- 1Key Laboratory of Xin’an Medicine, Ministry of Education, Anhui University of Chinese Medicine, Hefei, Anhui, China
- 2School of Graduate, Anhui University of Chinese Medicine, Hefei, Anhui, China
- 3Research and Technology Center, Anhui University of Chinese Medicine, Hefei, Anhui, China
- 4State Key Laboratory of Tea Plant Biology and Utilization, School of Tea and Food Science and International Joint Laboratory on Tea Chemistry and Health Effects of Ministry of Education, Anhui Agricultural University, Hefei, Anhui, China
- 5Institute of Surgery, Anhui Academy of Chinese Medicine, Anhui University of Chinese Medicine, Hefei, Anhui, China
Background: Diabetes that only appears or is diagnosed during pregnancy is referred to as gestational diabetes mellitus (GDM). The maternal physiological immune profile is essential for a positive pregnancy outcome. However, the causal relationship between GDM and immunophenotypes is not fully defined.
Methods: Based on the high-density genetic variation data at the genome-wide level, we evaluated the logical associations between 731 specific immune mediators and GDM using bidirectional Mendelian randomization (MR). The inverse variance weighted (IVW) was the main method employed for MR analysis. We performed multiple methods to verify the robustness and dependability of the MR results, and sensitivity measures were applied to rule out potential heterogeneity and horizontal pleiotropy.
Results: A substantial causal association between several immune mediators and GDM was detected. After FDR testing, HLA DR++ monocyte %leukocyte and HLA DR on plasmacytoid DC were shown to increase the risk of GDM; in contrast, CD127 on CD28+ CD45RA+ CD8br and CD19 on PB/PC were shown to attenuate the effect of GDM. Moreover, the progression of GDM has been shown to decrease the maternal levels of CD39+ activated Treg AC, CD39+ activated Treg %CD4 Treg, CD39+ resting Treg AC, CD39+ resting Treg %CD4 Treg, and CD39+ CD8BR %T cell.
Conclusions: Our findings support a possible causal association between GDM and various immunophenotypes, thus facilitating the provision of multiple options for preventive recognition as well as for the diagnostic and therapeutic management of GDM in clinical practice.
Introduction
Numerous physiological changes take place in a woman’s body during pregnancy. Throughout the long gestation period, the main energy pathway received by the fetus is glucose from the mother’s placenta (1). While pregnancy progresses, the fetal need for glucose grows, which leads to gestational diabetes mellitus (GDM) in women with otherwise healthy glucose metabolism or potentially impaired glucose tolerance prior to pregnancy (2, 3). As a common complication of pregnancy worldwide, uncontrolled GDM poses a serious threat to the mother, fetus, and newborn, increasing the likelihood of adverse pregnancy reactions (e.g., gestational hypertension, infection, and metabolic ketoacidosis) as well as malignant pregnancy outcomes (e.g., preterm abortion, neonatal hypoglycemia, and postpartum depression) (4). The focus of GDM prevention efforts is on preconception or early pregnancy, with only a minority of women with GDM requiring pharmacological treatment, and the identification of reliable underlying risk markers is valuable for the timely detection and prognosis of GDM (5).
The growth of a fetus from conception to successful delivery is a significant challenge for the physiological functions and regulatory systems of the mother (6). Patients with GDM frequently experience metabolic issues, such as increased insulin resistance, and generally suffer from systemic mild inflammation and immune dysregulation (7). In GDM, various types of immune cells, particularly regulatory T cells (Tregs), adapt spontaneously to prevent pregnancy interruption. Besides this, there is a proportional increase in circulating monocyte activation and an elevated level of cytokines, including IL-12 and IL-23, in mid to late gestation compared to non-pregnant women (8). It is quite predictable that changes in the quantity or function of immune mediators are involved in the development of GDM. Regrettably, the conclusions of the current studies on the correlation between maternal immune profiles and GDM are not entirely consistent, which may be due to factors such as differences in the samples and flaws in the design.
Nowadays, genome-wide association study (GWAS) and Mendelian randomization (MR) make it feasible to estimate the causal associations between immune traits and disease events on a large scale. GWAS identifies genome-wide sequence variation in specific human populations (9), whereas the existence of randomness in the process of genetic variation allows MR to be independent of common confounders and reverse causation (10). In this current study, by identifying single-nucleotide polymorphisms (SNPs) linked to complex traits, a comprehensive bivariate MR analysis was undertaken to identify causal relationships between immunocyte features and GDM. Figure 1 provides an illustration of this research.
