- 1Department of Obstetrics and Gynaecology, The First Affiliated Hospital of Chongqing Medical University, Chongqing, China
- 2Chongqing Key Laboratory of Maternal and Fetal Medicine, Chongqing Medical University, Chongqing, China
- 3Department of Statistics, The University of Auckland, Auckland, New Zealand
- 4Department of Health Sciences, University of Leicester, Leicester, United Kingdom
- 5College of Health, Massey University, Auckland, New Zealand
- 6School of Public Health and Management, Chongqing Medical University, Chongqing, China
- 7Chongqing Key Laboratory of Oral Diseases and Biomedical Sciences, Stomatological Hospital of Chongqing Medical University, Chongqing, China
- 8Department of Obstetrics and Gynecology, Women and Children’s Hospital of Chongqing Medical University, Chongqing, China
- 9Centre for Environmental Health and Sustainability & School of Geography, Geology and the Environment, University of Leicester, Leicester, United Kingdom
- 10Department of Oral Sciences, Sir John Walsh Research Institute, Faculty of Dentistry, University of Otago, Dunedin, New Zealand
- 11Molecular Immunity, Murdoch Children’s Research Institute, Royal Children’s Hospital, Melbourne, VIC, Australia
- 12Liggins Institute, The University of Auckland, Auckland, New Zealand
- 13Department of Obstetrics and Gynaecology, The Second Affiliated Hospital of Chongqing Medical University, Chongqing, China
- 14College of Life Sciences, University of Leicester, Leicester, United Kingdom
Background: Gestational diabetes mellitus (GDM) is a metabolic condition defined as glucose intolerance with first presentation during pregnancy. Many studies suggest that environmental exposures, including air pollution, contribute to the pathogenesis of GDM. Although hair metabolite profiles have been shown to reflect pollution exposure, few studies have examined the link between environmental exposures, the maternal hair metabolome and GDM. The aim of this study was to investigate the longitudinal relationship (from pre-conception through to the third trimester) between air pollution exposure, the hair metabolome and GDM in a Chinese cohort.
Methods: A total of 1020 women enrolled in the Complex Lipids in Mothers and Babies (CLIMB) birth cohort were included in our study. Metabolites from maternal hair segments collected pre-conception, and in the first, second, and third trimesters were analysed using gas chromatography-mass spectrometry (GC-MS). Maternal exposure to air pollution was estimated by two methods, namely proximal and land use regression (LUR) models, using air quality data from the air quality monitoring station nearest to the participant’s home. Logistic regression and mixed models were applied to investigate associations between the air pollution exposure data and the GDM associated metabolites.
Results: Of the 276 hair metabolites identified, the concentrations of fourteen were significantly different between GDM cases and non-GDM controls, including some amino acids and their derivatives, fatty acids, organic acids, and exogenous compounds. Three of the metabolites found in significantly lower concentrations in the hair of women with GDM (2-hydroxybutyric acid, citramalic acid, and myristic acid) were also negatively associated with daily average concentrations of PM2.5, PM10, SO2, NO2, CO and the exposure estimates of PM2.5 and NO2, and positively associated with O3.
Conclusions: This study demonstrated that the maternal hair metabolome reflects the longitudinal metabolic changes that occur in response to environmental exposures and the development of GDM.
Introduction
Industrialization accelerated the consumption of coal combustion and fossil fuels for transportation and other human activities. Environmental pollution, in particular air pollution, has become a major public health issue and a leading cause of disease especially in developing countries (1, 2). In China, air pollution was estimated to cause approximately 1.24 million deaths and a reduction in the mean life expectancy by about 1.25 years in 2017 (3). Air pollution is also associated with numerous inflammatory diseases that frequently arise with comorbidities, such as chronic obstructive pulmonary disease, cancer, metabolic diseases, and diabetes (4–8).
Pregnancy and early infancy are considered to be highly sensitive periods where exposures could lead to lifelong consequences (9). Pregnant women are exposed to hundreds of chemicals at low levels and these exposures could operate additively or interactively, raising the possibility of ‘mixture’ effects (10). Exposure to air pollution in pregnancy has been linked to a range of adverse outcomes, including miscarriage, pre-eclampsia, preterm birth, birth to an infant small for gestational age, and gestational diabetes mellitus (GDM) (11–17). Pregnancy involves a dynamic flow of metabolic changes week by week, with substantial physiological alterations in glucose and lipid metabolism (18). Pregnant women are particularly prone to abnormal glycaemia as elevated insulin resistance is part of the normal physiological adaptation to pregnancy, to ensure greater substrate availability for fetal growth (19). However, up to 20% of all pregnancies develop some degree of impaired glucose tolerance in China (20). GDM is associated with serious short-term and long-term adverse health consequences for both the mother and baby (21–23). Although the precise pathogenesis of GDM remains unclear, GDM shares similar pathophysiological features with type 2 diabetes mellitus (24). Several studies have reported that air pollution is an important risk factor for type 2 diabetes mellitus, through inflammation-related insulin resistance, endothelial dysfunction, and dysregulation of adipose tissue (8, 25–27). Genome-wide association studies indicate that genetic factors contribute to only a small proportion of GDM risk, and the increased prevalence of GDM has occurred with minor to no shift in the genetic composition of the population (28, 29). Specific air pollution exposures potentially associated with increasing GDM risk include exposure to particulate matter less than 10 μm in diameter (PM10), NO2, and SO2, among others (30–34). The timing of air pollution exposure in pregnancy may impact GDM risk but data are scarce and often contradictory (35). Therefore, it is of great significance to study the biological effects of environmental exposures during pregnancy and early infancy.
The burden of the risk attributable to environmental factors is unclear, and this is in part due to the limited capacity to accurately measure the complex mix of pollutants and other environmental exposures across an extended timeframe. Metabolomics is a powerful approach for directly identifying and quantifying low molecular weight compounds. Metabolomics aims to view the complex nature of how physiology is related to external exposure, and how these associations may be linked to disease outcomes (36–38). Although epidemiological studies have shown associations between air pollution and increased GDM risk, few studies have linked these associations to longitudinal metabolic changes in vivo. Conventional samples used in metabolomics studies, such as urine and blood, can be influenced by acute and transient factors such as recent dietary intake and immune status (39, 40). The dynamic nature of conventional biological samples can limit the discovery of robust biomarkers, making them less suitable for the study of long-term effects of environmental exposures on pregnancy outcomes. Conversely, hair is a highly stable structure that assimilates endogenous compounds and environmental compounds during growth. These accumulate in an ordered temporal manner as hair grows. Hair sampling is also non-invasive compared to the collection of other biological samples such as blood. As a result, a hair sample offers several potential advantages over other biospecimens, such as providing a metabolite profile that reflects environmental exposures over several months (41). In addition, our previous studies have found that the maternal hair metabolome can reflect differences between healthy pregnancies and complicated pregnancies, including fetal growth restriction (FGR) and GDM (42–44). Previously conducted hair metabolomic studies have used a case-control design with a relatively small sample size. To date, no study has investigated the maternal hair metabolome in a large cohort in an attempt to understand how external exposures may alter the metabolic profile in association with GDM.
The aim of this research was to comprehensively assess the relationship between air pollution, the maternal hair metabolome and GDM status from the pre-conception period through the three trimesters of pregnancy.
Methods
Study participants
Women were recruited from the prospective, longitudinal CLIMB (Complex Lipids In Mothers and Babies) cohort, which has been described previously (45). Recruitment of the CLIMB cohort began in September 2015 and ended in November 2016. Among 17,382 eligible participants, 1500 pregnant women (response rate 8.6%) enrolled between 11-14 gestational weeks at the First Affiliated Hospital of Chongqing Medical University (FCQMU) and Chongqing Health Centre for Women and Children (CHC) (46). Women were included in the study if they were between 20–40 years of age and had a singleton pregnancy. Women were excluded from the study if they had a previous pregnancy with complications which resulted in delivery before 32 weeks. We also excluded women lost to follow up (n=49), women with diabetes (n=5), women with a history of GDM (n=1), those with insufficient hair samples (weight < 1.5 mg, n=207; dyed hair, n=3) and those who did not provide a hair sample (n=215) (Figure 1). A 75-g oral-glucose-tolerance test (OGTT) was conducted between 24 and 28 weeks of gestation to identify women with and without GDM. Diagnosis of GDM occurred according to the International Association of Diabetic Pregnancy Study Group (IADPSG) guidelines (fasting plasma glucose ≥ 5.1 mmol/L, one-hour post 75g OGTT plasma glucose level ≥ 10 mmol/L, or two-hour post 75g OGTT plasma glucose level ≥ 8.5 mmol/L) (47). This study was conducted in accordance with the principles in the Declaration of Helsinki. Ethical approval was granted by the Ethics committee of the Chongqing Medical University (No. 2014034), and written informed consent was obtained from all participants.
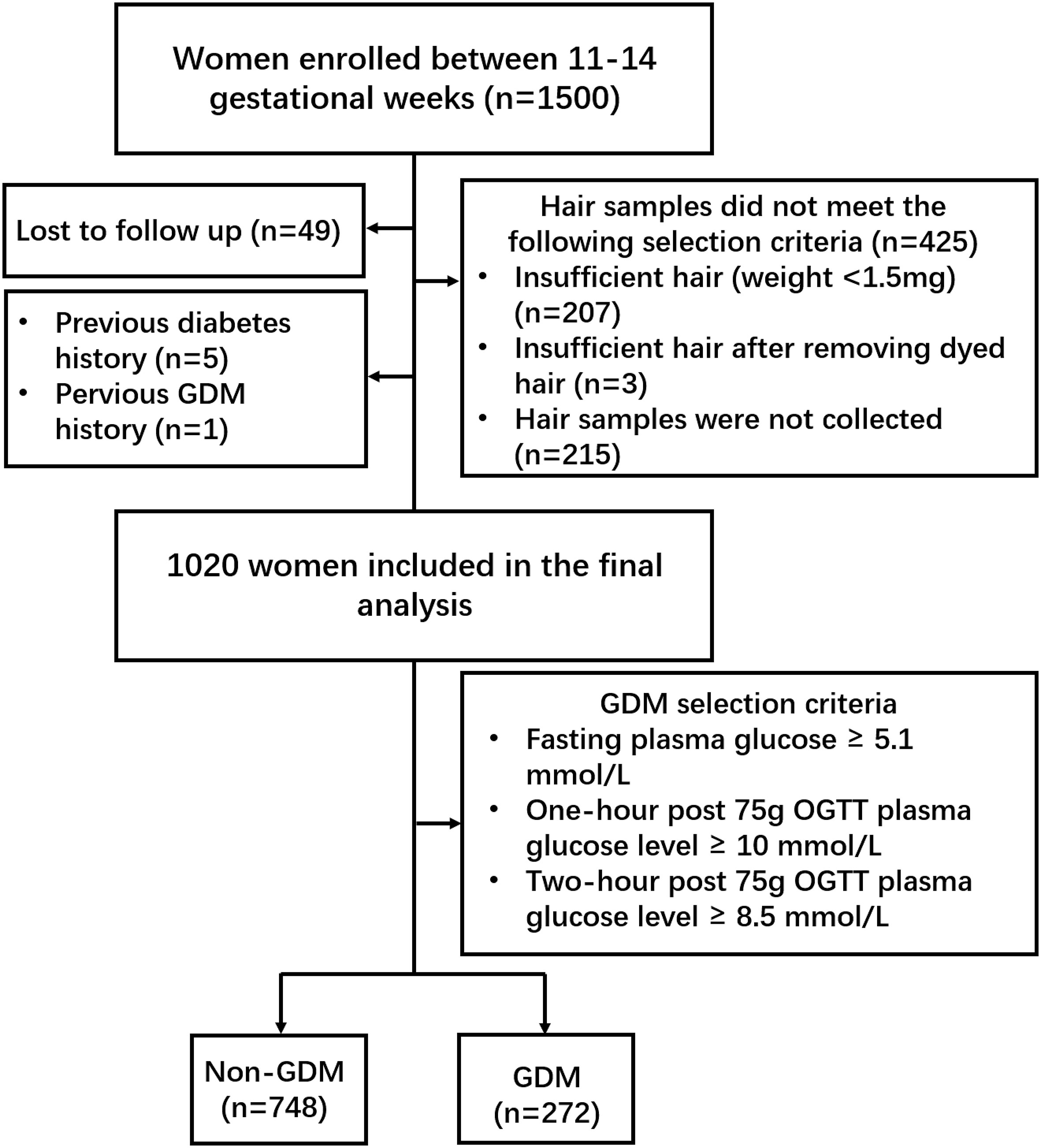
Figure 1 Flowchart of recruited participants. In total, 1500 pregnant women were enrolled between 11-14 gestational weeks. Forty-nine women were lost to follow up, five women with diabetes, one woman with a history of GDM, three women dyed hair, 207 women provided insufficient hair samples and 215 women did not provide a hair sample. A 75-g OGTT was conducted to diagnose GDM according to the IADPSG guidelines. Two hundred and seventy-two GDM women and 748 non-GDM women were included in the final analysis.
