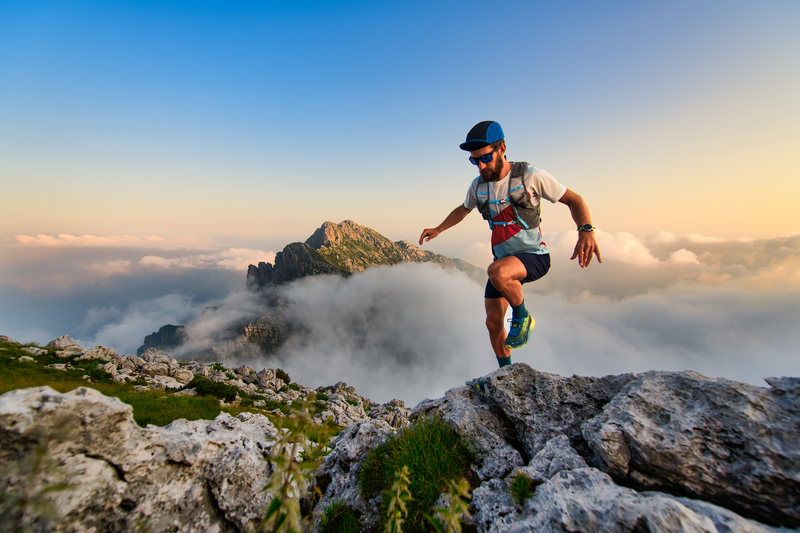
95% of researchers rate our articles as excellent or good
Learn more about the work of our research integrity team to safeguard the quality of each article we publish.
Find out more
ORIGINAL RESEARCH article
Front. Endocrinol. , 18 March 2022
Sec. Diabetes: Molecular Mechanisms
Volume 13 - 2022 | https://doi.org/10.3389/fendo.2022.853857
This article is part of the Research Topic Insights in Diabetes: Molecular Mechanisms 2021 View all 10 articles
Objective: The objective of this study was to reveal the potential crosstalk between immune infiltration and N6- methyladenosine (m6A) modification in the placentas of patients with gestational diabetes mellitus (GDM), and to construct a model for the diagnosis of GDM.
Methods: We analyzed imbalanced immune infiltration and differentially expressed m6A-related genes (DMRGs) in the placentas of patients with GDM, based on the GSE70493 dataset. An immune-related DMRG signature, with significant classifying power and diagnostic value, was identified using a least absolute shrinkage and selection operator (LASSO) regression. Based on the selected DMRGs, we developed and validated a nomogram model using GSE70493 and GSE92772 as the training and validation sets, respectively.
Results: Infiltration of monocytes was higher in GDM placentas than in control samples, while the infiltration of macrophages (M1 and M2) in GDM placentas was lower than in controls. A total of 14 DMRGs were strongly associated with monocyte infiltration, seven of which were significant in distinguishing patients with GDM from normal controls. These genes were CD81, CFH, FABP5, GBP1, GNG11, IL1RL1, and SLAMF6. The calibration curve, decision curve, clinical impact curve, and receiver operating characteristic curve showed that the nomogram recognized GDM with high accuracy in both the training and validation sets.
Conclusions: Our results provide clues that crosstalk between m6A modification and immune infiltration may have implications in terms of novel biomarkers and therapeutic targets for GDM.
Gestational diabetes mellitus (GDM) is a form of diabetes that is first diagnosed during pregnancy, with a worldwide prevalence of 9–21%. GDM frequently affects both short-term and long-term health in the mother and offspring, because of the diverse genetic background and epigenetic modifications that occur in response to nutritional and environmental factors (1, 2). Currently, the precise etiological mechanisms of GDM remain unclear; however, numerous studies have found that GDM is a multifactorial disease that involves genetic factors, lifestyle, and chronic inflammation. Insulin resistance (IR) and pancreatic β-cell dysfunction are regarded as essential for the pathogenesis of GDM (2, 3). Although the exact mechanisms remain to be clarified, chronic inflammation has been reported to participate in the development of IR and pancreatic β cell failure, which in turn leads to GDM (4). The placenta is a temporary organ formed during pregnancy, which serves as the only bridge connecting the mother and fetus, and has important endocrine function. Placenta-derived inflammatory cytokines, such as interleukin-1 beta (IL-1β), IL-6, IL-15, IL-10, IL-34, IL-38, and tumor necrosis factor alpha (TNF-α), can stimulate immune cells and aggravate immune and inflammatory responses, thereby exacerbating chronic inflammation and maternal IR and inducing β cell failure during pregnancy (5–11). Moreover, immune cells and inflammatory cytokines are important components of the placental microenvironment, which is essential for normal pregnancy (12, 13). Imbalanced immune infiltration in the placenta contributes to the pathogenesis and development of pregnancy-specific diseases, including GDM, and may affect GDM-related adverse pregnancy outcomes and clinical prognosis (13–15).
Chemical modifications of cellular RNAs can result in secondary structure modifications, splicing, degradation, or molecular stability, which are emerging layers of post-transcriptional gene regulation. More than 160 chemical modifications have been identified (16). N6- methyladenosine (m6A) RNA modification is the most prevalent type of RNA epigenetic processing (17, 18). m6A modification is mediated by its effector proteinsare in a dynamic and reversible pattern (17). m6A occurs mainly in the 3’- UTR and the vicinity of the termination codon mRNA, which is recogonized by “readers” (YTH domain family (YTHDF]1–3, and insulin-like growth factor 2 mRNA-binding proteins 1–3), catalyzed by methylases [methyltransferase-like (METTL)3/14, and Wilms’ tumour 1-associated protein], and removed by demethylases [fat-mass and obesity-associated protein (FTO), and alkylation repair homolog protein 5] (19). Recent evidence indicates that perturbations of m6A modifications dysregulate mRNA metabolism, including mRNA stability, mRNA splicing, RNA nucleation, RNA-protein teractions and mRNA translation, thereby contributing to various physiological and pathophysiological processes (20–22). Numerous m6A modifications have been shown to regulate adipogenesis, glucose metabolism, insulin resistance, and the related chronic immune response (17, 21, 23). This suggests that m6A modifications are implicated in the development of metabolic diseases, although the specific knowledge regarding GDM is still in its infancy.