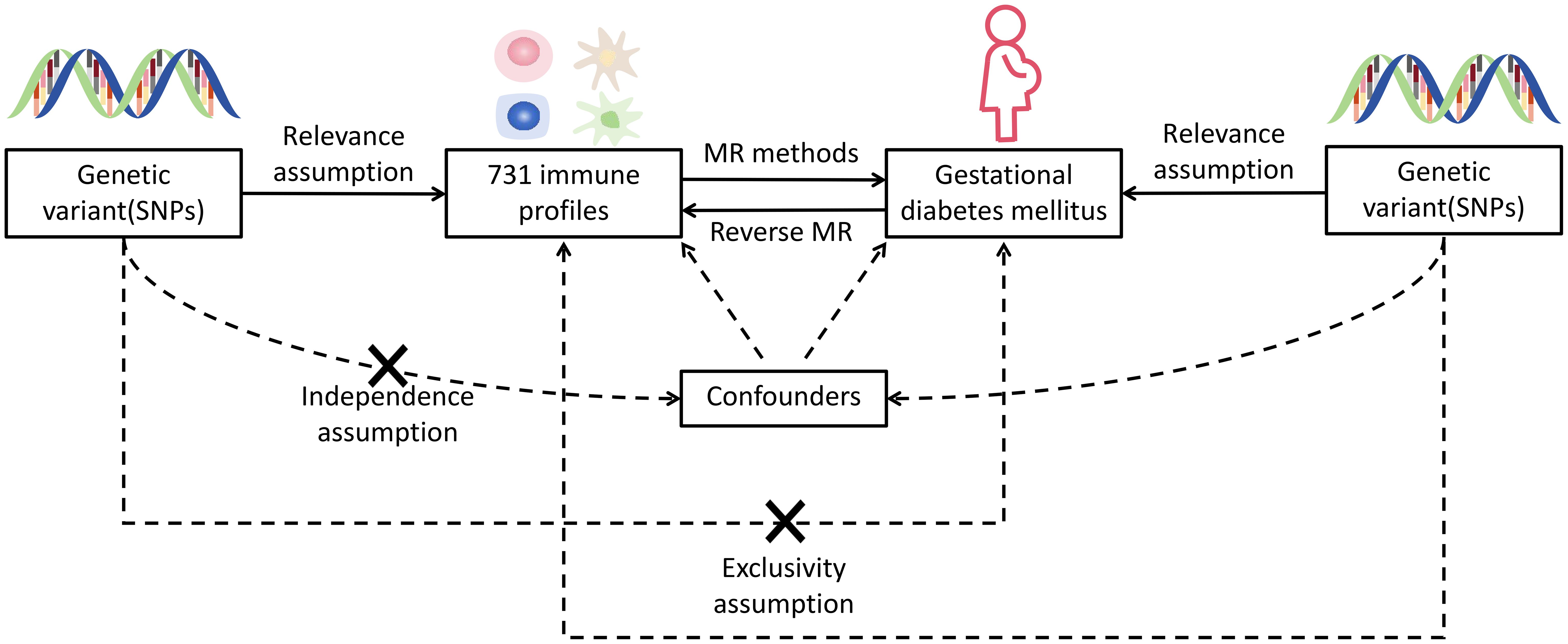
Figure 1 Hypothetical design of MR studies. Relevance: there is a significant relationship between exposure variables and genetic variations: genetic variants and confounding factors are independent of each other; exclusivity: genetic variation affects outcomes only through exposure and not by other means. X means no direct association.
Materials and methods
Study design
In this present research, we assessed the causal associations between 731 immunocyte features and GDM using MR analyses. MR employs genetic variants with strong correlations to exposure factors as instrumental variables (IVs), and IVs involved in causal inference have to comply three major assumptions of relevance, independence, and exclusivity (11).
Sources of data on exposure
The initial immune traits were performed with data from 3,757 Europeans, and the summary data for all traits are available from the publicly available GWAS catalogue (from GCST90001391 to GCST90002121) (12). In order to identify genetic variations occurring in 731 immune cells, GWAS detected approximately 20 million SNPs and 1.6 million indels through high-density genotyping and with reference to Sardinian sequences (13). Specifically, the characterization of immunophenotypes includes four immune trait types with median fluorescence intensities (MFI), relative count (RC), absolute count (AC), and morphological parameter (MP) as well as seven panels with B cell, Treg, myeloid cell, maturation stages, myeloid cell, maturation stages of T cell, monocyte, TBNK, and cDC.
Sources of data on outcome
The GWAS summary statistics for GDM were derived from finn-bGEST_DIABETES (14). For the study, GWAS were performed on 123,579 Europeans (Ncase = 5,687, Ncontrol = 117,892), and approximately 16 million variants were analyzed after quality control and filtering. All of the original research described above were publicly available, anonymous, and de-identified.
Screening of relevant IVs
To assure the relevance of causal inferences between immune characteristics and GDM, with reference to previous MR studies, we set the significance threshold of IVs for each immune characteristic at 1 × 10-5 (15). Considering the quantity of SNPs obtained, we scaled the significance level for immune cells to 5 × 10-8 in reverse extrapolation (16). Since genetic variants with similar genomic locations are more inclined to be co-inherited, in order to assure the independence among genetic tools and remove linkage disequilibrium (LD), we restricted the r2 value to 0.001, with a window range of 10,000 kb (r2 = 2 × MAF × [1 - MAF] × [β/SD]2; MAF: minor allele frequency; β: effect value of SNP on exposure factors) (17). In addition, a strong or weak bias in IVs also leads to weaker correlations with exposure. We have consequently selected the obtained IVs by means of F-statistic (F = (N - K - 1) × r2/[(1 - r2) × K]; N: sample size of GWAS; K: number of IVs), when the F value is above 10, it is generally thought that there are no significantly weak IVs (18).