Hair sample collection and preparation
Maternal hair strands (n=1020) were collected at 32-34 weeks of gestation. Hairs were cut 0.5 cm away from the scalp around the occipital area at the rear of the head and stored in aluminum foil at -20 °C. The hair segments were cut according to the method published by Delplancke et al. (48). The samples for the first segment from the scalp-end of the hair (0–3 cm) were enriched for growth that occurred in the early third trimester. The next 0.5 cm segment (3–3.5 cm) was discarded to avoid the overlap between the segments for the third and second trimester. The samples for the second segment (3.5-6.5 cm, second trimester), third segment (7-10 cm, first trimester), and fourth segment (10.5-13.5 cm, pre-conception) were collected; (0.5 cm between each segment was discarded). All four hair segments were used for our final analysis.
All hair segments were washed and metabolites extracted using the methods published by Sulek et al. (42). Dried hair extracts were stored at −80°C prior to chemical derivatization. Firstly, hair segments were washed with Milli-Q (MQ) water and methanol twice. The hair segments were put inside glass vials and weighed (range was between 1.5 to 5.5 mg). Alkaline hydrolysis was performed by adding 400 µl of potassium hydroxide (1M) and 20 µL of the internal standard mix (2,3,3,3-d4-alanine (10 mM); 2,3,4,5,6-d5-phenylalanine (10 mM); 3,3-d2-tyrosine (2 mM)) and incubated at 54°C for 18 h. Then, the hair extracts were neutralized to pH 7 by the addition of 67 µl of sulphuric acid (3M). To remove salts and proteins, 1 ml of methanol was added and the sample centrifuged at 4000 rpm for 5 min. Portions (350 µL) of the supernatant were then transferred to three microfuge tubes. The quality control (QC) samples were prepared by pooling the remaining supernatant (approx. 100 µL) from each sample into one 15 ml centrifuge tube, mixing, and then dividing into 350 µL aliquots. All the extracts and QCs were evaporated to dryness using a speedvac (CentriVap Concentrator 230V 50Hz, Cold Trap −105°C Models, Labconco, USA) for 8 h and stored at −80°C prior to derivatization.
Methyl chloroformate derivatization
All prepared samples were chemically derivatized by methyl chloroformate prior to GC-MS analysis, as described previously (49, 50). The dried hair extracts were resuspended in 200 μl of sodium hydroxide (1M). Then samples were transferred to a salinized glass tube and both 167 µl methanol and 34 µl pyridine were added. Derivatization was initiated by adding 20 µL MCF followed by mixing for 30 seconds and this step was repeated twice. MCF derivatives were partitioned by adding 400 µL chloroform and mixing for 10 seconds. Subsequently, 400 µL of sodium bicarbonate (50 mM) was added and mixed for an additional 10 seconds. The samples were then centrifuged at 1500 rpm for 5 minutes before the upper aqueous layer was discarded. Finally, the remaining water was eliminated by adding anhydrous sodium sulphate and the derivatized samples were transferred to GC vials for GC-MS analysis.
Gas chromatography-mass spectrometry analysis
The hair MCF derivatives were analyzed using an Agilent GC7890B system linked to a MSD5977A mass selective detector (EI) set at 70 eV. The GC column used for metabolite analysis was a ZB-1701 GC capillary column (30 m x 250 μm id x 0.15 μm with 5 m guard column, Phenomenex). The GC analysis parameters were as previously described (49, 51). All samples were introduced via pulsed splitless injection with the inlet temperature at 290°C. A constant helium gas flow rate of 1 mL/min was used. The GC-oven was first held at 45°C for 2 min, and then the temperature was elevated with a gradient of 9°C/min to 180°C and was held for 5 min. The temperature was then raised at 40°C/min to 220°C and was held for 5 min. Then the temperature was elevated at 40°C/min to 240°C and was held for 11.5 min. Finally, the temperature was raised at 40°C/min to 280°C and was held for 7 min. The mass spectrometer was run under scan mode with a speed of 3.12 scans/sec with a mass range between 38-550 amu. The solvent delay ended after 5 min. The auxiliary temperature was set to 250°C, the ion source temperature was set to 230°C, and the quadrupole temperature was 150°C (50).
Data extraction and normalization
Raw GC-MS chromatograms were deconvoluted using Automated Mass Spectral Deconvolution and Identification System (AMDIS) software and metabolites were identified using our in-house MCF mass spectral library of 461 compounds and National Institute of Standards and Technology (NIST14) mass spectral library. The criteria for metabolite identification included a match to the MS spectrum of the library compound of >75% and within a 1 min time window of its respective chromatographic retention time. The relative concentrations of the identified metabolites were calculated using MassOmics R-based software (52) that selected the peak height of the most abundant ion within an expected retention time bin. After manual removal of contaminants by comparison with the blank samples, GC-MS data were first normalised by the multiple internal standards (2,3,3,3-d4-alanine, 2,3,4,5,6-d5-phenylalanine, 3,3-d2-tyrosine) and initial batch correction was performed using median centring according to metabolite levels in the QC samples. Lastly, the data were normalised by hair biomass.
Data on air pollution exposure
The concentrations of particulate matter less than 2.5μm in diameter (PM2.5), less than 10μm in diameter (PM10), sulfur dioxide (SO2), nitrogen dioxide (NO2), ozone (O3), and carbon monoxide (CO) are indicators of air quality. Air pollution measurements were obtained from the reports published by the Chongqing Ecological Protection Bureau (53) between 1 January 2015 and 31 December 2017. Data were collected from twenty-one air quality monitoring stations in the main urban area of Chongqing (Figure 2). Monitoring sites were given a site ID number and classified as traffic sites if they were <100m from a major road (motorway, trunk road, primary road), and background sites if they were >100m away from a major road (Supplementary Table 1). In this study, we have used two methods, namely proximal and land use models, to estimate the amount of maternal exposure during pregnancy. With regards to the proximal model, each pregnant woman was linked to the nearest air quality monitoring station data, according to her residential address. Exposures were calculated as daily concentrations averaged over each of the trimesters. Furthermore, Harper et al. (54) developed spatiotemporal land use regression (LUR) models for PM2.5 and NO2 in Chongqing, China, and used the models to estimate PM2.5 and NO2 exposure for the participants in the CLIMB study. The accuracy of LUR model in estimating PM2.5 and NO2 exposures was assessed by the comparing measured and predicted pollutant levels using leave-one-out-cross validation (LOOCV), as displayed in Supplementary Table 2. Therefore, in additional to measured concentrations from the nearest monitoring site, we used the LUR model to estimate the PM2.5 and NO2 exposure of our study population.
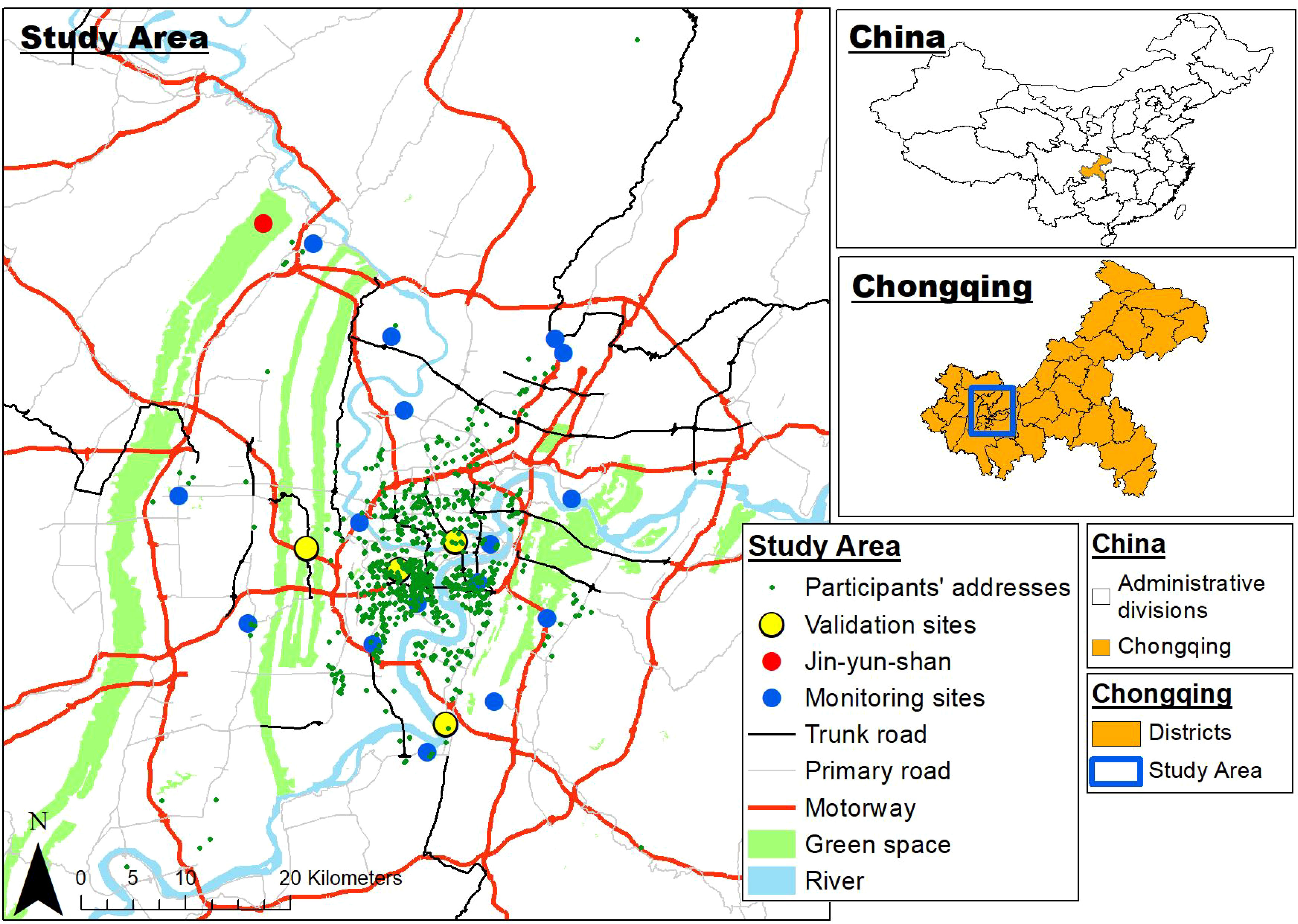
Figure 2 Map of Chongqing showing the location of the monitoring sites and participants’ residential locations.
