390/cells9030695
31. Zhang K, Kaufman RJ. From endoplasmic-reticulum stress to the inflammatory response. Nature. (2008) 454:455–62. doi: 10.1038/nature07203
32. Park JJ. Epidemiology, pathophysiology, diagnosis and treatment of heart failure in diabetes. Diabetes Metab J. (2021) 45:796. doi: 10.4093/dmj.2021.0239
33. Yao X, Huang X, Chen J, Lin W, Tian J. Roles of non-coding RNA in diabetic cardiomyopathy. Cardiovasc Diabetol. (2024) 23:227. doi: 10.1186/s12933-024-02252-9
34. Xie J, Wang M, Long Z, Ning H, Li J, Cao Y, et al. Global burden of type 2 diabetes in adolescents and young adults, 1990-2019: systematic analysis of the Global Burden of Disease Study 2019. BMJ (Clinical Res ed.). (2022) 379:e072385. doi: 10.1136/bmj-2022-072385
35. Kadoya T, Khurana A, Tcherpakov M, Bromberg KD, Didier C, Broday L, et al. JAMP, a Jun N-terminal kinase 1 (JNK1)-associated membrane protein, regulates duration of JNK activity. Mol Cell Biol. (2005) 25:8619–30. doi: 10.1128/mcb.25.19.8619-8630.2005
36. Preetha Rani MR, Salin Raj P, Nair A, Ranjith S, Rajankutty K, Raghu KG. In vitro and in vivo studies reveal the beneficial effects of chlorogenic acid against ER stress mediated ER-phagy and associated apoptosis in the heart of diabetic rat. Chem Biol Interact. (2022) 351:109755. doi: 10.1016/j.cbi.2021.109755
37. Sun S, Yang S, An N, Wang G, Xu Q, Liu J, et al. Astragalus polysaccharides inhibits cardiomyocyte apoptosis during diabetic cardiomyopathy via the endoplasmic reticulum stress pathway. J Ethnopharmacol. (2019) 238:111857. doi: 10.1016/j.jep.2019.111857
38. Wang W, Liu T, Liu Y, Yu L, Yan X, Weng W, et al. Astaxanthin attenuates alcoholic cardiomyopathy via inhibition of endoplasmic reticulum stress-mediated cardiac apoptosis. Toxicol Appl Pharmacol. (2021) 412:115378. doi: 10.1016/j.taap.2020.115378
39. Galeone A, Annicchiarico A, Buccoliero C, Barile B, Luciani GB, Onorati F, et al. Diabetic cardiomyopathy: role of cell death, exosomes, fibrosis and epicardial adipose tissue. Int J Mol Sci. (2024) 25. doi: 10.3390/ijms25179481
40. Peng S, Shi S, Tao G, Li Y, Xiao D, Wang L, et al. JKAMP inhibits the osteogenic capacity of adipose-derived stem cells in diabetic osteoporosis by modulating the Wnt signaling pathway through intragenic DNA methylation. Stem Cell Res Ther. (2021) 12:120. doi: 10.1186/s13287-021-02163-6
41. Falini B, Brunetti L, Sportoletti P, Martelli MP. NPM1-mutated acute myeloid leukemia: from bench to bedside. Blood. (2020) 136:1707–21. doi: 10.1182/blood.2019004226
42. Zhang S, Zhang Y, Duan X, Wang B, Zhan Z. Targeting NPM1 epigenetically promotes postinfarction cardiac repair by reprogramming reparative macrophage metabolism. Circulation. (2024) 149:1982–2001. doi: 10.1161/circulationaha.123.065506
43. Mishra PK, Ying W, Nandi SS, Bandyopadhyay GK, Patel KK, Mahata SK. Diabetic cardiomyopathy: an immunometabolic perspective. Front Endocrinol (Lausanne). (2017) 8:72. doi: 10.3389/fendo.2017.00072
44. Volmer R, van der Ploeg K, Ron D. Membrane lipid saturation activates endoplasmic reticulum unfolded protein response transducers through their transmembrane domains. Proc Natl Acad Sci United States America. (2013) 110:4628–33. doi: 10.1073/pnas.1217611110