In this study, we aimed to reveal the imbalanced immune infiltration in the placenta of patients with GDM, the differentially expressed m6A-related genes (DMRGs) involved, as well as the crosstalk between them, and also to develop a nomogram model for the diagnosis of GDM.
The human expression dataset GSE70493 was downloaded from the Gene Expression Omnibus database (https://www.ncbi.nlm.nih.gov/geo/). The expression data in GSE70493 contained 63 samples of the maternal placenta (GDM; n = 32 and normal glucose tolerance [NGT]; n = 31). We obtained 17,661 m6A-related genes by crossing data from the RMBase (24) and RMvar (25) databases. The expression data in GSE92772 were obtained for validation and contained RNA profiles of maternal whole blood cells from eight GDM and eight NGT pregnant women in their second trimester. The workflow of this research is shown in Figure 1.
Figure 1 Flowchart of the research workflow. Abbreviations are defined as follows: differentially expressed gene (DEG), gestational diabetes mellitus (GDM), differentially expressed m6A-related gene (DMRG), Gene Ontology (GO), Kyoto Encyclopedia of Genes and Genomes (KEGG), Pearson correlation coefficient (PCC), least absolute shrinkage and selection operator (LASSO).
Using the R software ‘limma’ package, we identified differentially expressed genes (DEGs) in the GSE70493 dataset, based on the criteria of |log2 fold change| > 0.1 and P value < 0.01. Heatmaps were generated using the R software ‘pheatmap’ package. In the case of multiple probes corresponding to the same gene, we selected the probe with the lowest P value. Genes without official symbols were removed, and all symbols were converted to symbols approved by the HUGO Gene Nomenclature Committee. We then crossed the DEGs with m6A-related genes to obtain the DMRGs.
To determine the potential functions and enriched pathways of DMRGs in GDM, Gene Ontology (GO) and Kyoto Encyclopedia of Genes and Genomes (KEGG) pathways were analyzed using the R software ‘enrichplot’ package. A P value of < 0.05 was set as the cutoff.
CIBERSORTx (26) was utilized to quantify the abundance of 22 immune cell types in each sample by imputing the gene expression profiles of GSE70493. We then compared the differences in immune cell infiltration between GDM patients and healthy subjects.
Pearson correlation coefficient (PCC) analysis was conducted to identify the DMRGs correlated with the differentially infiltrated immune cells between GDM and healthy patients. LASSO analysis, a linear regression model penalized with the L1 norm, was used to further narrow down the variables owing to its tendency to prefer solutions with fewer non-zero coefficients. A tuning parameter, lambda, was used to control the number of coefficients with a value of zero. The 10-fold K cross-validations for the centralization and normalization of selected variables to select the optimal lambda value using R software. The core DMRGs that correlated with immune infiltration were identified using LASSO analysis.
A logistic regression model was considered to evaluate the performance the core m6A-related genes selected by LASSO to estimate the probability of GDM. Based on this model, we constructed a nomogram for individual predictions of GDM using R software. To validate the classification ability of the nomogram model, calibration was analyzed using a bootstrapping approach and randomly repeated 1,000 times with replacement. Decision curve analysis and clinical impact curves were used to determine clinical usefulness. The receiver operating characteristic (ROC) curve was used to evaluate the sensitivity and specificity of the nomogram. To externally validate the nomogram, we then applied the calibration, decision curve, clinical impact curve, and ROC curve analysis on GSE92772.
Data processing and statistical analyses were performed using R software (version 4.0.3). Associations between quantitative variables were assessed using the Student’s t-test. Spearman’s rank correlation analysis was used to explore the correlations between different variables. LASSO regression, logistic regression, and nomogram development were conducted using “glmnet”, “survival”, and “rms” packages, respectively. P values < 0.05 were considered significant.
Based on the criteria described above, we identified 207 DEGs in GSE70493 and obtained 106 DMRGs by crossing the DEGs with 17,661 m6A-related genes. A heatmap of the DMRGs is shown in Figure 2. The list of all DMRGs is shown in Table S1. To elucidate the functions and pathways of the 106 DMRGs, we conducted enrichment analysis using R software. Based on the GO category biological process, DMRGs were mainly enriched for the terms interferon-gamma-mediated signaling pathway, antigen processing and presentation of endogenous antigen, positive regulation of leukocyte-mediated immunity, positive regulation of T cell-mediated cytotoxicity, cellular response to interferon-gamma, and cell killing (Table S2 and Figure 3A). KEGG pathways were mainly enriched in viral myocarditis, type 1 diabetes mellitus (T1DM), phagosomes, cell adhesion molecules, and autoimmune thyroid disease (Table S3 and Figure 3B).
Figure 2 Heatmap of differentially expressed m6A-related genes. The up- and down-regulation of genes are indicated with red and blue color, respectively.
Figure 3 Functional enrichment analysis. (A) The top 10 GO biological process categories. (B) The top 10 KEGG pathways.
We further explored differential immune cell infiltration in the placenta between GDM and control cases by quantifying the abundance of 22 immune cell types (Supplementary File 1). The results indicated that the infiltration of monocytes was higher in GDM placentas than in control samples, while the infiltration of macrophages M1 and M2 in GDM placentas were lower. No significant differences were observed among the other immune cells (Figure 4A). Higher propotion of M2 than M1 phenotype in GDM compared to controls was observed (Supplementary Figure 1).
Figure 4 Differentially expressed m6A-related genes (DMRGs) related to monocyte infiltration in GDM Pregnancies. (A) Landscape of immune infiltrations in GDM Pregnancies. (B) Correlation among the abundance of monocytes and 14 correlated DMRGs. Asterisks denote statistical significance (ns, no significance; *p < 0.05; **p < 0.01).