Statistical analysis
The distribution of maternal clinical characteristics and air exposure data were evaluated using the quantile-quantile (Q-Q) plot and Shapiro-Wilk Normality Test. Student’s T-tests were conducted for normally distributed data, while Mann-Whitney tests were used for non-normally distributed data. All metabolite intensities below the detectable threshold were replaced with 0.5 times the smallest non-zero value for the relevant metabolite and subsequently log transformed to produce a Gaussian distribution. The transformed metabolite profiles were used to perform Uniform Manifold Approximation and Projection (UMAP) dimensionality reduction and different clusters were annotated using the R package UMAP v0.2.8.0 (55). Logistic regression models were created for the four periods, including the confounding factors BMI and age. Metabolites were considered significant if they had a p-value and q-value less than 0.05. A linear mixed model was applied to assess the relationship between GDM and the hair metabolite levels across the four timepoints, using the lme4 R package (56). Hair metabolites were entered into the linear mixed model as the response variable; GDM and hair segment were entered as fixed effects; and experimental batch and individual were entered as random effects. Unadjusted models and models adjusted for major confounding variables as additional fixed effects were established. Confounding variables considered when assessing the relationship between GDM and hair metabolite levels were maternal age and maternal BMI relevant to the timepoint of each hair segment. Metabolites concentrations that were significantly different between GDM and non-GDM groups were based on the likelihood ratio test comparing a null model without GDM as a predictor to one with both a main effect of GDM, and an interaction between GDM and hair segment (indicating differential changes over time). False discovery rates (q-values) were used to account for multiple comparisons, using the R package “qvalue” (57). The parameter π0 was set to the conservative value of 1 rather than estimated. Metabolites were considered significant if they had a q-value less than 0.05. Significant results were then assessed for robustness by trimming the top 0.5% and bottom 0.5% of measurements. The Odds ratios (ORs) were estimated by logistic regression models to analyzed the association between air pollution exposures (increase per interquartile range (IQR)) and GDM risk from pre-conception to third trimester periods either with or without adjustment for maternal age and BMI. To investigate associations between the air pollution exposure data and the metabolites found to be significantly associated with GDM, mixed models were fitted with the GDM associated metabolite as the response, and the pollutant as the predictor, controlling for the effect of segment, batch, and individual effects. The set of p-values produced across pollutants and GDM associated metabolites underwent the q-value procedure, and associations were considered statistically significant if they had a q-value less than 0.05.
Results
Participant characteristics
The prevalence of GDM in our cohort (27.1%) was slightly higher than in the general population of China (ranging from 17.6% to 24.24%) (58, 59). In total, 1020 CLIMB participants were included in the study; 272 women were diagnosed with GDM according to the IADPSG guidelines and 748 women were included in the non-GDM group. Clinical characteristics for all participants are listed in Table 1. As anticipated, the fasting, one-hour, and two-hour blood glucose levels following the 75g OGTT were statistically different between GDM and control groups (p < 0.001). Compared to women in the control group, participants with GDM had a significantly higher age and BMI (in pre-conception and into the third trimester, p < 0.001). Moreover, women in the GDM group tended to deliver earlier than women in the control group (p = 0.027). Education level, blood pressure, placental weight, and birth weight exhibited no significant differences between the GDM and control groups.
Hair metabolites and GDM status
A total of 276 metabolites were identified in the hair of the participants. Information on all identified metabolites is listed in Supplementary Tables 3–7. All identified metabolites were integrated into a UMAP. Participant clusters were generated using low resolution nearest-neighbor clustering and these were divided into eight clusters according to GDM and non-GDM groups across four time points. The UMAP scatter plot of dimensional reduction colored by participants’ identity is shown in Figure 3 (A). Clusters were positioned approximately from pre-conception to the third trimester in a diagonal south-eastward direction. GDM groups were clustered in the middle while non-GDM groups were scattered around. The common significant metabolites in the logistic regression and linear mixed model were shortlisted to form a final list of metabolites significantly associated with GDM. After adjustment for multiple comparisons, fourteen metabolites were found to be significantly different (q < 0.05) between GDM cases and non-GDM controls at one timepoint or more from pre-conception to the third trimester of pregnancy and robust in adjusted for confounders (maternal age and BMI) (Table 2). In Figure 3B, the heatmap demonstrates the ratios of the fourteen significant metabolites between cases and controls from pre-conception through each of the trimesters. In pre-conception, only five hair metabolite levels were significantly reduced in the GDM group compared to the non-GDM group. Whereas, ten hair metabolites were significantly different between the two groups across all trimesters, including some amino acids and derivatives, fatty acids, organic acids, food additives, and exogenous compounds. 2-hydroxybutyric acid, methionine and myristic acid showed the most substantial ratio changes. Furthermore, nine of these fourteen metabolites demonstrated a significant interaction with the hair segment tested (timepoint 0, 1, 2, or 3) – indicating that there were longitudinal differences in the concentrations of these metabolites and their relationship with GDM (Figure 4). Of these metabolites, six showed the largest differences from pre-conception, with differences reducing in later trimesters. As displayed in Figures 4A, B, two of the fourteen metabolites were consistently higher in GDM participants compared to controls across all four timepoints; ten were consistently lower in GDM participants across the timepoints (i.e. 2-hydroxybutyric acid and myristic acid); and two metabolites had relationships with GDM which changed direction between timepoints (i.e. 2-ketoglutamarate, and methyl 4-oxo-2-pentenoate were lower in GDM participants in the pre-conception hair segments but were higher in GDM participants in the third trimester hair segments). Interestingly, both 2-hydroxybutyric acid and myristic acid displayed reduced levels in GDM subclusters, as highlighted in the UMAP (Figures 4C, D).
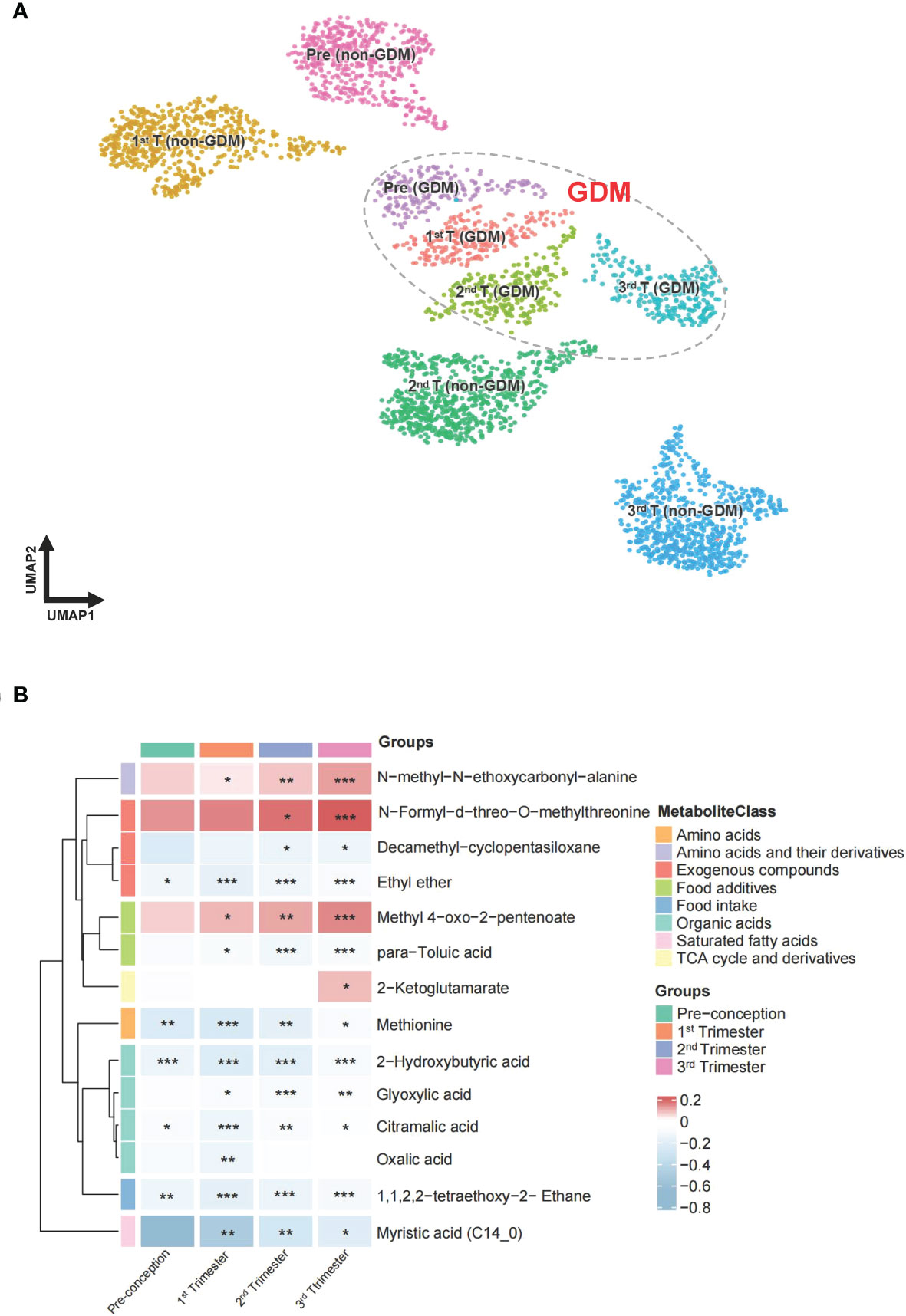
Figure 3 UMAP projection and heatmap of the metabolites across pregnancy. (A) UMAP clustering of all participants labelled and colored by GDM status and different periods of gestation. Groupings include pre-conception GDM [pre (GDM)], first trimester GDM [1st T (GDM)], second trimester GDM [2nd T (GDM)], third trimester GDM [3rd T (GDM)], pre-conception non-GDM [pre (non-GDM)], first trimester non-GDM [1st T (non-GDM)], second trimester non-GDM [2nd T (non-GDM)], and third trimester non-GDM (3rd T (non-GDM)). (B) The ratio of fourteen metabolite levels significantly different between GDM case and control groups. Red color indicates higher metabolite levels in the GDM group than the control group, while blue color indicates lower metabolite levels in the GDM group than the control group. Metabolites with both p-value and q-value less than 0.05 in the logistic regression adjusted for age and BMI are marked with *p-value and q-value less than 0.01 are marked with **p-value and q-value less than 0.001 are marked with ***.
