- 1North Eastern Mindanao State University, Tandag, Philippines
- 2Cebu Technological University, Cebu City, Philippines
STEM fields—Science, Technology, Engineering, and Mathematics—play crucial roles in advancing knowledge, driving innovation, and addressing challenges by means of several mechanisms including research. Consequently, STEM curricula in higher education institutions prepare undergraduate students taking these fields to engage and produce quality research outputs in preparation for their future careers or roles. The advent of several educational resources help these students to perform research-related tasks including artificial intelligence. Although AI use is viewed as inappropriate in doing scholarly works due to concerns about academic integrity and the fear of losing essential cognitive skills, the growing AI dependence among STEM undergraduate students is inevitable. In this regard, the present study seeks to empirically investigate the influence AI dependence toward students’ research productivity, and the mediating roles of research skills, disposition, and self-efficacy. Through literature review, a structural model was proposed and validated. Initially, a research instrument was developed reflective of the constructs present in the structural model where items were also generated using literature review. Eventually, an online survey was conducted and recorded 834 valid responses from STEM undergraduate students. Results revealed that from seven hypotheses proposed in the structural model, six are supported except the causal path between AI dependence and research productivity. The paths between AI dependence to research skills, dispositions, and self-efficacy are supported as well as the paths between these three to research productivity. This indicates the mediation of research skills, dispositions, and self-efficacy between the causal path linking AI dependence to research productivity. The findings of this study imply that strategic integration of AI resources may foster not only skills development but also research motivation and confidence, which together could enhance students’ overall research productivity in STEM fields.
Introduction
Education systems worldwide have increasingly prioritized enhancing Science, Technology, Engineering, and Mathematics (STEM) education to cultivate future-ready skills (Cortes et al., 2023). In the Philippines, the STEM curriculum prepares students for higher education and careers in science and technology while developing problem-solving skills for local and global challenges (Estonanto, 2017). However, inadequate primary science curricula and insufficient teacher preparation hinder student performance (Bernardo, 2004). In response to this, curriculum reforms have been proposed (Montebon, 2014), with strengths in the STEM track showing Philippine standards comparable to those in Japan and the United States (Orale and Sarmiento, 2016). This is to prepare Filipino graduates for competitive careers abroad and aligns with national industry needs. In doing so, one of the preparations is they must engage in research activities to cultivate critical thinking and problem-solving skills (Cabansag, 2014).
In this regard, research productivity among students, specifically in the STEM areas, has increasingly drawn attention in the context of higher education in the Philippines. However, studies show that students’ researches are often limited as a result of limited resources, inadequate training support, and lack of exposure and training. For instance, a study highlighted that a number of undergraduate students encounter difficulties with research endeavors due to time constraints and inadequate access to current academic literature and publications, which eventually hinders their research productivity (Rogayan et al., 2021). This scenario is not isolated as various studies have also shown the suboptimal status of students’ research productivity which is evident based on the reports made by other institutions on students’ low levels of engagement in research activities (Ricardo and Ricardo, 2023; Heng et al., 2020; Bai et al., 2013). In light of these challenges, institutions explore and integrate various resources and innovations including providing electronic databases, interactive educational frameworks, and digital tools, and platforms to improve research productivity. These tools encourage peer support and facilitate information access to foster a productive research culture among STEM undergraduates (Mustafakulov, 2023).
Nowadays, Artificial Intelligence (AI) has also been increasingly recognized as one of the resources in doing research and a revolutionary force in academia which reshapes educational and research practices. It serves as a notable digital tool for STEM undergraduate students in their studies. Overtime, students maximize the use of AIs for research endeavors leading to AI dependence. The latter refers to the reliance on AI technologies for tasks, decisions, and validation in research contexts for emotional reliance, minimizing performance anxiety, and external validation (Seo et al., 2021). AI tools like ChatGPT, Jenni, Co-pilot, citation managers like Zotero, data analysis software like SPSS, and collaborative platforms like Google Docs, have been increasingly adopted by students to enhance their research productivity. While these tools improve efficiency in data processing and access to information, they also present drawbacks, such as a decline in critical thinking and independent research skills and feeling of insecurity when not using these technologies as reported by several authors (e.g., Ahmad et al., 2023; Necula, 2024). Moreover, some educators and students view AI use as inappropriate in doing scholarly works due to concerns about academic integrity and the fear of losing essential cognitive skills (Lin and Chen, 2024).
Despite the aforementioned drawbacks of AIs, there is a growing dependence toward these resources among students. Hence, it is timely to examine how AI tools influence research productivity and even affect their research skills, disposition, and self-efficacy to guide policies and practice, and contribute to the growing literature concerning the importance of AIs to research. In particular, this study aims to (1) develop a conceptual model demonstrating how AI dependence contributes to the STEM undergraduate students’ research skills, self-efficacy, disposition, and ultimately productivity; and, (2) validate the conceptual model with empirical data using Structural Equation Modeling (SEM). The aim is to offer theoretical contribution and practical insights among higher education institutions in advancing understanding of how AI dependence influences research productivity. The knowledge gained from this work can also help create an enabling environment for undergraduate STEM student researchers who will be proactively engaged in conducting scholarly works, and minimize reluctance and stigma among students to use AIs in research if proven beneficial.
Literature review and hypothesis development
AI dependence as antecedent of research skills, disposition, and self-efficacy
AI Dependence can be conceptualized as a propensity or need to overly rely on automated systems for decisions, tasks, or validation (Zhai et al., 2024; Seo et al., 2021). While AI tools can streamline research tasks (e.g., data processing and literature review), there is a concern about over-reliance because it may erode traditional research skills, such as critical thinking and manual data evaluation (Wagner et al., 2021). Nevertheless, AIs increasing role in research is evident prompting significant examination of its implications on research skills development. As evidence, several studies pointed out that reliance on AI could develop research skills such as managing catalogs, descriptor books, and bibliographic records, and improving efficiency of information retrieval and metadata generation (e.g., UNESCO, 2023; Lund and Wang, 2023; Lund et al., 2024; Andersdotter, 2023). Wagner et al. (2021) further noted that AI-driven systems can help analyze previous research findings and suggest potential avenues for exploration, thereby helping researchers to develop skill in hypothesis development. AIs also allow predictive modeling and statistical analysis, thus, providing researchers with additional skills, particularly on deepening their insights and formulating research conclusions (Ahmad et al., 2023). Thus, the following hypothesis is proposed:
H1: STEM undergraduate students’ AI dependence positively and significantly influences their research skills.
Studies also show that reliance toward AI fosters greater student engagement and alleviates initial anxieties around research by enhancing students’ sense of accomplishment and encouraging active participation in research tasks (Nguyen et al., 2024; Fosner, 2024). As a result, this could lead to a positive disposition toward research (Damianus et al., 2019). It is defined as an individual’s unique combination of tendencies to act and is influenced by personal attitudes and environmental factors, both of which shape behaviors in research. In the same manner, Hemmings and Kaya (2015) found that the support for writing tasks, such as AI tools result to substantial impact on students’ positive behavior and disposition toward research. In line with this, it is posited that:
H2: STEM undergraduate students’ AI dependence positively and significantly influences their research disposition.
Although it may undermine critical thinking and cognitive skills, its application in data analysis, decision-making, and information retrieval promotes efficiency and offers potential benefits in enhancing learning experiences. These AI applications could eventually contribute in developing research self-efficacy (Mah and Groß, 2024). It is as an individual’s beliefs in his or her ability to successfully engage in research-related tasks (Livinți and Iliescu, 2021). Students who believe in their research abilities are more likely to embrace challenges and persist through difficulties, meaning high levels of self-efficacy encourage students and researchers to set ambitious goals and take ownership of their projects, fostering innovation and discovery (Bejtic, 2024). Research self-efficacy, therefore, develops competent and confident researchers as it empowers them to navigate the complexities of academic inquiry effectively. Hence, the hypothesis below is proposed:
H3: STEM undergraduate students’ AI dependence positively and significantly influences their research self-efficacy.
