- 1Guilin Power Supply Bureau, China Southern Power Grid, Guilin, China
- 2Guilin Yangshuo Power Supply Bureau, China Southern Power Grid, Guilin, China
- 3Guilin Lingui Power Supply Bureau, China Southern Power Grid, Guilin, China
- 4School of Control Science and Engineering, Shandong University, Jinan, China
Fault detection in charging piles is crucial for the widespread adoption of electric vehicles and the reliability of charging infrastructure. Currently, due to the lack of sufficient fault data for charging piles, achieving stable and accurate fault identification is challenging. Moreover, distinctive fault features are key to accurate fault recognition. To address this, we designed a simulated charging pile system and collected fault data at multiple power levels by manually introducing faults. Furthermore, we proposed a fault identification algorithm based on spatiotemporal feature fusion using machine learning. This algorithm first collects fault data through a sliding window and utilizes Fourier transform to extract frequency domain information to construct temporal features. These features are then fused with spatial current amplitude information to form a distinctive feature set, enabling fault identification based on a machine learning model. Extensive experiments conducted on the constructed dataset show that this method can accurately identify charging pile faults. Compared with random forest and gradient boosted decision tree, the proposed method improves the macro-average score by 2.99% and 7.28%, respectively. We also explored the importance of each feature for fault identification results and the impact of window length on identification outcomes, demonstrating the necessity of the extracted features and the robustness of the proposed method to data resolution.
Highlights
• In order to deal with the problem of insufficient fault detection data of current charging piles, charging pile simulation systems of various capacity levels are constructed to obtain rich fault data.
• The spatiotemporal information of current data is considered to obtain key features associated with faults.
• A method for fault detection in charging based on spatiotemporal fusion of machine learning is proposed, which realizes accurate and stable non-invasive fault identification.
1 Introduction
With the growing global awareness of environmental protection and the increasing demand for sustainable transportation, the electric vehicle (EV) industry has been rapidly developing. According to a report by the International Energy Agency (IEA), global electric vehicle sales have significantly increased over the past decade, and it is expected that by 2030, the number of electric vehicles will reach 145 million (Global EV Outlook, 2021). In this context, the construction of EV charging infrastructure has become a crucial component in promoting the adoption of electric vehicles. As the core equipment for EV charging, the reliability and safety of charging piles are directly related to the user experience and the promotion of electric vehicles. However, the current development of charging piles faces challenges. On one hand, there is a shortage of infrastructure, with overall development lagging behind the growth of electric vehicle and plugin hybrid vehicle penetration rates. On the other hand, the failure rate of charging piles is relatively high, with complex fault types involving various factors such as electrical faults, semiconductor device failures, and capacitor faults (Xu et al., 2024), further exacerbating the shortage of charging resources. It is of great significance to improve the availability of charging piles to realize early warning and rapid identification of charging pile faults, thereby promoting rapid maintenance.
Traditional fault identification methods typically rely on the installation of wired sensors and regular manual inspections, which not only increase operational costs but also risk missing the optimal maintenance window due to untimely inspections. In contrast, non-intrusive fault identification technology, with its advantages of not interfering with the normal operation of equipment, easy installation, and low cost, has become a current research hotspot (Yousaf MZ. et al., 2023; Yousaf et al., 2022; Yousaf M. Z. et al., 2023). Non-intrusive fault identification technology mainly achieves early fault detection and diagnosis by monitoring the operational parameters of charging piles (such as voltage and current) (Xu et al., 2024) and using advanced signal processing and machine learning algorithms for data analysis and pattern recognition. This approach not only enables real-time monitoring of the charging pile’s working status but also provides detailed information on fault types and locations, helping maintenance personnel to quickly take corrective actions and reduce the impact of faults.
