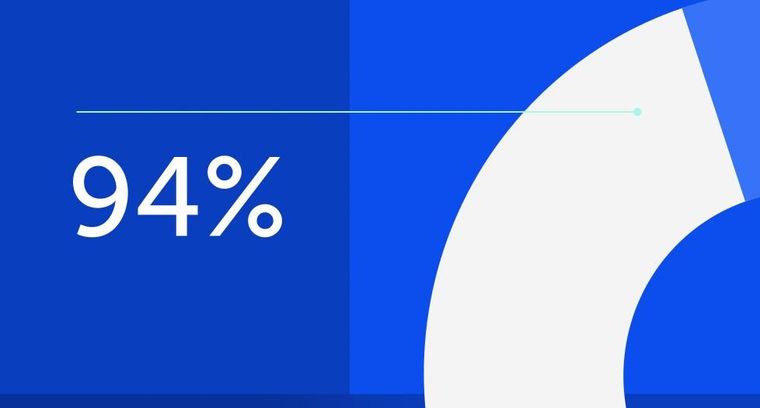
94% of researchers rate our articles as excellent or good
Learn more about the work of our research integrity team to safeguard the quality of each article we publish.
Find out more
ORIGINAL RESEARCH article
Front. Educ., 14 March 2025
Sec. Digital Education
Volume 10 - 2025 | https://doi.org/10.3389/feduc.2025.1532802
The increasing availability of digital technology for second language (L2) learning is transforming traditional teaching methods, yet the quality of these resources remains unclear. A survey was conducted among a stratified sample of second language teachers (N = 118) from UK primary and secondary schools to evaluate the use of digital tools used in classrooms. A rating tool, grounded in educational and language learning theories, was developed to assess individual software features. Results showed extensive use of digital resources, with 89% of teachers utilizing digital devices and over half employing more than three different software types. However, evaluations revealed varying adherence to language learning principles. Strengths were identified in the components of ‘engagement’ and ‘input’, whereas opportunities for improvement are observed in the aspects of ‘output’ and ‘social learning’. Additionally, higher software ratings did not correlate with usage frequency or price. These findings highlight the integration of digital tools in UK language learning pedagogy, but underscore the need for ongoing evaluation to improve software quality and effectiveness.
The rising interest in language learning combined with advances in digital technologies has resulted in a growing number of digital products intended for language learning (Gangaiamaran and Pasupathi, 2017; Rosell-Aguilar, 2017). However, despite both the rapidly expanding number of learning resources and governmental emphasis on language education (American Academy of Arts and Sciences et al., 2020; European Commission, 2018), language learning outcomes still fall short of expectations (Brecht, 2015; Collen, 2020; Costa and Albergaria-Almeida, 2015).
One of the potential explanations is related to the quality of the educational software produced. Despite increased quantity, most of the software remains unregulated and untested (Hirsh-Pasek et al., 2015), resulting in designs that could hinder instead of promote learning (Hirsh-Pasek et al., 2015; Sung et al., 2015). This chaotic landscape is frequently referred to as the “Digital Wild West” (Shing and Yuan, 2016), describing a market where quality control and clear guidelines are lacking, leaving users overwhelmed by the options. Rapidly changing trends, with new technologies emerging and disappearing within a matter of a few years (Zhang and Zou, 2020), make this gap in research increasingly obvious.
At the same time, while the use of digital technologies in language classrooms is generally encouraged, there is a lack of empirical research on how this technology is used (Heil et al., 2016; Reber and Rothen, 2018; Sung et al., 2015; Zhang and Zou, 2020; Ma and Yan, 2022). Although technology has the potential to bridge the gap between traditional and digital language education, the effectiveness of technology-assisted language learning ultimately depends on the context in which it is used and the specific needs it addresses (Burston, 2015; Kamasak et al., 2021; Sung et al., 2015).
Currently, the levels of competence in a second language remain (on average) low (Costa and Albergaria-Almeida, 2015). This is especially the case in the UK, where the systematic teaching of languages across primary schools in the United Kingdom is rather recent (Myles et al., 2019). This discrepancy between the number of possibilities for language learning and the disappointing outcomes leads to the assumption that more should be done to use the existing potential more efficiently.
One of the proposed solutions to address these issues is to “develop effective use of digital technology to support learning, training and reporting” (Myles et al., 2019). To achieve a truly effective approach to implementing L2 learning technologies, it is essential to integrate learning principles with a deep understanding of contextual factors. Therefore, it became increasingly apparent that to create educational software of high quality, the design process should include relevant scientific research and collaboration between different stakeholders such as classroom teachers, researchers, and technology specialists (O’Brien, 2020), as well as consistent use of evaluation tools (Kolak et al., 2021).
To that end, the following study was designed to evaluate the alignment of the current language learning software features with the theoretical principles of second language learning. Furthermore, it will examine the patterns of language software use in the classroom context.
Prior to presenting the study, we first introduce the theoretical principles of language learning that, ‘in theory’, should guide the evaluation of language learning software. We then consider the importance of the language learning setting, with an emphasis on the UK context.
Although the need to understand the relationship between theory and the design of technology-based materials was already identified three decades ago (Garret, 1991; Shaughnessy, 2003), this has not yet become standard. Around a decade ago it was estimated that 90% of language learning software was simply a digital reproduction of existing non-digital materials (Sweeney and Moore, 2012). Most of the software created around that time did not use the full potential of digitalization or consider the language learning principles that are specific for the digital content (Hirsh-Pasek et al., 2015). More recently, Yang and Shadiev (2019), as well as Li and Lan (2022) further identified a lack of theoretical grounding present in the field of digital language learning, urging for more effective knowledge transfer between research and industry.
To enable successful navigation on the market saturated with software of variable quality, it is essential to develop theoretically grounded evaluation tools (Kolak et al., 2021; Chapelle, 2001; O’Brien, 2020). In their review Hubbard and Levy (2016) point out that there is no single established theory that uniquely characterises CALL. Rather, this field uses and combines theories from different sources and traditions. Additionally, some theoretical approaches to L2 learning can sometimes be difficult to transform into practical implications for language learning software (Chapelle, 2008; Hubbard and Levy, 2016). For example, perspectives that attribute learning mostly to learners’ internal mechanisms are less concerned with the properties of the input, which is crucial in designing language software. On the other hand, psycholinguistic approaches are more focused on processing the input and the interaction, thereby offering potential guidelines that can be used to solve practical issues related to the software design (Chapelle, 2008).
Therefore, one solution to creating a theoretically grounded evaluation tool is to provide a holistic view that would include theories that explain critical aspects of language learning (Hubbard and Levy, 2016).
When talking both about the design and the evaluation of language learning software, understanding the linguistic and educational principles of second language learning has been identified as an important starting point (Chapelle, 2001; Reinders and Pegrum, 2015; Starke et al., 2020). To do so, it is useful to distinguish between general learning principles and language-specific learning principles.
Hirsh-Pasek et al. (2015) defined a set of general learning principles to advise the current design of educational apps. The “four pillars of learning” as set out by Hirsh-Pasek et al. (2015) are: active learning, meaningful learning, engaging learning and social learning. Including them as a cornerstone of educational software design helps in aligning the software to children’s natural inclination toward learning.