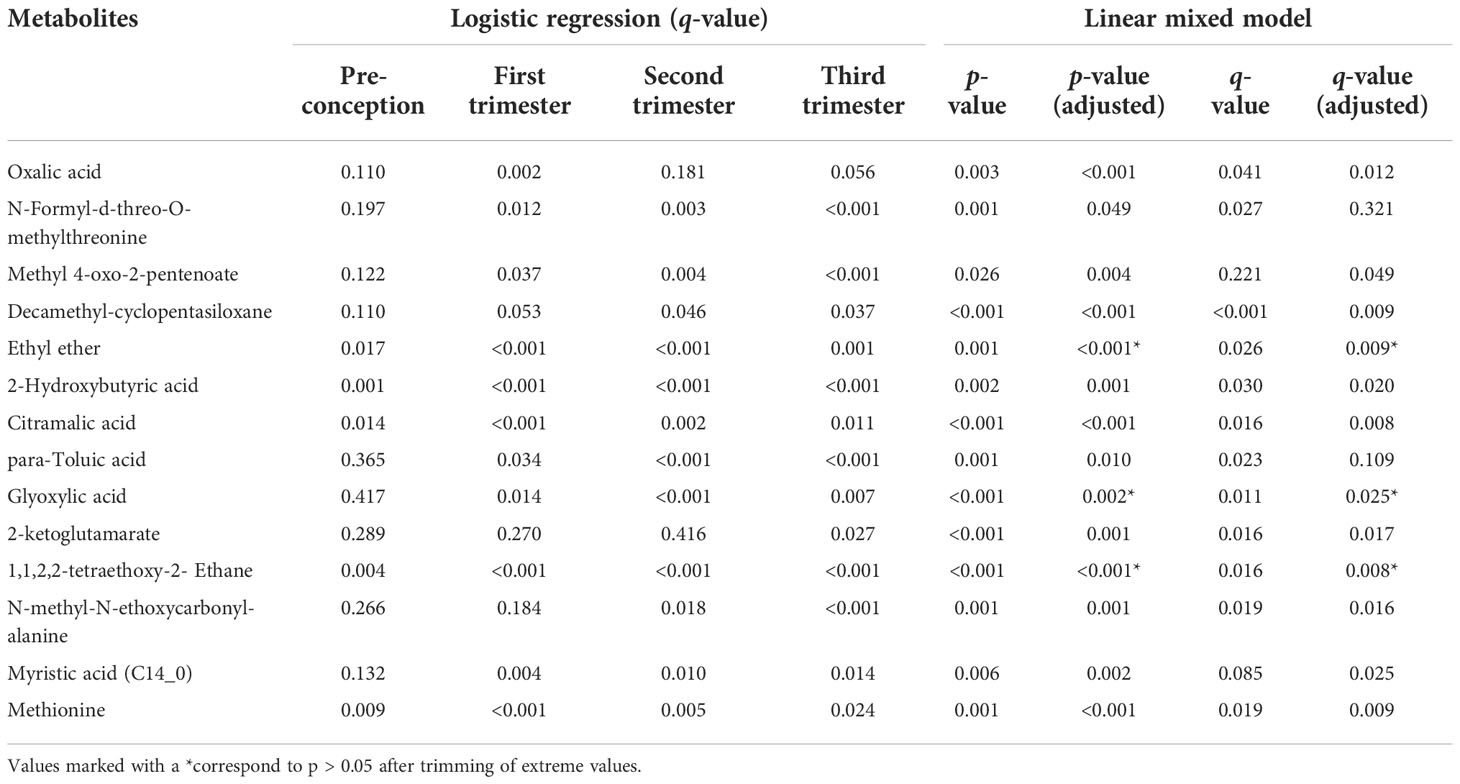
Table 2 Metabolites with significant and robust differences between GDM cases and non-GDM controls in logistic regression and linear mixed models.
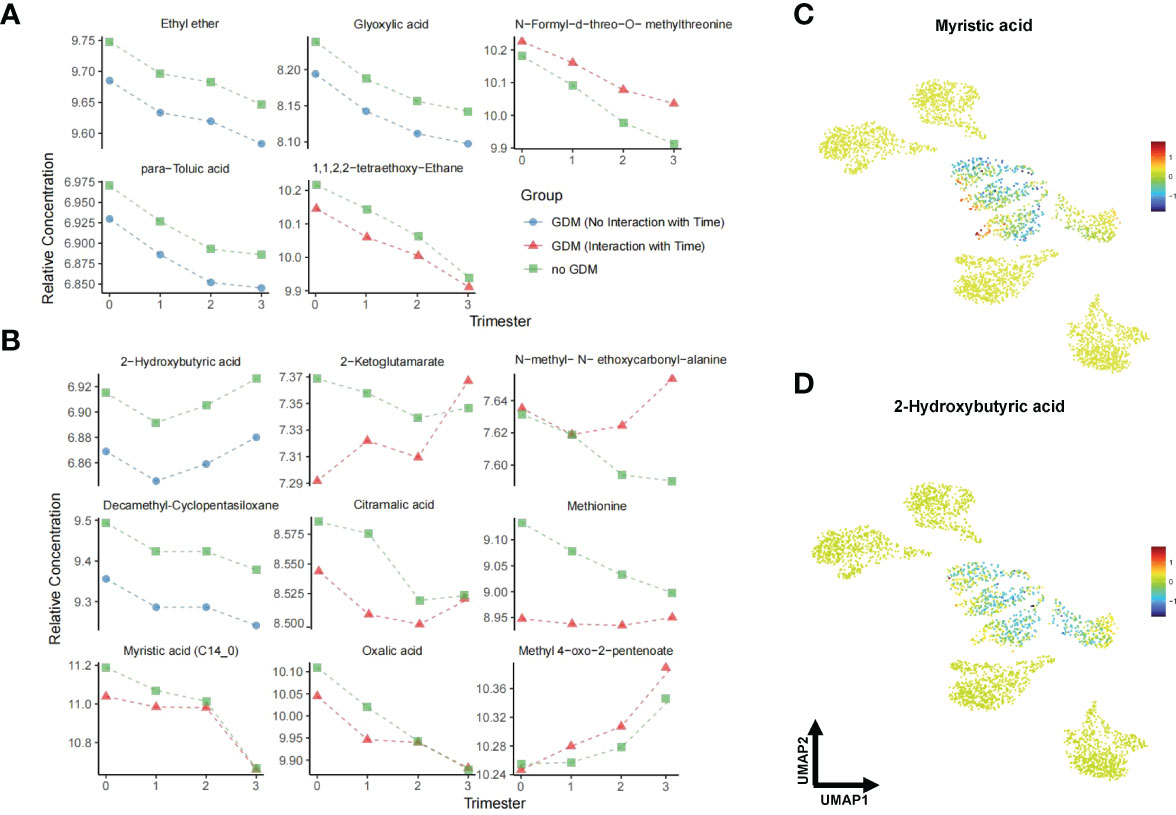
Figure 4 Line plots and UMAP of GDM-associated metabolites across pregnancy. (A) shows unadjusted models while (B) shows the results of linear mixed models adjusted for maternal age and BMI. Triangles represent the fitted effects in GDM maternal hair and squares represent non-GDM. Blue denotes a consistent effect across gestation in the GDM group (no significant interaction with time), while red corresponds to a time-specific effect. (C, D) UMAP representation of myristic acid and 2-hydroxybutyric acid from data in Figure 3 (A). Red dots indicate higher metabolite levels in GDM women compared with the non-GDM women, while blue dots indicate lower levels. Data are visualized after log transformation.
Air pollutions and GDM
The daily average calculated exposure concentrations of air pollution for each timepoint based on the proximal model are listed in Table 3. Table 4 describes the exposure estimates for PM2.5 and NO2 using the LUR model. The estimates of PM2.5 and NO2 according to the LUR model were higher in the GDM group during pre-conception and early pregnancy, respectively (p < 0.05). Figure 5 demonstrates the association between air pollution and GDM risk across four periods. After adjusting for maternal age and BMI, we only found that exposure to PM2.5 (LUR) within the pre-conception (OR=1.584, 95% CI: 1.032-2.432, p=0.035) was significantly associated with GDM occurrence. This result was consistent with PM2.5 estimated by the LUR model, which was higher in the GDM group during pre-conception.
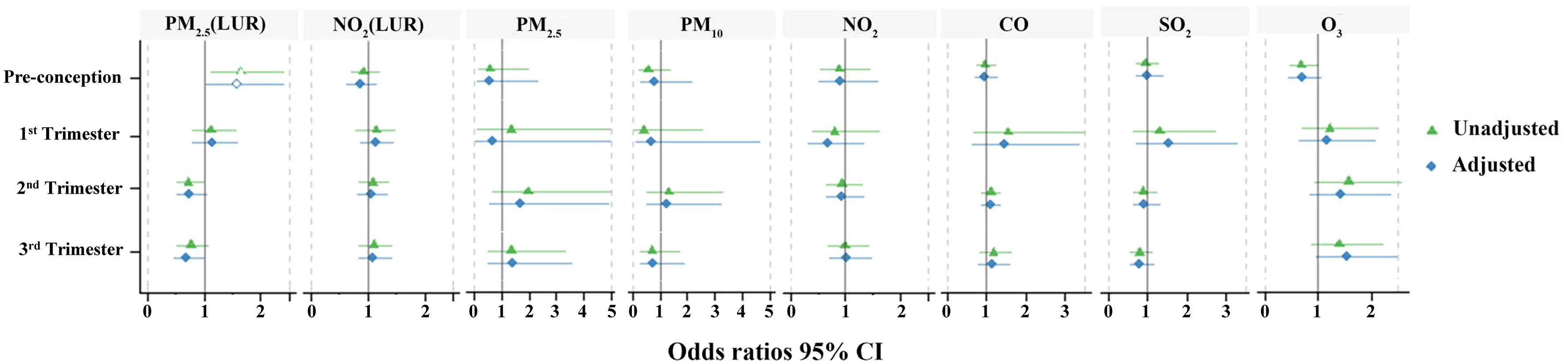
Figure 5 Odds ratios between air pollution exposures and GDM occurrence. Green indicates the unadjusted odds ratio (95% CI); blue indicates the adjusted odds ratio (95% CI). Hollow shapes represent statistical significance, while solid shapes are non-significant.
Air pollutions and hair metabolites
The relationships between GDM-associated hair metabolites and specific pollutants (including daily average concentrations of PM2.5, PM10, SO2, NO2, O3, CO and the exposure estimate data of PM2.5 and NO2) are illustrated in Figure 6. Three of the fourteen hair metabolites (2-hydroxybutyric acid, citramalic acid, and myristic acid) that showed a significant association with GDM were also significantly associated with some, or all of the air pollutant measures (q < 0.05, Table 5). Each of these were negatively associated with daily average concentrations of PM2.5, PM10, SO2, NO2, CO and the exposure estimate of PM2.5 and NO2, and positively associated with O3.
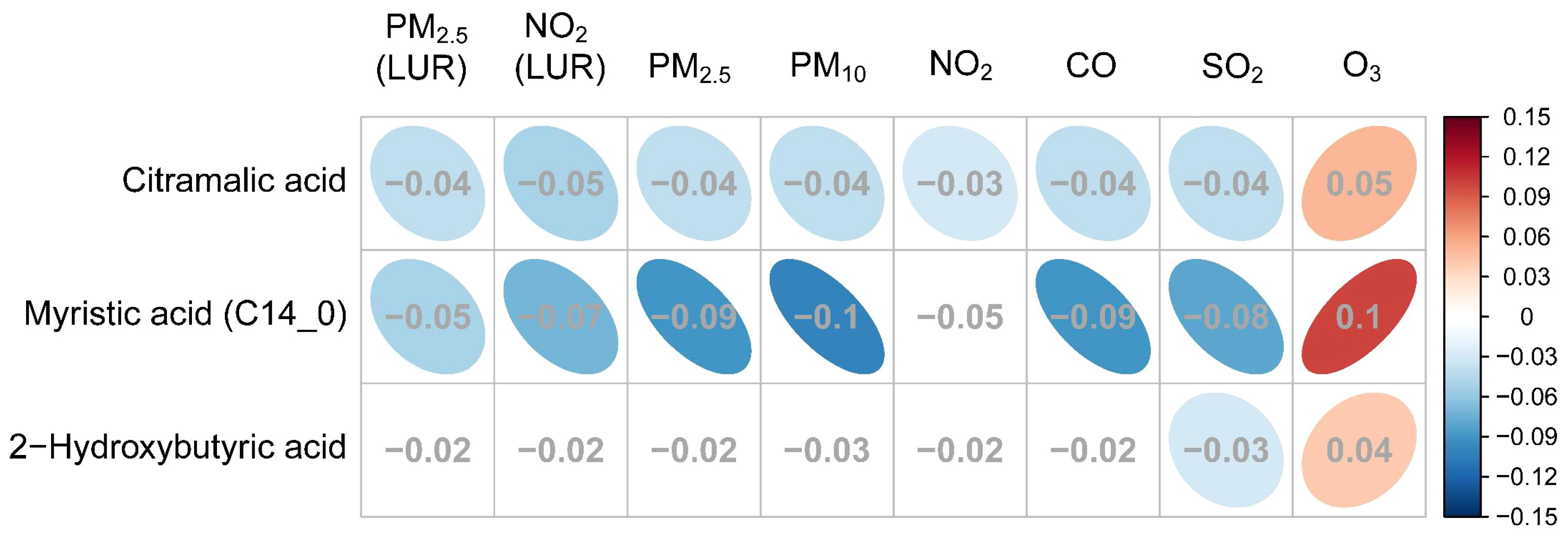
Figure 6 The association between GDM related metabolites and specific air pollutants. The grey numbers are standardized regression coefficients that show the effect of one standard derivation (SD) pollutant change on the standardized log metabolite concentration. The red right-handed ellipses indicate positive relationships between metabolites and air pollutants, while the blue left-handed ellipses indicate negative relationships. Only the significant associations (q-values < 0.05) between metabolites and pollutants are displayed by ellipses.