The main structure of a charging pile is the switched-mode power supply converter. In the early stages of charging pile fault research, fault detection was primarily carried out through physical modeling and feature matching analysis. These studies typically involve establishing an electrical model of the charging pile and predicting potential faults by building a fault feature library (Poon et al., 2017). Although this approach can provide some theoretical guidance, the actual operating environment is complex and variable, making it difficult to accurately reflect the real situation solely by relying on fault feature templates. In addition, such methods rely on preset failure modes and the physical characteristics of the system, lacking the ability to adapt to complex and unknown failure modes. Moreover, they are sensitive to real-time and environmental changes and have difficulty coping with the variability of different equipment and working conditions.
With the development of machine learning technology, researchers have begun to apply it to fault detection of charging piles (Gao et al., 2020; Dai et al., 2021; Wang et al., 2021; Yang et al., 2024). By collecting and analyzing the operation data of charging piles, machine learning models can adaptively learn fault features, thereby realizing the detection and prediction of charging pile faults. Common methods include support vector machines (SVM), decision trees, random forests, and artificial neural networks (Jiajia et al., 2019; Xu et al., 2019; Gao et al., 2020; Dui et al., 2023; Wang et al., 2023). Deng et al. (2022). collects arc data in a real-time digital simulation system and constructs a support vector machine model based on particle swarms to accurately identify arc faults in charging piles. Piao et al. (2023). decomposes and extracts features from fault data based on variational modal decomposition technology, and then uses support vector machine algorithms to detect open circuit faults in charging piles. Although these machine learning-based fault detection methods have improved accuracy, they face a high dependence on data quality and quantity. Insufficient training data or noisy data may lead to model overfitting or performance degradation. In addition, the model’s generalization ability is limited and may not be able to effectively adapt when encountering new fault types or system configurations, resulting in unstable detection results. In practice, obtaining sufficient fault data is often a significant challenge, especially when it comes to rare fault types.
In recent years, the rise of deep learning technology has provided new solutions for fault detection in charging piles. Deep learning models, particularly Convolutional Neural Networks (CNNs) (Gao et al., 2018) and Recurrent Neural Networks (RNNs) (Zhang et al., 2021), possess powerful capabilities in feature extraction and pattern recognition, allowing them to handle more complex data and tasks. A deep learning-based fault diagnosis method for DC charging piles was proposed in (Du et al., 2021), where the accuracy and effectiveness of the method were validated through the analysis of various fault types in the charging modules of DC charging piles, achieving an accuracy rate of over 95.56%. To address the issue of imbalanced fault data (Shen et al., 2021), proposed a fault data balancing correction algorithm based on Borderline-SMOTE, which improves the degradation of algorithms caused by differences in sample sizes. This method designed a LightGBM ensemble learning model that achieved high-precision fault diagnosis. In (Zhang et al., 2021), an improved RNN model was proposed to detect faulty conditions in charging piles. By modeling the relationship between the state parameters of the charging piles and the faulty conditions, the accuracy of fault detection was significantly enhanced. However, deep learning models also face the challenge of data scarcity. Without sufficient data, it is difficult to adequately train deep learning models, limiting their performance in practical applications. Additionally, although computationally intensive algorithms such as deep learning can adaptively extract fault features from data, the high hardware requirements and execution time constraints limit the speed of fault recognition. While machine learning models do not involve complex computations and offer a certain level of interpretability, the difficulty in obtaining distinctive features leads to lower fault recognition accuracy. Therefore, it is necessary to design fault features that are significantly correlated with fault characteristics.
To address the aforementioned challenges, we have designed a charging station simulation system. This system simulates charging station systems of various capacity levels and introduces different types of faults to generate sufficient fault data. Furthermore, we collect three-phase data within small time windows and extract temporal information and spatial information from the current through frequency domain transformation and key feature computation, respectively. Finally, efficient machine learning models are utilized to identify the type of fault under the current state. This approach effectively enhances the accuracy and reliability of fault detection in charging piles, ensuring the stable and safe operation of the charging station system.