The active learning principle (pillar I) draws on constructivist theories that propose that children play an active role in their own learning, which results in knowledge being constructed rather than simply transmitted (Phillips, 1995). This translates into software that is “minds-on” and require challenge, thinking and mental manipulation of the content, as opposed to automatic tapping or swiping or merely observing the content. The principle of engagement (pillar II) in the learning process embodies behavioral, emotional and cognitive engagement. Engagement allows the learner to stay on task, avoid distractions and benefit more from the learning content. To achieve this, learning software should reduce the features that draw attention away from the learning content (sounds, flashy animations, etc), that ultimately disrupt the learning process (Hirsh-Pasek et al., 2015). The third principle characterises meaningful learning (pillar III) as learning that is relevant and connects to the learner’s experience and existing knowledge. This is where software have great potential to offer examples and multimodal connections that relate to the real world and learner’s existing concepts. Finally, the principle of social interaction (pillar IV) emphasizes the value of learning from social and communicative partners, which is in line with Vygotsky (1978) Sociocultural theory. Although social interactions are difficult to represent through software, this can be achieved through inclusion of multiple users to interact and collaborate or through parasocial interactions with on-screen characters.
Similarly, key areas that contribute to the educational value of learning apps in general have been explored by others as well, highlighting some of the same principles, with the addition of “feedback,” “narrative,” “language” and “adjustable content” (Kolak et al., 2021; Meyer et al., 2021; Tauson and Stannard, 2018). In the context of language learning software, these additional areas are best considered in relation to language learning principles.
Second language learning methods have shifted in recent decades from behaviorist to constructivist learning methods (Szabó and Csépes, 2023). Constructivists hold that individualized drilling and reinforcement practices (staples of behavioral methods) are insufficient for both first and second language learning (Szabó and Csépes, 2023; Long, 1996; Swain, 2005; Vygotsky, 1978; Tomasello, 2003). Instead, the learner learns by blending pre-existing with new knowledge encountered in social contexts, thus making language learning a social activity (Heil et al., 2016). From the language learning and teaching perspective, there is much agreement on pivotal aspects of language learning research that should be included in the process of language learning (Dubiner, 2018; Reinders and Pegrum, 2015); sufficient input through reading or hearing, opportunities to produce output, interaction through conversations or simulations and rehearsal where the contextualized material is repeated and practiced. Through the inclusion of these aspects of language use, software can move away from traditional reinforcement practices and come closer to the naturalistic and well-rounded language learning experience associated with constructivist theories.
Receiving input is a first step and a crucial element of language learning as it provides a basis for creating form-meaning mappings, according to Input Processing theory (Van Patten, 1993). However, the nature of that exposure makes a difference for the learner. Input that is comprehensible, contextualized and adapted to the learner’s level leads to the best learning outcomes (Krashen, 1994). Another important aspect of the input is the modality through which it is presented. Theories such as Dual coding theory (Paivio, 1971) and Multimedia learning theory (Mayer, 2009) propose that learning should ideally occur through a combination of different modalities (vision, audition, etc.). This allows for multiple mental representations of concepts, thus enhancing learning. Complementary to input, output or production is also a fundamental part of language acquisition process, as described by the Output hypothesis (Swain, 2005). Production, both spoken and written, gives the learner the opportunity to test out their hypotheses about transforming ideas into linguistic messages, to notice the gaps in knowledge and to reflect on their language use (Dubiner, 2018). The Interaction account (Long, 1996) integrates Input and Output by emphasizing the role of interaction, which supports language learning by engaging the learner to consolidate form-meaning relationships. One of the crucial aspects that occurs as a product of interaction is feedback. The feedback information would typically be received from the interactional partner and would serve as a tool for the speaker to evaluate their own production. This relates back to the well-known Vygotsky’s (1978) sociocultural theory, according to which learning stems from social experience and from navigating interactions with peers. Therefore, to make up for the absence of the real social interactions that are important in language learning, software should be able to provide meaningful and constructive feedback information that can mirror the ones normally obtained through conversations. Finally, with the practical constrictions within the classroom contexts in terms of the learning outcomes, the proponents of the Skill Acquisition Theory (SAT; DeKeyser, 2007) and the associate-cognitive framework (Ellis, 2007) argue how it is important to include rehearsal opportunities. These theories conceptualize language learning as being similar to general learning mechanisms, suggesting that learning occurs through repeated exposure. This has practical implications for the design of language learning software. While acknowledging the importance of context, the advocates of this theory support a mixed approach achieved through retaining automatization activities, but modified to be meaningful and contextualized (Gatbonton and Segalowitz, 2005).
Using only one of the theoretical approaches mentioned to guide the design or evaluation of comprehensive language learning software would be limited. Therefore, there is a need to combine these approaches to be able to thoroughly study second language learning (Chapelle, 2008; Hubbard and Levy, 2016). The principles derived from these approaches, together with the previously mentioned general learning principles form a theoretical, pedagogical and empirical base that should be built into language learning software to make it comprehensive and well-rounded, thereby optimizing learning.
The properties of the technologies on their own are not sufficient to bring positive language learning effects (Sung et al., 2015). The way that the technology is being used is also critical (Burston, 2015). The same learning tool can be used in different ways and across different contexts, which need to be taken into consideration when attempting evaluation (Egbert et al., 2009; Jamieson and Chapelle, 2010). Much of the research on digital language learning tools has focused on software for individual use (Mihaylova et al., 2022; Reber and Rothen, 2018). However, despite many types of language learning resources available, exactly what and how resources are actually being used by teachers in the classroom is not well understood. This is particularly true for primary and secondary classrooms, since recent reviews show that the majority of the studies in this area were done in classrooms at higher academic levels, predominantly undergraduate students (Goksu et al., 2020; Shadiev and Wang, 2022). Moreover, understanding the classroom context and the viewpoints of teachers who are both the users of these technologies, but also the experts in the educational aspect of language learning, is a valuable and often overlooked source of information in CALL research (Egbert et al., 2009).
Studies have examined teachers’ perspectives to discover what drives and prevents the use of educational software within classrooms. Kaimara et al. (2021) groups the found barriers within the Greek education system into external and internal category. The major external barriers found were lack of financial resources, lack of infrastructure and lack of policy and framework, whereas the internal barriers were the preference to traditional teaching methods and lack of training. Similarly, in their study of teaching practice across primary and secondary schools in China, Wang et al. (2019) divide the predictors of digital educational resource use into school-level and teacher-level factors. However, here none of the school-level factors were associated with the use of digital educational resources, whereas teacher-level factors such as attitudes, knowledge and skills of teachers predicted the use of digital resources. In their review on the impact of digital technologies on education, Timotheou et al. (2023) highlight lack of digital competencies as one of the most common challenges appearing in the literature. This has been reported as one of the barriers both on the side of the students, as well as teachers. Although it is frequently assumed that the younger users are advanced users of new digital technology, Reddy et al. (2022a) warn that a gap exists between personal and academic use of technology.
Overall, the predictors of use are context-dependent and differ between countries (Kaimara et al., 2021; Wang et al., 2019), as well as different school subjects (Rončević Zubković et al., 2022; Howard et al., 2014), thus emphasizing the need to examine the impact of different factors influencing language learning classrooms.