Research skills, disposition, and self-efficacy as antecedents of research productivity
Research skills are essential for academic success and significantly impact research productivity in higher education (Vieno et al., 2022). These skills, defined as a set of teachable and actionable tasks encompassing the establishment of facts, hypothesis formation, data collection, and analysis (Laidlaw et al., 2012), have been shown to influence positively with research productivity (Tahsildar and Hasani, 2021). For instance, Patricio (2022) found that research writing and collaborative skills significantly impacted the quality of research output among students, linking skill improvement with higher output quality. Conversely, moderate skill levels may hinder productivity (Tahsildar and Hasani, 2021). Therefore, addressing challenges faced by academics, including time constraints and perceived deficiencies in research skills (Alvarez-Ochoa et al., 2022), can lead to improved research productivity. This finding reinforces the notion that research skills especially in data analysis, academic writing, and collaboration are crucial for producing high-quality research. Therefore, this study proposes that:
H4: STEM undergraduate students’ research skills positively and significantly influence their research productivity.
Research disposition is another potential predictor of research productivity. It refers to individual’s attitude, confidence, and motivation toward engaging in research activities, which includes their interest, enjoyment, and belief in their ability to succeed in research tasks (Papanastasiou, 2005). This disposition emerges from the dynamic interaction between an individual and their environment, where personal factors such as satisfaction with research and the importance placed on it shape one aspect of the relationship. Meanwhile, environmental influences, like the availability of time due to academic demands, highlight the external component of this interaction (Hemmings and Kaya, 2015). Empirical studies affirm that students who exhibit positive research dispositions are more likely to meet research-related deadlines, maintain quality in their research output, and manage time effectively to balance research with other academic demands (e.g., Atieno et al., 2021; Albert et al., 2018). This relationship emphasizes the value of nurturing intrinsic motivation and a positive disposition toward research, as these attitudes drive consistent effort in data gathering, analysis, and task completion, which are essential for research productivity and overall academic success (Prado, 2019; Vereijken et al., 2018; Whipple et al., 2015). Thus, it is posited that:
H5: STEM undergraduate students’ research disposition positively and significantly influences their research productivity.
Research self-efficacy—the belief in one’s ability to effectively perform research-related tasks—plays a crucial role in motivating engagement and persistence in research activities (Gaoat, 2022; Forester et al., 2004). It influences why researchers undertake specific tasks and the level of effort they invest when confronted with challenges, as high self-efficacy encourages them to set ambitious goals and take ownership of their projects, thereby fostering innovation and discovery (Bejtic, 2024). It is particularly relevant in academic settings as it significantly influences with research productivity. Students with strong research self-efficacy are often more productive, emphasizing how confidence in research capabilities is a significant predictor of research engagement and output (Pasupathy and Siwatu, 2014). Empirical research further supports a direct relationship between research self-efficacy and expected research outcomes, as evidenced by Bieschke (2006), who found that higher research self-efficacy leads to greater expectations of success. This underscores how self-efficacy shapes researchers’ attitudes toward anticipated outcomes. In view of this, it is hypothesized that:
H6: STEM undergraduate students’ research self-efficacy positively and significantly influences their research productivity.
AI dependence and research productivity
While over-relying on AIs could risk diminishing critical research skills, the use of AI in research is increasingly recognized for its ability to improve research productivity and ease workloads (Dwivedi et al., 2021). AI tools reduce drafting time and enhance writers’ confidence, making it easier for researchers to stay engaged in their work (Bouzar et al., 2024). Bonsay et al. (2021) also explain that AIs’ integration in academic settings can help overcome typical research productivity hurdles by automating time-consuming or complex tasks. AI tools have the potential to reduce the time required in composing academic manuscripts, while allowing writers to focus more on developing deeper and more substantial ideas and arguments (Baidoo-Anu and Ansah, 2023; Santos and Santos, 2015). This has accelerated the writing process, reduced workload, and enhanced efficiency in various fields, including academic writing (Zhao et al., 2024; Yang and Bai, 2020). AIs free academics from repetitive processes, empowering them to produce higher-quality work and share knowledge more broadly, and ensuring research productivity. Thus, it is posited that:
H7: STEM undergraduate students’ AI dependence positively and significantly influences research productivity.
In summary, the research hypotheses are illustrated into a conceptual framework as presented in Figure 1.
Research methodology
Data and sample
This study was conducted to explore the influence of AI dependence on research skills, self-efficacy, dispositions, and productivity among undergraduate students in STEM fields. This study utilized mixed-methods sequential exploratory design to develop and validate the proposed structural model. SEM studies use a mixed-methods sequential exploratory design to ensure constructs are theoretically grounded and empirically validated. The qualitative phase helps identify key constructs and develop measurement items, which can be done through interviews, focus groups, or literature reviews. These are then refined through confirmatory factor analysis in the quantitative phase. This approach strengthens the measurement model’s validity and reliability before testing causal relationships in SEM. In the present study, literature review was initially conducted and served as the qualitative phase to identify key constructs and generate hypotheses. These qualitative data are presented in the literature review section and were subsequently used and combined to propose the hypotheses and a conceptual framework or structural model (see Figure 1). To validate the structural model quantitatively, a survey was administered among STEM undergraduate students enrolled in a higher education institution in the Philippines. The survey was done following convenience sampling technique because of its practicality and cost-effectiveness. To recruit participants, a survey link was shared on university social media groups, STEM forums, and institutional platforms. The researchers also collaborated with faculty members and student organizations for wider distribution. The participants were ensured to meet the inclusion criteria by using screening questions and obtaining informed consent before they proceeded answering. Additionally, participants were encouraged to share the survey with eligible peers. The inclusion criteria include the following: (a) students agreed that their responses be used for research and publication purposes, (b) currently enrolled in any STEM undergraduate degree program, (c) conducted any research project, and (d) utilized AI tools for research. Table 1 shows the distribution of students when grouped according to demographic and academic profile. The table presents the demographic distribution of 834 undergraduate STEM students by age, sex, year level, and degree program. Most students are aged 18–22, with 18-year-olds being the largest group (24.58%), while 7.79% are 24 and above. Males slightly outnumber females (52.88 vs. 47.12%). First-year students make up the largest cohort (44.48%). Information Technology (28.54%), Industrial Technology (24.82%), and Computer Science (24.34%) make the most respondents, while Mathematics Education and Technology and Livelihood Education have the fewest respondents. Meanwhile, Table 2 presents the distribution of students according to the AIs they used, where more than half reported to have used ChatGPT.