2 Methods
2.1 Multi-level capacity charging station fault data simulation
Figure 1A shows the system structure of the charging station, which mainly consists of the power grid, transformer, and charging station. The internal structure of the charging station includes an AC/DC rectifier and a DC/DC converter. Figure 1B presents the topology of the converter. The AC/DC rectifier of the charging station uses a simple and reliable three-phase bridge rectifier circuit (Liserre et al., 2005; Safayatullah et al., 2022). To provide electrical isolation or extend the output voltage range, the DC/DC converter adopts an isolated Dual Active Bridge (DAB) converter (Zhao et al., 2014). The use of an isolated converter also greatly reduces the damage caused by faults. When a fault occurs on the secondary side of the isolated DC-DC converter, the presence of the isolation transformer does not affect the grid. In contrast, when a fault occurs on the primary side of the isolated DC-DC converter, the energy during the fault is supplied by the cascaded capacitor, and the grid current remains unchanged. The extended fault duration provides sufficient time for the circuit breaker to protect the circuit, thus minimizing the impact on the grid. Therefore, when a fault occurs in the bridge rectifier circuit directly connected to the grid, the impact on the grid is more severe and requires extra caution, along with adequate protective measures. This paper focuses on predictive studies of faults in bridge converter circuits.
A certain data set is required to make predictions, which is collected from the charging pile simulation system shown in Figure 1. The 600 VAC grid-side voltage is converted to 380 VAC three-phase AC voltage through a three-phase transformer. This is then rectified using a three-phase bridge rectifier circuit, converting the 380 V AC into 500 V DC. The collected data includes the three-phase voltage and three-phase current on the grid side. When different faults occur in the charging pile, the voltage and current on the grid side will change correspondingly, allowing for the identification of the fault type. To obtain data on faults occurring at different operational power levels in real scenarios, the experiments start at a power of 100 kW, increasing in steps of 5 kW, and ending at the rated power of 200 kW, resulting in a total of 21 sets of fault data at different power levels. Using Ohm’s Law, the current can be calculated from the power and voltage. For example, when the power is 100 kW, the DC side current is 200 A, and the RMS value of the AC side current is approximately 263 A.
Since the three-phase power of the grid is symmetrical, the impact of any fault of the same type in any phase on the grid is the same. Therefore, it is sufficient to collect and analyze fault data for phase a. Furthermore, in real scenarios, faults in the charging pile mainly arise from the damage to switches in the rectifier converter. At the same power level, the types of faults can be categorized into three types based on the condition of the damaged switches: Type A represents a short-circuit condition between two phases, where any two phases are directly connected due to overheating and failure of the switching devices. This failure may result from overcurrent or elevated environmental temperatures, leading to a short circuit between two AC bus phases. For instance, if the upper or lower bridge switches of phases a and b fail simultaneously, a short circuit will occur. Each phase, such as phase a, includes two switches: an upper bridge switch and a lower bridge switch. Type B indicates a situation where the upper bridge switch of phase a fails, while the rest of the external circuitry remains functional. Similarly, Type C describes a failure of the lower bridge switch in phase a, with all other external circuits operating normally. While Type B and Type C faults are more likely to occur, they can often escalate into Type A faults if not addressed promptly.
The waveforms of the three types of faults are shown in Figure 2. From the waveforms of the same fault under different power levels, it can be observed that power only affects the amplitude of the current before the fault but does not influence the current waveform after the fault. This is because the post-fault current is determined by the grid voltage and the parasitic resistance of the faulted line, which remain nearly constant across different power levels. As a result, the current waveform is essentially unchanged.
When a short circuit occurs between phases a and b of the grid, the grid current rises sharply, and the grid voltage is correspondingly affected and drops, causing significant impact on the grid, as shown in Figure 2A. Similarly, when a short circuit occurs between phase a and the positive or negative terminal of the DC side, the short-circuit current increases rapidly, also leading to severe impacts on the grid, as shown in Figures 2B, C.
