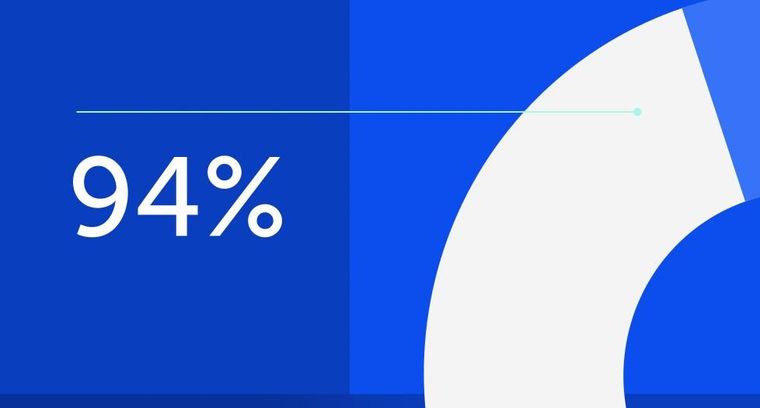
94% of researchers rate our articles as excellent or good
Learn more about the work of our research integrity team to safeguard the quality of each article we publish.
Find out more
STUDY PROTOCOL article
Front. Educ., 11 April 2025
Sec. Teacher Education
Volume 10 - 2025 | https://doi.org/10.3389/feduc.2025.1528805
Executive function refers to a set of cognitive processes essential for effectively managing complex tasks and enabling goal-directed behavior. Working memory (WM), one of the core executive functions, is the ability to temporarily hold and manipulate information. It plays an important role in various cognitive tasks and learning processes, with WM difficulties greatly affecting students’ academic performance and overall learning. Despite clinical efforts to improve WM in primary school children, results have been inconsistent. Second-generation research has shifted focus to the role of environmental factors, such as teacher-student interactions (TSIs), as a means to better support students’ WM. Based on the Teaching through interactions framework, TSIs are categorized into three domains: instructional support, emotional support, and classroom organization. This study protocol aims to (1) elucidate the theoretical foundations of each type of support and its relationship to WM, (2) provide a comprehensive overview of the intervention procedure, with a specific focus on its development, and (3) lay the groundwork for four microtrials to explore the immediate effects of instructional support, emotional support, and classroom organization provided by the teacher, on student’s WM-related problematic behaviors and performance, compared to a teaching-as-usual (TAU) control group. Each intervention includes a coaching session followed by a 4-week implementation period, during which five high-potential strategies focused on one type of support are applied at the classroom level. To achieve this, 168 teachers, together with students (aged 6–12, exhibiting WM-related problematic behaviors) and parents will be recruited to participate in the study. After the pre-tests, the participants will be randomly assigned to one of three experimental groups or the TAU group. Post-tests will be conducted after intervention implementation. The findings are expected to enhance theoretical understanding of effective WM intervention components and contribute to the development of targeted strategies to strengthen WM in educational settings.
As children advance through various developmental stages, the development and maturation of executive function (EF) plays a crucial role in shaping their ability to navigate the complex demands of their environment. EF encompasses a set of cognitive processes that support goal-driven behavior, especially in novel and complex situations (Huizinga et al., 2006). It comprises three core executive functions (EFs)—working memory (WM), inhibition, and cognitive flexibility—which form the foundation for higher-order EFs, including planning and organizing, problem-solving skills, and goal-setting (Diamond, 2013). Extensive research demonstrates the essential role of these cognitive processes in children’s learning and academic performance, particularly influencing skills in areas such as mathematics and literacy (Huizinga et al., 2018; Spiegel et al., 2021; Vandenbroucke et al., 2017b; for a review and meta-analysis, see Cortés Pascual et al., 2019).
By fostering robust EF, children acquire the cognitive skills necessary to navigate academic challenges more effectively (Diamond, 2013). Conversely, EF difficulties can result in academic delays and social developmental difficulties, including both internalizing and externalizing behaviors (Morgan et al., 2019; Riggs et al., 2003). Additionally, EF challenges are often associated with patterns observed in attention-deficit/hyperactivity disorder (ADHD; Barkley, 2015), autism spectrum disorder (ASD; Demetriou et al., 2018), and specific learning disabilities (SLD; Capodieci et al., 2023). Given the significant role of EF in children’s overall development, providing tailored support and early intervention is essential to adequately address children’s needs. Research shows that EF can be improved through targeted training interventions (Diamond and Ling, 2020). However, despite efforts to develop effective interventions, clinical approaches failed to consistently demonstrate the desired transfer effects and sustained efficacy (Schwaighofer et al., 2015; Zelazo et al., 2016; for meta-analyses, see Diamond and Ling, 2016; Melby-Lervåg and Hulme, 2013; Powers et al., 2013; Sala and Gobet, 2020; Verburgh et al., 2014).
Within second-generation intervention studies, there is increasing support for interventions integrated into real-life academic contexts (Mattera et al., 2021). Such classroom-based interventions show promise in fostering EF by combining direct training alongside teacher support, particularly through positive teacher-student interactions (TSIs). Research shows small-to-medium correlations between TSIs and EF, emphasizing the importance of positive interactions and a supportive classroom environment (for meta-analyses on associations, see Vandenbroucke et al., 2018a; Xu et al., 2024; for a systematic review on causal studies, see Sankalaite et al., 2021). However, given the rather recent shift in focus, research on TSIs and its effect on EFs is limited, therefore, warranting further research. This paper represents a preliminary effort to elucidate potential theoretical mechanisms at play concerning WM, derive TSI strategies based on these mechanisms, and test the effectiveness of TSI strategies in reducing WM problems.
WM is a cognitive ability to temporarily store and manipulate limited information, enabling real-time processing (Baddeley, 1986; Cowan, 2005). WM can be prioritized over other EFs in interventions for several reasons. First, it is the strongest predictor of academic achievement among EFs, supporting fundamental skills such as mathematics, reading, and spelling (Alloway and Alloway, 2010; Gathercole and Alloway, 2008; Peng et al., 2016, 2018; Vandenbroucke et al., 2017b; for a review and meta-analysis, see Cortés Pascual et al., 2019). Research consistently indicates that students with higher WM capacity typically demonstrate greater proficiency in managing complex academic tasks. This is particularly important for children from low socio-economic backgrounds, who often have poorer WM compared to their peers from higher socio-economic backgrounds (Chevalère et al., 2022; Peng and Kievit, 2020). Second, WM is one of the first EFs to develop (Best and Miller, 2010), with substantial growth between ages 6 and 12 and continuing into late adolescence (Carriedo et al., 2016; Diamond, 2013), offering a critical window for improvement through educational interventions (Constantinidis and Klingberg, 2016; Zelazo and Carlson, 2020). Third, WM is highly sensitive to environmental manipulation, especially through TSIs, showing small-to-medium effects compared to small and null effects for inhibition and cognitive flexibility, respectively (Vandenbroucke et al., 2018a). Finally, WM supports inhibition (Diamond, 2013; Kattner, 2021; Nyberg et al., 2009), cognitive flexibility (Blackwell et al., 2009), and higher order EFs such as planning and reasoning (Diamond and Ling, 2016; Ferguson et al., 2021). While some disagree (Barkley, 1997; Van Stockum and DeCaro, 2020), focusing on WM in interventions may yield the most significant cognitive benefits for children, optimizing resource allocation for maximum impact.