45. Rong X, Albert CJ, Hong C, Duerr MA, Chamberlain BT, Tarling EJ, et al. LXRs regulate ER stress and inflammation through dynamic modulation of membrane phospholipid composition. Cell Metab. (2013) 18:685–97. doi: 10.1016/j.cmet.2013.10.002
46. Zhang Q, Yao D, Rao B, Jian L, Chen Y, Hu K, et al. The structural basis for the phospholipid remodeling by lysophosphatidylcholine acyltransferase 3. Nat Commun. (2021) 12:6869. doi: 10.1038/s41467-021-27244-1
47. Li Z, Jiang H, Ding T, Lou C, Bui HH, Kuo MS, et al. Deficiency in lysophosphatidylcholine acyltransferase 3 reduces plasma levels of lipids by reducing lipid absorption in mice. Gastroenterology. (2015) 149:1519–29. doi: 10.1053/j.gastro.2015.07.012
48. Gawargi FI, Mishra PK. Regulation of cardiac ferroptosis in diabetic human heart failure: uncovering molecular pathways and key targets. Cell Death Discovery. (2024) 10:268. doi: 10.1038/s41420-024-02044-w
49. Iqbal S, Jabeen F, Kahwa I, Omara T. Suberosin alleviates thiazolidinedione-induced cardiomyopathy in diabetic rats by inhibiting ferroptosis via modulation of ACSL4-LPCAT3 and PI3K-AKT signaling pathways. Cardiovasc Toxicol. (2023) 23:295–304. doi: 10.1007/s12012-023-09804-7
50. Zhang Y, De Laurentiis E, Bohnsack KE, Wahlig M, Ranjan N, Gruseck S, et al. Ribosome-bound Get4/5 facilitates the capture of tail-anchored proteins by Sgt2 in yeast. Nat Commun. (2021) 12:782. doi: 10.1038/s41467-021-20981-3
51. Srivastava R, Zalisko BE, Keenan RJ, Howell SH. The GET system inserts the tail-anchored protein, SYP72, into endoplasmic reticulum membranes. Plant Physiol. (2017) 173:1137–45. doi: 10.1104/pp.16.00928
52. Zou F, Wang L, Liu H, Wang W, Hu L, Xiong X, et al. Sophocarpine suppresses NF-κB-mediated inflammation both In vitro and in vivo and inhibits diabetic cardiomyopathy. Front Pharmacol. (2019) 10:1219. doi: 10.3389/fphar.2019.01219
53. Gomez-Munoz L, Dominguez-Bendala J, Pastori RL, Vives-Pi M. Immunometabolic biomarkers for partial remission in type 1 diabetes mellitus. Trends Endocrinol Metab. (2024) 35:151–63. doi: 10.1016/j.tem.2023.10.005
54. Mathieu C, Lahesmaa R, Bonifacio E, Achenbach P, Tree T. Immunological biomarkers for the development and progression of type 1 diabetes. Diabetologia. (2018) 61:2252–8. doi: 10.1007/s00125-018-4726-8
55. Guo Q, Zhu Q, Zhang T, Qu Q, Cheang I, Liao S, et al. Integrated bioinformatic analysis reveals immune molecular markers and potential drugs for diabetic cardiomyopathy. Front Endocrinol (Lausanne). (2022) 13:933635. doi: 10.3389/fendo.2022.933635
56. Cui M, Wu H, An Y, Liu Y, Wei L, Qi X. Identification of important modules and biomarkers in diabetic cardiomyopathy based on WGCNA and LASSO analysis. Front Endocrinol (Lausanne). (2024) 15:1185062. doi: 10.3389/fendo.2024.1185062
57. Miao X, Tang Z, Wang Y, Su G, Sun W, Wei W, et al. Metallothionein prevention of arsenic trioxide-induced cardiac cell death is associated with its inhibition of mitogen-activated protein kinases activation in vitro and in vivo. (2013) 220:277–85. doi: 10.1016/j.toxlet.2013.04.025