Statistical analysis
We conducted MR analysis with the two-sample MR package (version 0.5.8) in R software (version 4.3.2) and adopted inverse-variance weighted (IVW) as the primary analytical method to estimate the causal effects of genes on traits (19, 20). Statistical approaches such as MR Egger, weighted median, and simple and weighted mode were also utilized to validate the MR results (21, 22). As a widely applied method of MR analysis, IVW is characterized by regressions that ignore the presence of the intercept term and are fitted with the inverse of the outcome variance as weights. MR Egger enables the estimation of bias among causal relationships when there is significant horizontal pleiotropy between SNPs. Even though half of the data were derived from genetic variation in invalid SNPs, the weighted median still yielded a consistent evaluation of causality. Furthermore, leave-one-out method was carried out to remove each SNP one by one, determine the meta impact of the SNPs that remained, and track the modifications in the results following the removal of every SNP (23). If the results changed remarkably after removing a certain SNP, it means that it has a significant effect on the results.
Results
Determining the causal role of immunophenotypes in GDM
During our investigation, we performed two-sample MR analyses utilizing the IVW method as the principal methodology. After F-statistics as well as an initial significance test, a total of 34 immune cells were detected to exhibit a causal association on GDM, including twelve B cell, seven Treg, four monocyte, four maturation stages of T cell, three TBNK, three cDC, and one myeloid cell panel. With further adjustment for FDR (PFDR< 0.04), we identified a total of four GDM risk immunophenotypes, which were respectively classified as Treg, B cell, TBNK, and cDC panel (Supplementary Table S1). Since then, we have found that certain alterations in the immune milieu influenced the progression of GDM. Concretely, the odds ratio (OR) of CD127 on CD28+ CD45RA+ CD8br on GDM risk was estimated to be approximately 0.919 (95% CI: 0.860–0.982, P = 0.0125, PFDR = 0.040) by the IVW method, whereas the MR Egger (95% CI: 0.830–1.071, OR = 0.943, P = 0.375) and weighted median (95% CI: 0.827–0.998, OR = 0.909, P = 0.045) analyses were consistent with the IVW. Simple mode (95% CI: 0.743–1.035, OR = 0.877, P = 0.138) and weighted mode (95% CI: 0.764–1.003, OR = 0.876, P = 0.071) also supported the genetic causal inference. The OR of CD19 on PB/PC on GDM risk was estimated to be 0.902 (95% CI: 0.839–0.970, P = 0.005, PFDR = 0.038) by the IVW method, whereas the MR Egger (95% CI: 0.770–1.009, OR = 0.881, P = 0.083) and weighted median (95% CI: 0.820–1.013, OR = 0.911, P = 0.085) analyses were consistent with the IVW. The OR of HLA DR on plasmacytoid DC on GDM risk was estimated to be approximately 1.078 (95% CI: 1.039–1.120, P = 8.76 × 10-5, PFDR = 0.003) by the IVW method, whereas MR Egger (95% CI 1.044-1.164, OR = 1.103, P = 0.002) and weighted median (95% CI 1.079-1.179, OR = 1.128, P = 1.07×10-7) analyses were consistent with the IVW. The odds ratio of HLA DR++ monocyte %leukocyte on GDM risk was estimated to be approximately 1.153 (95% CI 1.059-1.256, P = 0.001, PFDR = 0.018) by the IVW method, whereas the MR Egger (95% CI: 0.924–1.259, OR = 1.079, P = 0.371) and weighted median (95% CI: 1.043–1.314, OR = 1.170, P = 0.008) analyses were consistent with the IVW (Figure 2; Supplementary Table S2). Scatter plots and leave-one-out plots also support the stability of the results (Supplementary Figures S1, 2).