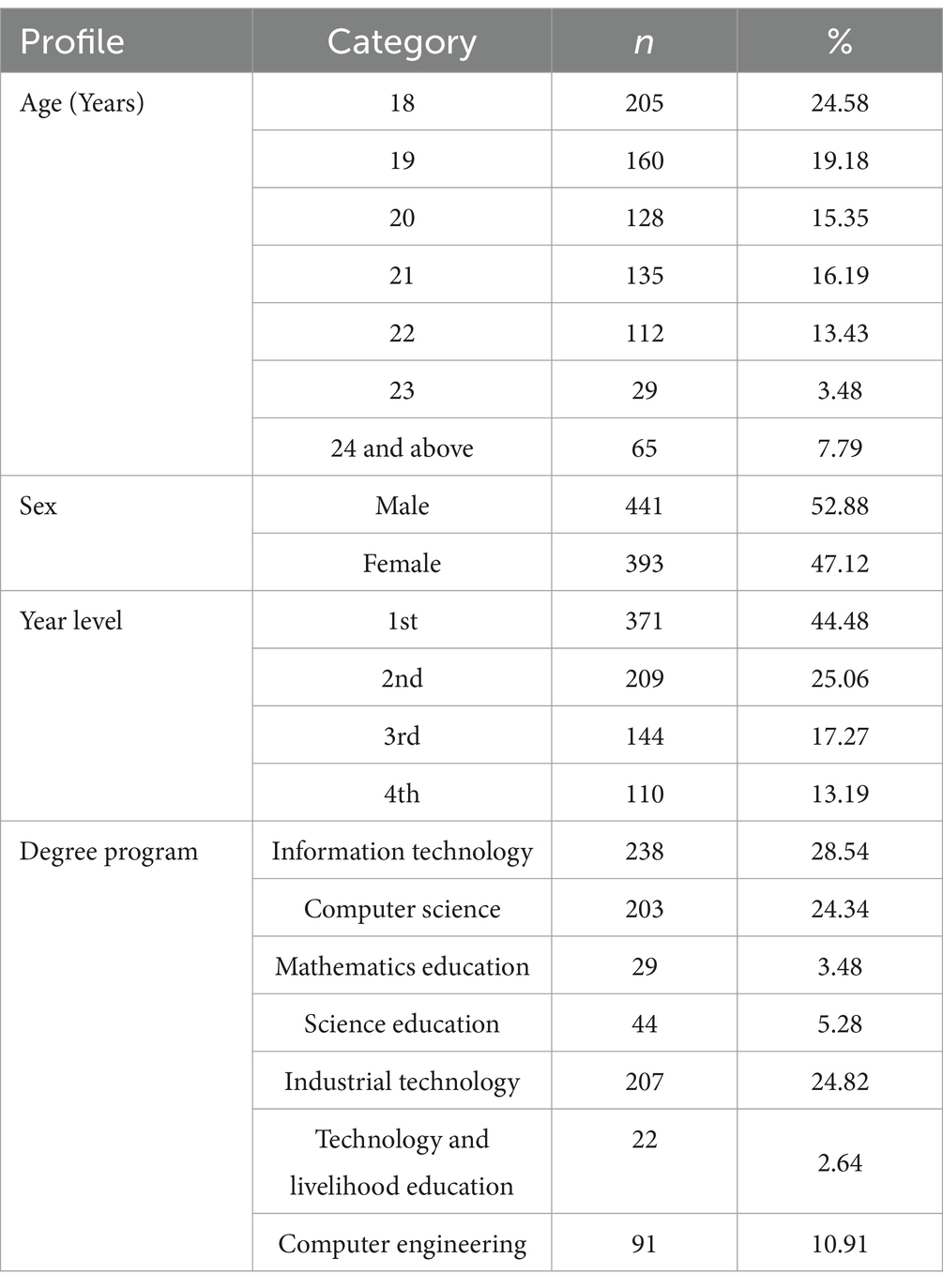
Table 1. Distribution of undergraduate STEM students when grouped according to age, sex, year level, and degree program enrolled (n = 834).
Research instruments
The instrument consists of three parts. The first part indicates the informed consent form which states the background, possible risks, discomforts, confidentiality and benefits of the study. The statements included in this section were subject for review and approval of the students. The second part inquired about their demographic and academic profiles, including sex, year level, STEM course or undergraduate degree enrolled, and age. Moreover, this part asked the students whether they were required to do research or not. This serves as a preliminary filter question, whereby those who responded “Yes” were considered as eligible participants. They were also asked to identify the type of AI they used. The third and final part assessed STEM undergraduate students’ perceived levels of AI dependence, research skills, research disposition, research self-efficacy, and research productivity. These constructs were operationally defined through a literature review to generate the items. Table 3 presents the five constructs of the instrument along with their corresponding items and sources. The constructs vary in the number of items assigned, ranging from four items for research disposition and research productivity to eight items for research skills. All items were evaluated using a five-point Likert scale, where 1 represents “strongly disagree” and 5 represents “strongly agree.”
Data gathering procedure
The researchers distributed the survey questionnaire through Google Form, with access provided through a link shared from August until October 2024, across multiple communication platforms, including email and messaging services. Prior to sharing the link, the researchers adhered to the ethical standards outlined by the University Research and Development Office, ensuring that all procedures before, during, and after data collection met ethical guidelines. Specifically, participants received detailed information about the study and were asked to review and sign a consent section, which stated: “I have read this form and decided to participate in the study as described above. Its general purposes, involvement specifics, possible risks, and benefits have been satisfactorily explained. I understand I may withdraw at any time and have received a copy of this form.” Only students who provided signed consent received the Google Form link. Furthermore, data gathered through the survey were handled with strict confidentiality and utilized solely for research and publication purposes. The collected data were then subjected to statistical treatment.
Data analysis
This study employed SPSS version 26 and AMOS 26.0 for data analysis. Descriptive statistics were utilized to summarize categorical data obtained from the survey, while Confirmatory Factor Analysis (CFA) was conducted to validate the measurement model and eventually establish its construct validity, internal consistency and dimensionality. Fornell-Larcker criterion served as reference to provide evidence of its discriminant validity. Lastly, Structural Equation Modeling (SEM) was performed to evaluate the structural model and test the hypothesized relationships.
Results
Measurement model assessment
The CFA was conducted using the maximum likelihood method, which is chosen for its asymptomatic efficiency in studies with large sample sizes (Tarima and Flournoy, 2019). Initially, the t-values and Standardized Factor Loading (SFL) for each item in the scale were evaluated to support the analysis of the model’s overall data fit. The observed t-values ranged from 19.410 (AID4) to 36.480 (RSE4), and the SFLs ranged from 0.688 (RS1) to 0.895 (RD2) (see Figure 2 and Table 4). According to the recommendations by Hair et al. (2009) and Kline (2016), t-values should reach a cut-off value of ≥1.96, and SFLs should reach ≤0.5, hence, all these items were retained. Subsequently, the five Goodness of Fit Indices (GFIs) were examined to evaluate the overall measurement model fit. These are shown in Table 5 alongside the proposed acceptable threshold values as suggested by Hair et al. (2009) and the actual values derived from the analysis. Notably, all five fit indices indicate an acceptable model fit after correlating items with high covariance values.
The convergent validity and internal consistency of the measurement model were eventually examined and presented in Table 4 using SFLs and composite reliability (CR), respectively. Gefen et al. (2000) and Hair et al. (2009) recommended that the minimum threshold value for both SFL and CR is ≥0.5. Both psychometric properties are established. As evidence, no SFL and CR have values lower than the proposed threshold. Finally, discriminant validity was assessed using the Fornell-Larcker criterion, which requires that the square root of the average variance extracted (√AVE) for a construct be higher than the construct’s correlation with any other construct. This ensures that the constructs are unique from each other. Table 6 shows the results of discriminant validity analysis, where √AVE for each of the five constructs consistently exceeds the correlation coefficients where it is correlated.
Structural model assessment
Structural model and hypotheses testing was conducted using SEM to assess the validity of the proposed structural model. As shown in Table 6 and Figure 3, six hypotheses were supported. Specifically, Al dependence positively and significantly influenced research skills (βH1 = 0.644, t = 14.639, p = 0.000 < 0.001), research disposition (βH2 = 0.646, t = 15.193, p = 0.000 < 0.001), and research self-efficacy (βH3 = 0.670, t = 15.563, p = 0.000 < 0.001), explaining 64, 65 and 67%, respectively, of their variance. Additionally, research skills (βH4 = 0.233, t = 7.238, p = 0.000 < 0.001), research disposition (βH5 = 0.166, t = 5.259, p = 0.000 < 0.001), and research self-efficacy (βH6 = 0.709, t = 17.518, p = 0.000 < 0.001) positively and significantly influenced research productivity, collectively explaining 80% of its variance. However, AI dependence (βH7, = −0.044, t = −0.895, p = 0.371 > 0.001) did not show a significant influence on research productivity. This means that research skills, research disposition, and research self-efficacy mediate the relationship between Al dependence and research productivity. Figure 4 presents the final structural model with causal link between AI dependence and research productivity removed (Table 7).