58. Xiong RQ, Li YP, Lin LP, Yao JY. Identification of potential biomarkers for diabetic cardiomyopathy using LC-MS-based metabolomics. Endocr Connect. (2024) 13. doi: 10.1530/EC-23-0384
59. Justice MJ, Siracusa LD, Stewart AF. Technical approaches for mouse models of human disease. Dis Models Mech. (2011) 4:305–10. doi: 10.1242/dmm.000901
60. Riehle C, Bauersachs J. Of mice and men: models and mechanisms of diabetic cardiomyopathy. Basic Res Cardiol. (2018) 114:2. doi: 10.1007/s00395-018-0711-0
61. Liu N, Olson EN. CRISPR modeling and correction of cardiovascular disease. Circ Res. (2022) 130:1827–50. doi: 10.1161/circresaha.122.320496
62. Boudina S, Abel ED. Diabetic cardiomyopathy revisited. Circulation. (2007) 115:3213–23. doi: 10.1161/circulationaha.106.679597
63. Justice MJ, Dhillon P. Using the mouse to model human disease: increasing validity and reproducibility. Dis Models Mech. (2016) 9:101–3. doi: 10.1242/dmm.024547
64. Subramanian A, Tamayo P, Mootha VK, Mukherjee S, Ebert BL, Gillette MA, et al. Gene set enrichment analysis: a knowledge-based approach for interpreting genome-wide expression profiles. Proc Natl Acad Sci United States America. (2005) 102:15545–50. doi: 10.1073/pnas.0506580102
65. Kanehisa M, Goto S. KEGG: kyoto encyclopedia of genes and genomes. Nucleic Acids Res. (2000) 28:27–30. doi: 10.1093/nar/28.1.27
Keywords: type 1 diabetes, diabetic cardiomyopathy, endoplasmic reticulum stress, immune infiltration, bioinformatics, marker genes
Citation: Tang Q, Ji Y, Xia Z, Zhang Y, Dong C, Sun Q and Lei S (2025) Identification and validation of endoplasmic reticulum stress-related diagnostic biomarkers for type 1 diabetic cardiomyopathy based on bioinformatics and machine learning. Front. Endocrinol. 16:1478139. doi: 10.3389/fendo.2025.1478139
Received: 09 August 2024; Accepted: 28 February 2025;
Published: 18 March 2025.
Edited by:
Ying Xin, Jilin University, ChinaReviewed by:
Yuriy L Orlov, I.M. Sechenov First Moscow State Medical University, RussiaCopyright © 2025 Tang, Ji, Xia, Zhang, Dong, Sun and Lei. This is an open-access article distributed under the terms of the Creative Commons Attribution License (CC BY). The use, distribution or reproduction in other forums is permitted, provided the original author(s) and the copyright owner(s) are credited and that the original publication in this journal is cited, in accordance with accepted academic practice. No use, distribution or reproduction is permitted which does not comply with these terms.
*Correspondence: Qian Sun, cXVlZW5pZV9zdW5Ad2h1LmVkdS5jbg==; Shaoqing Lei, bGVpc2hhb3FpbmdAMTYzLmNvbQ==
†These authors have contributed equally to this work and share first authorship
Disclaimer: All claims expressed in this article are solely those of the authors and do not necessarily represent those of their affiliated organizations, or those of the publisher, the editors and the reviewers. Any product that may be evaluated in this article or claim that may be made by its manufacturer is not guaranteed or endorsed by the publisher.
Research integrity at Frontiers
Learn more about the work of our research integrity team to safeguard the quality of each article we publish.