As illustrated in Figure 2, when fault Type A occurs, the current in phase a increases in both forward and reverse directions. However, during faults Type B and Type C, the phase a current exhibits a larger forward or reverse magnitude, resembling the addition of a positive or negative DC offset. In practice, due to the mutual influence of the three-phase currents, a surge in one phase current unbalances the grid, causing increases in the currents of the other phases. Consequently, other switches may also be affected, potentially resulting in cascading faults that lead to more severe failures.
2.2 Spatiotemporal information fusion-based fault detection method
During the operation of electric vehicle charging stations, if faults are not detected and identified in a timely manner, and appropriate safety measures such as shutdowns are not implemented, it may lead to physical damage to the equipment, and even result in safety accidents, endangering users’ personal safety and causing economic losses. Therefore, promptly and quickly identifying faults in charging stations is a critical task of this research. To this end, we collect three-phase alternating current data from the charging station within a small time window, instead of voltage data, as voltage changes are less apparent during fault occurrences. Additionally, constructing fault features with significant discriminative power is another crucial task to ensure that the machine learning model can accurately identify faults. This not only prevents the failure to detect faults but also reduces false positives during normal operation, thereby lowering the maintenance burden.
To achieve fast and accurate fault detection in charging stations, we propose a fault detection method based on spatiotemporal information fusion. The architecture of the proposed fault identification scheme is shown in Figure 3. Since the impact of a fault on voltage data is weak, we only consider the fault features contained in the three-phase AC current. We use a sliding window to obtain input data to achieve real-time fault identification. Based on the input current, we use feature engineering to extract data spatial features and time series features represented by the frequency domain to reduce the difficulty of learning the classification model. For the fault modeling part, the decision tree model is employed to adaptively obtain the relationship between the extracted features and the fault, thereby realizing the fault identification function. The detailed fault identification process is as follows. For the three-phase current data of the charging station, we first extract the operating data within a short time frame using a sliding window. Current methods primarily focus on identifying amplitude characteristics of operating currents while neglecting the extraction of temporal features. Therefore, we use Fourier transform to extract the frequency data of each phase current as the temporal features of faults. Since the amplitude in the high-frequency range is relatively small, we retain only the low-frequency temporal features. Additionally, we calculate the mean value of each phase current as the spatial amplitude information of the fault. Finally, we process the above spatiotemporal features simultaneously using simple machine learning models, such as decision trees, to output the fault type.
To demonstrate the features in the frequency domain that are significantly related to fault types, we visualized the current frequency characteristics for different types of faults and the no-fault condition, as shown in Figure 4. Based on the frequency characteristics under various conditions, the current amplitude in each phase at low frequencies exhibits significant distinction across different states. However, the behavior in the high-frequency range is consistent across these states. Therefore, using low-frequency data as the temporal information for fault detection is a reasonable approach.
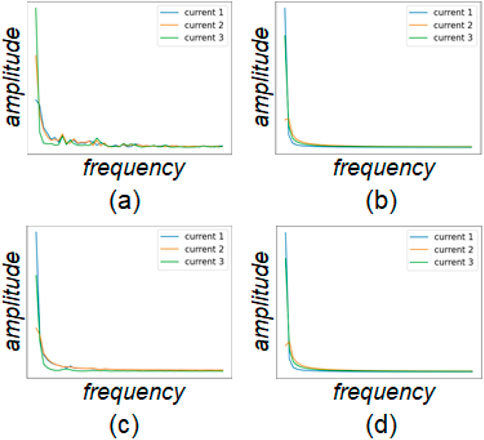
Figure 4. Current frequency characteristics under different faults and no-fault condition. (A) Fault free. (B) Fault A. (C) Fault B. (D) Fault C.