Discussion
Given the growing dependence of STEM undergraduate students in AI tools as resources to conducting research, the present study seeks to determine how use of these tools shapes their research skills, dispositions, self-efficacy, and ultimately productivity. The proposed structural model is anchored from existing literature emphasizing how AIs contribute to research. The limited insights in the domain literature calls for an empirical validation of the proposed hypotheses in the structural model to guide policy and practice, and contribute a theory regarding AI dependence and research.
The results revealed that AI Dependence positively and significantly influence research skills (H1), thus, supporting previous research findings (e.g., UNESCO, 2023; Lund and Wang, 2023; Lund et al., 2024; Andersdotter, 2023). This means the students who rely on AI tools enhances their research skills across multiple stages such as formulating scientific problems and hypotheses, performing literature review, analyzing and interpreting data, writing, and referencing. This further explains that STEM undergraduate students’ AIs dependence enable them to quickly summarize relevant studies and highlight critical findings, thereby building a solid foundation for their hypotheses. With AIs, students conveniently do literature review by automating searches, screening relevant studies, summarizing key findings, and identifying research gaps, thereby improving efficiency and allowing researchers to focus on critical analysis and synthesis. Furthermore, in data analysis, students are able to manage extensive datasets and do predictive analytics with the help of AIs, which improves the interpretation of results and helps refine hypotheses. Lastly, AIs streamline the referencing process by automatically generating accurate citations, upholding student academic integrity. Overall, while there are challenges associated with AIs, its integration empowers STEM undergraduate students to work more efficiently and effectively in their research activities.
Similarly, H2 is also supported. Utilizing AI tools to reshape students’ attitudes toward research significantly enhances engagement and learning, as reported by several studies (e.g., Darwin et al., 2023; Walter, 2024). It provides access to a wealth of academic resources, making research tasks less intimidating and encouraging a proactive approach. This accessibility allows STEM undergraduate students to focus on analysis rather than merely data gathering, thus, fostering deeper engagement with their work. Further, its adaptive features create personalized learning paths, which eventually caters individual needs and boosts confidence in dealing challenging research tasks. As Woolf et al. (2013) emphasized, AIs ability to recommend relevant resources encourages students to delve deeper into their research works leading to enhancing both their understanding and retention.
Moreover, integrating AIs in research works promotes students’ wellbeing by reducing their academic stress. Many students express high satisfaction with its capacity to personalize and simplify their learning experiences, particularly when they see its practical benefits firsthand. This positive disposition cultivates openness to AIs as valuable tools in research, reinforcing the findings of Almufarreh (2024) and Adair (2023). Additionally, AI can facilitate collaboration among students, enriching their learning experiences through peer review and group projects. This collaborative aspect promotes community, social skills, and more importantly positive disposition toward research as a shared endeavor. In other words, AIs integration in education transforms how students engage with research tasks, fostering a more inclusive, efficient, and dynamic learning environment leading to developing a better research disposition.
The hypothesis on AI dependence positively and significantly influencing STEM undergraduate students’ research self-efficacy is also supported (H3). The capacity of AIs to streamline complex tasks and provide precise insights makes them an essential tool in supporting research self-efficacy which is an important construct in academic development (Livinți and Iliescu, 2021). For STEM undergraduate students where the requirements for conducting research projects is substantial, it is essential as it encourages them to engage in challenging research projects, persist through adversities, and foster innovation (Bejtic, 2024). AIs’ balanced integration in research offers structured support, thus, helping them develop both the confidence and competence needed to navigate the complexities of academic inquiry effectively. AI dependence as an increasing reliance on AI tools for tasks such as data analysis, decision-making, and information retrieval can enhance research self-efficacy by providing students with tools that simplify complex research tasks (Zhai et al., 2024). This supports the idea that AI dependence positively influences research self-efficacy as shown in the results. By making processes more efficient, AIs can reduce some of the barriers associated with challenging research projects, thus allowing students to focus on higher-level thinking and build confidence in their abilities to manage these tasks. This sense of support and efficiency can contribute to a stronger belief in their research capabilities, supporting the development of research self-efficacy (Mah and Groß, 2024). In turn, students’ dependence on AI influences research self-efficacy letting students set higher goals for themselves and are more likely to intend to perform more challenging tasks.
The findings then indicate that research skills serve as a strong predictor of research productivity, highlighting their critical role in producing high-quality outputs (Vieno et al., 2022). Hence, H4 is supported. Students with well-developed competencies in critical thinking, data analysis, and academic writing are more adept and better equipped in defining precise research questions, selecting appropriate methodologies, and interpreting findings accurately. This supports the findings of Carter et al. (2016), which showed that students with established research competencies produced higher-quality outputs and achieved more reliable outcomes, which supports increased productivity (Alsaleh, 2020). Additionally, effective communication skills also enhance the clarity and impact of research findings, increasing their potential for productive output (Schmid et al., 2023). Beyond these technical skills, the capacity to manage time and resources effectively, along with researchers’ confidence in their skills, creates a positive feedback loop. As students develop and apply their research skills, they gain confidence and become more inclined to tackle new challenges, promoting further productivity (Mydin et al., 2021). This cycle of skill development and application not only contributes to increased productivity but also reinforces the essential capabilities of the student researcher that drive each stage of making good quality research (Tahsildar and Hasani, 2021). Ultimately, the influence of research skills to research output quality suggests that targeted skill-building interventions could significantly enhance students’ research productivity (Azmi and Daud, 2019; George-Reyes et al., 2023).
Research disposition is also found out to have a significant and positive influence on students’ research productivity, thus, supporting H5. When STEM undergraduate students feel engaged with research, they are more likely to put in the time and effort needed, seeing challenges as opportunities for growth rather than obstacles. This aligns to previous studies reporting that when students are interested and enjoy engaging research, they are more likely to increase the chances of succeeding research tasks (e.g., Atieno et al., 2021; Albert et al., 2018; Papanastasiou, 2005). In addition, encouraging intrinsic motivation and a positive attitude toward research is essential, as these qualities drive consistent effort in research-related tasks which are crucial for research productivity (Prado, 2019; Vereijken et al., 2018; Whipple et al., 2015). This motivation and attitude encourage students to look for resources, work with peers, and learn new skills, which makes their research performance more effective. Salainti (2024) also suggests that a positive mindset builds confidence, reducing hesitation and self-doubt, which leads to more consistent progress. In other words, students who experience self-doubt, especially in their early academic experiences, may struggle to engage fully with their research tasks, hindering their productivity. Therefore, a positive research disposition can lead to improved research outcomes and greater productivity.
Results showed that students’ research self-efficacy is associated with their research productivity, corroborating the findings of Woo et al. (2024) and supporting H6. Several aspects of the students’ research self-efficacy likely influence their research productivity, including confidence in skill application, motivation and goal setting, and effective feedback-seeking and collaboration. High self-efficacy fosters tenacity and sustained productivity, particularly when students are provided with opportunities for growth and innovation such as in formulating hypotheses, designing studies, collecting data, and analyzing results. Research is fraught with setbacks, challenges and uncertainties. However, students with high self-efficacy view challenges as learning opportunities rather than threats. This empowers them to be resilient, which is critical for maintaining consistent productivity over time as reiterated in the study of Cassidy (2015). Self-efficacious students are more motivated to set ambitious goals and effectively manage their time, resulting in improved organization and higher quality outputs. Their inclination to seek feedback from advisors and collaborate with peers further enhances their research efforts, thereby accelerating progress and productivity. Additionally, research self-efficacy alleviates stress and minimizes delays since students who trust their abilities are less likely to procrastinate tasks due to fear of failure (Forester et al., 2004; Pasupathy and Siwatu, 2014). Overall, research self-efficacy cultivates resilience, motivation, collaboration, and confidence. These are the elements which contribute to achieving and maintaining high levels of research productivity among students.