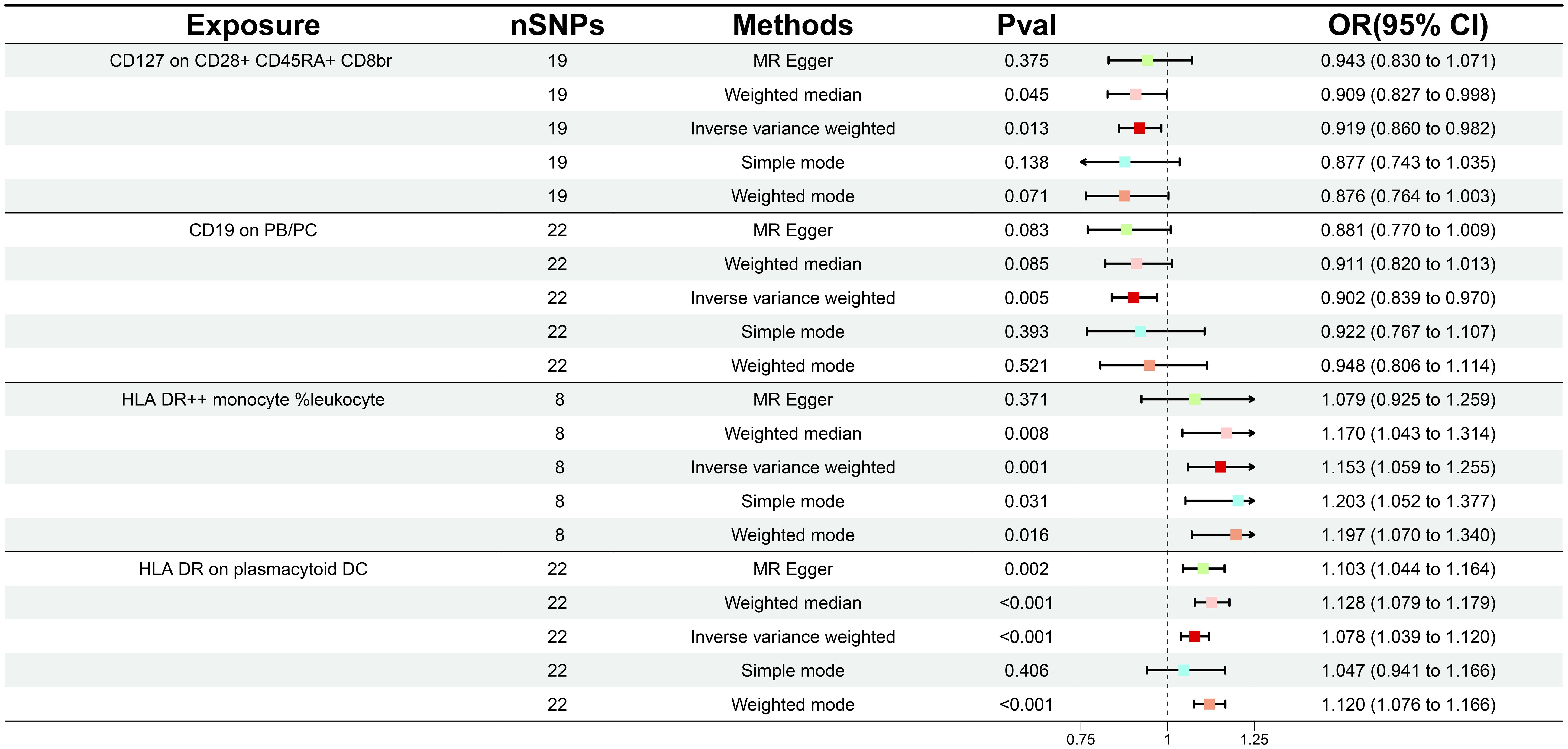
Figure 2 Forest plot illustrating the various ways in which the four immunological features and gestational diabetes mellitus are causally related.
Inferring causality of GDM on immunophenotypes
In order to ascertain the relationship between the onset and development of GDM with the body’s immunity, we verified the causality of GDM on 36 immune traits. Following GDM, the levels of five immunological features were found to change significantly (Supplementary Table 3); all of these traits belonged to the Treg panel and were adjusted for FDR (PFDR< 0.03). Interestingly, We found that GDM caused the CD39+ activated Treg %CD4 Treg (β = -0.183, 95% CI: 0.739–0.939, P = 0.003, PFDR = 0.024), CD39+ activated Treg AC (β = -0.183, 95% CI: 0.738–0.939, P = 0.003, PFDR = 0.021), CD39+ resting Treg % CD4 Treg (β = -0.183, 95% CI: 0.741–0.935, P = 0.002, PFDR = 0.024), CD39+ resting Treg AC (β = -0.164, 95% CI: 0.755–0.955, P = 0.006, PFDR = 0.028), and CD39+ CD8BR %T cell (β = -0.176, 95% CI: 0.744–0.946, P = 0.004, PFDR = 0.025) levels to show a similar decrease (Figure 3; Supplementary Table S4). The results from other MR methods and sensitivity analyses demonstrate the robustness of the observed causal associations (Supplementary Figures S3, 4).