Regarding WM development, the Multicomponent Working Memory Model (Baddeley, 2010, 2012) is widely used in research to explain the different mechanisms of WM. It divides WM into three subcomponents: the phonological loop, responsible for storing and rehearsing auditory information; the visuospatial sketchpad, handling visual and spatial information; and the central executive, which directs cognitive processes, manages information flow, allocates attention, and integrates information from the other two subsystems. A fourth component, the episodic buffer, was later introduced to address the integration of WM subsystems with long-term memory (Baddeley, 2000; Baddeley et al., 2011).
In contrast, Cowan’s Embedded-Process Model (Cowan, 1999) offers an alternative view, focusing on general information processing rather than modular subsystems. This model includes four components: the central executive, long-term memory, activated memory, and the focus of attention. Unlike Baddeley’s model, Cowan’s model does not separate phonological and visuospatial information but treats them as integrated aspects of WM (Adams et al., 2018). Both models acknowledge the limited capacity of WM, its interaction with long-term memory, and incorporate a central executive that acts as a supervisory system.
This study adheres to Baddeley’s definition of WM, a predominant perspective in the field, while simultaneously taking into account the foundational principles from Cowan’s Embedded-Process Theory. Specifically, children’s WM difficulties will be assessed using two complementary methods: performance-based tests to measure visual and verbal WM (Baddeley’s model) in a controlled setting, and behavior ratings to capture WM challenges in daily life, reflecting Cowan’s view of WM as an active, context-dependent construct.
The brain regions primarily involved in WM include the (dorso-lateral) prefrontal cortex, parietal cortex, and basal-ganglia (Tamnes et al., 2013; Ullman et al., 2014). Biological maturation supports the development of these brain regions through processes like cortical thinning, myelination, and synaptic pruning, leading to more efficient information processing (Kolk and Rakic, 2022; Korzeniowski et al., 2021; Zhong et al., 2014). WM develops gradually, beginning around 9 months of age and improving steadily from 4 years through early adolescence (Best and Miller, 2010; Diamond, 2013; Gathercole et al., 2004). This steady progression is driven by the repeated use and practice of WM, along with stimulation from external factors, including the school environment.
Primary school education plays a crucial role in the development of WM by providing structured academic tasks and clear expectations, along with formal instruction. This stage often marks the onset of WM-related challenges, creating valuable opportunities for effective interventions. Specifically, direct manipulation, such as guided instruction, task repetition, and gradually increasing task difficulty, can significantly support WM development. For instance, a study demonstrated that first graders who received structured instruction showed greater WM improvement compared to peers who delayed school entry (Davidson et al., 2023; Roberts et al., 2016). Researchers attribute this improvement to the frequent repetition of lesson content, often referred to as “rote learning” (Mayer, 2002). Several longitudinal studies have identified a bidirectional association between the repetition of increasingly challenging exercises and improved WM capacity (Holmes et al., 2009; ten Braak et al., 2021).
Given the significance of WM and its impact on children’s cognitive development, targeted interventions to strengthen these skills are essential, especially for children with WM difficulties. Initially, research on supporting children’s WM through interventions was centered on interventions taking place in a clinical setting (aside from the direct practice of EF through repetition), including computer-based training programs like Cogmed (Klingberg et al., 2005; Melby-Lervåg and Hulme, 2013). These programs are designed to improve WM by engaging children in progressively challenging WM tasks. While some interventions have shown small-to-medium effects on WM (Smithers et al., 2018), numerous studies have failed to observe such improvements (for a review, see Melby-Lervåg et al., 2016; Ripp et al., 2022). Improvements tend to occur primarily in tasks that closely resemble the training tasks (i.e., “near-transfer effects”) (Hampshire et al., 2019; Melby-Lervåg et al., 2016). However, achieving “far-transfer effects,” where the benefits of the intervention extend to different skills and contexts, remains challenging, with improvements often not sustained (Diamond and Ling, 2020; Melby-Lervåg and Hulme, 2013; Randall and Tyldesley, 2016; Rodas et al., 2024; Sala and Gobet, 2017).
These findings prompted a shift toward school-based interventions tailored to the school environment (i.e., classroom-based interventions), where WM is consistently engaged (Mattera et al., 2021; Rowe et al., 2019; Smid et al., 2020). School-based approaches to supporting WM generally fall into two categories: direct approaches and indirect approaches (Dörr and Perels, 2020). Direct approaches involve training through progressively challenging repetitive exercises (Zelazo et al., 2016), which are integrated into subjects like math to stimulate WM. In contrast, indirect approaches create an environment that fosters the development of WM without directly targeting it. Teachers can thus significantly contribute to supporting students’ WM by fostering an enriching and supportive learning environment. The current focus in research lies predominantly in examining the influence of the school, classroom environment, and the role of the teacher in addressing students’ WM challenges (Sankalaite et al., 2021; Vandenbroucke et al., 2017a).
Teachers can interact with their students on two levels: the dyadic level (i.e., teacher-student relationship; TSR) and the classroom level (i.e., TSI). TSR, grounded in Attachment Theory (Bowlby, 1969), is commonly defined through three dimensions: closeness, conflict, and dependency (Hamre and Pianta, 2001). Closeness represents the extent of openness, warmth, and security in the relationship; conflict indicates disagreements and misunderstandings; and dependency refers to an inappropriate level of overreliance or possessiveness by the child (Verschueren and Koomen, 2012). TSR significantly affects a range of student outcomes, such as internalizing and externalizing behaviors, school dropout, and academic performance (for a systematic review, see García-Rodríguez et al., 2023). While low-quality TSR has been shown to negatively affect WM (de Wilde et al., 2015; Graziano et al., 2016), high-quality TSR is recognized as a protective factor for its development (Vandenbroucke et al., 2018b). Xu et al. (2024) further found that TSR had a stronger impact on EF than TSI, highlighting its importance in supporting these functions. Some studies have even found that the quality of TSR is essential for children to fully benefit from classroom-level support (Crosnoe et al., 2010; Liu et al., 2023; Nguyen et al., 2020).
The importance of TSR in supporting student outcomes is well-documented and highlights the general value of teacher support. However, given the pressing need for efficient interventions targeting EF/WM, recent research has shifted its focus toward exploring TSIs at the classroom level (Sankalaite et al., 2021; Vandenbroucke et al., 2018a). Unlike TSR, which would require individualized, one-on-one interventions, TSI takes place at the classroom-level, allowing teachers to efficiently allocate resources to meet the needs of a larger group of children struggling with WM. A key contribution to understanding TSI is the teaching through interactions (TTI) framework by Hamre and Pianta (2007), which highlights TSI as a central driver of student learning and emphasizes the significant role teachers play in shaping students’ cognitive development. The TTI framework is organized around three broad dimensions: instructional support, emotional support, and classroom organization (in what follows referred to as “organizational support”). The correlational (and sometimes causal) relationship between TSIs and EF (Sankalaite et al., 2021; Xu et al., 2024), especially in the context of WM, is supported by various theories. The primary theoretical frameworks, along with those highlighted within the TTI framework (Downer et al., 2010; Hamre et al., 2013), will be discussed in more detail further, as well as potential cross-domain connections among them.
Instructional support involves teachers using strategies to promote higher-order thinking by engaging students in cognitively stimulating activities, such as asking open-ended questions, providing feedback, and encouraging advanced language use (Hamre et al., 2013; Vandenbroucke et al., 2018a). The main idea is the distinction between memorizing information and acquiring “usable knowledge,” which involves understanding how pieces of information interconnect and depend on one another (Hamre et al., 2013; Mayer, 2002). Research shows instructional support has a significant impact on EF, with Xu et al. (2024) finding a significant medium effect, Hamre et al. (2014) linking higher quality instructional support to improved WM performance, and Howes et al. (2008) identifying it as a predictor of academic functioning. Two key theories explain the effectiveness of instructional support: Vygotsky’s Socio-Cultural Theory (1987) (i.e., scaffolding) and Bandura’s Social Learning Theory (1971) (i.e., learning through imitation).