Finally, the most critical finding of this study is the unsupported relationship between AI dependence and STEM undergraduate student’s research productivity (H7). It supports previous research findings of Yi et al. (2024). The lack of positive influence may stem from several factors such as spread of misinformation and flawed research outcomes, increased laziness (Lund et al., 2024), reduction in critical thinking skills, and loss of independent thinking (Ahmad et al., 2023). Moreover, AIs may have the potential to significantly increase research productivity, but this potential still depends on the mediation of research skills, disposition, and self-efficacy. The results posit that these three mediators are influenced by AI dependence and consequently improve research output as the causal link between AI dependence and research productivity is not supported. For example, students who are able to traverse AI systems more effectively, eventually possess good research skills (e.g., information retrieval and processing). Similarly, students who are likely to see AIs as useful tools or resources rather than replacements of their talents or skills develops positive research disposition and self-efficacy (Liang et al., 2023; Collins et al., 2021). Without these mediators, relying only on AIs alone is unlikely to provide favorable effects in research productivity and may just potentially affect critical thinking and creativity. As the results suggest, the research skills, disposition and self-efficacy improve research productivity. This further shows that although AIs can streamline processes and increase efficiency, the quality of research outputs still relies on students’ research competence. If they lack the right amount of research skills, confidence in their research abilities, and attitudes and values toward research, productivity can still suffer even if AIs are available.
Conclusion
The study examines the role of STEM undergraduate students AI dependence in influencing research productivity. Findings show that AI dependence alone does not significantly impact productivity. However, it reveals that AIs dependence influence the development of research skills, disposition, and self-efficacy, and eventually, these factors are key drivers or predictors of research productivity. These findings highlight the role of AIs as a supportive tool in fostering research competencies rather than a direct catalyst for research productivity. In this regard, these findings offer theoretical, policy, and practical implications.
First, it emphasizes that AI dependence supports the development of research skills, research disposition, and self-efficacy. While, research skills, research disposition, and self-efficacy serve as predictors of research productivity. As these factors significantly impact students’ research productivity, evidently, there is growing reliance on AI tools among students. This means that the way they approach research has changed. They gain support on research by modernizing processes of gathering data, reviewing the literature and among others through AIs integration. Additionally, AIs allow students to focus on higher-level thinking and analysis, making learning deeper and more meaningful. The developed theory in this study also helps explain how students’ research skills, disposition, and attitudes developed through AI influence research productivity. Its assistance in performing research-related tasks generally helps students feel more capable of doing research.
Second, in terms of the implication of the study to policy-making, on a practical level, there is a need for policies that encourage the use of AIs in schools and universities. Educational institutions could consider including AIs literacy as a standard part of the curriculum, so students could acquire knowledge on how to use these tools effectively and responsibly. Policymakers could also address access issues, ensuring that all students, regardless of their background, benefit from AI resources. In addition, if the educators are trained on AI use, it could foster a supportive environment where students learn to use AIs not just as a simplified way or shortcut, but as a tool for probing knowledge, providing deeper engagement with research. With the benefits brought by AIs, come challenges and threats to academic integrity. While AIs can make research more efficient, there are risks of over-dependence, and concerns about ethical use, including plagiarism. Policies should focus on guiding students to use AIs as complement to, not a replacement for, their intellectual engagement, emphasizing the importance of critical thinking alongside AI support.
Finally, on the practice of AI use. Students’ motivation and general study experience will greatly benefit from AIs’ capacity to personalize research and make it seem less daunting. A collaborative research approach where students feel linked to their work and to one another could be fostered by policies that support the adaptive and exploratory use of AIs. By making research more accessible and less intimidating, AIs have the potential to shape a learning environment that is inclusive, engaging, and empowering for all students by lowering the barrier to research and making it more approachable.
Future directions
Future research should build on the findings of this study by incorporating longitudinal and experimental designs to strengthen causal interpretations, as a longitudinal approach would track changes in AI dependence and research productivity over time, while experimental studies could manipulate AI usage conditions to assess direct effects. Additionally, to mitigate biases associated with self-reported data, future studies should integrate objective measures of research productivity, such as the number of publications, citations, journal impact factors, and research engagement metrics. A mixed-methods approach, combining self-reports with objective indicators, would enhance the reliability of findings. Furthermore, considering the broad age range and the high proportion of first-year students in the sample, future research should explore academic level as a moderating factor or conduct subgroup analyses to examine how AI dependence influences research productivity across different student cohorts. It is also crucial to examine the potential negative effects of AI dependence, such as reduced critical thinking, increased risk of plagiarism, and over-reliance on technology, which may offset any perceived benefits. Introducing qualitative methods, such as interviews and case studies, would provide deeper insights into students’ experiences and attitudes toward AI in research, offering a more comprehensive understanding of its role in academic settings. These methodological enhancements will contribute to stronger causal evidence and a more nuanced perspective on both the benefits and risks of AI dependence in research productivity.
Data availability statement
The raw data supporting the conclusions of this article will be made available by the authors, without undue reservation.
Ethics statement
Ethical review and approval was not required for the study on human participants in accordance with the local legislation and institutional requirements. Written informed consent from the participants was not required to participate in this study in accordance with the national legislation and the institutional requirements.