3 Experiments and analysis
3.1 Experiment description and metrics
Since the fault is introduced at 0.2 s, the input data window containing this time is labeled as fault data, while other data are labeled as no-fault data. The sampling rate is set to 15 kHz, and the output window length is set to 100. This study validates the effectiveness of the proposed method using the following baseline algorithms: Adaptive Boosting (AdaBoost, ABA), SVM, Decision Tree (DT), Multilayer Perceptron (MLP), Gradient Boosting Decision Tree (GBDT), and Random Forest. The number of base classifiers in the Random Forest is set to 5. The GBDT uses a learning rate of 0.1 and has 5 base classifiers. The Multilayer Perceptron uses ReLU as the activation function, with two hidden layers, each containing 64 neurons, and a maximum of 100 iterations. The ABA algorithm also has 5 base classifiers. All other parameters for the baseline methods are set to the default values in the sklearn library.
To objectively evaluate the effectiveness and superiority of the proposed method, this paper introduces the metrics of accuracy, recall, and macro-average F-score (F-macro). The definition of accuracy is given in Equation 1:
where, TP, TN, FN, and FP represent the number of true positives, true negatives, false negatives, and false positives, respectively. The definition of recall is as Equation 2:
The F-macro metric provides a comprehensive evaluation of the classification results under imbalanced sample conditions. It is defined as Equation 3:
C is the number of fault categories, and F1 is defined as Equation 4:
3.2 Fault detection results and analysis
The average ± standard deviation of the fault detection results over three trials for various baseline methods and the proposed spatiotemporal fusion method are reported in Table 1. Upon comprehensive analysis, the proposed method achieves a significant improvement in accuracy for fault detection in charging stations, with the exception of the ABA method. This improvement is evident not only in the mean values of various metrics but also in the reduction of variance, demonstrating the significant robustness of the proposed method. Specifically, accuracy improvements of 5.56%, 4.15%, 4.75%, 15.58%, and 2.73% were observed for RF, GBDT, SVM, MLP, and DT, respectively. For the decision tree algorithm, its performance is nearly identical to that of the random forest algorithm, which uses multiple base classification trees. Moreover, compared to other algorithms, except for the random forest, it achieved the best performance. Therefore, considering computational cost and real-time requirements, we selected the decision tree as the classifier for fault detection.
To analyze the fault detection algorithms’ performance on different fault types in detail, we visualized the detailed fault classification results using confusion matrices, as shown in Figure 5. According to the confusion matrices for the DT and MLP methods, both with and without the proposed method, the spatiotemporal fusion features improve the identification accuracy for each type of fault. However, various methods show confusion in recognizing Fault A and Fault C. This may be due to the similar characteristics exhibited by the rectifier phase a lower bridge arm short circuit and the grid-side phase a-b short circuit fault.
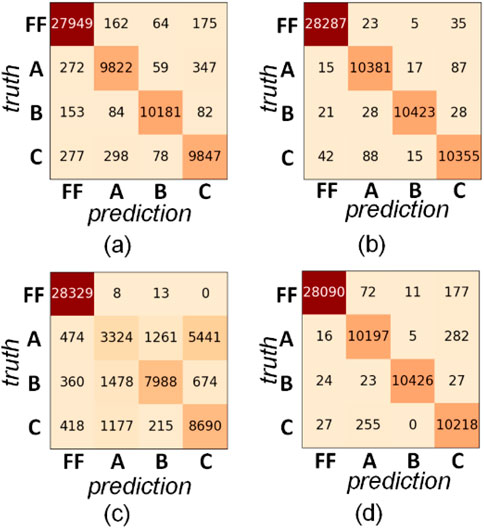
Figure 5. Confusion matrices for prediction results of decision tree and MLP methods. (A) DT without spatiotemporal fusion. (B) DT with spatiotemporal fusion. (C) MLP without spatiotemporal fusion. (D) MLP with spatiotemporal fusion.
We used the RF algorithm to output the fault detection results and the importance of various fault features to demonstrate the necessity of including frequency domain features. The importance of each feature is shown in Figure 6. Here, c1 to c3 represent the spatial features of the three-phase currents. c1_f1 to c1_f3, c2_f1 to c2_f3, and c3_f1 to c3_f3 represent the three lowest frequency values in the frequency domain for the three-phase currents. According to the importance results for each type of feature, there are strong correlations between the frequency domain features of each phase current and fault types. Additionally, the spatial current amplitudes also exhibit significant importance.