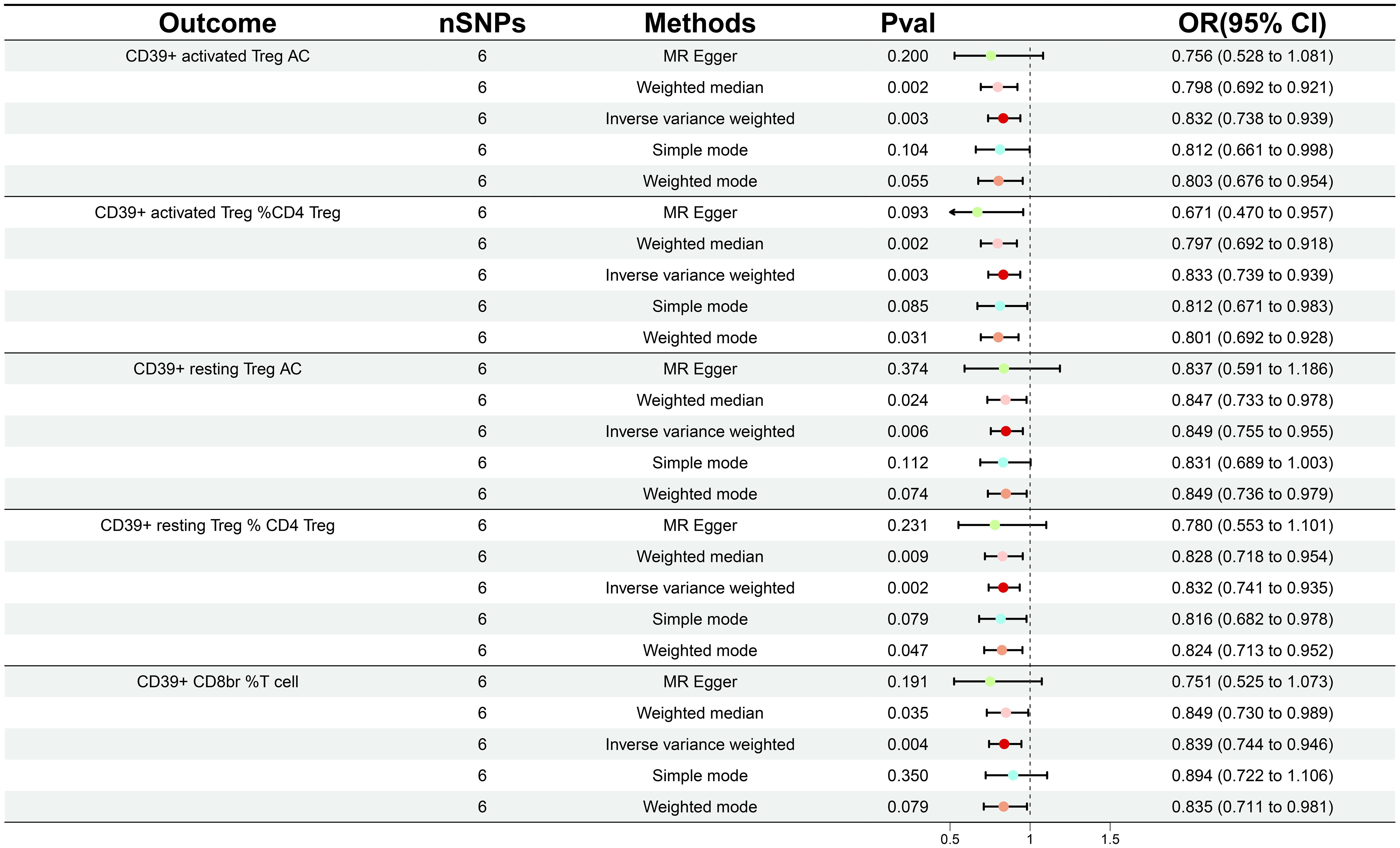
Figure 3 Forest plot illustrating the various ways in which gestational diabetes mellitus and five immunological features are causally related.
Discussion
GWAS studies have revealed associations between diseases and genetic variation, etc. Benefitting from a large, publicly available genomic data, our study attempted to offer genetic evidence for the causal link between GDM and multiple immune phenotypes. In this paper, we proved that four immune phenotypes have a significant causal association for GDM (PFDR< 0.04), while GDM has the same relationship for five other immune phenotypes (PFDR< 0.03).
As a globally prevalent obstetric disorder, GDM has been shown to be associated with many adverse maternal and fetal pregnancy outcomes. Abnormal maternal immune adaptation is key to the low-grade inflammation associated with the diagnosis of GDM, while immune cell infiltration of visceral adipose tissue causes the pathological dysregulation of insulin signaling and contributes to insulin resistance. Specifically, CD39 is located on the surface of trophoblast cells in the normal human placenta and regulates ATP-dependent trophoblast function, which is critical for immune tolerance and the maintenance of a normal pregnancy (24). Several studies have shown that measuring CD19+ subpopulations can help predict the pregnancy outcome in women, with a trend towards lower peripheral CD19+ B cells in women who miscarry compared to those who subsequently give birth (25). The frequency of CD19(+) CD5(+) cells was also significantly increased in the peripheral blood of patients with pre-eclampsia compared to normal pregnant women (26). In addition, the maternal humoral response to fetal anti-HLA-DR immunoglobulin antibodies may influence the development of pregnancy-induced hypertension (27).
Our results demonstrate that the elevated levels of HLA DR on plasmacytoid DC and HLA DR++ monocyte %leukocyte increase the risk of GDM. As a specialized antigen-presenting cell, dendritic cells (DCs) regulate the immune response and bridge the gap between innate and adaptive immunity (28). DCs play crucial roles in the growth and development of embryos and fetuses in the mother’s womb; dysregulation of the DC subpopulations appears to be linked to adverse pregnancy outcomes (29). HLA-DR is the most common MHC class II molecule on the surface of antigen-presenting cells. Specially, the expression of HLA-DR on the surface of DCs increases the abundance of protein complexes and is accompanied by the production of co-stimulatory molecules and cytokines (30). Moreover, recent studies have demonstrated that the level of HLA-DR is an indicator of monocyte immunocompetence, which not only assists in antigen presentation but also strengthens TLR-2-mediated signaling, cell proliferation, and maturation (31).