Author contributions
JB: Conceptualization, Data curation, Formal analysis, Investigation, Methodology, Project administration, Resources, Software, Visualization, Writing – original draft, Writing – review & editing, Funding acquisition, Supervision, Validation. JI: Conceptualization, Data curation, Formal analysis, Funding acquisition, Investigation, Methodology, Project administration, Resources, Software, Visualization, Writing – original draft, Writing – review & editing. OC: Conceptualization, Data curation, Formal analysis, Investigation, Methodology, Project administration, Resources, Software, Visualization, Writing – original draft, Writing – review & editing. KG: Conceptualization, Data curation, Formal analysis, Investigation, Methodology, Project administration, Resources, Software, Visualization, Writing – original draft, Writing – review & editing. RS: Conceptualization, Data curation, Formal analysis, Investigation, Methodology, Project administration, Resources, Software, Visualization, Writing – original draft, Writing – review & editing. NR: Conceptualization, Data curation, Formal analysis, Investigation, Methodology, Project administration, Resources, Software, Visualization, Writing – original draft, Writing – review & editing. JoC: Conceptualization, Data curation, Formal analysis, Investigation, Methodology, Project administration, Resources, Software, Visualization, Writing – original draft, Writing – review & editing. RA: Conceptualization, Data curation, Formal analysis, Investigation, Methodology, Project administration, Resources, Software, Visualization, Writing – original draft, Writing – review & editing. DD: Conceptualization, Data curation, Formal analysis, Investigation, Methodology, Project administration, Resources, Software, Visualization, Writing – original draft, Writing – review & editing. IR: Conceptualization, Data curation, Formal analysis, Investigation, Methodology, Project administration, Resources, Software, Visualization, Writing – original draft, Writing – review & editing. SA: Conceptualization, Data curation, Formal analysis, Investigation, Methodology, Project administration, Resources, Software, Visualization, Writing – original draft, Writing – review & editing. TD: Conceptualization, Data curation, Formal analysis, Investigation, Methodology, Project administration, Resources, Software, Visualization, Writing – original draft, Writing – review & editing. RP: Conceptualization, Data curation, Formal analysis, Investigation, Methodology, Project administration, Resources, Software, Visualization, Writing – original draft, Writing – review & editing. JS: Conceptualization, Data curation, Formal analysis, Investigation, Methodology, Project administration, Resources, Software, Visualization, Writing – original draft, Writing – review & editing. KM: Conceptualization, Data curation, Formal analysis, Investigation, Methodology, Project administration, Resources, Software, Visualization, Writing – original draft, Writing – review & editing. JJ: Conceptualization, Data curation, Formal analysis, Investigation, Methodology, Project administration, Resources, Software, Visualization, Writing – original draft, Writing – review & editing. MJ: Conceptualization, Data curation, Formal analysis, Investigation, Methodology, Project administration, Resources, Software, Visualization, Writing – original draft, Writing – review & editing, Funding acquisition, Supervision, Validation. KRM: Conceptualization, Data curation, Formal analysis, Investigation, Methodology, Project administration, Resources, Software, Visualization, Writing – original draft, Writing – review & editing. LL: Conceptualization, Data curation, Formal analysis, Investigation, Methodology, Project administration, Resources, Software, Visualization, Writing – original draft, Writing – review & editing. JaC: Conceptualization, Data curation, Formal analysis, Investigation, Methodology, Project administration, Resources, Software, Visualization, Writing – original draft, Writing – review & editing. PF: Conceptualization, Data curation, Formal analysis, Investigation, Methodology, Project administration, Resources, Software, Visualization, Writing – original draft, Writing – review & editing. ED: Conceptualization, Data curation, Formal analysis, Investigation, Methodology, Project administration, Resources, Software, Visualization, Writing – original draft, Writing – review & editing. FA: Conceptualization, Data curation, Formal analysis, Investigation, Methodology, Project administration, Resources, Software, Visualization, Writing – original draft, Writing – review & editing. MM: Conceptualization, Data curation, Formal analysis, Investigation, Methodology, Project administration, Resources, Software, Visualization, Writing – original draft, Writing – review & editing. FE: Conceptualization, Data curation, Formal analysis, Investigation, Methodology, Project administration, Resources, Software, Visualization, Writing – original draft, Writing – review & editing. MR: Conceptualization, Data curation, Formal analysis, Investigation, Methodology, Project administration, Resources, Software, Visualization, Writing – original draft, Writing – review & editing. JR: Conceptualization, Data curation, Formal analysis, Investigation, Methodology, Project administration, Resources, Software, Visualization, Writing – original draft, Writing – review & editing. FG: Conceptualization, Data curation, Formal analysis, Investigation, Methodology, Project administration, Resources, Software, Visualization, Writing – original draft, Writing – review & editing. LO: Conceptualization, Data curation, Formal analysis, Investigation, Methodology, Project administration, Resources, Software, Visualization, Writing – original draft, Writing – review & editing. JG: Conceptualization, Data curation, Formal analysis, Investigation, Methodology, Project administration, Resources, Software, Visualization, Writing – original draft, Writing – review & editing. NN: Conceptualization, Data curation, Formal analysis, Investigation, Methodology, Project administration, Resources, Software, Visualization, Writing – original draft, Writing – review & editing. GD: Conceptualization, Data curation, Formal analysis, Investigation, Methodology, Project administration, Resources, Software, Visualization, Writing – original draft, Writing – review & editing. SC: Conceptualization, Data curation, Formal analysis, Investigation, Methodology, Project administration, Resources, Software, Visualization, Writing – original draft, Writing – review & editing.
Funding
The author(s) declare that no financial support was received for the research and/or publication of this article.
Conflict of interest
The authors declare that the research was conducted in the absence of any commercial or financial relationships that could be construed as a potential conflict of interest.
Generative AI statement
The authors declare that no Gen AI was used in the creation of this manuscript.
Publisher’s note
All claims expressed in this article are solely those of the authors and do not necessarily represent those of their affiliated organizations, or those of the publisher, the editors and the reviewers. Any product that may be evaluated in this article, or claim that may be made by its manufacturer, is not guaranteed or endorsed by the publisher.
References
Adair, A. (2023). Teaching and learning with AI: how artificial intelligence is transforming the future of education. XRDS: Crossroads ACM Magazine Stu. 29, 7–9. doi: 10.1145/3589252
Ahmad, S. F., Han, H., Mansoor Alam, M., Khairul Rehmat, M., Irshad, M., Arraño-Muñoz, M., et al. (2023). Impact of artificial intelligence on human loss in decision making, laziness, and safety in education. Hum. Soc. Sci. Commun. 10:1787. doi: 10.1057/s41599-023-01787-8
Albert, C., Davia, M. A., and Legazpe, N. (2018). Job satisfaction amongst academics: the role of research productivity. Stud. High. Educ. 43, 1362–1377. doi: 10.1080/03075079.2016.1255937
Almufarreh, A. (2024). Determinants of students’ satisfaction with AI tools in education: a PLS-SEM-ANN approach. Sustain. For. 16:354. doi: 10.3390/su16135354
Alsaleh, N. J. (2020). Teaching critical thinking skills: literature review. Turkish Online J. Technol. 19, 1–10.
Alvarez-Ochoa, R., Torres-Criollo, L. M., Heredia-Cabrera, G., and Zhindon-Arevalo, C. (2022). Impact of research skills of university teachers on scientific productivity. Revista Científica INSPILIP 6, 47–55. doi: 10.31790/inspilip.v6i1.273
Andersdotter, K. (2023). Artificial intelligence skills and knowledge in libraries. J. Inf. Liter. 17:14. doi: 10.11645/17.2.14
Atieno, A. V., Onyancha, O. B., and Kwanya, T. (2021). Trends, patterns and determinants of research productivity at the technical University of Kenya. Inf. Dev. 38, 97–113. doi: 10.1177/0266666920983400
Azmi, N. A., and Daud, N. B. (2019). A relationship between research skills and autonomous learning among postgraduate students. Int. J. Bus. Econ. Law 18, 1552–2289.
Bai, L., Millwater, J., and Hudson, P. (2013). Factors that influence Chinese TEFL academics' research capacity building: an institutional case study. Asia Pac. Educ. Res. 22, 119–126. doi: 10.1007/s40299-012-0004-6
Baidoo-Anu, D., and Ansah, L. O. (2023). Education in the era of generative artificial intelligence (AI): understanding the potential benefits of ChatGPT in promoting teaching and learning. J. AI 7, 52–62. doi: 10.61969/jai.1337500
Bejtic, Z. (2024). Nurturing feedback and its impact on self-efficacy, empowerment, and professional growth in educational and corporate environments. iJAC 17, 17–27. doi: 10.3991/ijac.v17i2.45237
Bernardo, A. B. I. (2004). Constructivism, curriculum, and the challenges in transforming science education in the Philippines. Learning Edge 4, 1–33.
Bieschke, K. (2006). Research self-efficacy beliefs and research outcome expectations: implications for developing scientifically minded psychologists. J. Career Assess. 14, 77–91. doi: 10.1177/1069072705281366
Bonsay, J. O., Cruz, A. P., Firozi, H. C., and Camaro, P. J. (2021). Artificial intelligence and labor productivity paradox: the economic impact of AI in China, India, Japan, and Singapore. J. Econ. Financ. Accounting Studies 3, 120–139. doi: 10.32996/jefas.2021.3.2.13
Bouzar, A., El Idrissi, K., and Ghourdou, T. (2024). ChatGPT and academic writing self-efficacy: unveiling correlations and technological dependency among postgraduate students. AWEJ 1, 225–236. doi: 10.24093/awej/ChatGPT.15
Cabansag, M. G. S. (2014). Impact statements on the K-12 science program in the enhanced basic education curriculum in provincial schools. J. Arts Sci. Comm. 5:29.