Due to variations in sensor and data processing equipment across different application scenarios, the input data resolution for models may vary. Therefore, fault detection methods need to be capable of handling different data input window lengths. We collected data with various input window lengths and used DT as a baseline model to validate the adaptability of the proposed method to different data window lengths. The experimental results are shown in Figure 7. Compared to the DT algorithm using only spatial features, the proposed spatiotemporal fusion features provide better adaptability to changes in data window length for the DT algorithm. However, as the window length increases, the performance of the fault detection model decreases, which is due to longer data windows containing more non-fault data within fault samples.
4 Conclusion
As a key infrastructure for electric vehicles, fault detection in charging stations is crucial for ensuring the safety and reliability of the charging process. This study addresses the issues of scarce fault data and the lack of significant fault features in existing algorithms by proposing a machine learning-based fault recognition algorithm that integrates spatiotemporal features. In order to deal with the problems of scarce fault data and poor recognition rate of fault detection algorithms in current research on charging pile fault detection, we first build a charging pile simulation system to collect rich fault data. By building systems of different capacity levels, we can obtain fault characteristics of different application scenarios, thereby increasing the generalization of the fault detection algorithm. Continuously, in order to obtain key features related to faults in the data, we propose a fault spatiotemporal feature fusion method. This method applies Fourier transform to extract frequency domain information, and integrates it with spatial current amplitude information. The acquired spatiotemporal fusion features can not only reduce the modeling difficulty of the fault identification model, but also enhance the robustness of the algorithm.
Experimental results on the collected dataset show that the proposed method significantly improves fault detection accuracy compared to baseline methods. Additionally, we investigated the importance of various features for fault output results, demonstrating the necessity of the extracted features. We also examined the impact of window length on detection results, validating the robustness of the proposed method to data resolution. In this study, we explored three typical fault types of charging piles. However, some potential hazards, such as device aging, cannot be effectively simulated to obtain training data. In addition, the characteristics of some hazards in three-phase AC current may not be obvious, and the recognition effect of the proposed method will be limited. Therefore, richer data types need to be introduced.
Data availability statement
The original contributions presented in the study are included in the article/supplementary material, further inquiries can be directed to the corresponding author.
Author contributions
YD: Resources, Software, Writing–original draft. SS: Validation, Visualization, Writing–review and editing. YZ: Resources, Software, Writing–review and editing. HM: Investigation, Methodology, Writing–review and editing. HW: Investigation, Methodology, Writing–review and editing. CH: Methodology, Project administration, Writing–original draft. HT: Conceptualization, Data curation, Formal Analysis, Writing–original draft.
Funding
The author(s) declare that financial support was received for the research, authorship, and/or publication of this article. This research was funded by Guangxi Power Grid Company Science and Technology Project, grant number GXKJXM20230163.
Conflict of interest
Authors YD, SS and YZ were employed by Guilin Power Supply Bureau, China Southern Power Grid. Authors HM and HW were employed by Guilin Yangshuo Power Supply Bureau, China Southern Power Grid. Author CH were employed by Guilin Lingui Power Supply Bureau, China Southern Power Grid.
The authors declare that this study received funding from Guangxi Power Grid Company Science and Technology Project. The funder had the following involvement in the study: writing, investigation, data collection and methodology.
Publisher’s note
All claims expressed in this article are solely those of the authors and do not necessarily represent those of their affiliated organizations, or those of the publisher, the editors and the reviewers. Any product that may be evaluated in this article, or claim that may be made by its manufacturer, is not guaranteed or endorsed by the publisher.