Vygotsky’s Socio-Cultural Theory (1987) introduces scaffolding, a pedagogical approach that involves providing temporary support, and gradually reducing this support as the learner becomes more proficient and independent. A key element is the Zone of Proximal Development (ZPD), where teachers offer tailored support to guide students beyond their current abilities. As students progress, the support is reduced, transferring responsibility back to them (van de Pol et al., 2010). When teachers tailor their support to match students’ abilities, they allow students to gradually master complex tasks without becoming overwhelmed. As such, it also has a lesser, yet relevant, connection to the Cognitive Load Theory (see section on organizational support), as scaffolding can help prevent overloading WM (Jaeger et al., 2017; Sankalaite et al., 2023; van Nooijen et al., 2024).
Socio-Cultural Theory (1987) highlights the social context of learning, while Social Learning Theory (1971) focuses on how social interactions, such as observing and imitating role models like parents and teachers, facilitate learning. These interactions can support the limited capacity of WM, helping students efficiently integrate and apply lesson content before presenting something new (Rosenshine, 2012). In other words, when teachers demonstrate effective strategies for managing information, students can observe, imitate and apply these strategies, which enhances their understanding of the lesson content and aids their ability to absorb it fully. However, whether imitation occurs or not, depends on four factors: attention, retention, motoric reproduction and reinforcement/motivation (Bandura, 1971). In the context of WM, attention and retention are critical factors for effectively processing and retaining new information.
Both theories emphasize the role of language in children’s cognitive development. Vygotsky (1987) sees language as essential for higher-order thinking, with interactions in the ZPD helping children to articulate thoughts and grasp complex ideas. Bandura (1971) suggests that social interactions, particularly with teachers as language models, influence language acquisition. By using scaffolding, modeling, and language stimulation, teachers thus engage students’ WM, making learning more efficient and manageable.
By offering emotional support, teachers create a safe and supportive classroom climate that fosters students’ social and emotional functioning, contributing to a conducive learning environment where students feel valued, respected, and emotionally secure (Hamre and Pianta, 2005). Key features include fostering positive interactions, cultivating a constructive classroom climate, and acknowledging students’ unique needs. A recent meta-analysis by Xu et al. (2024) shows a significant medium effect of emotional support on EF. It also significantly contributes to students’ level of engagement, motivation, and academic performance (Ponitz et al., 2009; Roorda et al., 2011; Sankalaite et al., 2023). There are two main theories underlying this type of support: Attachment Theory (Ainsworth et al., 1978; Bowlby, 1969) and Self-Determination Theory (Ryan and Deci, 2000).
Bowlby’s Attachment Theory (Bowlby, 1969, 1982) posits that children naturally seek affectionate bonds with primary caregivers, including teachers who, as secondary attachment figures, play a particularly crucial role for young, vulnerable children and those with limited EF skills (Ainsworth et al., 1978; Bowlby, 1969; García-Rodríguez et al., 2023; Longobardi et al., 2024; Verschueren and Koomen, 2012; Zajac and Kobak, 2006). Emotional support from teachers improves WM by providing a secure base and a safe haven to encourage exploration and co-regulate emotions during distress, thereby helping children tackle new challenges, reduce stress, and free up cognitive resources (Ainsworth and Bowlby, 1991; Nordling et al., 2016; Vandenbroucke et al., 2017a). Emotional support is also relevant to Cognitive Load Theory, as well-being can influence cognitive load (for a review, see Hawthorne et al., 2019). Although Attachment Theory mainly focuses on dyadic relationships between a child and its caregiver, it can be applied to the classroom level as well (Birch and Ladd, 1998; Hamre and Pianta, 2001). Furthermore, it can be linked to the Self-Determination Theory (Ryan and Deci, 2000) through their shared emphasis on positive human connectedness.
According to the Self-Determination Theory, all humans require three fundamental psychological needs: competence, autonomy and relatedness (Ryan and Deci, 2000). Competence refers to feeling capable of achieving goals; autonomy is the sense of being in control over one’s actions; and relatedness is the feeling of being connected with others (Ryan and Deci, 2017). Previous research has shown that providing emotional support strengthens the three basic needs, thereby boosting children’s intrinsic motivation (Reeve, 2012; Ruzek et al., 2016), while also improving WM-related behaviors. For example, Sankalaite et al. (2023) found that granting students autonomy in their learning exposes them to opportunities to further develop their WM skills. This finding is supported by earlier research, showing a link between autonomy and student engagement (Ruzek et al., 2016). Moreover, WM has shown to be a mediating factor in the association between intrinsic motivation and academic performance (Pascoe et al., 2018). According to Shenhav et al. (2017), increased motivation may enhance the allocation of limited cognitive resources, suggesting that when students are more intrinsically motivated, they are better able to focus their cognitive efforts on the task, thereby maximizing WM efficiency.
Organizational support involves teachers creating a structured environment to help children navigate activities efficiently, and proactively managing the classroom (Pianta et al., 2012). It includes managing behavior, time, and attention, and features organized routines and varied teaching methods (Emmer and Stough, 2001; Pianta et al., 2012). Research has demonstrated a significant association between organizational support and EF (Raver et al., 2009; Rimm-Kaufman et al., 2009; Ursache et al., 2011; Xu et al., 2024). Students in classrooms with higher levels of organizational support are more engaged and spend less time off-task, leading to increased learning opportunities. Cognitive Load Theory (Sweller, 2011) and Instrumental Learning Theory (Skinner, 1938) shed light on the mechanisms underlying the effectiveness of organizational support.
The Cognitive Load Theory emphasizes that WM is limited (Cowan, 2001). To prevent overload and optimize learning, individuals should reduce external load and manage resources effectively (Sweller, 2011). Sweller (2011) distinguishes three types of cognitive load: intrinsic cognitive load, which is the inherent difficulty of the material, extraneous cognitive load, which includes non-essential demands that reduce WM capacity, and germane cognitive load, which involves the effort to integrate new information into long-term memory. Essentially, this theory suggests that presenting excessive or complex information may overwhelm students, leaving them with inadequate time to comprehend and process it, causing cognitive overload. However, through organizational support (i.e., creating a structured learning environment), students can free up space in their WM, which helps them to focus more effectively on understanding the learning materials, leading to improved learning and academic achievement (Paas and van Merriënboer, 2020; Sweller, 2011). Furthermore, a cross-domain connection exists in that a well-structured classroom can support children’s basic psychological needs, such as the need for competence, benefiting not only cognitive load reduction but also overall student well-being (Ford, 2019).
The Instrumental Learning Theory (Skinner, 1938) suggests that individuals learn based on the consequences of their actions: positive outcomes increase the likelihood of behavior repetition, while negative outcomes decrease it (Thorndike, 1935). Essentially, individuals modify their behavior through trial and error by linking their actions to environmental responses. This highlights the role of teachers in reinforcing or eliminating certain behaviors, (Skinner, 1974), especially those behaviors that disrupt focus and task engagement in students. By effectively addressing these behaviors, teachers can increase students’ on-task behavior, optimizing cognitive resources. Additionally, Park et al. (2023) found that when WM is overloaded, the ability to associate actions with their consequences weakens, slowing down the learning process. To prevent this, teachers can create structured environments that minimize distractions and encourage positive behaviors, which can prevent WM overload, ensuring better information processing (Elliott et al., 2010; Paas et al., 2010). Behavior change can also be explained through motivational theories, like the Self-Determination Theory, as children may view their actions as either fulfilling or failing to meet their needs, influencing their persistence or avoidance of those behaviors (Hagger et al., 2020).