The language learning context of the UK, as well as the other English-speaking countries, presents specific challenges. Due to English being predominantly taught as a second language in other countries (Busse, 2017), the motivation for native English speakers to learn foreign languages is often lacking (Lanvers and Coleman, 2017). The systematic teaching of languages across primary schools in the United Kingdom is rather recent. The official requirement to incorporate language learning into the primary school came into force in 2014 after its importance has been highlighted by experts in the field. Before that, as Myles et al. (2019) report, the implementation tended to be “localised, vulnerable to change and variable in quality.” Although the policy introduction was an improvement, the guidelines on implementation were lacking, leading to unsatisfactory learning outcomes (Tinsley and Doležal, 2018). The main issues seem to be lack of time, lack of progress tracking, and low levels of staff language proficiency and confidence that together lead to students’ lack of motivation and loss of interest (Myles et al., 2019).
One of the proposed solutions to address these issues is to “develop effective use of digital technology to support learning, training and reporting” (Myles et al., 2019). To develop a truly effective approach to implementing L2 learning technologies, it is essential to integrate learning principles with a deep understanding of contextual factors. As Zhang and Zou (2020) suggest, a comprehensive evaluation of L2 learning technologies should consider not only the technical capabilities of the tools but also how they fit within the educational environment. By taking into account the specific learning objectives, user preferences, and institutional limitations, more effective digital resources can be designed, that bridge the gap between theoretical research and real-world application.
A recent review of the use of digital educational resources in classrooms by Timotheou et al. (2023) revealed that the most commonly investigated were software relating to STEM and literacy subjects, with a lack of studies on other curriculum subjects. Studies focusing on language learning classrooms to date have focused on a single technology or technology type (Jamieson and Chapelle, 2010; Zhang and Zou, 2020). Additionally, recent reviews point out that the studies predominantly included digital learning contexts where English is taught as a foreign language (Elaish et al., 2023; Shadiev and Yang, 2020; Zhang and Zou, 2020). In their review of 398 studies, Shadiev and Yang (2020) found that English was studied in 267 cases. The second most studied language was Chinese, occurring in only 26 studies.
To address these gaps, the main aim of the present study was to understand the patterns of use and quality of digital language learning software within the specific context of UK primary and secondary school classrooms. By approaching language teachers, we aimed to gain comprehensive insights into their use and quality, benefiting from teachers’ experience and expertise.
Our research questions related both to (1) identifying the patterns of use of language learning software across the UK classrooms and (2) to assessing the quality of software used.
In relation to the first goal, we aimed to outline the patterns of use through:
a) Understanding the nature of the digital environment in modern foreign language classrooms (e.g., how equipped are the language classrooms across the UK, are there prerequisites to the use of software?)
b) Identifying the specific digital language learning resources used in the language classrooms and the dynamics of their use
c) Identifying specific teacher-and school-level factors that explain the use of digital educational resources
In relation to the second goal, our aim was to rate language learning software based on the theoretical principles of learning (i.e., how do the features of digital language learning tools used in the classrooms relate to the theories of language learning?).
Capturing a snapshot of the current state of the use of digital language learning resources in the UK provides valuable and updated insights, with the ultimate goal of informing educational policies. Given that investing in technology does not automatically increase the use of these tools by educators [Yang and Huang, 2008, in Wang et al. (2019)], nor do markers such as price or popularity of a software often correlate with its quality (Callaghan and Reich, 2018; Kolak et al., 2021), we hoped that evaluation guided by the theoretical principles of four pillars of learning (Hirsh-Pasek et al., 2015) and informed by language learning theory, would inform practice and future software development.
We employed a questionnaire to collect data from second language learning teachers in the UK. The questionnaire included a rating tool that was internally constructed to reflect the theoretical frameworks relating to the principles of learning.
To ensure an appropriate and representative sample, a stratified random sampling method was employed (Dörnyei and Taguchi, 2010). Since there are no exhaustive lists of second language teachers available in the UK (due to data protection rules), lists of schools available from government websites were used as the sample frame. The total number of primary and secondary schools listed across the UK (N = 24,916) was divided into strata according to school type and location, including both private and state schools. Appropriate relative sizes were calculated for each stratum by using Cochran’s sample size formula, with additional modification for strata of a smaller size where applicable (Bartlett et al., 2001). The total stratified sample of primary and secondary schools obtained in this way was N = 3,294. The schools for this sample were randomly selected from each strata. In addition, two replacement schools were randomly chosen for each of the sampled schools during the initial sampling to account for missing responses. Schools were contacted with the information about the study to be forwarded to their language teacher(s). Based on previous studies that recruited in a similar way, the expected return rate was 10% (Dörnyei and Taguchi, 2010), which would be 329 teachers. In case of no reply, two follow-up emails were sent as reminders.
The questionnaire used was developed for the purpose of this study and consisted of five parts: (a) Demographic questions, (b) Digital environment in modern foreign language teaching, (c) Digital language learning resources, (d) Software rating and (e) Vocabulary teaching methods.
In the present study we focus on the first four parts. After the first section with introductory demographic questions, sections two and three were designed to inform on the situation in the classrooms relating to the available equipment and the patterns of use of digital language learning resources. The fourth section consisted of a software rating instrument. The following paragraphs describe each of the questionnaire sections in more detail.
The first section consisted of eight demographic questions which capture the properties of the sample and provide information for comparisons between different groups. The questions were designed to inform about the school-or teacher-level variables, such as the type and location of school or age and experience of the teacher. The questions were structured as multiple-choice questions.
The questions in the second section related to the digital language learning environment in modern foreign language classrooms and the equipment available to language teachers. Studies have shown that lack of resources in terms of institutional and infrastructure issues are consistently regarded as one of the major barriers to implementation of digital tools in education (Kaimara et al., 2021; Sánchez-Mena and Martí-Parreño, 2017; Timotheou et al., 2023). Therefore, through nine multiple-choice and Likert-type questions this section explored the language classroom equipment, as well as the frequency of teachers’ and students’ access to particular tools.
The third part narrowed down to specific language learning resources and their use. There are different ways of categorizing educational software used in second language learning and teaching, ranging from the age of the intended user, to their content or type of software. For the purpose of this questionnaire, a part of the taxonomy by Rosell-Aguilar (2017) and Reinders and Pegrum (2015) was adopted, in which differentiation is made between applications designed specifically for language learning (“dedicated”) and applications designed for a different purpose that serve as a tool for language learning (“generic”). Furthermore, to achieve a more fine-grained distinction, the group of software designed for language learning was divided into those intended for individual use and the those intended specifically for classroom use. A separate category was formed around the use of social media apps for language learning as they have also been explored as a useful tool both within language learning research and practice (Reinhardt, 2019). For each of the categories there was a section consisting of four open-ended questions where the respondents were asked to name different software that they use, as well as Likert-type question about the frequency of use. Additionally, there was an open-ended question about the software from different categories that the participants discontinued to use and the reason for discontinuing. Those answers were coded to analyse the most common obstacles to software use in teaching.
The fourth part consisted of the rating tool and focused on the quality ratings of individual applications or software used. Participants were instructed to choose from language learning software that they listed in the previous sections the ones that they are most familiar with. Since it has been estimated that the rapidly evolving market makes it practically impossible to evaluate every educational app (Starke et al., 2020), this study focused on the ones that are most relevant in terms of their actual use among practitioners.
Creation of this evaluation tool was guided by McMurry et al. (2016), who issued a call to improve the evaluation tools in CALL by following standards set by the field of formal evaluation. In their work they introduce the framework derived from the formal evaluators such as those of the American Evaluation Association (AEA) and use it to review the two of the most prominent CALL evaluation frameworks by Hubbard (2011) and Chapelle (2001). They suggest that in creating a CALL evaluation tool the following steps should be considered: (a) identifying the evaluand, (b) identifying stakeholders, (c) determining the purpose of the evaluation, (d) selecting an evaluation type, (e) setting evaluation criteria, (f) asking evaluation questions, (g) collecting and analysing the data, (h) reporting findings and implications, and (i) evaluating the evaluation.