Considering the obviously high infiltration of monocytes in the GDM placentas, we calculated the PCCs of the abundance of monocytes and the expression levels of DMRGs. Fourteen DMRGs (methanethiol oxidase [SELENBP1], fatty acid-binding protein 5 [FABP5], G-protein coupled receptor 183 [GPR183], inhibitor of differentiation 4 [ID4], G-protein-coupled receptor 65 [GPR65], G-protein subunit γ 11 [GNG11], guanylate binding protein 1 [GBP1], complement factor H [CFH], tetraspanin [CD81], interleukin-1 receptor-like 1 [IL1RL1], cathepsin K [CTSK], sterile alpha motif domain-containing protein 9-like [SAMD9L], spermatogenesis associated serine-rich 2-like [SPATS2L], and signaling lymphocytic activation molecule family 6 [SLAMF6]), with a |PCC| > 0.3 and P value < 0.01, were selected from 106 DMRGs for further analysis (Figure 4B). Based on LASSO regression analysis, seven DMRGs (CD81, CFH, FABP5, GBP1, GNG11, IL1RL1, and SLAMF6) had nonzero coefficients, with a lambda coefficient of 0.1059 (Figures 5A, B). The count of the potential m6A modification sites are shown in Figure 5C. The expression matrix of the seven key DMRGs, based on the 63 samples, was extracted from the dataset GSE70493. The expression levels of CD81, CFH, GBP1, IL1RL1, and SLAMF6 in GDM samples were lower than those in control samples, while expression levels of FABP5 and GNG11 were higher in GDM placentas than those in controls (Figures 5D, E). As shown in Figure 4B, these seven DMRGs were significantly correlated with each other.
Figure 5 Differentially expressed m6A-related genes (DMRGs) signature selection through LASSO regression analysis. (A) LASSO coefficient profiles of 14 differentially expressed m6A-related genes (DMRGs). The coefficient profile plot was produced against the log (lambda). (B) The partial likelihood deviance (binomial deviance) curve was plotted versus log (lambda) to verify the optimal lambda value. Dotted vertical lines were drawn based on the 1-SE criteria. Seven DMRGs with non-zero coefficients were selected by optimal lambda. (C) Counts of potential m6A modification sites of the selected DMRGs. (D) Relative expression levels of CD81, CFH, FABP5, GBP1, GNG11, IL1RL1, and SLAMF6. (E) Hierarchical clustering of the expression pattern of CD81, CFH, FABP5, GBP1, GNG11, IL1RL1, and SLAMF6. Asterisks denote statistical significance ( *p < 0.05; **p < 0.01).
We extracted the expression matrix of the seven core DMRGs based on the training set of 63 samples extracted from dataset GSE70493. A model incorporating the DMRGs CD81, CFH, FABP5, GBP1, GNG11, IL1RL1, and SLAMF6 was developed and presented as a nomogram (Figure 6A). The probability of GDM was accurately predicted using a calibration curve (Figure 6B). The decision curve (Figure 6C) and clinical impact curve (Figure 6D) revealed that our model demonstrated a positive net benefit without increasing the number of false positives. In addition, ROC curve analysis revealed that the area under the curve (AUC) was 83% (Figure 6E), indicating a good classification ability of the nomogram model.
Figure 6 Development and internal validation of a nomogram model for GDM based on GSE70493. (A) Nomogram model for patients with GDM. (B) Calibration curve for predicting possibility of GDM. Decision curve (C) and clinical impact curve (D) for assessing the net benefit of the nomogram. (E) ROC curve to assess classifying ability of the nomogram model.
Considering that screening for GDM is usually performed during 24–28 weeks of gestation, we selected GSE92772 as the validation set to evaluate the diagnostic value of the core DMRG signature, which is based on blood samples extracted during the second trimester. GSE92772 contains the expression matrix of SLAMF6, FABP5, GBP1, GNG11, IL1RL1, and CD81, without CFH present. In the validation set, the calibration curve (Figure 7A), decision curve (Figure 7B), and clinical impact curve (Figure 7C) also exhibited good performance. Moreover, the nomogram model exhibited high diagnostic value in distinguishing patients with GDM from those with NGT, with an AUC value of 85.9% (Figure 7D).
Figure 7 Diagnostic value of the monocyte infiltration related DMRGs signature based on GSE92772. (A) Calibration curve to identify the diagnostic value for GDM. Decision curve (B) and clinical impact curve (C) for assessing the clinical usage. (D) ROC curve used for assessing the sensitivity and specificity of the model.