Building on the previous theoretical discussion, TSI is seen as instrumental in optimizing and allocating cognitive resources effectively, with instructional support drawing predominantly on the Socio-Cultural and Social Learning Theory, emotional support on Attachment and Self-Determination Theory, and organizational support on Cognitive Load and Instrumental Learning Theory. Additionally, potential cross-domain connections may exist between the three TSI domains and their underlying theories.
While these theories explain the relationship between TSIs and WM by optimizing classroom conditions in which EF can thrive, intervention studies on TSI’s effectiveness in improving WM in general are scarce. Moreover, even effective interventions face notable challenges and limitations. First, previous research indicates that intensive interventions tend to be most effective (Diamond and Ling, 2016), yet this contrasts with teachers’ already demanding schedules, leaving little room for adjustments or additional tasks (for a Belgian report on teacher workload, see Stichting Innovatie and Arbeid, 2024). Additionally, most interventions typically last between 4 months (Cappella et al., 2012), and a full school year (Colmar et al., 2020; Pianta et al., 2017; Wolf et al., 2018), further complicating the feasibility of implementation. Such extended durations demand sustained commitment and consistency, which can be difficult for teachers to maintain, especially when room for additional activities is already limited. As a potential solution to the challenge posed by extensive training programs, targeted training emerges as a viable alternative. Kincade et al. (2020) discuss the distinction between “manualized versus potentially more nimble, customizable modular approaches” (p.738), advocating for strategies that are cost-effective, modular, and easy to implement. Second, variation in both the implemented intervention strategies and tasks that are used (e.g., simple tasks that focus on basic skills vs. complex tasks that requires more advanced cognitive skills), complicates the comparison of interventions (Diamond, 2013; Morra and Borella, 2015; Pergher et al., 2019). Third, although individual studies have explored the impact of specific types of support on WM (e.g., emotional support being positively associated with WM; Vandenbroucke et al., 2017a), there has yet to be a comprehensive study comparing the effects of the three types of support on children’s WM (Sankalaite et al., 2021). Given the scarcity of studies and uneven focus on different types of support, a direct comparison of instructional, emotional, and organizational support can, therefore, provide insights into which approach is most effective.
To address both the effectiveness and efficiency of interventions, the current study aims to (1) identify the most effective strategies within each support type for alleviating challenges related to WM and (2) empirically assess the impact of these strategies on WM through a series of microtrials. Microtrials are small-scaled randomized experiments that evaluate the impact of brief, targeted environmental changes. Unlike Randomized Controlled Trials (RCTs), which aim to achieve full treatment effects, microtrials focus on identifying whether specific program elements (i.e., teacher behaviors) contribute to meaningful changes (Howe et al., 2010), providing a cost-efficient alternative for participants. Using this design, the study will examine the immediate effects of instructional, emotional, and organizational support through three interventions specifically designed to improve the WM-related problems of primary school children in Flanders (Belgium).
More specifically, the aim of this study is to determine whether modifications to TSI strategies improve WM-related behavior and performance in children with poor WM. To achieve this, four microtrials will assess the effectiveness of each support type (instructional, emotional, organizational) on both informant-reported and performance-based WM, compared to a teaching-as-usual (TAU) control group.
First, a manipulation check will assess whether there is an increase in the use of the targeted support type in the experimental groups (compared to TAU), confirming that teachers effectively implemented the targeted support strategies. At the same time, a contamination check will ensure that the use of other types of support remains unchanged throughout the microtrials, indicating that teachers do not adopt strategies from other TSI groups.
Second, considering that students’ EF, including WM, can be improved (Diamond and Ling, 2020), and given the small-to-medium effect sizes observed between TSIs and WM (Vandenbroucke et al., 2018a), it is expected that there will be an immediate change effect (from pre- to post-intervention) of informant-reported WM and performance based-WM in the experimental groups, but not in the TAU condition. More specifically, organizational support is expected to have the largest impact on WM, followed by instructional support, with emotional support having the smallest effect. This is consistent with Xu et al. (2024), who found the largest effect size for organizational support (r = 0.136) and smaller effect sizes for instructional (r = 0.079) and emotional support (r = 0.086). Similarly, the systematic review conducted by Sankalaite et al. (2021) reported the strongest effects for organizational support and the weakest for emotional support, with the latter being regarded as the least WM-specific (Sankalaite et al., 2023).
Third, it is hypothesized that larger effects will be found in students demonstrating more problematic WM-related behaviors. A study by Diamond and Ling (2016) suggests that children with poorer EF tend to benefit most from interventions that aim to improve these skills. Although this study focuses on children with poor WM, variation in scores is anticipated among these students, indicating that those who exhibit greater WM difficulties are likely to show the most substantial gains.
Last, the study will explore whether there is a moderation effect of TSR in the effectiveness of the intervention. Findings from various studies emphasize that the quality of TSR is essential for maximizing the effectiveness of support provided at the classroom level (Crosnoe et al., 2010; Liu et al., 2023; Nguyen et al., 2020). Accordingly, it is expected that the effectiveness of the classroom-level intervention will be positively influenced by a strong, positive relationship between the teacher and student (as indicated by a high closeness score), and negatively influenced by a relationship characterized by high levels of conflict and dependency. This aligns with evidence showing that positive TSR fosters a more supportive and encouraging learning environment (Liu et al., 2023; Rimm-Kaufman et al., 2014).
Participants will be organized into triads consisting of a primary school teacher, one of their students exhibiting poor WM, and the student’s parent. Only teachers from regular primary education are eligible; those from special education schools are excluded due to variations in structural factors, such as classroom size and staff-to-student ratio, and the specialized pre-service training these teachers have received to support students with special educational needs. Teachers must work at least half-time in the same classroom to build a relationship with the student and to ensure sufficient implementation of the intervention strategies, and have at least 2 years of teaching experience to ensure solid practical knowledge and confidence. Given the Belgian education system, where part-time or co-teaching is common, only one teacher per triad will participate. To minimize potential bias in the data, participation is limited to no more than five teachers from the same school. Additionally, to prevent contamination, all teachers within the same school will be in the same condition (one of the three experimental groups or the control group). To ensure feasible data collection, each teacher will participate with one student aged 6–12 (grades 1–6) who exhibits WM-related problematic behaviors (for definition, see the section on Screening), and only one parent per student is invited to participate.
To determine the necessary sample size, a power analysis was conducted (using G*Power 3.1). The analysis, aimed at detecting main effects and two-way (within-between) interaction effects in a repeated-measures ANOVA, revealed that a minimum of 38 teacher-student dyads per condition is required. This calculation was based on small-to-medium effect sizes (f = 0.15), a correlation among repeated measures of 0.75, a desired power of 0.80, and an alpha level of 0.05. To account for an anticipated attrition rate of 10%, the recruitment target is set at 168 participants from the Flemish speaking community in Belgium, organized into 42 dyads per condition, each consisting of a teacher and a student (including one parent). For the experimental groups, regular primary schools across Flanders will be randomly selected for participation by sending invitation mails to the principals who will be asked to forward it to their teaching staff. Principals, school counselors (“zorgcoördinatoren”), and interested teachers will then be invited to an information session about the study. After this session, teachers can complete an online consent form, thereby confirming their participation. The recruitment of the control group will take place through an online advertisement that will be posted on social media (i.e., Facebook). Teachers that sign up, will receive further information via email.