References
Dai, J., Zhu, L., Liu, Z., Zhao, Z., Ji, S., Li, X., et al. (2021). “Research on fault diagnosis method of power module for charging pile based on neural network,” in 22nd International Symposium on High Voltage Engineering (ISH 2021), Hybrid Conference, Xi’an, China, 21-26 November 2021 (Institution of Engineering and Technology), 1294–1299. doi:10.1049/icp.2022.0123
Deng, H., Zeng, W., Huang, R., Wu, Z., He, X., Liu, X., et al. (2022). A particle swarm optimization-based support vector machine model to detect charging pile arc faults by using the pre-treatment generalized S transform. J. Eng. 2022, 928–935. doi:10.1049/tje2.12175
Du, J., An, W., Zhou, M., Mao, W., Huang, G., and Deng, S. (2021). “Research on fault diagnosis method of DC charging pile based on deep learning,” in 2021 11th international conference on power and energy systems (ICPES) (Shanghai, China: IEEE), 426–431. doi:10.1109/ICPES53652.2021.9683841
Dui, H., Dong, X., Chen, L., and Wang, Y. (2023). IoT-enabled fault prediction and maintenance for smart charging piles. IEEE Internet Things J. 10, 21061–21075. doi:10.1109/JIOT.2023.3285206
Gao, D.-X., Hou, J.-J., Liang, K., and Yang, Q. (2018). “Fault diagnosis system for electric vehicle charging devices based on Fault tree analysis,” in 2018 37th Chinese Control Conference (CCC), (Wuhan: IEEE), Wuhan, China, 25-27 July 2018, 5055–5059. doi:10.23919/ChiCC.2018.8482691
Gao, X., Yuan, G., and Zhang, M. (2020). “Fault detection of electric vehicle charging piles based on extreme learning machine algorithm,” in 2020 Fourth International Conference on Computing Methodologies and Communication (ICCMC), Erode, India, 11-13 March 2020 (IEEE), 849–852. doi:10.1109/ICCMC48092.2020.ICCMC-000157
Jiajia, W., Xingying, C., and Li, J. (2019). Research on fault diagnosis of DC charging pile power device based on wavelet packet and elman neural network. IOP Conf. Ser. Mater. Sci. Eng. 486, 012086. doi:10.1088/1757-899X/486/1/012086
Liserre, M., Blaabjerg, F., and Hansen, S. (2005). Design and control of an LCL-filter-based three-phase active rectifier. IEEE Trans. Industry Appl. 41 (5), 1281–1291. doi:10.1109/TIA.2005.853373
Piao, J., Li, H., Zhou, Y., Feng, D., and Zhao, X. (2023). “VMD-LSSA-SVM based open-circuit fault diagnosis method for electric vehicle DC charging pile,” in Proceedings of the 2023 7th international conference on electronic information technology and computer engineering, (xiamen China: acm), 79–85. doi:10.1145/3650400.3650414
Poon, J., Jain, P., Konstantakopoulos, I. C., Spanos, C., Panda, S. K., and Sanders, S. R. (2017). Model-based fault detection and identification for switching power converters. IEEE Trans. Power Electron. 32, 1419–1430. doi:10.1109/TPEL.2016.2541342
Safayatullah, M., Elrais, M. T., Ghosh, S., Rezaii, R., and Batarseh, I. (2022). A comprehensive review of power converter topologies and control methods for electric vehicle fast charging applications. IEEE Access 10, 40753–40793. doi:10.1109/ACCESS.2022.3166935
Shen, W., Fan, W., and Chen, C. (2021). “An electric vehicle charging pile fault diagnosis system using Borderline-SMOTE and LightGBM,” in Tenth international symposium on precision mechanical measurements. Editors L. Yu, L. X. Yang, and H. Xia (Qingdao, China: SPIE), 125. doi:10.1117/12.2617310
Wang, Q., Lu, X., and Yang, D. (2021). Fault diagnosis of DC-DC module of V2G charging pile based on Fuzzy Neural Network. IOP Conf. Ser. Earth Environ. Sci. 772, 012027. doi:10.1088/1755-1315/772/1/012027