Carter, S. R., Moles, R. J., Krass, I., and Kritikos, V. S. (2016). Using social cognitive theory to explain the intention of final-year pharmacy students to undertake a higher degree in pharmacy practice research. Am. J. Pharm. 80:95. doi: 10.5688/ajpe80695
Cassidy, S. (2015). Resilience building in students: the role of academic self-efficacy. Front. Psychol. 6:1781. doi: 10.3389/fpsyg.2015.01781
Collins, C., Dennehy, D., Conboy, K., and Mikalef, P. (2021). Artificial intelligence in information systems research: a systematic literature review and research agenda. Int. J. Inf. Manag. 60:102383. doi: 10.1016/j.ijinformgt.2021.102383
Cortes, S., Agero, A., Agravante, E. M., Arado, J., Arbilon, C. A., Lampawog, E., et al. (2023). Factors influencing students’ intention to enroll in bachelor of science in biology: a structural equation modeling approach. Cogent Educ. 10:635. doi: 10.1080/2331186X.2023.2273635
Damianus, A., Magallanes, T., Encarnacion, M. J., and Foronda, S. L. (2019). The attitude of graduate students toward research and their intention to conduct research in the future. Int. J. Modern Res. Eng. Manage. 2, 74–87.
Darwin, R., Mukminatien, N., Suryati, N., and Laksmi, E. (2023). Critical thinking in the AI era: an exploration of EFL students’ perceptions, benefits, and limitations. Cogent Educ. 11:2290342. doi: 10.1080/2331186X.2023.2290342
Dwivedi, Y. K., Hughes, L., Ismagilova, E., Aarts, G., Coombs, C., Crick, T., et al. (2021). Artificial Intelligence (AI): Multidisciplinary perspectives on emerging challenges, opportunities, and agenda for research, practice and policy. Int. J. Inf. Manag. 57:101994. doi: 10.1016/j.ijinfomgt.2019.08.002
Estonanto, A. J. J. (2017). Acceptability and difficulty of the STEM track implementation in senior high school. Asia Pacific J. Multidisciplinary Res. 5, 43–50.
Forester, M., Kahn, J., and Hesson-McInnis, M. (2004). Factor structures of three measures of self-efficacy. SAGE Open 42, 29–45. doi: 10.1177/07342829231204507
Fosner, A. (2024). University students' attitudes and perceptions towards AI tools: implications for sustainable educational practices. Sustain. For. 16:668. doi: 10.3390/su16198668
Gaoat, M. (2022). Relationship of research self-efficacy, perceptions of the research training environment and interest in research among business education students. Heritage Sci. 7:11. doi: 10.11648/j.her.20220706.11
Gefen, D., Straub, D., and Boudreau, M. (2000). Structural equation modeling and regression: guidelines for research practice. Commun. Assoc. Inf. Syst. 4, 407–420. doi: 10.17705/1CAIS.00407
George-Reyes, C. E., Lopez-Caudana, E. O., and Ramirez-Montoya, M. S. (2023). Research competencies in university students: intertwining complex thinking and education 4.0. Contemp. Educ. Technol. 15:13767. doi: 10.30935/cedtech/13767
Hair, J. F., Black, W. C., Babin, B. J., and Anderson, R. E. (2009). Multivariate data analysis. New York, NY: Pearson Education.
Hemmings, B., and Kaya, R. (2015). The relationship between research self-efficacy, research disposition, and publication output. Educ. Psychol. Int. J. Exp. Educ. Psychol. 35, 345–360. doi: 10.1080/01443410.2015.1029463
Heng, K., Hamid, O., and Khan, A. (2020). Factors influencing academics’ research engagement. Issues Educ. Res. 30, 965–987.
Ipanaque-Zapata, M., Figueroa-Quinones, J., Bazalar-Palacios, J., Arhuis-Inca, W., Quinones-Negrete, M., and Villarreal-Zegarra, D. (2023). Research skills for university students’ thesis in E-learning: scale development and validation in Peru. Heliyon 9:e13770. doi: 10.1016/j.heliyon.2023.e13770
Kline, R. B. (2016). Principles and practice of structural equation modeling. 4th Edn. London: The Guilford Press.
Laidlaw, A., Aiton, J., Struthers, J., and Guild, S. (2012). Developing research skills in medical students: AMEE guide no. 69. Med. Teach. 34, 754–771. doi: 10.3109/0142159X.2012.704438
Liang, J., Wang, L., Luo, J., Yan, Y., and Fan, C. (2023). The relationship between student interaction with generative artificial intelligence and learning achievement: serial mediating roles of self-efficacy and cognitive engagement. Front. Psychol. 14:1285392. doi: 10.3389/fpsyg.2023.1285392
Lin, H., and Chen, Q. (2024). Artificial intelligence (AI)-integrated educational applications and college students’ creativity and academic emotions: Students' and teachers’ perceptions and attitudes. BMC Psychol. 12:1979. doi: 10.1186/s40359-024-01979-0
Livinți, R. G. L., and Iliescu, D. (2021). Research self-efficacy: a meta-analysis. Educ. Psychol. 56, 215–242. doi: 10.1080/00461520.2021.1886103
Lund, B., Lamba, M., and Oh, S. H. (2024). The impact of AI on academic research and publishing. Int. J. Educ. Technol. High. Educ. 18:292. doi: 10.48550/arXiv.2406.06009
Lund, B. D., and Wang, T. (2023). Chatting about ChatGPT: how may AI and GPT impact academia and libraries. Library Hi Tech News 40, 26–29. doi: 10.1108/LHTN-01-2023-0009
Mah, D. K., and Groß, N. K. (2024). Artificial intelligence in higher education: exploring faculty use, self-efficacy, distinct profiles, and professional development needs. Int. J. Educ. Technol. High. Educ. 21, 1–17. doi: 10.1186/s41239-024-00490-1
Manitzas Hill, H. M., Zwahr-Castro, J., and Gonzalez, A. (2022). Evaluating research self-efficacy in undergraduate students: experience matters. J. Scholar. Teach. Learning 22, 67–80. doi: 10.14434/josotl.v22i1.31203
Montebon, D. T. (2014). K12 science program in the Philippines: student perception on its implementation. Int. J. Educ. Res. 2, 153–164.
Morales, D. X., Grineski, S. E., and Collins, T. W. (2017). Increasing research productivity in undergraduate research experiences: exploring predictors of collaborative faculty-student publications. CBE Life Sci. Educ. 16:326. doi: 10.1187/cbe.16-11-0326
Morales-García, W. C., Sairitupa-Sanchez, L. Z., Morales-García, S. B., and Morales-García, M. (2024). Development and validation of a scale for dependence on artificial intelligence in university students. Frontiers in Education 9:1323898. doi: 10.3389/feduc.2024.1323898
Mustafakulov, S. (2023). Research activities of students during the learning process. Eur. Int. J. Pedagogics 3, 21–23. doi: 10.55640/eijp-03-12-06
Mydin, F., Rahman, R. S., and Mohammad, W. M. (2021). Research collaboration: enhancing the research skills and self-confidence of early career academics. Asian J. Univ. Educ. 17:142. doi: 10.24191/ajue.v17i3.14508
Necula, S. C. (2024). Assessing the impact of artificial intelligence tools on employee productivity: insights from a comprehensive survey analysis. Electronics 13:758. doi: 10.3390/electronics13183758
Nguyen, A., Kremantzis, M., Essien, A., Petrounias, I., and Hosseini, S. (2024). Enhancing student engagement through artificial intelligence (AI): understanding the basics, opportunities, and challenges. J. Univ. Teach. Learn. Prac. 21:92. doi: 10.53761/caraaq92
Obuku, E. A., Lavis, J. N., Kinengyere, A., Ssenono, R., Ocan, M., Mafigiri, D. K., et al. (2018). A systematic review on academic research productivity of postgraduate students in low- and middle-income countries. Health Res. Policy Syst. 16:86. doi: 10.1186/s12961-018-0360-7
Orale, D. H. S. R. L., and Sarmiento, D. H. (2016). Senior high school curriculum in the Philippines, USA, and Japan. J. Acad. Res. 1, 12–23.