GDM is a common complication of pregnancy, adversely affecting both the mother and fetus (1, 2). The etiology of GDM, which involves genetic background and epigenetic modifications, remains unclear. Chronic low-grade inflammation during pregnancy can contribute to the pathogenesis of GDM by exacerbating maternal IR and inducing β cell failure (4). As an endocrine organ, the placenta derives inflammatory cytokines that stimulate immune cells and aggravates the immune/inflammatory response (12, 13). Moreover, the disturbance of immune cell infiltration in the placenta is attributed to pregnancy-specific diseases, including GDM, as well as GDM-related adverse outcomes (13–15). In this study, we found that the infiltration of monocytes was higher in GDM placentas than in control samples, while the infiltration level of macrophages (M1 and M2) in GDM placentas was lower than that in the controls. Monocyte infiltration has been shown to be crucial during inflammation. As important mediators of the innate immunity, monocytes circulate in the bloodstream and pass into tissues during the steady state and in increased quantities during inflammation (27). GDM is considered as a low-degree inflammation, and elevated levels of monocytes in the peripheral blood of patients with GDM have been previously reported (28). Based on the expression of superficial CD14 and CD16 in flow cytometry, monocytes can be divided into three subsets: classical (CD14++CD16-), intermediate (CD14+CD16++) and non-classical (CD14+CD16+) (29). Angelo et al. (28) observed increased percentage of classical monocytes, decreased frequency of intermediate monocytes in the peripheral blood of patients with GDM compared to controls. By contraries, an increase in the intermediate subset and a decreased frequency of classical monocytes were detected in healthy pregnancy compared to non-pregnant women (30). Considering that the variation in levels of monocyte subsets may contribute to the development of inflammation in GDM, it is essential to develop new studies on this topic to validate these findings. During gestation, bone marrow-derived monocytes can migrate from the bloodstream to the uterus and differentiate into decidua-specific macrophages upon exposure to this local microenvironment (31–34). A proportion of tissue-resident macrophages is constantly replaced by blood monocytes, and the mechanisms behind these differential renewal patterns are not fully understood and may be controlled by the tissue specific microenvironment (27, 35, 36). Inflammatory stimuli often depleted macrophages and induce monocyte recruitment; these monocytes might potentially contribute to tissue-resident macrophages upon the resolution of inflammation (27). Therefore, the decrease in macrophages and increased monocytes may be due to the inflammation during GDM. Decidual macrophages are highly plastic (37). It is generally accepted that macrophages are mainly the M1 (pro-inflammatory) phenotype during the pre-implantation period, and change to M2 (anti-inflammatory) phenotype following trophoblast attachment and invasion; macrophages seem to revert to M1 phenotype at the time of delivery (37–39). Inappropriate macrophage polarization may cause adverse pregnancy outcomes (30, 37). There are controversies regarding the use of placental macrophages in describing GDM. An imbalance of M2 to M1 macrophages has been observed in the placentas of diabetic patients and rats (40), as well as in placentas of GDM patients (41). Opposing conclusions have been reported in other studies, in which macrophages maintain the M2 phenotype in GDM compared to controls (42–44). In the present study, we also observed higher propotion of M2 than M1 phenotype in GDM compared to controls (Supplementary Figure 1).
m6A methylation plays a vital role in glucose/lipid metabolism as well as its related chronic inflammatory processes (23, 45–47). FTO moduates glucose metabolism via regulating forkhead box protein O1 and activating transcription factor 4 of m6A modification (46, 48). FTO also regulates adipogenesis by controlling cell cycle progression in an YTHDF2 dependent mechanism (45). METTL3 regulates lipid metabolism via mediating JAK1 mRNA stability an m6A-YTHDF2 dependent manner (47), and regulating NF-κB and MAPK via meditating m6A modification of TNF receptor associated factor 6 (49). Due to the dynamic and reversible nature, m6A methylation can be reversed by environmental stressors, including changes in nutrition. High-fat diet affecs METTL3 and FTO mRNA expression, and fasting state leads to the reduced FTO mRNA expression and increases m6A levels (50, 51). It remains unknown whether m6A modifications play a role in GDM. Exploration of the crosstalk between m6A modification and GDM may provide a potential strategy for the diagnosis, prognosis and treatment. We obtained m6A-related genes from the RMBase and RMvar databases and identified DMRGs based on the GSE70493 dataset. Enrichment analysis was conducted to determine the biological functions of the DMRGs. Notably, several pathways, such as type 1 diabetes mellitus and autoimmune thyroid disease, were closely correlated with the development and mal outcome of GDM. Recent studies have revealed that a small but significant population of patients with GDM develop postpartum T1DM (52, 53). Emerging evidence suggests that perturbations of the thyroid hormone signaling pathway and antibodies are associated with GDM development and adverse outcomes (54, 55). In terms of the GO biological process category, the DMRGs were closely related to inflammatory- and immune-related biological processes. Therefore, we suggest that, in addition to chronic inflammation, the immune response may also contribute to the pathophysiology of GDM.
As stated above, monocyte infiltration is aberrant in the placentas of patients with GDM. We obtained DMRGs related to monocyte infiltration, of which seven DMRGs (CD81, CFH, FABP5, GBP1, GNG11, IL1RL1, and SLAMF6) were selected through LASSO regression analysis to construct a nomogram. FABP5 belongs to the calycin superfamily and fatty-acid binding protein family, and serves as a gatekeeper for mitochondrial integrity to modulate regulatory T cells (Treg) and subdue immune responses (56). It has been reported that increased intra-tumoral FABP5 contributes to CD8+ T-cell infiltration and is linked to overall and recurrence-free survival, indicating that FABP5 could be an immunometabolic marker in hepatocellular carcinoma (57). Moreover, FABP5 has been observed to be enriched in classical monocytes of heart failure patients, suggesting that FABP5 contributes to monocyte activation (58). CD81 is a tetraspanin that participates in adaptive immunity and host-virus interactions (59, 60). As an inhibitor of the alternative complement pathway, CFH protects self-surfaces from immune attacks, thereby engaging in host-virus interactions and innate immunity (61–63). GBP1 is involved in macrophage apoptosis and pyroptosis (64). Interleukin-33 (IL-33) is the only known ligand of IL1RL1, and IL1RL1/IL-33 signaling participates in various inflammatory diseases (65). SLAMF6 is expressed in a variety of immune cells and may be involved in crosstalk between different microenvironments (66–68). Finally, GNG11, a member of the guanine nucleotide-binding protein family, is involved in various transmembrane signaling systems (69, 70). The nomogram showed a robust performance in distinguishing GDM patients from normal controls in the training set (GSE70493), with an AUC of 83%. GDM diagnosis is usually confirmed by a 75 g-oral glucose tolerance test during the second trimester. GSE92772, which is based on blood samples extracted during 24–28 weeks of gestation, was selected to externally validate the diagnostic capacity of the nomogram. The nomogram model exhibited a high diagnostic value with an AUC value of 85.9%, although it lacked the expression matrix of CFH. Therefore, our findings suggest that this m6A-related signature, correlated with monocyte infiltration, can be regarded as a novel biomarker and potential therapeutic target for GDM.