On the contrary, the growing levels of CD127 on CD28+ CD45RA+ CD8br and CD19 on PB/PC decrease the risk of GDM. The reduced activity of inhibitory Treg isoforms in GDM was pointed out to be associated with the upregulation of pro-inflammatory factor concentrations which include IL-6 and TNF-alpha (32). Another study showed that the percentage of circulating Treg subpopulation cells defined by CD3+CD4+CD25 bright/dim CD127 expression was reduced in GDM pregnancies compared with glucose-tolerant pregnancies (33). CD19 is a transmembrane protein on the surface of B cells, which is tightly connected with B cell activation, signaling, and growth regulation. For IgG4-related diseases, the peripheral blood was significantly enriched in B cell populations, including CD19+ CD24-CD38hi PB/PC. After glucocorticoid administration, the levels of these cells declined, accompanied by an improvement in clinical symptoms (34). In summary, our research indicates that immune cells have a significant role in GDM’s early diagnosis, therapeutic monitoring, disease activity assessment, and adaptive therapies.
On the other hand, we also revealed that the percentage of various immunological phenotypes was altered as a result of GDM. Strikingly, we found that GDM commonly lowered the abundance of CD39+ activated Treg %CD4 Treg, CD39+ activated Treg AC, CD39+ resting Treg % CD4 Treg, CD39+ resting Treg AC, and CD39+ CD8BR %T cell. Extracellular ATP is an effective proinflammatory factor in vivo, and its hydrolysis is important for its immunosuppressive function. As an extracellular ectonucleotidase, CD39 has been implicated as a major marker of FOXP3+ Treg and cleaves ATP to form AMP in the rate-limiting step. It is notable that the percentage of D39+ Treg cells was significantly decreased in type 2 diabetes patients as compared to the controls. In vivo experiments have shown that CD39-deficient mice exhibit impaired glucose tolerance in an oral glucose tolerance test (35). The supplementation of soluble CD39 to pre-diabetic NOD mice reduces the extent of extracellular ATP, inhibits the multiplication of CD4+ T cells, and delays the further progression of diabetes (36). It reminds us that individualized treatment for CD39 is probably a promising option for pregnant women.
Apparently, our study offers a foundation for delineating the intricate causal association between immune cells and GDM. However, there are still several limitations in our work. Firstly, although we performed MR analyses with a large-scale GWAS cohort and avoided potential confounders or reverse causation, genetic heterogeneity among different human populations still attenuates the credibility and validity of the GWAS results. Secondly, when examining the association of immune cells with GDM, a more relaxed threshold was chosen to ensure accurate data on SNPs. Even with the FDR test applied, this may still lead to a minor bias in the results. Finally, for GDM, our study was unable to further probe specific traits (for example—age, weight, and hormone levels) in the group of pregnant women.
Conclusions
In summary, we emphasized the causal relationship between a number of immune phenotypes and GDM through a full bidirectional MR analysis. To our knowledge, this is the first MR analysis carried out between immune phenotypes and GDM, providing novel insights into understanding the delicate balance between maternal immune mediators and GDM. GDM is a complicated and dynamic condition, and the pathophysiological mechanisms are not fully clarified. This research enables researchers to better explain the physiological mechanisms, with a view to filtering and monitoring high-risk groups for GDM, contributing to the early intervention and the development of new treatments of GDM.
Data availability statement
The datasets presented in this study can be found in online repositories. The names of the repository/repositories and accession number(s) can be found in the article/Supplementary Material.
Author contributions
ZJ: Conceptualization, Data curation, Investigation, Visualization, Writing – original draft. CZ: Conceptualization, Data curation, Visualization, Writing – original draft. JY: Supervision, Validation, Writing – review & editing. QH: Validation, Visualization, Writing – review & editing. XZ: Validation, Visualization, Writing – review & editing. DY: Validation, Visualization, Writing – review & editing. NX: Formal Analysis, Funding acquisition, Project administration, Supervision, Writing – review & editing. JC: Formal Analysis, Funding acquisition, Project administration, Supervision, Writing – review & editing.
Funding
The author(s) declare that financial support was received for the research, authorship, and/or publication of this article. This study was supported by the Research Project of Universities in Anhui Province (2023AH050835), the Research Funds of Joint Research Center for Food Nutrition and Health of IHM (2023SJY02-2), the Natural Science Key Programs for Colleges and Universities in Anhui Province (2022AH050894), the Research and Development of Key Technologies for Intelligent Processing and High Value Utilization of High Quality Tea (2021YFD1601102), as well as the Open Fund of State Key Laboratory of Tea Plant Biology and Utilization (SKLTOF20210101). None of the funders were involved in the study design, data processing and manuscript editing. No additional external funding was received for this study.
Acknowledgments
Special thanks to the GWAS participants and researchers involved in the systematic statistics of the data.
Conflict of interest
The authors declare that the research was conducted in the absence of any commercial or financial relationships that could be construed as a potential conflict of interest.
Publisher’s note
All claims expressed in this article are solely those of the authors and do not necessarily represent those of their affiliated organizations, or those of the publisher, the editors and the reviewers. Any product that may be evaluated in this article, or claim that may be made by its manufacturer, is not guaranteed or endorsed by the publisher.