Standardized coefficients (showing the effect of one standard derivation pollutant change on the standardized log metabolite concentration) for PM2.5 and NO2 exposure estimated by the proximal and LUR models displayed a similar correlation, three metabolites were all negatively associated with PM2.5 and NO2. However, only citramalic acid was significantly associated with NO2 exposures estimated by the proximal model, but myristic acid and citramalic acid were remarkable in the LUR model, indicating that exposure estimation by the LUR model showed more statistical significance than the proximal model.
Discussion
To our knowledge, this is the first study to investigate associations between environmental exposures, the hair metabolome, and GDM longitudinally from pre-conception through to the third trimester. The main focus of hair metabolomic research to date has been the identification of metabolic biomarkers for the prediction of pregnancy complications (42–44). Our results demonstrated that the maternal hair metabolome was altered in response to endogenous and exogenous exposures, prior to, and throughout pregnancy. Moreover, exposure to PM2.5 during pre-conception may increase the risk of GDM. The levels of 2-hydroxybutyric acid, citramalic acid, and myristic acid in the hair of GDM women were negatively associated with the air pollutant levels. Our findings suggest that the hair metabolome changes in response to maternal and environmental perturbations with substantial potential to estimate exposure risk factors and better understand the underlying GDM pathophysiology.
Pre-conception exposure to air pollution may increase the risk of GDM
Air pollution has become a major public health issue in developed and developing countries (1). Particulate matter (PM) is composed of complex chemical constituents from a variety of sources, such as elemental carbon, metals, and organic chemicals derived from coal combustion, biomass burning, vehicle emissions, dust, and industrial sources (60). We have published a LUR model that was developed on a daily basis incorporating measurement data, temporal variables on meteorology, and spatial variables produced using a geographical information system (54). This spatiotemporal model demonstrated better performance than the proximal model in discriminating GDM and non-GDM groups from pre-conception to the first trimester. Our results demonstrated that the estimates of PM2.5 and NO2 by the LUR model were higher in the GDM group during pre-conception and in early pregnancy, respectively. Meanwhile, the calculated daily average exposure to PM2.5 and NO2 based on the proximal model did not show any differences from pre-conception to third trimester. Consistently, evidence from our study also suggested that PM2.5 exposure during pre-conception increased the risk of GDM. Similar to our results, Zhang et al. found that higher exposure to PM2.5 within three months before pregnancy was associated with increased GDM risk, as well as elevated fasting glucose levels (61). Another study in China showed that pre-conception PM2.5 and PM10 exposure was associated with a higher risk of developing GDM (62). In a case-control study in Taiwan, researchers found that higher pre-conception and pregnancy exposures to PM2.5 for mothers were associated with a significantly elevated risk of GDM (63). Yu et al. indicated that exposure to PM2.5 in the second trimester of pregnancy was associated with an increased risk of GDM (64). A pregnancy cohort in Southern California showed that pre-conception NO2 was associated with an increased risk of GDM, and first trimester NO2 was weakly associated with GDM (65). The majority of studies found that maternal exposure to PM2.5 and NO2 was associated with a significantly elevated risk of GDM. However, the results of recent meta-analyses indicated that increased exposure to PM2.5 was not statistically related to the incidence of GDM (30, 66). It is worth noting that inconsistence among these studies may reflect intrinsic dissimilarities in exposure assessment, outcome definition, effect estimation models and adjusted variables, which may contribute to the heterogeneity (31). Overall, these results suggest that exposure to higher levels of PM2.5 and NO2 during early pregnancy may increase susceptibility to GDM development.
The potential relationship between air pollution and GDM
It remains unclear how air pollution and its components are associated with GDM. Previous studies suggested that oxidative stress and inflammation may play important roles in the development of GDM. Oxidative stress is a well-recognised risk factor for insulin resistance. Several studies have shown unequivocally that oxidative stress precedes insulin resistance (67–69). An epidemiological study showed that air pollution exposure could increase markers for oxidative stress among pregnant women (70). Particulate matter (PM) is enriched with metals and organic chemicals and has been thought to initiate toxic effects and induce oxidative damage (71, 72). For instance, PM2.5 alters levels of oxidative stress biomarkers in mice including glutathione peroxidase and malonic dialdehyde (73). Research has identified oxidative stress as one potential feature underlying the toxic effect of air pollution, which activates NF-κB translocation into the nucleus and causes an inflammatory response and cytokine production (74–77). Meanwhile, air pollution can produce pro-inflammatory mediators, including highly sensitive C-Reactive Protein, TNF-α, IL-1β, IL-6 and IL-8, resulting in local or systemic inflammation (8, 78, 79). Increased inflammatory response plays an important role in the development of GDM (80). Moreover, It has been argued that NO2 can cause similar inflammatory responses to those of PM (81). Both experimental and epidemiological studies indicate that air pollution may induce oxidative damage and trigger the release of inflammatory cytokines, subsequently contributing to insulin resistance, which consequently promotes the development of GDM.
The association of air pollutants and hair metabolites in GDM
The metabolome reflects phenotypic changes in response to physiological status and environmental stimuli. There were three metabolites, myristic acid, 2-hydroxybutyric acid, and citramalic acid, found in significantly lower levels in the hair samples of women with GDM that were negatively associated with PM2.5, PM10, SO2, and CO. Of these three metabolites, myristic acid (14:0) levels were observed at lower levels in the hair samples of our GDM participants from the first trimester to the third trimester. Cumulative evidence has shown that fatty acids with different degrees of saturation affect insulin sensitivity and lipid/glucose metabolism differently. Saturated fatty acids are generally more likely to cause insulin resistance via inflammatory processes, while unsaturated fatty acids ameliorate the pathology associated with diabetes (82, 83). On the contrary, there are several studies showed that myristic acid has been inversely associated with a higher risk of type 2 diabetes (84–87). Interestingly, myristic acid has been demonstrated to increase glucose uptake in C2C12 skeletal muscle cells and reduce hyperglycaemia and insulin resistance in spontaneously diabetic Nagoya–Shibata–Yasuda (NSY) mice (88–90). However, the detailed effects of myristic acid on hyperglycaemia and insulin resistance have not been thoroughly investigated. It has been shown that the activity of delta-6(Δ6) desaturase (D6D) can be increased by myristic acid in cultured rat hepatocytes (91). Delta-6 desaturase, encoded by the fatty acid desaturase 2 (FADS2) gene, is the rate-limiting enzyme for the conversion of α-linolenic acid (ALA) to eicosapentaenoic acid (EPA) and docosahexaenoic acid (DHA) (92). Meanwhile, myristic acid has been reported to elevate EPA and DHA levels in human and rat plasma (93–95). EPA and DHA are long-chain polyunsaturated fatty acids (PUFAs), which have been found to be inversely associated with IL-6 and TNF-α concentrations and positively associated with the concentrations of the anti-inflammatory cytokines IL-10 and TGF-β (96). Since myristic acid promotes the production of EPA and DHA and is negatively correlated with air pollutants, the lower levels of myristic acid in the GDM hair samples could potentially link air pollution exposure to reduced anti-inflammatory capacity in women with GDM.
2-Hydroxybutyric acid (2-HB) was another hair metabolite that exhibited a lower concentration in GDM from pre-conception to the third trimester and was negatively associated with SO2. The results from a meta-analysis and cohort studies have found that maternal exposure to SO2 increased the risk of GDM (31, 63, 97, 98). Plasma 2-hydroxybutyric acid has been previously reported to be associated with insulin resistance and type 2 diabetes (99, 100). Research has demonstrated that 2-hydroxybutyric acid was produced from 2-ketobutyric acid in response to oxidative stress-induced glutathione synthesis (100, 101). Elevated oxidative stress may increase methionine catabolism by cystathionine β-synthase to produce cysteine for glutathione synthesis, while 2-ketobutyric acid is generated as a by-product (102). Indeed, a lower level of 2-ketobutyric acid (1st and 2nd trimesters), methionine (all four periods), and cysteine (3rd trimester) were also observed in the hair of women diagnosed with GDM. Since glutathione metabolism could be downregulated by hyperglycemia, a lower level of 2-hydroxybutyric acid may be reflecting poor antioxidant capacity in women with GDM.
Limitations
Lastly, we found that the spatiotemporal LUR model estimate data of PM2.5 and NO2 related to hair metabolites were more statistically significant than the classical proximal-distance model. LUR models are trained and validated against measured pollutant concentrations using variables generated from a geographic information system (GIS), such as distance to nearest source, road network density, land use, terrain, population density, and meteorological variables to predict the concentrations at residential locations. The specific limitations of the LUR models used in this study are described elsewhere (54). Despite the fact that the LUR model provides a better basis for air exposure estimation, only PM2.5 and NO2 LUR models are currently available for the CLIMB study. Furthermore, air pollutant exposures usually have a hysteresis effect, distributed lag non-linear model (DLNM) might be more appropriate to consider the lag time when estimating the effects of air pollutants on metabolites. Future studies should establish LUR models to estimate other air pollutants (PM10, SO2, CO, and O3) and consider delayed effects of air pollution for our Chongqing cohort. We should also validate our findings in a large multicentre cohort with diverse environmental exposures. In addition, it would be beneficial for future studies to consider pollutant exposure beyond the residential setting which was used in this study, expanding to include exposures from participants’ workplaces and outdoor activities. A potential limitation of this study was that lifestyle behaviours such as nutritional intake, physical activity, and stress during pregnancy were not accounted for and may have been a source of residual confounding. Future studies should also validate the significant metabolites by targeted metabolomics using calibration curves to determine their limits of detection (LODs).
Conclusions
This study was the first to investigate the association between environmental pollution, the hair metabolome, and GDM status longitudinally from pre-conception through to the third trimester. We demonstrated that there were significant alterations to the maternal hair metabolome, which were related to later GDM development. Three of these altered metabolites were also associated with maternal air pollution exposure reflecting that exposure to environmental pollutants can increase the risk of GDM. Based on these findings we propose that air pollution contributes to altered metabolism, and potentially an increase in oxidative stress and inflammatory reaction, all of which may contribute to the aetiology of GDM (Figure 7). Our findings that the hair metabolome is altered in response to maternal and environmental perturbations suggest that maternal hair could be used to estimate exposure risk factors and better understand the underlying GDM pathophysiology.