This study had a few limitations. A comprehensive analysis of the placenta and peripheral blood is warranted to verify the mRNA expression, protein expression and m6A-modification status of CD81, CFH, FABP5, GBP1, GNG11, IL1RL1, and SLAMF6. The diagnostic ability of the nomogram model may require further validation using a larger sample size. For subsequent research, more clinical parameters regarding valuable prognosis risk characteristics should be incorporated to verify the predictive ability of the nomogram.
In this study, we analyzed the immune landscape and DMRGs in the placentas of patients with GDM. Some DMRGs were strongly associated with monocyte infiltration, which was higher in GDM placentas than in the control group. Based on seven selected DMRGs linked to monocyte infiltration in GDM placentas, we developed and validated a highly accurate nomogram for recognizing GDM.
The raw data of GSE70493 and GSE92772 were obtained from public databases (https://www.ncbi.nlm.nih.gov/geo/). The processed data are available from the corresponding author upon reasonable request.
RD: Conceptualization, Data curation, Formal analysis, Software, Writing—original draft. YW Conceptualization, Supervision, Validation, Writing—review and editing. LL: Funding acquisition, Methodology. All authors contributed to the article and approved the submitted version.
The study was supported by the Clinical Research Project of Liaoning Diabetes Medical Nutrition Prevention Society [grant number LNSTNBYXYYFZXH-RS01A].
The authors declare that the research was conducted in the absence of any commercial or financial relationships that could be construed as a potential conflict of interest.
All claims expressed in this article are solely those of the authors and do not necessarily represent those of their affiliated organizations, or those of the publisher, the editors and the reviewers. Any product that may be evaluated in this article, or claim that may be made by its manufacturer, is not guaranteed or endorsed by the publisher.
We gratefully acknowledge Yi Zhang for providing technical assistance and intellectual support.
The Supplementary Material for this article can be found online at: https://www.frontiersin.org/articles/10.3389/fendo.2022.853857/full#supplementary-material
Supplementary Figure 1 | Higher proportion of M2 than M1 phenotype of macrophages in GDM compared to controls.
1. Reece EA, Leguizamon G, Wiznitzer A. Gestational Diabetes: The Need for a Common Ground. Lancet (2009) 373:1789–97. doi: 10.1016/S0140-6736(09)60515-8
2. American Diabetes Association. 2. Classification and Diagnosis of Diabetes: Standards of Medical Care in Diabetes-2020. Diabetes Care (2020) 43:S14–31. doi: 10.2337/dc20-S002
3. Nguyen-Ngo C, Jayabalan N, Salomon C, Lappas M. Molecular Pathways Disrupted by Gestational Diabetes Mellitus. J Mol Endocrinol (2019) 63:R51–72. doi: 10.1530/JME-18-0274
4. Khambule L, George JA. The Role of Inflammation in the Development of GDM and the Use of Markers of Inflammation in GDM Screening. Adv Exp Med Biol (2019) 1134:217–42. doi: 10.1007/978-3-030-12668-1_12
5. Zhang J, Chi H, Xiao H, Tian X, Wang Y, Yun X, et al. Interleukin 6 (IL-6) and Tumor Necrosis Factor Alpha (TNF-Alpha) Single Nucleotide Polymorphisms (SNPs), Inflammation and Metabolism in Gestational Diabetes Mellitus in Inner Mongolia. Med Sci Monit (2017) 23:4149–57. doi: 10.12659/msm.903565
6. Li J, Li Y, Zhou X, Wei L, Zhang J, Zhu S, et al. Upregulation of IL-15 in the Placenta Alters Trophoblasts Behavior Contributing to Gestational Diabetes Mellitus. Cell Biosci (2021) 11:33. doi: 10.1186/s13578-021-00533-4
7. Amirian A, Mahani MB, Abdi F. Role of Interleukin-6 (IL-6) in Predicting Gestational Diabetes Mellitus. Obstet Gynecol Sci (2020) 63:407–16. doi: 10.5468/ogs.20020
8. Schulze F, Wehner J, Kratschmar DV, Makshana V, Meier DT, Hauselmann SP, et al. Inhibition of IL-1beta Improves Glycaemia in a Mouse Model for Gestational Diabetes. Sci Rep (2020) 10:3035. doi: 10.1038/s41598-020-59701-0
9. Wei Q, Chen X, Chen H. Association of Single Nucleotide Polymorphisms of the IL-6, IL-10, and TNF-Alpha Genes With Susceptibility to Gestational Diabetes Mellitus. Genet Test Mol Biomarkers (2020) 24:390–8. doi: 10.1089/gtmb.2020.0069
10. Piao C, Wang X, Peng S, Guo X, Zhao H, He L, et al. IL-34 Causes Inflammation and Beta Cell Apoptosis and Dysfunction in Gestational Diabetes Mellitus. Endocr Connect (2019) 8:1503–12. doi: 10.1530/EC-19-0436
11. Yu Z, Liu J, Zhang R, Huang X, Sun T, Wu Y, et al. IL-37 and 38 Signalling in Gestational Diabetes. J Reprod Immunol (2017) 124:8–14. doi: 10.1016/j.jri.2017.09.011