Supplementary material
The Supplementary Material for this article can be found online at: https://www.frontiersin.org/articles/10.3389/fendo.2024.1358144/full#supplementary-material
References
1. Vince K, Perković P, Matijević R. What is known and what remains unresolved regarding gestational diabetes mellitus (GDM). J Perinat Med. (2020) 48:757–63. doi: 10.1515/jpm-2020-0254
2. Retnakaran R, Ye C, Hanley AJ, Connelly PW, Sermer M, Zinman B. Treatment of gestational diabetes mellitus and maternal risk of diabetes after pregnancy. Diabetes Care. (2023) 46:587–92. doi: 10.2337/dc22-1786
3. Lende M, Rijhsinghani A. Gestational diabetes: overview with emphasis on medical management. Int J Environ Res Public Health. (2020) 17:9573. doi: 10.3390/ijerph17249573
4. Mistry SK, Das Gupta R, Alam S, Kaur K, Shamim AA, Puthussery S. Gestational diabetes mellitus (GDM) and adverse pregnancy outcome in South Asia: A systematic review. Endocrinol Diabetes Metab. (2021) 4:e00285. doi: 10.1002/edm2.285
5. Juan J, Yang H. Prevalence, prevention, and lifestyle intervention of gestational diabetes mellitus in China. Int J Environ Res Public Health. (2020) 17:9517. doi: 10.3390/ijerph17249517
6. Chu AHY, Godfrey KM. Gestational diabetes mellitus and developmental programming. Ann Nutr Metab. (2020) 76 Suppl 3:4–15. doi: 10.1159/000509902
7. McElwain CJ, McCarthy FP, McCarthy CM. Gestational diabetes mellitus and maternal immune dysregulation: what we know so far. Int J Mol Sci. (2021) 22:4261. doi: 10.3390/ijms22084261
8. Tagoma A, Haller-Kikkatalo K, Oras A, Roos K, Kirss A, Uibo R. Plasma cytokines during pregnancy provide insight into the risk of diabetes in the gestational diabetes risk group. J Diabetes Investig. (2022) 13:1596–606. doi: 10.1111/jdi.13828
9. Tam V, Patel N, Turcotte M, Bossé Y, Paré G, Meyre D. Benefits and limitations of genome-wide association studies. Nat Rev Genet. (2019) 20:467–84. doi: 10.1038/s41576-019-0127-1
10. Bowden J, Holmes MV. Meta-analysis and Mendelian randomization: A review. Res Synth Methods. (2019) 10:486–96. doi: 10.1002/jrsm.1346
11. Richmond RC, Davey Smith G. Mendelian randomization: concepts and scope. Cold Spring Harb Perspect Med. (2022) 12:a040501. doi: 10.1101/cshperspect.a040501
12. Orrù V, Steri M, Sidore C, Marongiu M, Serra V, Olla S, et al. Complex genetic signatures in immune cells underlie autoimmunity and inform therapy. Nat Genet. (2020) 52:1036–45. doi: 10.1038/s41588-020-0684-4
13. Sidore C, Busonero F, Maschio A, Porcu E, Naitza S, Zoledziewska M, et al. Genome sequencing elucidates Sardinian genetic architecture and augments association analyses for lipid and blood inflammatory markers. Nat Genet. (2015) 47:1272–81. doi: 10.1038/ng.3368
14. Kurki MI, Karjalainen J, Palta P, Sipilä TP, Kristiansson K, Donner KM, et al. FinnGen provides genetic insights from a well-phenotyped isolated population. Nature. (2023) 613:508–18. doi: 10.1038/s41586-022-05473-8
15. Gu J, Yan GM, Kong XL, Zhang YY, Huang LH, Lu HM. Assessing the causal relationship between immune traits and systemic lupus erythematosus by bi-directional Mendelian randomization analysis. Mol Genet Genomics. (2023) 298:1493–503. doi: 10.1007/s00438-023-02071-9
16. Wang C, Zhu D, Zhang D, Zuo X, Yao L, Liu T, et al. Causal role of immune cells in schizophrenia: Mendelian randomization (MR) study. BMC Psychiatry. (2023) 23:590. doi: 10.1186/s12888-023-05081-4
17. Purcell S, Neale B, Todd-Brown K, Thomas L, Ferreira MA, Bender D, et al. PLINK: a tool set for whole-genome association and population-based linkage analyses. Am J Hum Genet. (2007) 81:559–75. doi: 10.1086/519795
18. Brion MJ, Shakhbazov K, Visscher PM. Calculating statistical power in Mendelian randomization studies. Int J Epidemiol. (2013) 42:1497–501. doi: 10.1093/ije/dyt179
19. Yavorska OO, Burgess S. MendelianRandomization: an R package for performing Mendelian randomization analyses using summarized data. Int J Epidemiol. (2017) 46:1734–9. doi: 10.1093/ije/dyx034
20. Yang Q, Borges MC, Sanderson E, Magnus MC, Kilpi F, Collings PJ, et al. Associations between insomnia and pregnancy and perinatal outcomes: Evidence from mendelian randomization and multivariable regression analyses. PloS Med. (2022) 19:e1004090. doi: 10.1371/journal.pmed.1004090
21. Burgess S, Thompson SG. Interpreting findings from Mendelian randomization using the MR-Egger method. Eur J Epidemiol. (2017) 32:377–89. doi: 10.1007/s10654-017-0255-x
22. Bowden J, Davey Smith G, Haycock PC, Burgess S. Consistent estimation in mendelian randomization with some invalid instruments using a weighted median estimator. Genet Epidemiol. (2016) 40:304–14. doi: 10.1002/gepi.21965
23. Wu F, Huang Y, Hu J, Shao Z. Mendelian randomization study of inflammatory bowel disease and bone mineral density. BMC Med. (2020) 18:312. doi: 10.1186/s12916-020-01778-5
24. McRae JL, Russell PA, Chia JS, Dwyer KM. Overexpression of CD39 protects in a mouse model of preeclampsia. Nephrol (Carlton). (2013) 18:351–5. doi: 10.1111/nep.12058
25. Tu W, Li Y, Ding Q, Wang L, Frempong ST, Ruan J, et al. Association between peripheral CD19+ B cells and reproductive outcome in women with recurrent implantation failure. Clin Lab. (2020) 66. doi: 10.7754/Clin.Lab.2019.190510
26. Lewicki S, Skopińska-Różewska E, Zdanowski R. The decrease in number of splenic lymphocytes in mice fed Rhodiola kirilowii during pregnancy and lactation concerns mainly CD19+ and CD4+ cells. Cent Eur J Immunol. (2017) 42:331–5. doi: 10.5114/ceji.2017.72826
27. Hoff C, Peevy K, Giattina K, Spinnato JA, Peterson RD. Maternal-fetal HLA-DR relationships and pregnancy-induced hypertension. Obstet Gynecol. (1992) 80:1007–12.