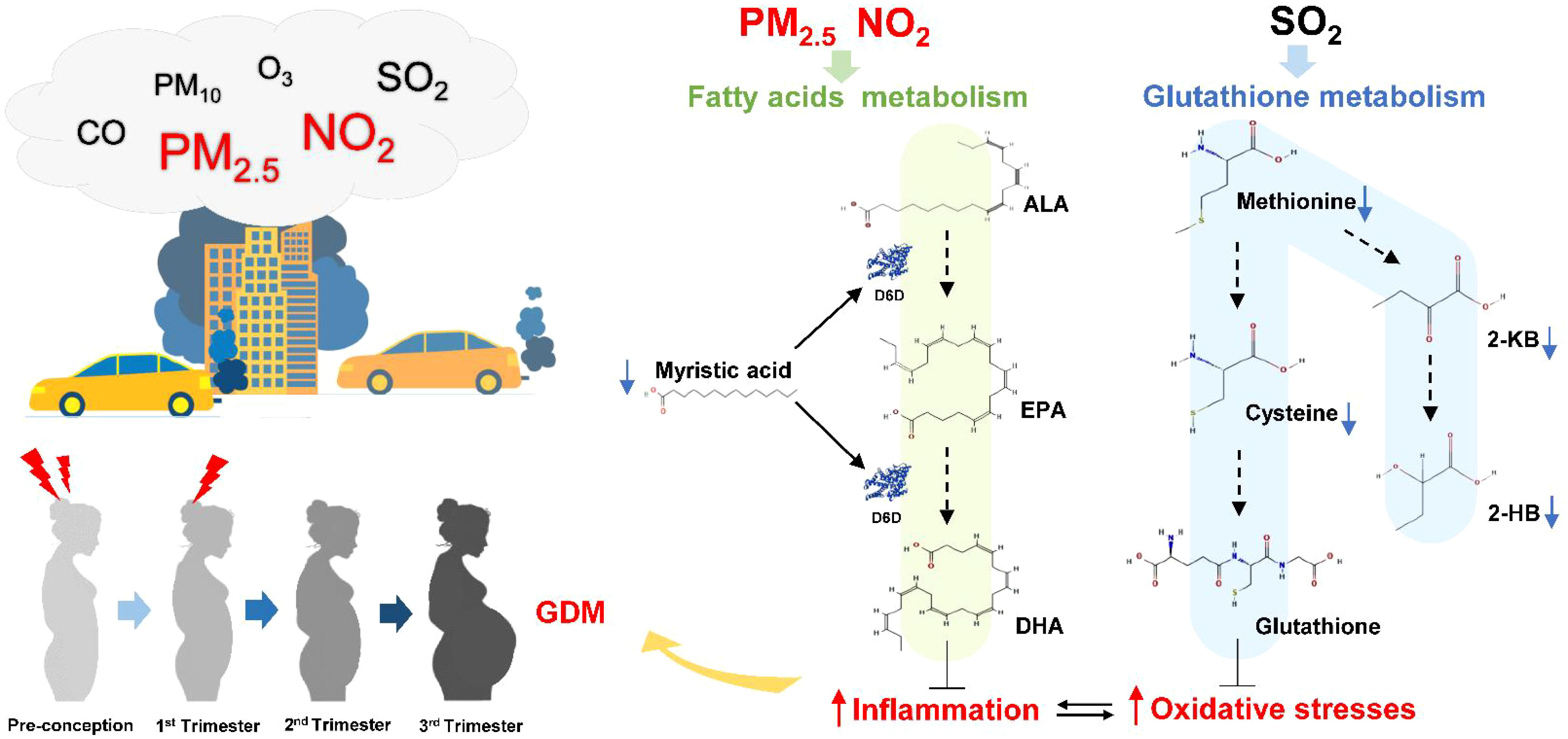
Figure 7 The potential pathways that air pollution exposure influences GDM development through fatty acids and glutathione metabolism. Higher PM2.5 and NO2 air pollution exposure from pre-conception to early pregnancy are likely to increase pregnant women’s susceptibility to GDM through induced oxidative stress and inflammation. Myristic acid could promote the production of EPA and DHA to compete against inflammation. Methionine is catablised into cysteine and 2-ketobutyric acid (2-KB), which in turn is converted into glutathione and 2-hydroxybutyric acid (2-HB) respectively. The lower level of myristic acid and 2-HB in hair could be related to poor antioxidant and anti-inflammatory capacity in women with GDM. Therefore, increased exposure to air pollutants might promote GDM pathogenesis by downregulating fatty acids and glutathione metabolism. Abbreviations as follows: ALA, α-linolenic acid; EPA, eicosapentaenoic acid; DHA, docosahexaenoic acid; D6D, delta-6 desaturase; 2-HB, 2-hydroxybutyric acid; 2-KB, 2-ketobutyric acid.
Data availability statement
The raw data supporting the conclusions of this article will be made available by the authors, without undue reservation.
Ethics statement
The studies involving human participants were reviewed and approved by the Ethics committee of the Chongqing Medical University. The patients/participants provided their written informed consent to participate in this study.
Author contributions
HQ, PB, JG, HZ, and T-LH conceived the idea and designed the study. YX and TZ take responsibility for the data and sample collection. XZ and YY performed sample preparation and GC-MS analysis. XC, XZ, MJ, AH, and T-LH analyzed data. XC, JS, and T-LH contributed to the writing of the manuscript. RS, PB, HQ, RC, and HZ critically revised the manuscript. All authors contributed to the article and approved the submitted version.
Funding
This work was supported by the National Natural Science Foundation of China (No. 81971406, 81871185), The 111 Project (Yuwaizhuan (2016)32), Chongqing Science & Technology Commission (cstc2021jcyj-msxmX0213), Chongqing Municipal Education Commission (KJZD-K202100407), Chongqing Health Commission and Chongqing Science & Technology Commission (2021MSXM121, 2020MSXM101).
Acknowledgments
The authors thank all collaborators for data collection. We also thank all the study participants. The authors are also grateful to support provided by The Chongqing Key Laboratory of Translational Medicine in Major Metabolic Diseases.
Conflict of interest
The authors declare that the research was conducted in the absence of any commercial or financial relationships that could be construed as a potential conflict of interest.
The handling editor RZ declared a shared affiliation with the authors XC, XZ, YY, YX, TZ, HQ, HZ, T-LH at the time of review.
Publisher’s note
All claims expressed in this article are solely those of the authors and do not necessarily represent those of their affiliated organizations, or those of the publisher, the editors and the reviewers. Any product that may be evaluated in this article, or claim that may be made by its manufacturer, is not guaranteed or endorsed by the publisher.
Supplementary material
The Supplementary Material for this article can be found online at: https://www.frontiersin.org/articles/10.3389/fendo.2022.1060309/full#supplementary-material
Abbreviations
ALA, α-linolenic acid; BMI, body mass index; CO, carbon monoxide; CLIMB, Complex Lipids in Mothers and Babies; D6D, delta-6 desaturase; DHA, docosahexaenoic acid; EPA, eicosapentaenoic acid; GC-MS, gas chromatography-mass spectrometry; GDM, Gestational diabetes mellitus; LUR, land use regression; MCF, Methyl chloroformate; NO2, nitrogen dioxide; O3, ozone; PM2.5, particulate matter less than 2.5μm in diameter; PM10, particulate matter less than 10μm in diameter; SO2, sulfur dioxide; UMAP, Uniform Manifold Approximation and Projection; 2-HB, 2-hydroxybutyric acid; 2-KB, 2-ketobutyric acid.
References
1. Cohen AJ, Brauer M, Burnett R, Anderson HR, Frostad J, Estep K, et al. Estimates and 25-year trends of the global burden of disease attributable to ambient air pollution: An analysis of data from the global burden of diseases study 2015. Lancet (2017) 389(10082):1907–18. doi: 10.1016/S0140-6736(17)30505-6
2. Landrigan PJ, Fuller R, Acosta NJR, Adeyi O, Arnold R, Basu NN, et al. The lancet commission on pollution and health. Lancet (2018) 391(10119):462–512. doi: 10.1016/S0140-6736(17)32345-0
3. Yin P, Brauer M, Cohen AJ, Wang H, Li J, Burnett RT, et al. The effect of air pollution on deaths, disease burden, and life expectancy across China and its provinces, 1990-2017: An analysis for the global burden of disease study 2017. Lancet Planet Health (2020) 4(9):e386–e98. doi: 10.1016/S2542-5196(20)30161-3
4. Andersen ZJ, Hvidberg M, Jensen SS, Ketzel M, Loft S, Sorensen M, et al. Chronic obstructive pulmonary disease and long-term exposure to traffic-related air pollution: A cohort study. Am J Respir Crit Care Med (2011) 183(4):455–61. doi: 10.1164/rccm.201006-0937OC
5. Liu F, Chen G, Huo W, Wang C, Liu S, Li N, et al. Associations between long-term exposure to ambient air pollution and risk of type 2 diabetes mellitus: A systematic review and meta-analysis. Environ Pollut (2019) 252(Pt B):1235–45. doi: 10.1016/j.envpol.2019.06.033
6. Meo SA, Memon AN, Sheikh SA, Rouq FA, Usmani AM, Hassan A, et al. Effect of environmental air pollution on type 2 diabetes mellitus. Eur Rev Med Pharmacol Sci (2015) 19(1):123–8. Available at: https://www.ncbi.nlm.nih.gov/pubmed/25635985
7. Raaschou-Nielsen O, Andersen ZJ, Beelen R, Samoli E, Stafoggia M, Weinmayr G, et al. Air pollution and lung cancer incidence in 17 European cohorts: Prospective analyses from the European study of cohorts for air pollution effects (Escape). Lancet Oncol (2013) 14(9):813–22. doi: 10.1016/S1470-2045(13)70279-1
8. Sun Q, Yue P, Deiuliis JA, Lumeng CN, Kampfrath T, Mikolaj MB, et al. Ambient air pollution exaggerates adipose inflammation and insulin resistance in a mouse model of diet-induced obesity. Circulation (2009) 119(4):538–46. doi: 10.1161/CIRCULATIONAHA.108.799015
9. Vrijheid M, Slama R, Robinson O, Chatzi L, Coen M, van den Hazel P, et al. The human early-life exposome (Helix): Project rationale and design. Environ Health Perspect (2014) 122(6):535–44. doi: 10.1289/ehp.1307204
10. Royal College of Obstetricians & Gynaecologists. Chemical exposures during pregnancy: Dealing with potential, but unproven, risks to child health (2013). Available at: https://www.rcog.org.uk/en/guidelines-research-services/guidelines/sip37/.