12. Knofler M, Haider S, Saleh L, Pollheimer J, Gamage T, James J. Human Placenta and Trophoblast Development: Key Molecular Mechanisms and Model Systems. Cell Mol Life Sci (2019) 76:3479–96. doi: 10.1007/s00018-019-03104-6
13. Tong M, Abrahams VM. Immunology of the Placenta. Obstet Gynecol Clin North Am (2020) 47:49–63. doi: 10.1016/j.ogc.2019.10.006
14. Schumacher A, Sharkey DJ, Robertson SA, Zenclussen AC. Immune Cells at the Fetomaternal Interface: How the Microenvironment Modulates Immune Cells To Foster Fetal Development. J Immunol (2018) 201:325–34. doi: 10.4049/jimmunol.1800058
15. De Luccia TPB, Pendeloski KPT, Ono E, Mattar R, Pares DBS, Yazaki Sun S, et al. Unveiling the Pathophysiology of Gestational Diabetes: Studies on Local and Peripheral Immune Cells. Scand J Immunol (2020) 91:e12860. doi: 10.1111/sji.12860
16. Boccaletto P, Baginski B. MODOMICS: An Operational Guide to the Use of the RNA Modification Pathways Database. Methods Mol Biol (2021) 2284:481–505. doi: 10.1007/978-1-0716-1307-8_26
17. Zhong H, Tang HF, Kai Y. N6-Methyladenine RNA Modification (M(6)A): An Emerging Regulator of Metabolic Diseases. Curr Drug Targets (2020) 21:1056–67. doi: 10.2174/1389450121666200210125247
18. Zaccara S, Ries RJ, Jaffrey SR. Reading, Writing and Erasing mRNA Methylation. Nat Rev Mol Cell Biol (2019) 20:608–24. doi: 10.1038/s41580-019-0168-5
19. Niu Y, Zhao X, Wu YS, Li MM, Wang XJ, Yang YG. N6-Methyl-Adenosine (M6a) in RNA: An Old Modification With a Novel Epigenetic Function. Genomics Proteomics Bioinformatics (2013) 11:8–17. doi: 10.1016/j.gpb.2012.12.002
20. Berulava T, Buchholz E, Elerdashvili V, Pena T, Islam MR, Lbik D, et al. Changes in M6a RNA Methylation Contribute to Heart Failure Progression by Modulating Translation. Eur J Heart Fail (2020) 22:54–66. doi: 10.1002/ejhf.1672
21. De Jesus DF, Zhang Z, Kahraman S, Brown NK, Chen M, Hu J, et al. M(6)A mRNA Methylation Regulates Human Beta-Cell Biology in Physiological States and in Type 2 Diabetes. Nat Metab (2019) 1:765–74. doi: 10.1038/s42255-019-0089-9
22. Chen J, Du B. Novel Positioning From Obesity to Cancer: FTO, an M(6)A RNA Demethylase, Regulates Tumour Progression. J Cancer Res Clin Oncol (2019) 145:19–29. doi: 10.1007/s00432-018-2796-0
23. Yang Y, Shen F, Huang W, Qin S, Huang JT, Sergi C, et al. Glucose Is Involved in the Dynamic Regulation of M6a in Patients With Type 2 Diabetes. J Clin Endocrinol Metab (2019) 104:665–73. doi: 10.1210/jc.2018-00619
24. Xuan JJ, Sun WJ, Lin PH, Zhou KR, Liu S, Zheng LL, et al. RMBase V2.0: Deciphering the Map of RNA Modifications From Epitranscriptome Sequencing Data. Nucleic Acids Res (2018) 46:D327–34. doi: 10.1093/nar/gkx934
25. Luo X, Li H, Liang J, Zhao Q, Xie Y, Ren J, et al. RMVar: An Updated Database of Functional Variants Involved in RNA Modifications. Nucleic Acids Res (2021) 49:D1405–12. doi: 10.1093/nar/gkaa811
26. Steen CB, Liu CL, Alizadeh AA, Newman AM. Profiling Cell Type Abundance and Expression in Bulk Tissues With CIBERSORTx. Methods Mol Biol (2020) 2117:135–57. doi: 10.1007/978-1-0716-0301-7_7
27. Liu Z, Gu Y, Chakarov S, Bleriot C, Kwok I, Chen X, et al. Fate Mapping via Ms4a3-Expression History Traces Monocyte-Derived Cells. Cell (2019) 178:1509–1525 e1519. doi: 10.1016/j.cell.2019.08.009
28. Angelo AGS, Neves CTC, Lobo TF, Godoy RVC, Ono E, Mattar R, et al. Monocyte Profile in Peripheral Blood of Gestational Diabetes Mellitus Patients. Cytokine (2018) 107:79–84. doi: 10.1016/j.cyto.2017.11.017
29. Ozanska A, Szymczak D, Rybka J. Pattern of Human Monocyte Subpopulations in Health and Disease. Scand J Immunol (2020) 92:e12883. doi: 10.1111/sji.12883
30. Faas MM, Spaans F, De Vos P. Monocytes and Macrophages in Pregnancy and Pre-Eclampsia. Front Immunol (2014) 5:298. doi: 10.3389/fimmu.2014.00298
31. Zhang YH, He M, Wang Y, Liao AH. Modulators of the Balance Between M1 and M2 Macrophages During Pregnancy. Front Immunol (2017) 8:120. doi: 10.3389/fimmu.2017.00120
32. Houser BL, Tilburgs T, Hill J, Nicotra ML, Strominger JL. Two Unique Human Decidual Macrophage Populations. J Immunol (2011) 186:2633–42. doi: 10.4049/jimmunol.1003153
33. Jaiswal MK, Mallers TM, Larsen B, Kwak-Kim J, Chaouat G, Gilman-Sachs A, et al. V-ATPase Upregulation During Early Pregnancy: A Possible Link to Establishment of an Inflammatory Response During Preimplantation Period of Pregnancy. Reproduction (2012) 143:713–25. doi: 10.1530/REP-12-0036
34. Aldo PB, Racicot K, Craviero V, Guller S, Romero R, Mor G. Trophoblast Induces Monocyte Differentiation Into CD14+/CD16+ Macrophages. Am J Reprod Immunol (2014) 72:270–84. doi: 10.1111/aji.12288