In setting the evaluation criteria of the tool, the principle-based approach was followed (Jamieson et al., 2004), meaning that the development of the evaluation tool was guided by the pre-defined theoretical principles. These were operationalized through a set of questions forming specific variables. The questions were based on the theoretical accounts of language learning and educational software design which was described previously (section 1.1.1.). The structure reflecting the learning principles is outlined in Figure 1.
Figure 1. The overview of the subcomponents of the two main sections that contribute to the final rating score.
In terms of educational design, the questionnaire investigated whether the software follow the principles defined by Hirsh-Pasek et al. (2015). In this questionnaire they were addressed through eight Likert-type questions corresponding to the four pillars of learning; whether the child is active during the use of the software, if the software promotes engagement, if the learning is meaningful and connects to childrens’ experiences and if it promotes interactions with characters or people. The accuracy of statements describing how the software features reflect the four principles was rated (e.g., ‘The topics in the software are relevant to learner’s experience’).
Language learning features relating to input, output, interaction and practice were assessed through Likert-type questions that reflected the theoretical background of each of the components. The goal was to evaluate how comprehensively the software manages to address the concepts crucial for successful language learning. For input, the questions addressed contextualization of the language input (whether words are presented in isolation or in context), appropriate complexity (if there is a gradual increase in difficulty) and input modalities (if the input is presented through multiple modalities). Output was addressed through questions about the software providing opportunities for producing output and whether this is represented in different forms (speaking, writing). Regarding interaction, it was examined whether the software enables interactions (real or simulated) and feedback that is meaningful and constructive. Finally, rehearsal was rated through questions about the opportunities to rehearse and recycle the learned materials, as well as tracking of the learners’ progress. Additional table with an description and sources for each of the variables can be found in the Supplementary Appendix section.
To evaluate and ensure the appropriateness of the questionnaire, language teachers and researchers in the field of L2 learning were consulted throughout the process. In the preparation stages we conducted two experts to obtain their insights. After constructing the questionnaire, pre-testing validation through expert reviews (N = 4) was conducted to ensure that the items are clear, relevant and representative of the intended constructs. This process resulted mostly in changes regarding wording that improved the representatives of questions for specific contexts.
Recruitment took place through online communication. Initial emails with Invitation Letters and survey links attached were sent out in February 2022 to selected schools’ and headteachers’ email addresses, which were publicly available in the gov.uk online registries or on the schools’ websites. They were asked to forward the emails to all the foreign language teachers in their school, who were invited to take part by following an attached link. Two follow-up emails with reminders were sent a month apart. The questionnaire was hosted on the Qualtrics platform. The questionnaire took 30 min or less to complete.
The final number of the respondents included in the analysis was 118 language teachers from primary and secondary schools across the UK, representing a response rate of 3.6%. The data from participants who completed at least the first two sections were included in the analysis. The detailed demographic data are listed in the Table 1.
It was first investigated if the classrooms have the basic infrastructure to support the use of technology in teaching, as this is commonly mentioned as one of the frequently occurring barriers to use (Sánchez-Mena and Martí-Parreño, 2017; Timotheou et al., 2023). The results showed that the classrooms in our sample are well-equipped, containing the basic prerequisites for implementing software for classroom activities (see Figure 2; Table 2). The average number of digital devices reported is between 5 and 6 and there were no classrooms in the sample not equipped with at least some kind of technology accessible to teachers. Most of the respondents reported that their classrooms have Internet connection (94.1%) and sound speakers (89%) and over half of the participants indicated that they also have an interactive whiteboard, projector and a PC computer (Figure 2). These items together create a basic set-up for the classroom use of software.
Figure 2. Bar chart showing the percent of classrooms that have access to particular digital equipment and if they had been used over the last month.
The availability of necessary prerequisites for the classroom use of software is reflected in high levels of usage. In over 85% of cases the main equipment has been reported to be in use “every day.” In contrast, classrooms are not equipped at a similar level with technologies for individual use, such as tablets and smartphones, resulting naturally in their lower general frequency of use. However, even when available, they are used less frequently. Particular devices and their frequencies of use can be found in the Table 2.
Participants were asked to list the names of the software they use. The results showed that language teachers actively use and are familiar with a large number of digital resources. Collectively, teachers identified over 100 different types of software that they use now or have used in the past. Out of the participants who use digital learning resources, more than a half use at least 3 different types of learning software. One third use 4 different software types and 10% listed that they use more than 5. Given a great diversity of software utilized, the questions were grouped to focus on two large categories of software. One group of questions addressed specific language learning software and the other non-specific types of software that are being used for language learning, but it is not their main purpose. The most used language learning software was Quizlet, with 27 occurrences, followed by Linguascope (N = 24). When asked about the generic resources used in the lessons by far the most frequent response was YouTube. The top 10 digital language learning resources from each category are shown in Table 3. The responses illustrate some overlap in the software listed in both groups (marked by an asterisk). While until recently the classification of software and applications used in second language learning grouped them into language specific and generic (Reinders and Pegrum, 2015; Rosell-Aguilar, 2017), responses in this survey indicate that this binary classification might no longer be applicable. Rather, educational platforms appear to be a new tool that supports learning in a different way than traditional apps and software, by combining aspects of both language-specific and generic software. They offer customizable templates, that can be used for any subject, but also include language-specific features. They also allow teachers to modify and create content for language learning, while maintaining general educational frameworks. For example, while Kahoot was not designed specifically for language learning, it is widely used in language classrooms possibly because teachers can create language-focused games and activities using its general quiz format. Similarly, Quizlet began as a flashcard tool, but evolved to include language-specific features which can be implemented into classrooms.
When it comes to specific language learning software, around one quarter of respondents (24.5%) use them every day and 31.1% use them on a weekly basis – from 1 to 4 times per week. Almost 20% of teachers use them on a monthly basis (between 1 and 3 times per month). 14.2% of teacher use them a few times per year and only 10.4% never use them.
The second group of software, language non-specific software, is used slightly less frequently. Compared to specific language learning software, less respondents answered that they use them on a daily basis. Mostly they are used several times per week, ranging from 1 to 2 times per week (25.8%) to 3–4 times per week (15.7%). A large number of participants use them up to 3 times a month (21.3%). A smaller number of participants uses them less frequently, ranging from once per month (9%), over few times per year (10.1%) to never (3.4%).
When comparing the distributions of frequencies, it can be noticed that the language learning software are more frequently used on a daily basis, whereas the non-specific software are usually used on a weekly or bi-weekly basis.
For every type of app, the participants were also asked about the software that they started to use, but discontinued using, and the main reasons for this. The answers were open-ended and analyzed in a qualitative way through the use of codes and themes identified manually through the common responses. The results showed that the main obstacles to their use were expenses, quality of the content and the overall usability of the software (Table 4).
Table 4. Table showing most frequent reasons for discontinuing the use of language learning software that respondents named.