Papanastasiou, E. C. (2005). Factor structure of the “attitudes toward research” scale. Stat. Educ. Res. J. 4, 16–26. doi: 10.52041/serj.v4i1.523
Papanastasiou, E. C. (2014). Revised-attitudes toward research scale (R-ATR); a first look at its psychometric properties. J. Res. Educ. 24, 146–159. doi: 10.1037/t35506-000
Pasupathy, R., and Siwatu, K. (2014). An investigation of research self-efficacy beliefs and research productivity among faculty members at an emerging research university in the USA. High. Educ. Res. Dev. 33, 728–741. doi: 10.1080/07294360.2013.863843
Patricio, G. (2022). Research, writing, and collaborative skills and research output quality of senior high school students under the new normal. J. World Eng. Educ. Prac. 4, 35–69. doi: 10.32996/jweep.2022.4.2.5
Pires da Costa, W., Ferreira Melo, A., Mota Venâncio, P. E., de São Bernardo, M. A., Noll, P. R. E. S., and Noll, M. (2024). Students’ research experience, self-perceptions, and scientific productivity in undergraduate research programs: a case study of a Brazilian Midwest institute. Innov. Educ. Teach. Int. 62, 688–703. doi: 10.1080/14703297.2024.2339312
Prado, N. I. (2019). Predictors of research productivity among administrators, faculty, and students. Liceo J. f Higher Educ. Res. 15, 55–68. doi: 10.7828/LJHER.V15I2.1319
Ricardo, M., and Ricardo, R. (2023). Self-efficacy, mentoring experience, and performance levels of STEM students in research through distance learning modalities. Psychol. Educ. Multidisciplinary J. 13:8363991. doi: 10.5281/zenodo.8363991
Rogayan, D. Jr., Rafanan, R. J., and De Guzman, C. Y. (2021). Challenges in STEM learning: a case of Filipino high school students. J. Penelitian Pembelajaran IPA 7:232. doi: 10.30870/jppi.v7i2.11293
Salainti, E. (2024). The impact of students’ self-confidence in their learning process. J. Scientia 13, 760–771. doi: 10.58471/scientia.v13i01.2260
Santos, J. A., and Santos, M. C. (2015). Strategies for writing a research paper. Tour. Manage. Stu. 11, 6–11.
Schmid, K. M., Hall, S. E., and Wiles, J. R. (2023). Different approaches for engaging undergraduates in research: variable impacts on students' self-efficacy, science research skills, and future goals. Bioscene 49, 15–28.
Seo, K., Tang, J., Roll, I., Fels, S., and Yoon, D. (2021). The impact of artificial intelligence on learner–instructor interaction in online learning. Int. J. Educ. Techno. High. Educ. 18:292. doi: 10.1186/s41239-021-00292-9
Tahsildar, N., and Hasani, R. (2021). Faculty-perceived research skills and research productivity: a case study at a public university in Afghanistan. Indian J. Sci. Technol. 14, 229–238. doi: 10.17485/IJST/v14i3.2065
Tarima, S., and Flournoy, N. (2019). Asymptotic properties of maximum likelihood estimators with sample size recalculation. Stat. Med. 60, 373–394. doi: 10.1007/s00362-019-01095-x
UNESCO (2023). A global roadmap for internet universality: Advancing digital rights and principles for all (UNESCO series on internet freedom). Geneva: UNESCO.
Vereijken, M., van der Rijst, R. M., De Beaufort, A. J., van Driel, J. V., and Dekker, F. (2018). Fostering first-year student learning through research integration into teaching: student perceptions, beliefs about the value of research, and student achievement. Innov. Educ. Teach. Int. 55, 425–432. doi: 10.1080/14703297.2016.1260490
Vieno, K., Rogers, K., and Campbell, N. (2022). Broadening the definition of research skills to enhance students' competence across undergraduate and master's programs. Educ. Sci. 12:642. doi: 10.3390/educsci12100642
Wagner, G., Lukyanenko, R., and Paré, G. (2021). Artificial intelligence and the conduct of literature reviews. SAGE Open 37, 209–226. doi: 10.1177/02683962211048201
Walter, Y. (2024). Embracing the future of artificial intelligence in the classroom: the relevance of AI literacy, prompt engineering, and critical thinking in modern education. Int. J. Educ. Technol. High. Educ. 21:448. doi: 10.1186/s41239-024-00448-3
Wester, K. L., Gonzalez, L., DiAnne Borders, L., and Ackerman, T. (2019). Initial development of the faculty research self-efficacy scale (FaRSES): evidence of reliability and validity. J. Prof. 10, 78–99.
Whipple, E., Hughes, A. K., and Bowden, S. (2015). Evaluation of a BSW research experience: improving student research competency. J. Soc. Work. Educ. 35, 397–409. doi: 10.1080/08841233.2015.1063568
Woo, H., Kim, N., Lee, J., Chae, K., and Mathew, A. (2024). Research self-efficacy and research productivity of doctoral students in counseling programmes: research training environment as a moderator. Br. J. Guidance Counsel. 52, 1071–1080. doi: 10.1080/03069885.2023.2297892
Woolf, B. P., Lane, H. C., Chaudhri, V. K., and Kolodner, J. L. (2013). AI grand challenges for education. AI Mag. 34, 66–84. doi: 10.1609/aimag.v34i4.2490
Yang, S., and Bai, H. (2020). The integration design of artificial intelligence and normal students' education. J. Phys. Conf. Ser. 1453:12090. doi: 10.1088/1742-6596/1453/1/012090
Zhai, C., Wibowo, S., and Li, L. (2024). The effects of over-reliance on AI dialogue systems on students' cognitive abilities: a systematic review. Smart Learn. Environ. 11, 1–37. doi: 10.1186/s40561-024-00316-7
Keywords: science education trends, AI in higher education, educational technology, student research behavior, digital literacy
Citation: Buniel JM, Intano J, Cuartero O, Grustan KJ, Sumaoy R, Reyes N Jr, Calipayan J Jr, Arreo R, Duero D, Rosil I, Agustin S, Diron TJ, Pingol RJ, Sapuras JV, Miranda K, Julve J, Josol M, Mercado KR, Latoja L, Cubillan J, Fallado PAJ, Duran EL, Ambray FI, Miranda M, Etchon FM, Ramoso MM, Rubenial JR, Ganancias F, Orozco L, Gracia J, Notado N, Darao G and Cortes S (2025) Modeling the influence of AI dependence to research productivity among STEM undergraduate students: case of a state university in the Philippines. Front. Educ. 10:1535466. doi: 10.3389/feduc.2025.1535466
Edited by:
Dina Tavares, Polytechnic University of Leiria, PortugalReviewed by:
Doras Sibanda, University of KwaZulu-Natal, South AfricaOlga Tapalova, Abai University, Kazakhstan
Copyright © 2025 Buniel, Intano, Cuartero, Grustan, Sumaoy, Reyes, Calipayan, Arreo, Duero, Rosil, Agustin, Diron, Pingol, Sapuras, Miranda, Julve, Josol, Mercado, Latoja, Cubillan, Fallado, Duran, Ambray, Miranda, Etchon, Ramoso, Rubenial, Ganancias, Orozco, Gracia, Notado, Darao and Cortes. This is an open-access article distributed under the terms of the Creative Commons Attribution License (CC BY). The use, distribution or reproduction in other forums is permitted, provided the original author(s) and the copyright owner(s) are credited and that the original publication in this journal is cited, in accordance with accepted academic practice. No use, distribution or reproduction is permitted which does not comply with these terms.
*Correspondence: Sylvester Cortes, c3lsdmVzdGVyLmNvcnRlc0BjdHUuZWR1LnBo