35. Bain CC, Hawley CA, Garner H, Scott CL, Schridde A, Steers NJ, et al. Long-Lived Self-Renewing Bone Marrow-Derived Macrophages Displace Embryo-Derived Cells to Inhabit Adult Serous Cavities. Nat Commun (2016) 7:ncomms11852. doi: 10.1038/ncomms11852
36. Guilliams M, Scott CL. Does Niche Competition Determine the Origin of Tissue-Resident Macrophages? Nat Rev Immunol (2017) 17:451–60. doi: 10.1038/nri.2017.42
37. Ding J, Zhang Y, Cai X, Diao L, Yang C, Yang J. Crosstalk Between Trophoblast and Macrophage at the Maternal-Fetal Interface: Current Status and Future Perspectives. Front Immunol (2021) 12:758281. doi: 10.3389/fimmu.2021.758281
38. Yao Y, Xu XH, Jin L. Macrophage Polarization in Physiological and Pathological Pregnancy. Front Immunol (2019) 10:792. doi: 10.3389/fimmu.2019.00792
39. Zhang YH, Aldo P, You Y, Ding J, Kaislasuo J, Petersen JF, et al. Trophoblast-Secreted Soluble-PD-L1 Modulates Macrophage Polarization and Function. J Leukoc Biol (2020) 108:983–98. doi: 10.1002/JLB.1A0420-012RR
40. Sisino G, Bouckenooghe T, Aurientis S, Fontaine P, Storme L, Vambergue A. Diabetes During Pregnancy Influences Hofbauer Cells, a Subtype of Placental Macrophages, to Acquire a Pro-Inflammatory Phenotype. Biochim Biophys Acta (2013) 1832:1959–68. doi: 10.1016/j.bbadis.2013.07.009
41. Yu J, Zhou Y, Gui J, Li AZ, Su XL, Feng L. Assessment of the Number and Function of Macrophages in the Placenta of Gestational Diabetes Mellitus Patients. J Huazhong Univ Sci Technolog Med Sci (2013) 33:725–9. doi: 10.1007/s11596-013-1187-7
42. Schliefsteiner C, Peinhaupt M, Kopp S, Logl J, Lang-Olip I, Hiden U, et al. Human Placental Hofbauer Cells Maintain an Anti-Inflammatory M2 Phenotype Despite the Presence of Gestational Diabetes Mellitus. Front Immunol (2017) 8:888. doi: 10.3389/fimmu.2017.00888
43. Bari MF, Weickert MO, Sivakumar K, James SG, Snead DR, Tan BK, et al. Elevated Soluble CD163 in Gestational Diabetes Mellitus: Secretion From Human Placenta and Adipose Tissue. PloS One (2014) 9:e101327. doi: 10.1371/journal.pone.0101327
44. Barke TL, Goldstein JA, Sundermann AC, Reddy AP, Linder JE, Correa H, et al. Gestational Diabetes Mellitus Is Associated With Increased CD163 Expression and Iron Storage in the Placenta. Am J Reprod Immunol (2018) 80:e13020. doi: 10.1111/aji.13020
45. Wu R, Liu Y, Yao Y, Zhao Y, Bi Z, Jiang Q, et al. FTO Regulates Adipogenesis by Controlling Cell Cycle Progression via M(6)A-YTHDF2 Dependent Mechanism. Biochim Biophys Acta Mol Cell Biol Lipids (2018) 1863:1323–30. doi: 10.1016/j.bbalip.2018.08.008
46. Peng S, Xiao W, Ju D, Sun B, Hou N, Liu Q, et al. Identification of Entacapone as a Chemical Inhibitor of FTO Mediating Metabolic Regulation Through FOXO1. Sci Transl Med (2019) 11:eaau7116. doi: 10.1126/scitranslmed.aau7116
47. Yao Y, Bi Z, Wu R, Zhao Y, Liu Y, Liu Q, et al. METTL3 Inhibits BMSC Adipogenic Differentiation by Targeting the JAK1/STAT5/C/EBPbeta Pathway via an M(6)A-YTHDF2-Dependent Manner. FASEB J (2019) 33:7529–44. doi: 10.1096/fj.201802644R
48. Li K, Zhang J, Yu J, Liu B, Guo Y, Deng J, et al. MicroRNA-214 Suppresses Gluconeogenesis by Targeting Activating Transcriptional Factor 4. J Biol Chem (2015) 290:8185–95. doi: 10.1074/jbc.M114.633990
49. Zong X, Zhao J, Wang H, Lu Z, Wang F, Du H, et al. Mettl3 Deficiency Sustains Long-Chain Fatty Acid Absorption Through Suppressing Traf6-Dependent Inflammation Response. J Immunol (2019) 202:567–78. doi: 10.4049/jimmunol.1801151
50. Li X, Yang J, Zhu Y, Liu Y, Shi X, Yang G. Mouse Maternal High-Fat Intake Dynamically Programmed mRNA M(6)A Modifications in Adipose and Skeletal Muscle Tissues in Offspring. Int J Mol Sci (2016) 17:1336. doi: 10.3390/ijms17081336
51. Tung YC, Ayuso E, Shan X, Bosch F, O'Rahilly S, Coll AP, et al. Hypothalamic-Specific Manipulation of Fto, the Ortholog of the Human Obesity Gene FTO, Affects Food Intake in Rats. PloS One (2010) 5:e8771. doi: 10.1371/journal.pone.0008771
52. Unnikrishnan R, Shanthi Rani CS, Anjana RM, Uthra SC, Vidya J, Sankari GU, et al. Postpartum Development of Type 1 Diabetes in Asian Indian Women With Gestational Diabetes. Indian J Endocrinol Metab (2016) 20:702–6. doi: 10.4103/2230-8210.190562
53. Dereke J, Palmqvist S, Nilsson C, Landin-Olsson M, Hillman M. The Prevalence and Predictive Value of the SLC30A8 R325W Polymorphism and Zinc Transporter 8 Autoantibodies in the Development of GDM and Postpartum Type 1 Diabetes. Endocrine (2016) 53:740–6. doi: 10.1007/s12020-016-0932-7
54. Knabl J, de Maiziere L, Huttenbrenner R, Hutter S, Juckstock J, Mahner S, et al. Cell Type- and Sex-Specific Dysregulation of Thyroid Hormone Receptors in Placentas in Gestational Diabetes Mellitus. Int J Mol Sci (2020) 21:4056. doi: 10.3390/ijms21114056