To identify students with poor WM skills, teachers are invited to complete the WM subscale of the Behavior Rating Inventory of Executive Function (BRIEF-2; Gioia et al., 2015; Huizinga and Smidts, 2020), assessing observable WM-related behaviors in terms of frequency and severity using a 10-point Likert scale. Based on these scores, only students exhibiting at least four problematic WM-related behaviors (i.e., a score of 5 or higher in both frequency and severity) are considered for inclusion in the study, as they will benefit most from the intervention. The scores on the BRIEF-2 WM subscale are solely used by the teacher to determine a student’s eligibility for study participation. After the pre-screening of students, teachers receive an information letter they can distribute to the parents of these students. A link to an online consent form for parents is included in this information letter. After parents have signed the consent form, they are invited to complete the adapted BRIEF-2 WM-subscale for validation of the teacher’s report, allowing for a comprehensive understanding of the students’ WM-related behaviors across different settings, as these can vary between school and home environments. Following this, the teacher-student dyad will be confirmed. If multiple students from the same classroom sign up for participation, the student with the highest scores in terms of both frequency and severity, as perceived by the teacher, will be prioritized. In the event of identical scores, one student is randomly selected for participation in the intervention. After student selection, the teacher-student dyads will be randomly assigned to one of three experimental groups or control group, using a case-control matching algorithm that accounts for the students’ age and gender.
Socio-demographic information will be collected from both teachers and parents using an adaptation of the socio-demographic questionnaire as constructed by Vandenbroucke et al. (2017a). The teacher questionnaire includes variables such as gender, age, teaching experience, classroom size, and their current position. Parents are asked questions about family type, profession, education level, ethnicity, and (both general and school-related) difficulties the child experiences.
WM will be assessed through multiple methods and informants. This approach is essential for accurately capturing the multi-component and dynamic nature of WM (Huizinga et al., 2018). WM will serve as both primary and secondary outcomes, enabling an assessment of both the interventions’ overall impact on WM-related problematic behavior and its specific effects on WM capacity.
The primary outcomes (i.e., informant-reported WM) will be collected using an adapted version of the BRIEF-2 WM subscale (Gioia et al., 2015; Huizinga and Smidts, 2020). The BRIEF-2 WM is an ecological valid instrument that provides insight into how students experience and manage challenges, such as those related to WM, in everyday contexts, reflecting the functional impact of WM difficulties on learning and behavior. It includes items such as “Forgets what he/she needs to do when multiple things are asked,” and “Forgets to hand in homework.” Each item will be rated on frequency and severity using a 10-point Likert scale, ranging from 1 (not at all frequent/problematic) to 10 (extremely frequent/problematic). While the BRIEF-2 typically uses a 3-point Likert scale, ranging from 1 (never) to 3 (often), to assess the child’s behavior over the past 6 months, the scale has been modified here by implementing a 10-point Likert scale, allowing for more variability in responses. Furthermore, the separate frequency and severity ratings allows the assessment of both the behaviors’ occurrence and intensity. These adjustments are intended to detect subtle and more nuanced changes in students’ WM-related behavior throughout the intervention period of 4 weeks.
For teachers in the experimental groups, the intervention will target the four WM-related behaviors with the highest frequency and severity scores in the pre-test (i.e., baseline), with scores verified prior to the intervention’s start. To monitor progress throughout the intervention, teachers will use the m-Path application1 to reassess these problematic behaviors at the intervention’s midpoint (i.e., 2 weeks after interventions starts) and at its conclusion (i.e., after 4 weeks). The total scores at each timepoint (i.e., baseline, midpoint, end) will be calculated by summing and averaging the frequency and severity scores for each of the four behaviors separately.
Additionally, to compare the experimental groups with the control group, the full 8-item WM subscale of the BRIEF-2 will be used to evaluate children’s informant-reported WM problems. Both teachers and parents will complete the questionnaire pre- and post-intervention. Total scores will be calculated for the teacher and parent questionnaires by summing and averaging the raw scores of the frequency and severity subscales separately, with scores ranging from 1 to 10.
More exploratory, students’ performance-based WM will be evaluated both pre- and post-intervention. Students’ performance-based WM will be assessed using the Corsi block tapping test (Corsi, 1972) and the Digit Span subtest of the WISC-V (Wechsler, 2014). The Corsi block tapping test assesses children’s visuospatial WM. For the microtrial studies, a computerized adaptation of this test will be used to improve test-retest reliability and standardization (Brunetti et al., 2014). Moreover, a systematic review by Arce and McMullen (2021) found no significant performance differences between physical and digital versions. During the test, children are presented with a grid of nine blocks arranged randomly on their screens, with specific blocks lighting up in a predetermined order. The challenge for children is to remember the sequence in which these blocks illuminate. Once a “Go” signal appears, children must click the blocks in the correct order. Each sequence comprises two items, with immediate feedback provided after each attempt. The test concludes if errors occur on both items within the same sequence. As participants progress, the sequences become progressively longer. The task has two conditions: forward and backward. The forward condition assesses short-term visuospatial memory by having the child replicate the sequence in the presented order. The backward condition tests the ability to manipulate and rearrange spatial information by recalling the sequence in reverse, demanding both short-term memory and spatial manipulation skills. A total raw score of correct answers in both conditions, as well as the span score (i.e., the longest sequence the child can reproduce), will be calculated. The Corsi block tapping test has adequate psychometrics (Berch et al., 1998; Kessels et al., 2000).
The second task administered to the children will be the Digit Span subtest of the WISC-V (Wechsler, 2014), which measures children’s verbal WM. During this task, the researcher verbally presents a series of digits to the child. The child’s objective is to accurately repeat the digits either in the same order or in reverse, depending on the specific condition. The test continues until the child provides incorrect responses for two items of the same sequence. The scoring method mirrors that of the Corsi block tapping test, ensuring consistency. The WISC-V, including Digit Span, has shown adequate reliability and validity, with lambda values of 0.73, 0.79, and 0.85 for Digit Span forwards, Digit Span backwards, and Digit Span total, respectively (Wechsler, 2014).
To measure TSR as a moderator of the relationship between children’s pre- and post-intervention WM scores, both teachers and students will complete a questionnaire pre- and post-intervention. Teachers will fill out the Student-Teacher Relationship Scale (STRS; Koomen et al., 2012; Pianta, 2001), which evaluates three dimensions of TSR: closeness, conflict, and dependency. This questionnaire consists of 28 items rated on a 5-point Likert scale, ranging from 1 (not at all applicable) to 5 (highly applicable). Examples include “I share an affective, warm relationship with this child” (closeness), “This child and I always seem to struggle with each other” (conflict), and “This child reacts strongly when he/she cannot be with me” (dependency). Subscale scores are calculated by summing raw scores for items within each dimension. The STRS has an excellent reliability and validity (Bernaerts et al., 2015; Koomen et al., 2007; Pianta, 2001), with Cronbach’s alpha values of 0.84, 0.91, and 0.80, for closeness, conflict, and dependency, respectively.
Children, on the other hand, will complete the Child Appraisal of Relationship with Teacher Scale (CARTS; Frewen et al., 2013; Vervoort et al., 2015). This questionnaire also assesses three dimensions: closeness, conflict and dependency. It consists of 16 items, each rated on a 5-point Likert scale, ranging from 1 (no, always) to 5 (yes, always). Examples include “I have fun with my teacher” (closeness), “I often argue with my teacher” (conflict), and “I often ask my teacher for help” (dependency). The scores for each subscale are calculated by summing the raw scores for the items within each subscale. The CARTS has demonstrated strong psychometric properties, with Cronbach’s alpha values 0.80, 0.85, and 0.73 for closeness, conflict, and dependency, respectively (Gregoriadis et al., 2020; Vervoort et al., 2015).