28. Khan FU, Khongorzul P, Raki AA, Rajasekaran A, Gris D, Amrani A. Dendritic cells and their immunotherapeutic potential for treating type 1 diabetes. Int J Mol Sci. (2022) 23:4885. doi: 10.3390/ijms23094885
29. Friebe-Hoffmann U, Antony L, Kruessel JS, Pawlowski B, Hoffmann TK. Peripheral Immunological Cells in Pregnant Women and their Change during Diabetes. Exp Clin Endocrinol Diabetes. (2017) 125:677–83. doi: 10.1055/s-0043-104935
30. Guo CC, Jin YM, Lee KK, Yang G, Jing CX, Yang X. The relationships between HLA class II alleles and antigens with gestational diabetes mellitus: A meta-analysis. Sci Rep. (2016) 6:35005. doi: 10.1038/srep35005
31. Restrepo BI, Twahirwa M, Jagannath C. Hyperglycemia and dyslipidemia: Reduced HLA-DR expression in monocyte subpopulations from diabetes patients. Hum Immunol. (2021) 82:124–9. doi: 10.1016/j.humimm.2020.11.005
32. Siddiqui S, Waghdhare S, Jha S, Dubey S. Role of immunological markers in gestational diabetes mellitus-a brief review. Diabetes Metab Syndr. (2019) 13:2983–5. doi: 10.1016/j.dsx.2018.07.018
33. Lobo TF, Borges CM, Mattar R, Gomes CP, de Angelo AGS, Pendeloski KPT, et al. Impaired Treg and NK cells profile in overweight women with gestational diabetes mellitus. Am J Reprod Immunol. (2018) 79:e12810. doi: 10.1111/aji.12810
34. Lin W, Zhang P, Chen H, Chen Y, Yang H, Zheng W, et al. Circulating plasmablasts/plasma cells: a potential biomarker for IgG4-related disease. Arthritis Res Ther. (2017) 19:25. doi: 10.1186/s13075-017-1231-2
35. Chia JS, McRae JL, Cowan PJ, Dwyer KM. The CD39-adenosinergic axis in the pathogenesis of immune and nonimmune diabetes. J BioMed Biotechnol. (2012) 2012:320495. doi: 10.1155/2012/320495
Keywords: gestational diabetes mellitus, immunity, causal inference, genetic variation, Mendelian randomization
Citation: Ji Z, Zhang C, Yuan J, He Q, Zhang X, Yang D, Xu N and Chu J (2024) Is there a causal association between gestational diabetes mellitus and immune mediators? A bidirectional Mendelian randomization analysis. Front. Endocrinol. 15:1358144. doi: 10.3389/fendo.2024.1358144
Received: 19 December 2023; Accepted: 19 March 2024;
Published: 19 April 2024.
Edited by:
Åke Sjöholm, Gävle Hospital, SwedenReviewed by:
Erick Saul Sánchez Salguero, Monterrey Institute of Technology and Higher Education (ITESM), MexicoGlilciane Morceli, Minas Gerais State University, Brazil
Copyright © 2024 Ji, Zhang, Yuan, He, Zhang, Yang, Xu and Chu. This is an open-access article distributed under the terms of the Creative Commons Attribution License (CC BY). The use, distribution or reproduction in other forums is permitted, provided the original author(s) and the copyright owner(s) are credited and that the original publication in this journal is cited, in accordance with accepted academic practice. No use, distribution or reproduction is permitted which does not comply with these terms.
*Correspondence: Jun Chu, Q2h1akBhaHRjbS5lZHUuY24=; Na Xu, bmF4dTIwMTRAYWhhdS5lZHUuY24=
†These authors have contributed equally to this work