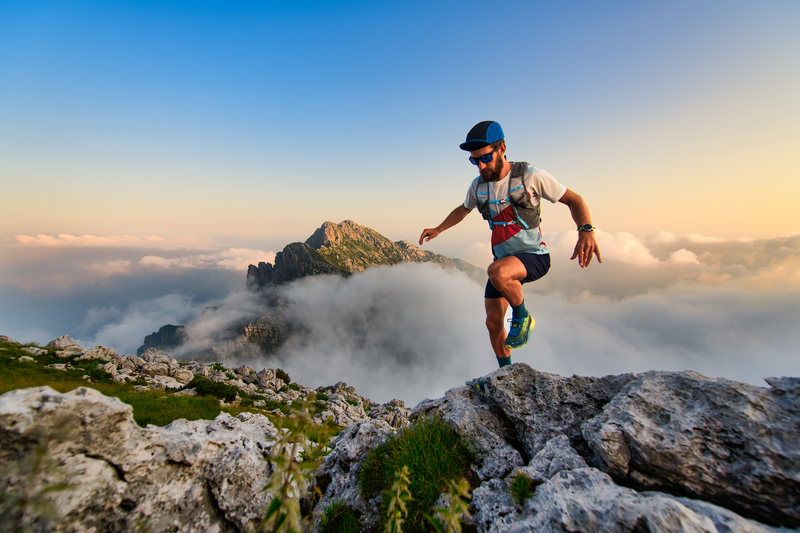
94% of researchers rate our articles as excellent or good
Learn more about the work of our research integrity team to safeguard the quality of each article we publish.
Find out more
ORIGINAL RESEARCH article
Front. Endocrinol.
Sec. Gut Endocrinology
Volume 15 - 2024 | doi: 10.3389/fendo.2024.1337245
The final, formatted version of the article will be published soon.
You have multiple emails registered with Frontiers:
Please enter your email address:
If you already have an account, please login
You don't have a Frontiers account ? You can register here
The gut microbiota plays a pivotal role in various metabolic disorders. Orlistat has shown beneficial effects on weight loss and metabolism, but its direct impact on the gut microbiota has not been extensively reported. Thus, this study aimed to explore the effects of orlistat on the gut microbiota in mice with high-fat diet-induced obesity. Thirty male C57BL/6J mice were randomly divided into a normal control group (fed a standard diet, N), and a model group (fed a 60% fat diet). A body weight exceeding the basal body weight by 130% defined a successfully established obesity model. The model group was further divided into a positive control group (fed a 60% fat diet, F), and an orlistat group (fed a 60% fat diet and treated with orlistat at 30 mg/kg, bid, A), with 10 mice in each group. The parameters assessed included weight loss, fasting plasma glucose (FPG) levels, and intestinal hormones. Gut microbiota diversity was analyzed using high-throughput sequencing. Orlistat treatment significantly reduced body weight and FPG levels, and increased glucagon-like peptide-1 (GLP-1) and gastric inhibitory polypeptide (GIP) levels in obese mice. High-fat diet-fed mice exhibited increased microbial diversity and richness, which were significantly diminished by orlistat administration. Additionally, orlistat treatment led to a significant decrease in the proportion of Bacteroidetes and an increase in the proportion of Helicobacter and Allobaculum. Notable shifts in the abundances of Bacteroidetes were observed, correlating with changes in several functional metabolic pathways, including "cell motility" and "neurodegenerative diseases." Co-occurrence network analysis suggested a more complex bacterial network in orlistat-treated mice, alongside a reduction in the density of bacterial correlation networks. Our study demonstrates that orlistat's beneficial effects on body weight, FPG, GLP-1, and GIP are likely mediated through modifications in the gut microbiota composition.
Keywords: Orlistat, Obesity, intestinal hormones, Gut Microbiota, high-throughput sequencing
Received: 29 Nov 2023; Accepted: 16 Dec 2024.
Copyright: © 2024 Xue, Wang, Chen, Wang, Zhang and Li. This is an open-access article distributed under the terms of the Creative Commons Attribution License (CC BY). The use, distribution or reproduction in other forums is permitted, provided the original author(s) or licensor are credited and that the original publication in this journal is cited, in accordance with accepted academic practice. No use, distribution or reproduction is permitted which does not comply with these terms.
* Correspondence:
Qiang Li, Shenzhen University General Hospital, Shenzhen, 518055, China
Disclaimer: All claims expressed in this article are solely those of the authors and do not necessarily represent those of their affiliated organizations, or those of the publisher, the editors and the reviewers. Any product that may be evaluated in this article or claim that may be made by its manufacturer is not guaranteed or endorsed by the publisher.
Research integrity at Frontiers
Learn more about the work of our research integrity team to safeguard the quality of each article we publish.