The Classroom Assessment Scoring System (CLASS; Pianta et al., 2008) is an observational tool designed to evaluate the quality of TSIs within the classroom. It is categorized into three domains: instructional support, emotional support and organizational support. Underlying the three domains are 12 dimensions that each consist of concrete examples (indicators) of TSI behaviors. Drawing from the CLASS indicators outlined in Hamre et al. (2013), a questionnaire was developed intended for teachers to complete pre- and post-intervention. The questionnaire consists of 42 questions, rated on a 7-point Likert scale ranging from 1 (never applicable) to 7 (always applicable). Subscale scores are calculated by summing the raw scores. In the experimental groups, the questionnaire will serve a dual role: as a manipulation check to ensure the intervention’s effective implementation, and as a contamination check to monitor the influences from the two other TSI domains. In the control group, it will function solely as a contamination check. Comparisons between the experimental groups and control group will help to verify whether observed changes in the intervention groups can be attributed to the intervention itself, rather than external factors such as natural variations or improvements in teaching strategies.
While implementing the intervention, teachers of the experimental groups will maintain a weekly diary to record their strategy use. Through the m-Path application,1 they will be prompted to report the extent to which they have used each of the five trained strategies during that specific week, as well as any additional strategies employed to address the WM-related problematic behaviors exhibited by the student (i.e., fidelity check). Responses are rated on a 5-point Likert scale, ranging from 1 (I did not use this strategy) to 5 (I used this strategy very often). Scores for each strategy, are totaled for each of the two target behaviors and then averaged.
The interventions will be focused on strategies within one type of TSI support (i.e., emotional support, organizational support or instructional support) to reduce WM-related problematic behaviors on the classroom-level. High-potential strategies for each support type were selected by adhering to the methodology used in a meta-analysis by Kincade et al. (2020). Each of the three interventions incorporates strategies derived from effective WM interventions identified in a systematic literature review on the relationship between TSI/TSR and EF performance (Sankalaite et al., 2021). In this review, three WM interventions were categorized as having high methodological quality, one was rated as moderate, and one study was identified as low quality, according to the Grading of Recommendations Assessment, Development and Evaluation method. Additionally, a qualitative study using semi-structured interviews with primary school teachers from Belgium and the Netherlands provided insights into the implementation of TSI strategies in the classroom (Sankalaite et al., 2023).
To distil the strategies, studies from Sankalaite et al.’s (2021) systematic literature review were examined, specifically focusing on those interventions demonstrating significant, positive outcomes in children’s WM. This analysis involved identifying common practice elements between the effective interventions (i.e., specific strategies that are consistently implemented across various effective interventions to enhance TSIs).
First, the manuals of specific interventions were obtained from authors or drawn from broader research sources (i.e., published manuscripts). All information about the interventions was thoroughly reviewed, focusing on extracting strategies relevant to the study’s objectives. Additionally, the qualitative study by Sankalaite et al. (2023) offered valuable insights into TSI strategies to address WM-related difficulties, with specific attention to how and in which instances these strategies can be effectively employed, according to the perspectives of Flemish teachers. This step ensured that all strategies translate well into the Flemish education context. The strategies extracted from all sources were then compared across the different interventions, resulting in a comprehensive list of teacher strategies for each type of support. Next, the list of strategies was distilled, a process defined by Chorpita et al. (2005) as “the reduction of data to a simpler, smaller data set of meaningful units” (p. 13). Specifically, similar practices were combined (e.g., “Make eye contact,” “Use a warm voice,” and “Use soft and friendly voice and keep open, engaged posture” were combined into one strategy called “Non-verbal communication”). The full overview of the results from the data distillation can be found in Supplementary material. Drawing from these results, the five most frequently used strategies for each type of support were selected (Tables 1–3). These carefully selected strategies serve as high-potential components for addressing WM difficulties within each respective support type.
Following the selection of the strategies, literature on these strategies was summarized to develop the initial intervention sheets, which provided a strong foundation for further refinement. Following team discussions, the literature was revisited to clarify the mechanisms of each support type (e.g., Attachment Theory) and their potential to improve WM (Tables 4–6). Generally, instructional support strategies are based on scaffolding principles, imitation, and language stimulation; emotional support strategies are drawn from Attachment Theory and Self-Determination Theory; and organizational support strategies are informed by Cognitive Load Theory, with some also incorporating principles from Instrumental Learning Theory. Through multiple iterations and team input, the content of the sheets was refined, leading to the final versions of the intervention sheets. In order to give teachers concrete examples of the strategies and sub-strategies, three fictional case studies were made, each focusing on a different type of support. Each case study, presented on a separate sheet, was integrated into the intervention sheets, providing teachers with concrete examples of how to apply the theoretical concepts in practice.
Each intervention sheet follows a consistent structure, comprising the following elements: (1) name of the strategy, (2) definition, (3) strategy sub-components, (4) impact of the strategy on TSI and WM, and (5) implementation parameters. Some of these strategies are intended for use before the behavior occurs (antecedent strategy), others following the behavior (consequent strategy), or during the behavior itself (behavioral strategy). In some cases, a strategy can be applied across multiple stages. For example, modeling, as an instructional support strategy, can be used both before and during the behavior to set an example or to provide immediate support as the behavior unfolds. Detailed information on the strategies is provided in Tables 4–6.
Once teachers are assigned to the emotional, organizational or instructional support condition, and prior to implementing the intervention, they will be provided with the intervention materials (i.e., information sheets and case study). Next, they will be able to sign up for a coaching session with one of three coaches, all of whom are experienced teacher educators with expertise in EF. After teachers have familiarized themselves with the materials, they will be prompted to take a short quiz on an online platform to assess their understanding. A score of 7 or higher (out of 10) on the quiz indicates sufficient understanding of the content, while those scoring below 7 will be encouraged to review the materials again before taking part in the coaching session.
During a 90-min coaching session, attention will be directed toward addressing the two most problematic WM-related behaviors (out of four that were selected). The coaching session will start with a functional analysis, using an ABC-scheme. Teachers will present two specific situations in which the WM-related problematic behaviors occurred. The coach will then conduct a series of follow-up questions aimed at delving deeper into the circumstances surrounding each behavior: the antecedent (What led up to the behavior?), the behavior itself (What did the student do at that moment?), and the consequence (What was the result or outcome of the behavior?). Both examples will then be compared based on common elements. In the next part of the coaching session, teachers will be encouraged to reflect on the five targeted strategies and will contemplate together with the coach how they might use them to improve the two WM-related problematic behaviors. Through this reflective process, a personalized intervention plan will be developed, detailing specific action points for each of the strategies, if applicable. Following the coaching session, teachers will receive a written overview of the intervention plan that they can use as a reference.
Next, teachers will be tasked with implementing the intervention plan at the classroom-level for 4 weeks, applying the discussed strategies whenever the student exhibits WM-related problematic behavior. Throughout the intervention, teachers will keep a weekly diary using the m-Path application,1 documenting the frequency of their use of the trained strategies to address the WM-related problematic behaviors of the selected student, or the application of alternative strategies. The weekly diaries will confirm the implementation of the trained strategies as the primary approach to address the student’s behaviors, and will ensure that any observed effects of the intervention can be attributed to these strategies. Teachers who fail to complete the weekly diaries or report using strategies different from those specified will be contacted and provided with further guidance on what is expected. After 2 weeks (mid-intervention) and after 4 weeks (post-intervention), the effectiveness of these strategies in addressing the two identified WM-related behaviors will be evaluated through the m-Path application. If both behaviors score below 5 on the 10-point Likert scale for both frequency and severity, teachers will take part in a second coaching session. This second session will focus on the remaining two behaviors identified prior to the initial coaching session, and a new intervention plan will be devised accordingly. Teachers in the control group will not receive the materials and will continue teaching as usual throughout the 4-week period.