Wang, W., Wang, J., Peng, X., Yang, Y., Xiao, C., Yang, S., et al. (2023). Exploring best-matched embedding model and classifier for charging-pile fault diagnosis. Cybersecurity 6, 7. doi:10.1186/s42400-023-00138-z
Xu, M., Long, X., and Zhou, W. (2019). “Research on new energy grid connection and electric vehicle charging fault resolution method,” in 2019 IEEE International Conferences on Ubiquitous Computing and Communications (IUCC) and Data Science and Computational Intelligence (DSCI) and Smart Computing, Shenyang, China, 21-23 October 2019 (IEEE), 752–755. doi:10.1109/IUCC/DSCI/SmartCNS.2019.00154
Xu, Y., Zou, Z., Liu, Y., Zeng, Z., Zhou, S., and Jin, T. (2024). Deep learning-based multi-feature fusion model for accurate open circuit fault diagnosis in electric vehicle DC charging piles. IEEE Trans. Transp. Electrific, 1. doi:10.1109/TTE.2024.3418866
Yang, F., Bao, J., Chen, L., Zhao, Y., and Xu, K. (2024). “Charging pile fault diagnosis method based on BOA-SSA-BP neural network,” in 2024 IEEE/IAS 60th Industrial and Commercial Power Systems Technical Conference (I& CPS), Las Vegas, NV, USA, 19-23 May 2024, 1–6. doi:10.1109/ICPS60943.2024.10563312
Yousaf, M. Z., Khalid, S., Tahir, M. F., Tzes, A., and Raza, A. (2023a). A novel dc fault protection scheme based on intelligent network for meshed dc grids. Int. J. Electr. Power and Energy Syst. 154, 109423. doi:10.1016/j.ijepes.2023.109423
Yousaf, M. Z., Liu, H., Raza, A., and Mustafa, A. (2023b). Deep learning-based robust DC fault protection scheme for meshed HVDC grids. CSEE J. Power Energy Syst. 9 (6), 2423–2434. doi:10.17775/CSEEJPES.2021.03550
Yousaf, M. Z., Tahir, M. F., Raza, A., Khan, M. A., and Badshah, F. (2022). Intelligent sensors for dc fault location scheme based on optimized intelligent architecture for HVdc systems. Sensors 22 (24), 9936. doi:10.3390/s22249936
Zhang, J., Li, D., Xie, Q., Liu, W., and Liang, B. (2021). “Real-time fault monitoring method of charging pile based on improved RNN neural network,” in 2021 International Conference on Wireless Communications and Smart Grid (ICWCSG), Hangzhou, China, 13-15 August 2021 (IEEE), 536–539. doi:10.1109/ICWCSG53609.2021.00114
Keywords: charging pile, fault identification, machine learning, spatiotemporal information fusion, fault detection
Citation: Duan Y, Shu S, Zhao Y, Mo H, Wu H, Hou C and Tian H (2024) Machine learning-based spatiotemporal fusion method for non-intrusive charging pile fault identification. Front. Electron. 5:1490939. doi: 10.3389/felec.2024.1490939
Received: 04 September 2024; Accepted: 02 December 2024;
Published: 20 December 2024.
Edited by:
Fanfan Lin, The Zhejiang University-University of Illinois at Urbana-Champaign Institute, United StatesReviewed by:
Muhammad Zain Yousaf, Hubei University of Automotive Technology, ChinaMohamed Ali Zdiri, Zhejiang University, China
Copyright © 2024 Duan, Shu, Zhao, Mo, Wu, Hou and Tian. This is an open-access article distributed under the terms of the Creative Commons Attribution License (CC BY). The use, distribution or reproduction in other forums is permitted, provided the original author(s) and the copyright owner(s) are credited and that the original publication in this journal is cited, in accordance with accepted academic practice. No use, distribution or reproduction is permitted which does not comply with these terms.
*Correspondence: Hao Tian, aGFvdGlhbkBlbWFpbC5zZHUuZWR1LmNu