11. Gomez-Roig MD, Pascal R, Cahuana MJ, Garcia-Algar O, Sebastiani G, Andreu-Fernandez V, et al. Environmental exposure during pregnancy: Influence on prenatal development and early life: A comprehensive review. Fetal diagnosis Ther (2021) 48(4):245–57. doi: 10.1159/000514884
12. Zanini MJ, Dominguez C, Fernandez-Oliva T, Sanchez O, Toda MT, Foraster M, et al. Urban-related environmental exposures during pregnancy and placental development and preeclampsia: A review. Curr Hypertens Rep (2020) 22(10):81. doi: 10.1007/s11906-020-01088-4
13. Rappazzo KM, Nichols JL, Rice RB, Luben TJ. Ozone exposure during early pregnancy and preterm birth: A systematic review and meta-analysis. Environ Res (2021) 198:111317. doi: 10.1016/j.envres.2021.111317
14. Quenby S, Gallos ID, Dhillon-Smith RK, Podesek M, Stephenson MD, Fisher J, et al. Miscarriage matters: The epidemiological, physical, psychological, and economic costs of early pregnancy loss. Lancet (2021) 397(10285):1658–67. doi: 10.1016/S0140-6736(21)00682-6
15. Bearblock E, Aiken CE, Burton GJ. Air pollution and pre-eclampsia; associations and potential mechanisms. Placenta (2021) 104:188–94. doi: 10.1016/j.placenta.2020.12.009
16. Jia L, Liu Q, Hou H, Guo G, Zhang T, Fan S, et al. Association of ambient air pollution with risk of preeclampsia during pregnancy: A retrospective cohort study. BMC Public Health (2020) 20(1):1663. doi: 10.1186/s12889-020-09719-w
17. Kim JH, Choi YY, Yoo SI, Kang DR. Association between ambient air pollution and high-risk pregnancy: A 2015-2018 national population-based cohort study in Korea. Environ Res (2021) 197:110965. doi: 10.1016/j.envres.2021.110965
18. Liang L, Rasmussen MH, Piening B, Shen X, Chen S, Rost H, et al. Metabolic dynamics and prediction of gestational age and time to delivery in pregnant women. Cell (2020) 181(7):1680–92 e15. doi: 10.1016/j.cell.2020.05.002
19. Lain KY, Catalano PM. Metabolic changes in pregnancy. Clin obstetrics gynecology (2007) 50(4):938–48. doi: 10.1097/GRF.0b013e31815a5494
20. Zhu WW, Yang HX, Wang C, Su RN, Feng H, Kapur A. High prevalence of gestational diabetes mellitus in Beijing: Effect of maternal birth weight and other risk factors. Chin Med J (2017) 130(9):1019–25. doi: 10.4103/0366-6999.204930
21. Reece EA, Leguizamon G, Wiznitzer A. Gestational diabetes: The need for a common ground. Lancet (2009) 373(9677):1789–97. doi: 10.1016/S0140-6736(09)60515-8
22. Vounzoulaki E, Khunti K, Abner SC, Tan BK, Davies MJ, Gillies CL. Progression to type 2 diabetes in women with a known history of gestational diabetes: Systematic review and meta-analysis. Bmj (2020) 369:m1361. doi: 10.1136/bmj.m1361
23. American Diabetes Association. 2. classification and diagnosis of diabetes: Standards of medical care in diabetes-2021. Diabetes Care (2021) 44(Suppl 1):S15–33. doi: 10.2337/dc21-S002
24. Ben-Haroush A, Yogev Y, Hod M. Epidemiology of gestational diabetes mellitus and its association with type 2 diabetes. Diabetic Med J Br Diabetic Assoc (2004) 21(2):103–13. doi: 10.1046/j.1464-5491.2003.00985.x
25. Riant M, Meirhaeghe A, Giovannelli J, Occelli F, Havet A, Cuny D, et al. Associations between long-term exposure to air pollution, glycosylated hemoglobin, fasting blood glucose and diabetes mellitus in northern France. Environ Int (2018) 120:121–9. doi: 10.1016/j.envint.2018.07.034
26. Rao X, Montresor-Lopez J, Puett R, Rajagopalan S, Brook RD. Ambient air pollution: An emerging risk factor for diabetes mellitus. Curr Diabetes Rep (2015) 15(6):603. doi: 10.1007/s11892-015-0603-8
27. Rajagopalan S, Brook RD. Air pollution and type 2 diabetes: Mechanistic insights. Diabetes (2012) 61(12):3037–45. doi: 10.2337/db12-0190
28. Angueira AR, Ludvik AE, Reddy TE, Wicksteed B, Lowe WL Jr., Layden BT. New insights into gestational glucose metabolism: Lessons learned from 21st century approaches. Diabetes (2015) 64(2):327–34. doi: 10.2337/db14-0877
29. Zhang C, Bao W, Rong Y, Yang H, Bowers K, Yeung E, et al. Genetic variants and the risk of gestational diabetes mellitus: A systematic review. Hum Reprod Update (2013) 19(4):376–90. doi: 10.1093/humupd/dmt013
30. Tang X, Zhou JB, Luo F, Han Y, Heianza Y, Cardoso MA, et al. Air pollution and gestational diabetes mellitus: Evidence from cohort studies. BMJ Open Diabetes Res Care (2020) 8(1):e000937. doi: 10.1136/bmjdrc-2019-000937
31. Zhang H, Wang Q, He S, Wu K, Ren M, Dong H, et al. Ambient air pollution and gestational diabetes mellitus: A review of evidence from biological mechanisms to population epidemiology. Sci total Environ (2020) 719:137349. doi: 10.1016/j.scitotenv.2020.137349
32. Elshahidi MH. Outdoor air pollution and gestational diabetes mellitus: A systematic review and meta-analysis. Iranian J Public Health (2019) 48(1):9–19. doi: 10.18502/ijph.v48i1.778
33. Lim CC, Thurston GD. Air pollution, oxidative stress, and diabetes: A life course epidemiologic perspective. Curr Diabetes Rep (2019) 19(8):58. doi: 10.1007/s11892-019-1181-y
34. Zhang H, Zhao Y. Ambient air pollution exposure during pregnancy and gestational diabetes mellitus in shenyang, China: A prospective cohort study. Environ Sci Pollut Res Int (2021) 28(7):7806–14. doi: 10.1007/s11356-020-11143-x
35. Zheng Y, Wen X, Bian J, Lipkind H, Hu H. Associations between the chemical composition of Pm2.5 and gestational diabetes mellitus. Environ Res (2021) 198:110470. doi: 10.1016/j.envres.2020.110470
36. Holmes E, Wilson ID, Nicholson JK. Metabolic phenotyping in health and disease. Cell (2008) 134(5):714–7. doi: 10.1016/j.cell.2008.08.026
37. Clish CB. Metabolomics: An emerging but powerful tool for precision medicine. Cold Spring Harbor Mol Case Stud (2015) 1(1):a000588. doi: 10.1101/mcs.a000588
38. Saravanan Dayalan JX, Rachel A, Spicer, Salek R, Roessner U. Metabolome analysis. In: Ranganathan S, Gribskov M, Nakai K, Schönbach C, editors. Encyclopedia of bioinformatics and computational biology. 2. Amsterdam, Netherlands: Academic Press (2019). p. 396–409.
39. Pellis L, van Erk MJ, van Ommen B, Bakker GC, Hendriks HF, Cnubben NH, et al. Plasma metabolomics and proteomics profiling after a postprandial challenge reveal subtle diet effects on human metabolic status. Metabolomics Off J Metabol Soc (2012) 8(2):347–59. doi: 10.1007/s11306-011-0320-5
40. Dunn WB, Broadhurst D, Begley P, Zelena E, Francis-McIntyre S, Anderson N, et al. Procedures for Large-scale metabolic profiling of serum and plasma using gas chromatography and liquid chromatography coupled to mass spectrometry. Nat Protoc (2011) 6(7):1060–83. doi: 10.1038/nprot.2011.335
41. Eastman RR, Jursa TP, Benedetti C, Lucchini RG, Smith DR. Hair as a biomarker of environmental manganese exposure. Environ Sci Technol (2013) 47(3):1629–37. doi: 10.1021/es3035297
42. Sulek K, Han TL, Villas-Boas SG, Wishart DS, Soh SE, Kwek K, et al. Hair metabolomics: Identification of fetal compromise provides proof of concept for biomarker discovery. Theranostics (2014) 4(9):953–9. doi: 10.7150/thno.9265
43. He X, de Seymour JV, Sulek K, Qi H, Zhang H, Han TL, et al. Maternal hair metabolome analysis identifies a potential marker of lipid peroxidation in gestational diabetes mellitus. Acta Diabetol (2016) 53(1):119–22. doi: 10.1007/s00592-015-0737-9
44. Chen X, de Seymour JV, Han TL, Xia Y, Chen C, Zhang T, et al. Metabolomic biomarkers and novel dietary factors associated with gestational diabetes in China. Metabolomics (2018) 14(11):149. doi: 10.1007/s11306-018-1445-6
45. Huang S, Mo TT, Norris T, Sun S, Zhang T, Han TL, et al. The climb (Complex lipids in mothers and babies) study: Protocol for a multicentre, three-group, parallel randomised controlled trial to investigate the effect of supplementation of complex lipids in pregnancy, on maternal ganglioside status and subsequent cognitive outcomes in the offspring. BMJ Open (2017) 7(10):e016637. doi: 10.1136/bmjopen-2017-016637
46. Norris T, Souza R, Xia Y, Zhang T, Rowan A, Gallier S, et al. Effect of supplementation of complex milk lipids in pregnancy on fetal growth: Results from the complex lipids in mothers and babies (Climb) randomized controlled trial. J Matern Fetal Neonatal Med (2021) 34(20):3313–22. doi: 10.1080/14767058.2019.1683539
47. Metzger BE, Gabbe SG, Persson B, Buchanan TA, Catalano PA, Damm P, et al. International association of diabetes and pregnancy study groups recommendations on the diagnosis and classification of hyperglycemia in pregnancy. Diabetes Care (2010) 33(3):676–82. doi: 10.2337/dc09-1848
48. Delplancke TDJ, de Seymour JV, Tong C, Sulek K, Xia Y, Zhang H, et al. Analysis of sequential hair segments reflects changes in the metabolome across the trimesters of pregnancy. Sci Rep (2018) 8(1):36. doi: 10.1038/s41598-017-18317-7
49. Smart KF, Aggio RB, Van Houtte JR, Villas-Boas SG. Analytical platform for metabolome analysis of microbial cells using methyl chloroformate derivatization followed by gas chromatography-mass spectrometry. Nat Protoc (2010) 5(10):1709–29. doi: 10.1038/nprot.2010.108
50. Han TL, Cannon RD, Gallo SM, Villas-Boas SG. A metabolomic study of the effect of candida albicans glutamate dehydrogenase deletion on growth and morphogenesis. NPJ Biofilms Microbiomes (2019) 5(1):13. doi: 10.1038/s41522-019-0086-5
51. Yang Y, Yin Y, Chen X, Chen C, Xia Y, Qi H, et al. Evaluating different extraction solvents for gc-Ms based metabolomic analysis of the fecal metabolome of adult and baby giant pandas. Sci Rep (2019) 9(1):12017. doi: 10.1038/s41598-019-48453-1
52. George GUO, Elizabeth J. McKenzie M, Jones B, Zarate E, de Seymour J, Baker PN, et al. Massomics: An r package of a cross-platform data processing pipeline for Large-scale gc-Ms untargeted metabolomics datasets. Geneva, Switzerland (2021). doi: 10.5281/zenodo.4961895.
53. Chongqing Ecological Protection Bureau. Atmospheric environmental quality (2020). Available at: http://sthjj.cq.gov.cn/hjzl_249/.
54. Harper A, Baker PN, Xia YY, Kuang T, Zhang H, Chen YX, et al. Development of spatiotemporal land use regression models for Pm2.5 and No2 in chongqing, China, and exposure assessment for the climb study. Atmospheric pollut Res (2021) 12(7):101096. doi: 10.1016/j.apr.2021.101096
55. Konopka T. Umap: Uniform manifold approximation and projection (2022). Available at: http://CRAN.R-project.org/package=umap.
56. Bates D, Machler M, Bolker BM, Walker SC. Fitting linear mixed-effects models using Lme4. J Stat Softw (2015) 67(1):1–48. doi: 10.18637/Jss.V067.I01
57. Storey JD, Bass AJ, Dabney A, Robinson D. Qvalue: Q-value estimation for false discovery rate control (2020). Available at: http://github.com/jdstorey/qvalue.