55. Sert UY, Buyuk GN, Engin Ustun Y, Ozgu Erdinc AS. Is There Any Relationship Between Thyroid Function Abnormalities, Thyroid Antibodies and Development of Gestational Diabetes Mellitus (GDM) in Pregnant Women? Medeni Med J (2020) 35:195–201. doi: 10.5222/MMJ.2020.29964
56. Field CS, Baixauli F, Kyle RL, Puleston DJ, Cameron AM, Sanin DE, et al. Mitochondrial Integrity Regulated by Lipid Metabolism Is a Cell-Intrinsic Checkpoint for Treg Suppressive Function. Cell Metab (2020) 31:422–437 e425. doi: 10.1016/j.cmet.2019.11.021
57. Liu F, Liu W, Zhou S, Yang C, Tian M, Jia G, et al. Identification of FABP5 as an Immunometabolic Marker in Human Hepatocellular Carcinoma. J Immunother Cancer (2020) 8:e000501. doi: 10.1136/jitc-2019-000501
58. Abplanalp WT, John D, Cremer S, Assmus B, Dorsheimer L, Hoffmann J, et al. Single-Cell RNA-Sequencing Reveals Profound Changes in Circulating Immune Cells in Patients With Heart Failure. Cardiovasc Res (2021) 117:484–94. doi: 10.1093/cvr/cvaa101
59. Feneant L, Levy S, Cocquerel L. CD81 and Hepatitis C Virus (HCV) Infection. Viruses (2014) 6:535–72. doi: 10.3390/v6020535
60. Levy S. Function of the Tetraspanin Molecule CD81 in B and T Cells. Immunol Res (2014) 58:179–85. doi: 10.1007/s12026-014-8490-7
61. Kajander T, Lehtinen MJ, Hyvarinen S, Bhattacharjee A, Leung E, Isenman DE, et al. Dual Interaction of Factor H With C3d and Glycosaminoglycans in Host-Nonhost Discrimination by Complement. Proc Natl Acad Sci USA (2011) 108:2897–902. doi: 10.1073/pnas.1017087108
62. Blaum BS, Hannan JP, Herbert AP, Kavanagh D, Uhrin D, Stehle T. Structural Basis for Sialic Acid-Mediated Self-Recognition by Complement Factor H. Nat Chem Biol (2015) 11:77–82. doi: 10.1038/nchembio.1696
63. Losse J, Zipfel PF, Jozsi M. Factor H and Factor H-Related Protein 1 Bind to Human Neutrophils via Complement Receptor 3, Mediate Attachment to Candida Albicans, and Enhance Neutrophil Antimicrobial Activity. J Immunol (2010) 184:912–21. doi: 10.4049/jimmunol.0901702
64. Fisch D, Bando H, Clough B, Hornung V, Yamamoto M, Shenoy AR, et al. Human GBP1 Is a Microbe-Specific Gatekeeper of Macrophage Apoptosis and Pyroptosis. EMBO J (2019) 38:e100926. doi: 10.15252/embj.2018100926
65. Griesenauer B, Paczesny S. The ST2/IL-33 Axis in Immune Cells During Inflammatory Diseases. Front Immunol (2017) 8:475. doi: 10.3389/fimmu.2017.00475
66. Yigit B, Wang N, Ten Hacken E, Chen SS, Bhan AK, Suarez-Fueyo A, et al. SLAMF6 as a Regulator of Exhausted CD8(+) T Cells in Cancer. Cancer Immunol Res (2019) 7:1485–96. doi: 10.1158/2326-6066.CIR-18-0664
67. Yigit B, Wang N, Herzog RW, Terhorst C. SLAMF6 in Health and Disease: Implications for Therapeutic Targeting. Clin Immunol (2019) 204:3–13. doi: 10.1016/j.clim.2018.10.013
68. Wang N, Keszei M, Halibozek P, Yigit B, Engel P, Terhorst C. Slamf6 Negatively Regulates Autoimmunity. Clin Immunol (2016) 173:19–26. doi: 10.1016/j.clim.2016.06.009
69. Hossain MN, Sakemura R, Fujii M, Ayusawa D. G-Protein Gamma Subunit GNG11 Strongly Regulates Cellular Senescence. Biochem Biophys Res Commun (2006) 351:645–50. doi: 10.1016/j.bbrc.2006.10.112
Keywords: gestational diabetes mellitus, N6-methyladenosine modification, immune infiltration, monocyte, nomogram
Citation: Du R, Li L and Wang Y (2022) N6-Methyladenosine-Related Gene Signature Associated With Monocyte Infiltration Is Clinically Significant in Gestational Diabetes Mellitus. Front. Endocrinol. 13:853857. doi: 10.3389/fendo.2022.853857
Received: 13 January 2022; Accepted: 18 February 2022;
Published: 18 March 2022.
Edited by:
Undurti Narasimha Das, UND Life Sciences LLC, United StatesReviewed by:
Yingmei Feng, Capital Medical University, ChinaCopyright © 2022 Du, Li and Wang. This is an open-access article distributed under the terms of the Creative Commons Attribution License (CC BY). The use, distribution or reproduction in other forums is permitted, provided the original author(s) and the copyright owner(s) are credited and that the original publication in this journal is cited, in accordance with accepted academic practice. No use, distribution or reproduction is permitted which does not comply with these terms.
*Correspondence: Yanjun Wang, d2FuZ3lqc3lAMTYzLmNvbQ==
Disclaimer: All claims expressed in this article are solely those of the authors and do not necessarily represent those of their affiliated organizations, or those of the publisher, the editors and the reviewers. Any product that may be evaluated in this article or claim that may be made by its manufacturer is not guaranteed or endorsed by the publisher.
Research integrity at Frontiers
Learn more about the work of our research integrity team to safeguard the quality of each article we publish.