As previously mentioned, four hypotheses regarding the impact of TSI on WM were proposed. First, an increase in the targeted support type is anticipated in the experimental groups compared to TAU. Second, immediate changes in informant-reported and performance-based WM are expected in the experimental groups, but not in TAU, with organizational support anticipated to have the most significant effect. Third, larger effects are predicted for students with the lowest WM. Fourth, the potential moderating effect of TSR on intervention effectiveness will be investigated, with TSI expected to be positively influenced by high levels of closeness and negatively affected by high levels of conflict.
As a first step in the analyses, the extent and pattern of missing data in the dataset will be assessed to determine whether the data is Missing Completely at Random (MCAR), Missing at Random (MAR), or Missing Not at Random (MNAR). If the pattern of missingness indicates data to be MCAR or MAR, data imputation techniques (i.e., multiple imputation) will be applied to estimate and replace the missing values. Variables will be grouped based on their construct (i.e., informant-reported WM, performance-based WM, and TSR), and conditions will be separated. Subsequent analyses will be conducted on the imputed dataset. If the missing data pattern is found to be MNAR, a sensitivity analysis will be conducted to test various scenarios. Prior to testing the hypotheses, a preliminary analysis will assess any significant baseline differences between conditions regarding the primary and secondary outcomes (i.e., WM and TSR) as well as for CLASS, using an ANOVA with four groups. Cronbach’s alpha will be calculated to evaluate the internal consistency of both the BRIEF-2 (specifically the frequency and severity scales of the four selected behaviors) and the CLASS. Furthermore, confirmatory factor analysis will be performed on the pre-measurement data of the CLASS to assess the factor structure of the three sets of TSI.
To test the first hypothesis, paired-samples t-tests will be employed to examine whether there is an increase in the use of the specified type of support within the experimental groups (i.e., manipulation check), while the other types of support remain the same (i.e., contamination check). Specifically, pre-intervention CLASS scores at will be compared with the post-intervention CLASS scores for each of the four conditions separately.
For the second hypothesis, repeated-measures ANOVA will be used to see if there are changes in informant-reported and performance-based WM between the experimental and control groups. The pre- and post-intervention scores of the WM assessments will be used as within-subject variables, while the conditions will be used as between-subject factors. Effect sizes will be assessed using partial eta squared (ηp2). According to Cohen (1988) and Miles and Shevlin (2001), a partial eta squared of 0.01 suggests a small effect, 0.06 indicates a medium effect, and 0.14 indicates a large effect. Following this, pairwise comparisons will be performed to identify which conditions show significant improvements. Additional analyses using paired-samples t-tests will be executed to determine in which conditions there is a significant change in WM. Moreover, scores from all timepoints (pre-, mid-, and post-intervention) will be compared to assess the trajectory of changes in WM throughout the intervention period.
To assess the third and fourth hypothesis, namely whether children who score highest on WM-related problematic behaviors benefit the most from the intervention and whether the influence of the intervention on informant-reported WM and performance WM varies depending on the aspects and level of TSR, a moderation analysis (i.e., repeated measures ANOVA) will be conducted. For the third hypothesis this will include the BRIEF-2 (frequency and severity) scores for both teacher and parent report. For the fourth hypothesis, six moderator variables will be added: closeness, conflict, and dependency, with separate reports by both teachers and children. Effect sizes will be calculated using Cohen’s d to quantify the magnitude of changes observed in the outcome measures. Specifically, a Cohen’s d of approximately 0.2 indicates a small effect, around 0.5 signifies a moderate effect, and 0.8 or higher indicates a large effect (Cohen, 1988).
Though few studies have explored TSI’s impact on WM, existing research indicates a small yet significant association between TSIs and WM (Vandenbroucke et al., 2018a), warranting need for further research. To address this gap, microtrials will be conducted to identify the most effective TSI components, assess their impact on WM, and determine the moderators that influence these outcomes.
Numerous studies indicate that positive and supportive interactions between teachers and students are associated with improvements in WM (Sankalaite et al., 2021; Vandenbroucke et al., 2018a). This finding suggests that enhancing TSI quality could be a viable strategy for improving children’s WM performance. However, the specific mechanisms driving the effects remain underexplored, even though such understanding is crucial for designing both effective and efficient interventions. Moreover, the existing EF/WM intervention studies often vary in their methodological approaches (e.g., design, intervention characteristics, measurements), making it challenging to compare results and draw definitive conclusions (Rowe et al., 2019). This study will use adapted versions of the BRIEF-2 to assess immediate changes in WM and the CLASS as a self-report measure, as no other psychometrically validated instruments with adequate sensitivity are currently available. While these adaptations allow for a more tailored assessment, they may also affect the reliability and comparability of the measures, requiring careful interpretation of the findings.
Another challenge is that the current body of research primarily demonstrates correlations rather than causation, leaving the precise nature of the relationship uncertain. Nonetheless, understanding the teacher’s role in supporting students’ WM development is essential for developing interventions tailored to the specific needs of students that can ultimately improve student learning and well-being. Identifying effective TSI components and conducting rigorous longitudinal research to explore potentially causal relationships is thus necessary. Furthermore, it is important to consider that improvements in WM may not be the only outcome of TSI within the intervention. High quality TSIs may also enhance other aspects of students’ experience, such as motivation, engagement, and self-efficacy, factors that, while not measured in this study can indirectly support better WM functioning (Sankalaite et al., 2023). Consequently, WM improvements might not only stem directly from WM-specific interventions but could also be amplified by positive shifts in these related areas.
In order to confirm improvements in children’s WM capacity, it is important to evaluate whether the effects extend to other contexts, such as the home environment. With parents blind to the condition, their ratings of students’ WM can provide unbiased assessments of possible far-transfer effects. Additionally, near-transfer effects can be demonstrated through children’s performance on WM tasks. In case no direct effects of the TSIs are found on children’s WM performance, it is possible that TSI only enhances WM outcomes indirectly by fostering a more supportive learning environment, which, in turn, increases mental capacity. Over the long term, it can then be assumed that any lasting effects would likely be observable through objective measures. However, given that subjective (i.e., behavior ratings) and objective measures (i.e., performance-based tests) both have different underlying constructs (i.e., assessment of WM in context-loaded versus controlled settings), and correlations between these measures are often low (Dekker et al., 2017; Gerst et al., 2017; Toplak et al., 2013), it is not surprising that changes in students’ WM capacity may not always be reflected in objective measures. Observing transfer effects from the school environment to the home environment, even if not mirrored in objective measures, can therefore still yield valuable insights into their connection. Another explanation for a lack of far-transfer effects to the home environment may lie in the degree of support the environment provides to address the challenges children face. Initially, there may be a mismatch between the demands of the school and/or home environments and the children’s needs, potentially limiting their ability to fully demonstrate their capabilities in the informant-reported behavior ratings (Hendry and Scerif, 2023). Addressing this mismatch through more supportive environments could unlock the children’s potential. This can be achieved by implementing interventions that enrich the learning environment, integrate EF-related tasks, and reduce students’ stress levels (Hendry and Scerif, 2023). Consequently, an intervention applied in the school context may not necessarily transfer to the home environment.