58. Juan J, Yang H. Prevalence, prevention, and lifestyle intervention of gestational diabetes mellitus in China. Int J Environ Res Public Health (2020) 17(24):9517. doi: 10.3390/ijerph17249517
59. McIntyre HD, Catalano P, Zhang C, Desoye G, Mathiesen ER, Damm P. Gestational diabetes mellitus. Nat Rev Dis Primers (2019) 5(1):47. doi: 10.1038/s41572-019-0098-8
60. Yao L, Yang L, Yuan Q, Yan C, Dong C, Meng C, et al. Sources apportionment of Pm2.5 in a background site in the north China plain. Sci Total Environ (2016) 541:590–8. doi: 10.1016/j.scitotenv.2015.09.123
61. Zhang M, Wang X, Yang X, Dong T, Hu W, Guan Q, et al. Increased risk of gestational diabetes mellitus in women with higher prepregnancy ambient Pm2.5 exposure. Sci Total Environ (2020) 730:138982. doi: 10.1016/j.scitotenv.2020.138982
62. Yao MN, Liu Y, Jin D, Yin WJ, Ma SS, Tao RX, et al. Relationship betweentemporal distribution of air pollution exposure and glucose homeostasis during pregnancy. Environ Res (2020) 185:109456. doi: 10.1016/j.envres.2020.109456
63. Shen HN, Hua SY, Chiu CT, Li CY. Maternal exposure to air pollutants and risk of gestational diabetes mellitus in Taiwan. Int J Environ Res Public Health (2017) 14(12):1604. doi: 10.3390/ijerph14121604
64. Yu G, Ao J, Cai J, Luo Z, Martin R, Donkelaar AV, et al. Fine particular matter and its constituents in air pollution and gestational diabetes mellitus. Environ Int (2020) 142:105880. doi: 10.1016/j.envint.2020.105880
65. Jo H, Eckel SP, Chen JC, Cockburn M, Martinez MP, Chow T, et al. Associations of gestational diabetes mellitus with residential air pollution exposure in a Large southern California pregnancy cohort. Environ Int (2019) 130:104933. doi: 10.1016/j.envint.2019.104933
66. Bai W, Li Y, Niu Y, Ding Y, Yu X, Zhu B, et al. Association between ambient air pollution and pregnancy complications: A systematic review and meta-analysis of cohort studies. Environ Res (2020) 185:109471. doi: 10.1016/j.envres.2020.109471
67. Houstis N, Rosen ED, Lander ES. Reactive oxygen species have a causal role in multiple forms of insulin resistance. Nature (2006) 440(7086):944–8. doi: 10.1038/nature04634
68. Hoehn KL, Salmon AB, Hohnen-Behrens C, Turner N, Hoy AJ, Maghzal GJ, et al. Insulin resistance is a cellular antioxidant defense mechanism. Proc Natl Acad Sci USA (2009) 106(42):17787–92. doi: 10.1073/pnas.0902380106
69. Styskal J, Van Remmen H, Richardson A, Salmon AB. Oxidative stress and diabetes: What can we learn about insulin resistance from antioxidant mutant mouse models? Free Radical Bio Med (2012) 52(1):46–58. doi: 10.1016/j.freeradbiomed.2011.10.441
70. Nagiah S, Phulukdaree A, Naidoo D, Ramcharan K, Naidoo RN, Moodley D, et al. Oxidative stress and air pollution exposure during pregnancy: A molecular assessment. Hum Exp Toxicol (2015) 34(8):838–47. doi: 10.1177/0960327114559992
71. Nel A. Atmosphere. air pollution-related illness: Effects of particles. Science (2005) 308(5723):804–6. doi: 10.1126/science.1108752
72. Lui KH, Jones T, BeruBe K, Ho SSH, Yim SHL, Cao JJ, et al. The effects of particle-induced oxidative damage from exposure to airborne fine particulate matter components in the vicinity of landfill sites on Hong Kong. Chemosphere (2019) 230:578–86. doi: 10.1016/j.chemosphere.2019.05.079
73. Araujo JA, Barajas B, Kleinman M, Wang X, Bennett BJ, Gong KW, et al. Ambient particulate pollutants in the ultrafine range promote early atherosclerosis and systemic oxidative stress. Circ Res (2008) 102(5):589–96. doi: 10.1161/CIRCRESAHA.107.164970
74. Kelly FJ. Oxidative stress: Its role in air pollution and adverse health effects. Occup Environ Med (2003) 60(8):612–6. doi: 10.1136/oem.60.8.612
75. Lappas M, Hiden U, Desoye G, Froehlich J, Hauguel-de Mouzon S, Jawerbaum A. The role of oxidative stress in the pathophysiology of gestational diabetes mellitus. Antioxid Redox Signal (2011) 15(12):3061–100. doi: 10.1089/ars.2010.3765
76. Shukla A, Timblin C, BeruBe K, Gordon T, McKinney W, Driscoll K, et al. Inhaled particulate matter causes expression of nuclear factor (Nf)-Kappab-Related genes and oxidant-dependent nf-kappab activation in vitro. Am J Respir Cell Mol Biol (2000) 23(2):182–7. doi: 10.1165/ajrcmb.23.2.4035
77. Gorini F, Sabatino L, Gaggini M, Chatzianagnostou K, Vassalle C. Oxidative stress biomarkers in the relationship between type 2 diabetes and air pollution. Antioxidants-Basel (2021) 10(8):1234. doi: 10.3390/Antiox10081234
78. Guan L, Geng X, Stone C, Cosky EEP, Ji Y, Du H, et al. Pm2.5 exposure induces systemic inflammation and oxidative stress in an intracranial atherosclerosis rat model. Environ Toxicol (2019) 34(4):530–8. doi: 10.1002/tox.22707
79. Pope CA, Bhatnagar A, McCracken JP, Abplanalp W, Conklin DJ, O'Toole T. Exposure to fine particulate air pollution is associated with endothelial injury and systemic inflammation. Circ Res (2016) 119(11):1204–+. doi: 10.1161/Circresaha.116.309279
80. Abell SK, De Courten B, Boyle JA, Teede HJ. Inflammatory and other biomarkers: Role in pathophysiology and prediction of gestational diabetes mellitus. Int J Mol Sci (2015) 16(6):13442–73. doi: 10.3390/ijms160613442
81. Hesterberg TW, Bunn WB, McClellan RO, Hamade AK, Long CM, Valberg PA. Critical review of the human data on short-term nitrogen dioxide (No2) exposures: Evidence for No2 no-effect levels. Crit Rev Toxicol (2009) 39(9):743–81. doi: 10.3109/10408440903294945
82. Riserus U, Willett WC, Hu FB. Dietary fats and prevention of type 2 diabetes. Prog Lipid Res (2009) 48(1):44–51. doi: 10.1016/j.plipres.2008.10.002
83. Holzer RG, Park EJ, Li N, Tran H, Chen M, Choi C, et al. Saturated fatty acids induce c-src clustering within membrane subdomains, leading to jnk activation. Cell (2011) 147(1):173–84. doi: 10.1016/j.cell.2011.08.034
84. Tong X, Dong JY, Wu ZW, Li W, Qin LQ. Dairy consumption and risk of type 2 diabetes mellitus: A meta-analysis of cohort studies. Eur J Clin Nutr (2011) 65(9):1027–31. doi: 10.1038/ejcn.2011.62
85. Aune D, Norat T, Romundstad P, Vatten LJ. Dairy products and the risk of type 2 diabetes: A systematic review and dose-response meta-analysis of cohort studies. Am J Clin Nutr (2013) 98(4):1066–83. doi: 10.3945/ajcn.113.059030
86. Ericson U, Hellstrand S, Brunkwall L, Schulz CA, Sonestedt E, Wallstrom P, et al. Food sources of fat may clarify the inconsistent role of dietary fat intake for incidence of type 2 diabetes. Am J Clin Nutr (2015) 101(5):1065–80. doi: 10.3945/ajcn.114.103010
87. Drehmer M, Pereira MA, Schmidt MI, Del Carmen BMM, Alvim S, Lotufo PA, et al. Associations of dairy intake with glycemia and insulinemia, independent of obesity, in Brazilian adults: The Brazilian longitudinal study of adult health (Elsa-brasil). Am J Clin Nutr (2015) 101(4):775–82. doi: 10.3945/ajcn.114.102152
88. Sakiyama S, Usuki T, Sakai H, Sakane F. Regulation of diacylglycerol kinase Delta2 expression in C2c12 skeletal muscle cells by free fatty acids. Lipids (2014) 49(7):633–40. doi: 10.1007/s11745-014-3912-9
89. Wada Y, Sakiyama S, Sakai H, Sakane F. Myristic acid enhances diacylglycerol kinase delta-dependent glucose uptake in myotubes. Lipids (2016) 51(8):897–903. doi: 10.1007/s11745-016-4162-9
90. Takato T, Iwata K, Murakami C, Wada Y, Sakane F. Chronic administration of myristic acid improves hyperglycaemia in the Nagoya-Shibata-Yasuda mouse model of congenital type 2 diabetes. Diabetologia (2017) 60(10):2076–83. doi: 10.1007/s00125-017-4366-4
91. Jan S, Guillou H, D'Andrea S, Daval S, Bouriel M, Rioux V, et al. Myristic acid increases Delta6-desaturase activity in cultured rat hepatocytes. Reprod nutrition Dev (2004) 44(2):131–40. doi: 10.1051/rnd:2004020
92. Tosi F, Sartori F, Guarini P, Olivieri O, Martinelli N. Delta-5 and delta-6 desaturases: Crucial enzymes in polyunsaturated fatty acid-related pathways with pleiotropic influences in health and disease. Adv Exp Med Biol (2014) 824:61–81. doi: 10.1007/978-3-319-07320-0_7
93. Rioux V, Catheline D, Bouriel M, Legrand P. Dietary myristic acid at physiologically relevant levels increases the tissue content of C20 : 5 n-3 and C20 : 3 n-6 in the rat. Reprod Nutr Dev (2005) 45(5):599–612. doi: 10.1051/rnd:2005048
94. Dabadie H, Peuchant E, Bernard M, LeRuyet P, Mendy F. Moderate intake of myristic acid in Sn-2 position has beneficial lipidic effects and enhances dha of cholesteryl esters in an interventional study. J Nutr Biochem (2005) 16(6):375–82. doi: 10.1016/j.jnutbio.2005.01.010
95. Dabadie H, Motta C, Peuchant E, LeRuyet P, Mendy F. Variations in daily intakes of myristic and alpha-linolenic acids in Sn-2 position modify lipid profile and red blood cell membrane fluidity. Br J Nutr (2006) 96(2):283–9. doi: 10.1079/bjn20061813
96. Ferrucci L, Cherubini A, Bandinelli S, Bartali B, Corsi A, Lauretani F, et al. Relationship of plasma polyunsaturated fatty acids to circulating inflammatory markers. J Clin Endocrinol Metab (2006) 91(2):439–46. doi: 10.1210/jc.2005-1303
97. Robledo CA, Mendola P, Yeung E, Mannisto T, Sundaram R, Liu D, et al. Preconception and early pregnancy air pollution exposures and risk of gestational diabetes mellitus. Environ Res (2015) 137:316–22. doi: 10.1016/j.envres.2014.12.020
98. Zhang H, Dong H, Ren M, Liang Q, Shen X, Wang Q, et al. Ambient air pollution exposure and gestational diabetes mellitus in guangzhou, China: A prospective cohort study. Sci total Environ (2020) 699:134390. doi: 10.1016/j.scitotenv.2019.134390
99. Gall WE, Beebe K, Lawton KA, Adam KP, Mitchell MW, Nakhle PJ, et al. Alpha-hydroxybutyrate is an early biomarker of insulin resistance and glucose intolerance in a nondiabetic population. PloS One (2010) 5(5):e10883. doi: 10.1371/journal.pone.0010883
100. Cobb J, Eckhart A, Motsinger-Reif A, Carr B, Groop L, Ferrannini E. Alpha-hydroxybutyric acid is a selective metabolite biomarker of impaired glucose tolerance. Diabetes Care (2016) 39(6):988–95. doi: 10.2337/dc15-2752
101. Ferrannini E, Natali A, Camastra S, Nannipieri M, Mari A, Adam KP, et al. Early metabolic markers of the development of dysglycemia and type 2 diabetes and their physiological significance. Diabetes (2013) 62(5):1730–7. doi: 10.2337/db12-0707
Keywords: gestational diabetes mellitus, cohort study, metabolomics, hair analysis, air pollution
Citation: Chen X, Zhao X, Jones MB, Harper A, de Seymour JV, Yang Y, Xia Y, Zhang T, Qi H, Gulliver J, Cannon RD, Saffery R, Zhang H, Han T-L and Baker PN (2022) The relationship between hair metabolites, air pollution exposure and gestational diabetes mellitus: A longitudinal study from pre-conception to third trimester. Front. Endocrinol. 13:1060309. doi: 10.3389/fendo.2022.1060309
Received: 03 October 2022; Accepted: 18 November 2022;
Published: 02 December 2022.
Edited by:
Niya Zhou, Women and Children’s Hospital of Chongqing Medical University, ChinaCopyright © 2022 Chen, Zhao, Jones, Harper, de Seymour, Yang, Xia, Zhang, Qi, Gulliver, Cannon, Saffery, Zhang, Han and Baker. This is an open-access article distributed under the terms of the Creative Commons Attribution License (CC BY). The use, distribution or reproduction in other forums is permitted, provided the original author(s) and the copyright owner(s) are credited and that the original publication in this journal is cited, in accordance with accepted academic practice. No use, distribution or reproduction is permitted which does not comply with these terms.
*Correspondence: Hua Zhang, emgyODQ0QGdtYWlsLmNvbQ==; Ting-Li Han, dGluZ2xpaGFuQGNxbXUuZWR1LmNu
†These authors have contributed equally to this work and share first authorship