To understand what drives the use of software in language learning classrooms, an ordinal logistic regression model was employed, due to its ability to handle ordinal data (Harrell, 2015). In the present study, the dependent variable was the response to question about the frequency of use of language learning software, scored on a 7-point Likert scale, making ordinal logistic regression the most appropriate analysis choice. The examined predictors included respondents’ age, years of experience, availability of equipment, and school type (primary or secondary), which were all found to be related to software use in previous studies (Kaimara et al., 2021; Timotheou et al., 2023; Vermeulen et al., 2017; Wang et al., 2019). Overall, the full model was significant when compared to the null model (likelihood ratio test comparing full and null model: χ2 = 36.28, df = 4, p < 0.001). With the significance criterion of α < 0.05 for p-values, the results indicated that age, experience and equipment did not affect the use of language learning software (see Table 5). The only variable that emerged as a significant predictor was school type, showing that software is used significantly more in secondary than in primary schools. The size of this effect was estimated using the odds ratio (OR = 4.68), indicating a large effect (Chen et al., 2010).
The reason behind age and teaching experience not influencing technology adoption might be due to the institutional and pedagogical expectation playing a more dominant role in driving software use than individual characteristics. Additionally, growing immersion of digital tools in education may be diminishing age-related and experience-related barriers. As digital resources become more intuitive, standardized and widely integrated, teachers across all age groups and experience levels may feel increasingly comfortable using them, reducing the previously observed generational divide. On the other hand, the reason might be the opposite – despite the frequent assumption that the younger users will be more advanced when it comes to new digital technologies, Reddy et al. (2022a) warn that a gap exists between personal and academic use of technology. Although younger educators might be more frequent users of digital technologies in their personal time, this might not be reflected in their adoption of digital tools in their classrooms. Regarding availability of equipment, it may not be a strong factor in contexts where the infrastructure is already well-developed. Since most schools in our study had adequate technological resources, it might have not come up as a differentiating factor driving the software use.
In the software rating section participants chose one language learning software type that they are most familiar with from the list that they provided in the previous sections. Eighty-one participant responded to this section, rating the features of the software on a scale from 1 to 7. Since this rating instrument was developed with language learning software in mind, excluded from the list were software non-specific to language learning (e.g., YouTube), resulting in 74 ratings.
Since this part of the questionnaire was scale-based, we conducted validity analysis upon collecting the data. Validity and internal consistency of the questionnaire were assessed using Cronbach’s Alpha. Three out of eight scales (scales measuring output, interaction and practice) demonstrated good reliability, exceeding the α = 0.7 threshold (α = 0.75, α = 0.73 and α = 0.73). Three additional scales (scales measuring input, meaningfulness and social components) fell close below the threshold, indicating moderate consistency (α = 0.59, α = 0.66 and α = 0.59). Two scales (scales ‘active’ and ‘engagement’) fell further from the threshold (α = 0.3 and α = 0.26), indicating the need for further refinement of this part in future research.
First of all, the ratings for all of the rated software were combined to show an overview of the properties of currently used language learning software. The mean ratings for each of the components for both general and language learning principles are shown in the Supplementary Appendix B and the overview shown in Figure 3.
The evaluation of the software revealed a range of mean scores for the rated categories from 3.20 to 5.53 out of a possible 7. When analyzing the results within the framework of language and general learning principles, distinct patterns emerged. In the domain of language principles Input produced the highest ratings (M = 5.53, SD = 1.04), while both Output (M = 4.30, SD = 1.68) and Interaction (M = 4.30, SD = 1.56) received poor ratings. Within the general learning principles, Engagement stood out (M = 5.53, SD = 1.30) as a particularly strong aspect, while the Social learning principle scored the lowest (M = 3.20, SD = 1.92).
In this section, the ratings of all the individual software which were chosen by at least two respondents were analyzed, which resulted in 62 ratings of 11 different software types.
In the final table (Table 6), the ratings are summarized to create a final total score. The software with the highest overall score was Language Angels.
Table 6. Table showing total ratings according to individual software in descending order by the final score.
The study also compared the software according to their pricing, since previous studies showed that price did not guarantee quality among the educational apps downloaded from the mobile app stores (Callaghan and Reich, 2018; Kolak et al., 2021). To establish if there is a significant difference between the software according to their cost the software was grouped into free versus paid and a Wilcoxon rank-sum test was used. The outcome variables were the scores on the subcomponents of the questionnaire that were relating either to the theory of general learning principles, language learning principles or teachers’ satisfaction. The results showed that there was no difference in ratings between free and paid software on any of the measured subcomponents (all p > 0.05). Detailed analysis outputs can be found in the Supplementary Appendix C. These findings suggest that price alone does not systematically influence educational quality. This is in line with findings from Kolak et al. (2021), who found that the difference between free and paid educational apps was not present on the measures of content quality, but in the frequency of animations and elements on screen. This suggests that the price cannot be seen as an indicator of the educational quality and it might instead be driven by branding, marketing or additional features focused on entertainment.
The results of the survey provide insights into the state of the use of language learning software and their quality vis-a-vis ratings of dimensions pertaining to theoretical principles of language learning and learning in general. In relation to our aims, the study identified the patterns of use of language learning software across the UK classrooms in terms of highlighting specific software used, their frequency of use, as well as their quality.
When it comes to understanding the drivers of digital software use, the results are in contrast with previous findings. Although it was expected that both the school-level and teacher-level variables would predict the frequency of use, this was not the case among this sample. The only predictor that emerged as significant was type of school, but not in the direction expected based on the previous research. Whereas Wang et al. (2019) reports how, in China, the teachers in primary schools used digital tools more frequently and believed in their efficiency more than their secondary school colleagues, results of this study were in the opposite direction, with higher reports of software use in the secondary schools compared to primary schools. This might be due to the fact that in the UK, and in contrast to education policy in many countries, there is generally less emphasis put on language teaching in primary schools. These findings confirm the importance of understanding different contexts when evaluating use and quality of language learning software (Jamieson and Chapelle, 2010). At the same time the findings reveal that classrooms in our sample are well-equipped with the infrastructure necessary for integrating technology into language teaching, potentially indicating that one of the major technical barriers to use found in other contexts and countries (Sánchez-Mena and Martí-Parreño, 2017; Timotheou et al., 2023) does not apply to the primary and secondary schools in the UK.
The availability of technologies for language learning in the classroom is also reflected in the high frequencies of use, suggesting a consistent incorporation of digital tools into language education. With this growing number of CALL resources available, stakeholders need to become increasingly critical when choosing the most appropriate ones. Many parameters should be considered when deciding on which resources to use in language classrooms, such as cost, technical requirements or usability. However, one of the crucial aspects that is frequently not sufficiently explored, is the pedagogical and linguistic soundness of the software (O’Brien, 2020). By creating a theory-based rating tool, this study enabled a fine-grained understanding of the strengths and weaknesses of digital language learning software. This becomes particularly relevant in light of the mismatch found between the quality of software and the frequency of use. Specifically, the software which obtained the highest score on these principles was not among the top ten most used software, and the same is true for four out of the top five rated software (Tables 6, 7). This is in line with results from Kolak et al. (2021), who also discovered that most used software were not necessarily the best ones in terms of their educational design. A further mismatch that was found between quality of software and price, which is also in line with other studies on educational applications (Callaghan and Reich, 2018; Kolak et al., 2021), showing that price did not guarantee quality. Given these findings, schools and educators should exercise caution and be mindful when choosing the language learning software to use, taking a more informed approach and prioritizing evidence-based evaluations. Both educational institutions and teachers could benefit from structured guidelines or workshops focused on assessing the pedagogical soundness of digital language learning tools to ensure that high-quality and research-based tools are more widely recognized and adopted.
