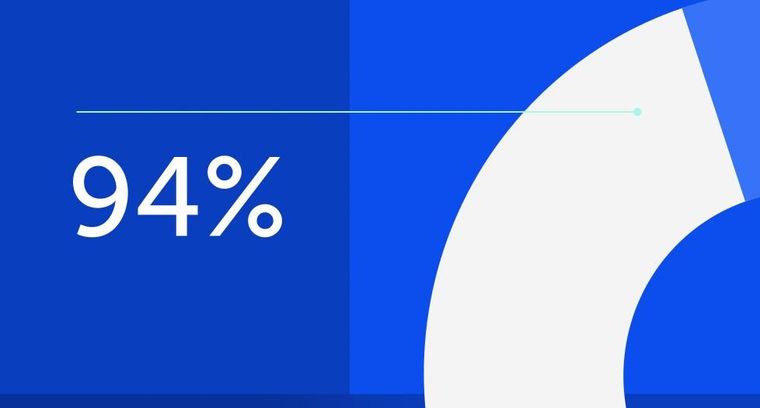
94% of researchers rate our articles as excellent or good
Learn more about the work of our research integrity team to safeguard the quality of each article we publish.
Find out more
ORIGINAL RESEARCH article
Front. Educ.
Sec. Leadership in Education
Volume 10 - 2025 | doi: 10.3389/feduc.2025.1580683
The final, formatted version of the article will be published soon.
Select one of your emails
You have multiple emails registered with Frontiers:
Notify me on publication
Please enter your email address:
If you already have an account, please login
You don't have a Frontiers account ? You can register here
School principals encounter contemporary demands that impact their job satisfaction and leadership effectiveness. Despite the significance of this issue, there is limited research on satisfaction predictors for these professionals, particularly using machine learning approaches.This study identified key predictors of job satisfaction among Peruvian school principals by applying an ensemble of feature selection methods and evaluating five machine learning algorithms (Random Forest, Decision Trees-CART, Histogram-Based Gradient Boosting, XGBoost, and LightGBM) with data from the 2018 National Survey of Directors. The principal variables identified included satisfaction with salary, geographic location of the educational institution, relationships with students and teachers, workplace climate, student learning achievements, and job benefits. Economic factors proved important, such as gross and net income, and the minimum monthly amount required to meet household needs. Timerelated aspects also exerted influence, including hours dedicated to training, time spent on administrative and/or teaching duties outside working hours, travel time to and from the Local Educational Management Unit (UGEL), duration of stays at the UGEL, and commuting time from principal residence to the educational institution. The Histogram-Based Gradient Boosting algorithm, optimized with Bayesian techniques and trained with data balanced through Random Oversampling, achieved a balanced accuracy of 0.63 on a test set with real-world class distribution. 1 Holgado-Apaza et al.When using Generative Adversarial Networks to balance only the training set, better results were obtained in recall (0.74), precision (0.72), and F1 score (0.70). SHAP analysis revealed that economic factors primarily influenced dissatisfied principals, while interpersonal factors were more important for highly satisfied principals, suggesting a hierarchical pattern of needs. The findings could inform strategies to enhance principals' job satisfaction and strengthen data-driven educational policies.
Keywords: Educational Leadership, Feature selection ensemble, Job Satisfaction, machine learning, Peruvian education, school principals
Received: 25 Feb 2025; Accepted: 17 Apr 2025.
Copyright: © 2025 Holgado-Apaza, Isuiza-Perez, ULLOA GALLARDO, Vilchez-Navarro, Aragon-Navarrete, Quispe Layme, Castellon-Apaza, Choquejahua-Acero and Prieto-Luna. This is an open-access article distributed under the terms of the Creative Commons Attribution License (CC BY). The use, distribution or reproduction in other forums is permitted, provided the original author(s) or licensor are credited and that the original publication in this journal is cited, in accordance with accepted academic practice. No use, distribution or reproduction is permitted which does not comply with these terms.
* Correspondence: Luis Alberto Holgado-Apaza, Departamento Académico de Ingeniería de Sistemas e Informática, Universidad Nacional Amazónica de Madre de Dios, Puerto Maldonado 17001, Peru, Puerto Maldonado, Peru
Disclaimer: All claims expressed in this article are solely those of the authors and do not necessarily represent those of their affiliated organizations, or those of the publisher, the editors and the reviewers. Any product that may be evaluated in this article or claim that may be made by its manufacturer is not guaranteed or endorsed by the publisher.
Supplementary Material
Research integrity at Frontiers
Learn more about the work of our research integrity team to safeguard the quality of each article we publish.