Table 7. Table showing the most frequently used and highest rated software in ascending order, from most frequently used to the less frequently used. Additionally, the general properties of each software are listed.
The ratings in this study indicated that current software used shows strength in the areas of Input and Engagement, but weaknesses in the domains of Output, Interaction and Social learning. The high scores for Input and Practice are in line with the Chapelle (2008) observation that second language theories relating to the nature of input and practice, such as Input Processing (Krashen, 1994) and Skill Acquisition theory (DeKeyser, 2007) offer clearer and more applicable guidelines that are easier to implement into software design. The low scores obtained in the area of Social learning and Output corresponds to drawbacks identified 5–10 years ago (Burston, 2015; Heil et al., 2016), and demonstrate that the majority of software still remain predominately behaviorist in nature, despite the pedagogical shift from behaviorism toward more communicative-based and learner-centred approaches such as constructivism. To change this, the software developers should aim to provide more opportunities for students to create output and contextualize language through including more components that mimic social interactions. This could include implementing AI-driven conversational partners, enhanced speech recognition for pronunciation feedback or collaborative tasks that engage students in communication with peers.
The current state of the language learning software can still be summarized in an observation that the software are not entirely living up to their potential (Burston, 2015; Heil et al., 2016). The key to achieving their full potential would be in becoming more innovative and collaborative, thus aligning better with language and educational principles. Until this is achieved, the educators should remain mindful of the limitations of the language learning software and compensate by putting more emphasis on those areas through other classroom activities.
The wide array of language learning software now available in a rapidly changing market also marks changes to how one can conceptualize software. For example, up until recently the software and applications used in second language learning have been classified into language specific and generic (Reinders and Pegrum, 2015; Rosell-Aguilar, 2017; Shadiev and Yang, 2020). This distinction is questioned by the responses in this survey with educational platforms emerging as comprehensive systems that support learning on a broader scale than traditional apps and software. With the technology that has evolved to include multiple features, they outgrew the existing categories (Shadiev and Yang, 2020). For example, the recently emerged platforms, situated at the intersection of language-specific and generic software, offer adaptive and engaging learning environments, empowering teachers to tailor content while maintaining a generic core. The emergence of template-sharing communities providing pre-made language learning products that can be easily adapted further complicates the distinction between the two traditional types of software, as well as evaluation of their quality. This results in less adaptation requirements and almost a ready-made language learning product that still retains the possibility of modifying the content. The flexibility with the content creation could potentially be one of the major reasons for their success, especially seeing how the participants in the survey listed “low quality of content” as one of the main reasons against the use of some software. Notably, the most frequently used software all offer users the ability to create or modify content, underscoring the importance of customization in effective language learning tools.
One limitation of the present study is the low response rate from a large cohort of teachers sampled across the UK. This may be due to self-selection and great demands that teachers have in their workloads, limiting time. This potentially introduces bias by having teachers who are less engaged with technology underrepresented. Future research could explore additional strategies to enhance response rates, such as providing financial incentives. Additionally, Dörnyei and Taguchi (2010) propose complementing questionnaire studies with follow-up interviews to address and further explore the findings from the questionnaire by using a “sequential exploratory design.” Moreover, in addition to the judgemental evaluation analysis done in this study, Chapelle (2001) suggests that software evaluation can benefit from being complemented by the analysis of learning outcomes. Future studies might also focus on describing the use of specific software features identified in this study, thus deepening our understanding of the software use in the classroom context. It would be also insightful to collect more information on the teachers’ digital literacy and information literacy levels, as these constructs are emerging as crucial in accessing and using digital media (Reddy et al., 2022a; Reddy et al., 2022b). Furthermore, the data collected here could be used to further enhance validity and reliability of the software rating tool.
Overall, the results of the survey highlight the imperative for future software development to align more closely with social and interactive learning principles, emphasizing the need for innovation to bridge the gap between theory and current software offerings. Insights from those delivering language learning in the classroom merit careful consideration by relevant stakeholders to establish quality standards for educational software, ensuring alignment with pedagogical principles. Moreover, developers need to work hand-in-hand with teachers to ensure that applications are developed with maximum efficacy, and in line with leading pedagogical and educational principles. Furthermore, understanding teachers’ habits and preferences, as well as challenges related to specific software types, can inform policies aimed at optimizing resource allocation and training for teachers.
The raw data supporting the conclusions of this article will be made available by the authors, without undue reservation.
The studies involving humans were approved by the School of Psychology Research Ethics Subcommittee; University of East Anglia. The studies were conducted in accordance with the local legislation and institutional requirements. The participants provided their written informed consent to participate in this study.
PJ: Conceptualization, Data curation, Formal analysis, Investigation, Methodology, Software, Validation, Visualization, Writing – original draft, Writing – review & editing. KC: Conceptualization, Funding acquisition, Investigation, Methodology, Project administration, Resources, Supervision, Validation, Writing – review & editing.
The author(s) declare that financial support was received for the research, authorship, and/or publication of this article. This work was supported by the European Union’s Horizon 2020 Research and Innovation Programme under the Marie Sklodowska-Curie Actions grant agreement no 857897.
The authors declare that the research was conducted in the absence of any commercial or financial relationships that could be construed as a potential conflict of interest.
The author(s) declared that they were an editorial board member of Frontiers, at the time of submission. This had no impact on the peer review process and the final decision.
The authors declare that no Gen AI was used in the creation of this manuscript.
All claims expressed in this article are solely those of the authors and do not necessarily represent those of their affiliated organizations, or those of the publisher, the editors and the reviewers. Any product that may be evaluated in this article, or claim that may be made by its manufacturer, is not guaranteed or endorsed by the publisher.
The Supplementary material for this article can be found online at: https://www.frontiersin.org/articles/10.3389/feduc.2025.1532802/full#supplementary-material
American Academy of Arts and Sciences, British Academy, Academy of the Social Sciences in Australia, Australian Academy of the Humanities, and Royal Society of Canada. (2020). The importance of languages in global context: an international call to action. Available online at: https://www.amacad.org/sites/default/files/media/document/2020-11/Joint-Statement-on-Languages.pdf (Accessed September 24, 2024).
Bartlett, J. E., Kotrlik, J. W., and Higgins, C. C. (2001). Organizational research: determining appropriate sample size in survey research. Inf. Technol. Learn. Perform. J. 19, 43–50.
Brecht, R. D. (2015). America’s languages: challenges and promise. American Academy of Arts and Sciences. Available online at: https://www.amacad.org/multimedia/pdfs/AmericasLanguagesChallengesandPromise.pdf (Accessed September 24, 2024).
Burston, J. (2015). Twenty years of MALL project implementation: a meta-analysis of learning outcomes. ReCALL 27, 4–20. doi: 10.1017/S0958344014000159
Busse, V. (2017). Plurilingualism in Europe: exploring attitudes toward English and other European languages among adolescents in Bulgaria, Germany, the Netherlands, and Spain. Mod. Lang. J. 101, 566–582. doi: 10.1111/modl.12415
Callaghan, M. N., and Reich, S. M. (2018). Are educational preschool apps designed to teach? An analysis of the app market. Learn. Media Technol. 43, 280–293. doi: 10.1080/17439884.2018.1498355
Chapelle, C. (2001). Computer applications in second language acquisition: Foundations for teaching, testing and research. Cambridge, UK: Cambridge University Press.
Chapelle, C. A. (2008). “Computer assisted language learning” in The handbook of educational linguistics. eds. B. Spolsky and F. M. Hult (Malden, MA: Blackwell Publishing Ltd.).
Chen, H., Cohen, P., and Chen, S. (2010). How big is a big odds ratio? Interpreting the magnitudes of odds ratios in epidemiological studies. Commun. Statistics Simulation Computation 39, 860–864. doi: 10.1080/03610911003650383
Collen, I. (2020). Language trends 2020: language teaching in primary and secondary schools in England. British Council. Available online at: https://www.britishcouncil.org/sites/default/files/language_trends_2020_0.pdf (Accessed June 17, 2024).
Costa, P., and Albergaria-Almeida, P. (2015). The European survey on language competences: measuring foreign language student proficiency. Procedia Social Behavioral Sciences 191, 2369–2373. doi: 10.1016/j.sbspro.2015.04.255
DeKeyser, R. (2007). Skill acquisition theory. In B. PattenVan and J. Williams (Eds.), Theories in second language acquisition: An introduction (pp. 97–113). New Jersey: Lawrence Erlbaum Associates, Inc.
Dörnyei, Z., and Taguchi, T. (2010). Questionnaires in second language research: Construction, administration, and processing. 2nd Edn. New York, NY: Routledge.
Dubiner, D. (2018). Second language learning and teaching: from theory to a practical checklist. TESOL J. 10:e398. doi: 10.1002/tesj.398
Egbert, J., Huff, L., McNeil, L., Preuss, C., and Sellen, J. (2009). Pedagogy, process, and classroom context: integrating teacher voice and experience into research on technology-enhanced language learning. Mod. Lang. J. 93, 754–768. doi: 10.1111/j.1540-4781.2009.00971.x
Elaish, M. M., Hussein, M. H., and Hwang, G.-J. (2023). Critical research trends of mobile technology-supported English language learning: a review of the top 100 highly cited articles. Educ. Inf. Technol. 28, 4849–4874. doi: 10.1007/s10639-022-11352-6
Ellis, N. C. (2007). The associative-cognitive CREED. In B. PattenVan and J. Williams (Eds.), Theories in second language acquisition: An introduction (pp. 77–95). Mahwah, NJ: Erlbaum.
European Commission (2018). Commission staff working document accompanying the document proposal for a council recommendation on a comprehensive approach to the teaching and learning of languages. Brussels: Publications Office of the European Union.
Gangaiamaran, R., and Pasupathi, M. (2017). Review on use of mobile apps for language learning. Int. J. Appl. Eng. Res. 12, 11242–11251.
Gatbonton, E., and Segalowitz, N. (2005). Rethinking communicative language teaching: a focus on access to fluency. Can. Mod. Lang. Rev. 61, 325–353. doi: 10.3138/cmlr.61.3.325
Goksu, I., Ozkaya, E., and Gunduz, A. (2020). The content analysis and bibliometric mapping of CALL journal. Comput. Assist. Lang. Learn. 35, 2018–2048. doi: 10.1080/09588221.2020.1857409
Harrell, F. E. (2015). “Ordinal Logistic Regression” in Regression modeling strategies. Springer series in statistics (Cham: Springer).
Heil, C. L., Wu, J. S., Lee, J. J., and Schmidt, T. (2016). A review of Mobile language learning applications: trends, challenges, and opportunities. Euro Call Review 24, 32–50. doi: 10.4995/eurocall.2016.6402
Hirsh-Pasek, K., Zosh, J. M., Golinkoff, R. M., Gray, J. H., Robb, M. B., and Kaufman, J. (2015). Putting education in “educational” apps: lessons from the science of learning. Psychol. Sci. Public Interest 16, 3–34. doi: 10.1177/1529100615569721
Howard, S. K., Chan, A., and Caputi, P. (2014). More than beliefs: Subject areas and teachers’ integration of laptops in secondary teaching. Br. J. Educ. Technol. 46, 360–369. doi: 10.1111/bjet.12139
Hubbard, P. (2011). “Evaluation of courseware and websites” in Present and future promises of CALL: From theory and research to new directionsin foreign language teaching. eds. L. Ducate and N. Arnold (CALICO: San Marcos), 407–440.
Hubbard, P., and Levy, M. (2016). “Theory in computer-assisted language learning research and practice” in The Routledge handbook of language learning and technology. eds. F. Farr and L. Murray (London, UK: Routledge) 24–38.
Jamieson, J., and Chapelle, C. A. (2010). Evaluating CALL use across multiple contexts. System 38, 357–369. doi: 10.1016/j.system.2010.06.014
Jamieson, J., Chapelle, C., and Preiss, S. (2004). Putting principles into practice. ReCALL 16, 396–415. doi: 10.1017/S0958344004001028
Kaimara, P., Fokides, E., Oikonomou, A., and Delianis, I. (2021). Potential barriers to the implementation of digital game-based learning in the classroom: pre-service teachers’ views. Tech. Know Learn 26, 825–844. doi: 10.1007/s10758-021-09512-7
Kamasak, R., Özbilgin, M., Atay, D., and Kar, A. (2021). “The effectiveness of mobile-assisted language learning (MALL): a review of the extant literature” in Handbook of research on determining the reliability of online assessment and distance learning. eds. A. Moura, P. Reis, and M. Cordeiro (Hershey, PA: IGI Global), 194–212.
Kolak, J., Norgate, S. H., Monaghan, P., and Taylor, G. (2021). Developing evaluation tools for assessing the educational potential of apps for preschool children in the UK. J. Child. Media 15, 410–430. doi: 10.1080/17482798.2020.1844776
Krashen, S. (1994). “The input hypothesis and its rivals” in Implicit and explicit learning of languages. ed. N. Ellis (UK: Academic Press), 45–77.
Lanvers, U., and Coleman, J. A. (2017). The UK language learning crisis in the public media: a critical analysis. Lang. Learn. J. 45, 3–25. doi: 10.1080/09571736.2013.830639
Li, P., and Lan, Y.-J. (2022). Digital language learning (DLL): insights from behavior, cognition, and the brain. Biling. Lang. Congn. 25, 361–378. doi: 10.1017/S1366728921000353
Long, M. H. (1996). “The role of linguistic environment in second language acquisition” in Handbook of second language acquisition. eds. W. Ritchie and T. K. Bhatia (San Diego: Academic Press), 413–468.
Ma, Q., and Yan, J. (2022). How to empirically and theoretically incorporate digital technologies into language learning and teaching. Biling. Lang. Congn. 25, 392–393. doi: 10.1017/S136672892100078X
McMurry, B. L., West, R. E., Rich, P. J., Williams, D. D., Anderson, N. J., and Hartshorn, K. J. (2016). An evaluation framework for CALL. Electronic J. English Second Lang 20, 1–31.
Meyer, M., Zosh, J. M., McLaren, C., Robb, M., McCafferty, H., Golinkoff, R. M., et al. (2021). How educational are ‘educational’ apps for young children? App store content analysis using the four pillars of learning framework. J. Child. Media 15, 526–548. doi: 10.1080/17482798.2021.1882516
Mihaylova, M., Gorin, S., Reber, T. P., and Rothen, N. (2022). A Meta-analysis on Mobile-assisted language learning applications: benefits and risks. Psychologica Belgica 62, 252–271. doi: 10.5334/pb.1146
Myles, F., Tellier, A., and Holmes, B. (2019). Embedding languages policy in primary schools in England: Summary of the RiPL white paper proposing solutions. [White Paper]. Multilingualism: Empowering Individuals, Transforming Societies (MEITS). doi: 10.17863/CAM.41901
O’Brien, M. G. (2020). Facilitating language learning through technology: A literature review on computer-assisted language learning, Canadian Association of Second Language Teachers (CASLT) Available at: https://www.caslt.org/wp-content/uploads/2021/12/sample-call-lit-review-en.pdf
Phillips, D. C. (1995). The good, the bad, and the ugly: the many faces of constructivism. Educ. Res. 24, 5–12. doi: 10.3102/0013189X024007005
Reber, T. P., and Rothen, N. (2018). Educational app-development needs to be informed by the cognitive neurosciences of Learning & Memory. NPJ Science Learn 3:22. doi: 10.1038/s41539-018-0039-4
Reddy, P., Chaudhary, K., Sharma, B., and Chand, R. (2022a). Talismans of digital literacy: a statistical overview. Electronic J. e-Learning 20, 570–587. doi: 10.34190/ejel.20.5.2599
Reddy, P., Sharma, B., Chaudhary, K., Lolohea, O., and Tamath, R. (2022b). Information literacy: a desideratum of the 21st century. Online Inf. Rev. 46, 441–463. doi: 10.1108/OIR-09-2020-0395
Reinders, H., and Pegrum, M. (2015). “Supporting language learning on the move. An evaluative framework for mobile language learning resources” in Second Language Acquisition Research and materials development for language learning. ed. B. Tomlinson (London, UK: Taylor & Francis), 116–141.
Reinhardt, J. (2019). Social media in second and foreign language teaching and learning: blogs, wikis, and social networking. Lang. Teach. 52, 1–39. doi: 10.1017/S0261444818000356
Rončević Zubković, B., Pahljina-Reinić, R., and Kolić-Vehovec, S. (2022). Predictors of ICT use in teaching in different educational domains. Humanities Today 1, 75–91. doi: 10.2478/htpr-2022-0006
Rosell-Aguilar, F. (2017). State of the app: a taxonomy and framework for evaluating language learning mobile applications. CALICO J. 34, 243–258. doi: 10.1558/cj.27623
Sánchez-Mena, A., and Martí-Parreño, J. (2017). Drivers and barriers to adopting gamification: Teachers’ perspectives. Electronic J. e-Learning 15, 434–443.
Shadiev, R., and Wang, X. (2022). A review of research on technology-supported language learning and 21st century skills. Front. Psychol. 13:897689. doi: 10.3389/fpsyg.2022.897689
Shadiev, R., and Yang, M. (2020). Review of studies on technology-enhanced language learning and teaching. Sustain. For. 12:524. doi: 10.3390/su12020524
Shaughnessy, M. (2003). CALL, commercialism and culture: inherent software design conflicts and their results. ReCALL 15, 251–268. doi: 10.1017/S0958344003000922
Starke, A., Leinweber, J., and Ritterfeld, U. (2020). “Designing apps to facilitate first and second language acquisition in children” in International perspectives on digital media and early literacy: The impact of digital devices on learning. eds. K. J. Rohlfing and C. Müller-Brauers (Language Acquisition and Social Interaction: Routledge).
Sung, Y., Chang, K., and Yang, J. M. (2015). How effective are mobile devices for language learning? A meta-analysis. Educ. Res. Rev. 16, 68–84. doi: 10.1016/j.edurev.2015.09.001
Sweeney, P., and Moore, C. (2012). Mobile apps for learning vocabulary: categories, evaluation and design criteria for teachers and developers. Int. J. Computer Assisted Language Learning Teaching 2, 1–16. doi: 10.4018/ijcallt.2012100101
Szabó, F., and Csépes, I. (2023). Constructivism in language pedagogy. Hungarian Educ. Res. J. 13, 405–417. doi: 10.1556/063.2022.00136
Tauson, M., and Stannard, L. (2018). EdTech for learning in emergencies and displaced settings: A rigorous review and narrative synthesis. Save the Children. Available online at: https://resourcecentre.savethechildren.net/node/13238/pdf/edtechlearning.pdf (Accessed May 15, 2024).
Timotheou, S., Miliou, O., Dimitriadis, Y., Sobrino, S. V., Giannoutsou, N., Cachia, R., et al. (2023). Impacts of digital technologies on education and factors influencing schools’ digital capacity and transformation: a literature review. Educ. Inf. Technol. 28, 6695–6726. doi: 10.1007/s10639-022-11431-8
Tinsley, T., and Doležal, N. (2018). Language trends 2018: Language teaching in primary and secondary schools in England. London, UK: British Council.
Tomasello, M. (2003). Constructing a language: a usage-based theory of language acquisition. Cambridge, MA: Harvard University Press.
Van Patten, B. (1993). Grammar teaching for the acquisition-rich classroom. Foreign Lang. Ann. 26, 435–450. doi: 10.1111/j.1944-9720.1993.tb01179.x
Vermeulen, M., Kreijns, K., van Buuren, H., and Van Acker, F. (2017). The role of transformative leadership, ICT-infrastructure and learning climate in teachers’ use of digital learning materials during their classes. Br. J. Educ. Technol. 48, 1427–1440. doi: 10.1111/bjet.12478
Vygotsky, L. S. (1978). Mind in society: The development of higher psychological processes. Massachusetts: Harvard University Press.
Wang, J., Tigelaar, D. E., and Admiraal, W. (2019). Connecting rural schools to quality education: rural teachers’ use of digital educational resources. Comp. Human Behav. 101, 68–76. doi: 10.1016/j.chb.2019.07.009
Yang, S. C., and Huang, Y.-F. (2008). A study of high school English teachers’ behavior, concerns and beliefs in integrating information technology into English instruction. Comput. Human Behav. 24, 1085–1103. doi: 10.1016/j.chb.2007.03.009
Yang, M. K., and Shadiev, R. (2019). Review of research on Technologies for Language Learning and Teaching. Open J. Soc. Sci. 7, 171–181. doi: 10.4236/jss.2019.73014
Keywords: computer assisted language learning, foreign language education, technology, digital educational resources, evaluation
Citation: Janjić P and Coventry KR (2025) Digital language learning resources: analysis of software features and usage patterns in UK schools. Front. Educ. 10:1532802. doi: 10.3389/feduc.2025.1532802
Received: 22 November 2024; Accepted: 24 February 2025;
Published: 14 March 2025.
Edited by:
Bibhya Sharma, University of the South Pacific, FijiReviewed by:
Kaylash Chand Chaudhary, University of the South Pacific, FijiCopyright © 2025 Janjić and Coventry. This is an open-access article distributed under the terms of the Creative Commons Attribution License (CC BY). The use, distribution or reproduction in other forums is permitted, provided the original author(s) and the copyright owner(s) are credited and that the original publication in this journal is cited, in accordance with accepted academic practice. No use, distribution or reproduction is permitted which does not comply with these terms.
*Correspondence: Paula Janjić, cC5qYW5qaWNAdWVhLmFjLnVr
Disclaimer: All claims expressed in this article are solely those of the authors and do not necessarily represent those of their affiliated organizations, or those of the publisher, the editors and the reviewers. Any product that may be evaluated in this article or claim that may be made by its manufacturer is not guaranteed or endorsed by the publisher.
Research integrity at Frontiers
Learn more about the work of our research integrity team to safeguard the quality of each article we publish.