Additionally, several practical implications need to be considered. First, the microtrial design, which does not aim for a complete treatment effect, addresses the common challenge of lengthy, impractical interventions for teachers already managing multiple tasks through its concise 4-week duration and straightforward implementation. By focusing on strengthening teachers’ implementation of existing strategies rather than introducing new ones, it seamlessly integrates into daily practice, making the intervention more feasible and manageable. However, while the microtrial design demonstrates the potential of a brief and targeted intervention to improve WM, it also limits the ability to assess the persistence or changes in its effects over time, as well as the long-term effectiveness of the implemented strategies. Yet, understanding these aspects is crucial for determining the interventions’ sustainability in real-world educational settings. While short-term outcomes can be evaluated, further research is needed to explore potential lasting benefits. Additionally, the brief intervention may mask meaningful changes that could emerge only after its completion (i.e., sleeper effect) (van Aar et al., 2017). As the primary goal of this study is to assess the potential of brief and targeted interventions, follow-up measures will not be included. However, if the interventions result in meaningful changes in WM-related behaviors, future research should incorporate follow-up assessments to further evaluate their impact. Second, as participation in the microtrial study is voluntary, it is likely that the involved teachers are highly motivated, which may reduce the observable effects of the intervention and introduce selection bias that affects the generalizability of the findings. However, this issue will be mitigated by recruiting schools instead of individual teachers and conducting contamination checks. If positive effects of the intervention on WM challenges are found, this selection bias will highlight the need to integrate EF training and TSI into the teacher curriculum to better equip teachers to recognize/identify WM-related issues and to evaluate the quality of their interactions with students. Third, research indicates that the effectiveness of TSIs in classrooms is greatly influenced by the quality of TSR (Crosnoe et al., 2010; Liu et al., 2023; Nguyen et al., 2020). However, it will remain unclear whether the interventions’ effectiveness would be consistent across all types of TSRs, particularly in strained or less supportive relationships and whether the results will improve if a TSR intervention targeting the teacher-student dyad were implemented prior to the TSI interventions. Therefore, future research should explore the interplay between TSI and TSR to better understand and interpret the outcomes of these two types of interventions. Although the microtrial studies do not directly target TSR, a notable strength is its assessment of both teachers’ and students’ perspectives on their relationships before and after the intervention. By helping teachers manage challenging situations more effectively, the interventions may enhance their self-efficacy, potentially improving TSR (Hajovsky et al., 2020; Summers et al., 2017). However, while the interventions target TSIs at the classroom level, analyses will address only one student exhibiting the most problems in WM, and not the whole classroom. Given the limited time in educational settings to provide individualized interventions, the focus will be on the student most likely to benefit, while teachers implement the intervention class-wide. This approach aims to maximize observable effects by concentrating on the student expected to gain the most from the intervention.
If two or three TSI components prove effective to support students with poor WM, future research could integrate these components and their strategies into one comprehensive intervention. By employing an RCT, researchers can then evaluate which combination of these components is most effective and whether their effects are sustainable long term. Ultimately, the goal is to contribute to the development of evidence-based practices that (1) support children’s WM, and (2) offer teachers a more practical alternative to complex interventions. Moreover, the interventions strive to not only strengthen teaching practices by integrating effective strategies, but also to enhance overall student learning outcomes. Through these efforts, schools will be provided with resources to foster cognitive development and academic success for students. In the context of Flanders’ tiered support system (in Dutch “zorgcontinuüm”), the interventions could be integrated within Tier 1 (in Dutch “brede basiszorg”). This tier encompasses a range of universal strategies and interventions designed to support all students, aiming to create an optimal learning environment that accommodates diverse needs. A school counselor can assist teachers in conducting a functional analysis and can provide guidance on the targeted and comprehensive implementation of individual strategies based on their well-defined theoretical mechanisms. This approach enables schools to effectively use current knowledge and strategies to tackle WM-related challenges within the framework of the existing support system.
The studies involving humans were approved by the Sociaal-maatschappelijke Ethische Commissie (SMEC). The studies were conducted in accordance with the local legislation and institutional requirements. Written informed consent for participation in this study was provided by the participants’ legal guardians/next of kin.
SP: Writing – original draft, Writing – review & editing. SS: Writing – review & editing. MH: Writing – review & editing. MM: Writing – review & editing. JS: Writing – review & editing. DB: Writing – review & editing.
The authors declare that financial support was received for the research and/or publication of this article. This work was supported by the “Fonds voor Wetenschappelijk Onderzoek” (FWO; G035523N#57357538).
The authors declare that the research was conducted in the absence of any commercial or financial relationships that could be construed as a potential conflict of interest.
The authors declare that no Generative AI was used in the creation of this manuscript.
All claims expressed in this article are solely those of the authors and do not necessarily represent those of their affiliated organizations, or those of the publisher, the editors and the reviewers. Any product that may be evaluated in this article, or claim that may be made by its manufacturer, is not guaranteed or endorsed by the publisher.
The Supplementary Material for this article can be found online at: https://www.frontiersin.org/articles/10.3389/feduc.2025.1528805/full#supplementary-material
Adams, E. J., Nguyen, A. T., and Cowan, N. (2018). Theories of working memory: Differences in definition, degree of modularity, role of attention, and purpose. Lang. Speech Hear. Serv. Schools 49, 340–355. doi: 10.1044/2018_LSHSS-17-0114
Ainsworth, M. D. S., Blehar, M. C., and Waters, E. (1978). Patterns of Attachment: A Psychological Study of the Strange Situation. Hove: Erlbaum.
Ainsworth, M. S., and Bowlby, J. (1991). An ethological approach to personality development. Am. Psychol. 46, 333–341. doi: 10.1037/0003-066X.46.4.333
Alloway, T. P., and Alloway, R. G. (2010). Investigating the predictive roles of working memory and IQ in academic attainment. J. Exp. Child Psychol. 106, 20–29. doi: 10.1016/j.jecp.2009.11.003
Alter, P., and Haydon, T. (2017). Characteristics of effective classroom rules: A review of the literature. Teach. Educ. Special Educ. 40, 114–127. doi: 10.1177/0888406417700962
Anderson, L. M., and Prawat, R. S. (1983). Responsibility in the classroom: A synthesis of research on teaching self-control. Educ. Leaders. 40, 62–66.
Ansari, A., and Pianta, R. C. (2018). Effects of an early childhood educator coaching intervention on preschoolers: The role of classroom age composition. Early Childh. Res. Quart. 44, 101–113. doi: 10.1016/j.ecresq.2018.03.001
Arce, T., and McMullen, K. (2021). The corsi block-tapping test: Evaluating methodological practices with an eye towards modern digital frameworks. Comput. Hum. Behav. Rep. 4:100099. doi: 10.1016/j.chbr.2021.100099
Attai, S. L., Reyes, J. C., Davis, J. L., York, J., Ranney, K., and Hyde, T. W. (2021). Investigating the impact of flexible furniture in the elementary classroom. Learn. Environ. Res. 24, 153–167. doi: 10.1007/s10984-020-09322-1
Ausubel, D. P. (1968). Educational Psychology: A Cognitive View. Boston, MA: Holt, Rinehart and Winston.
Baddeley, A. (2000). The episodic buffer: A new component of working memory? Trends Cogn. Sci. 4, 417–423. doi: 10.1016/S1364-6613(00)01538-2
Baddeley, A. (2012). Working memory: Theories, models, and controversies. Annu. Rev. Psychol. 63, 1–29. doi: 10.1146/annurev-psych-120710-100422
Baddeley, A. D., Allen, R. J., and Hitch, G. J. (2011). Binding in visual working memory: The role of the episodic buffer. Neuropsychologia 49, 1393–1400. doi: 10.1016/j.neuropsychologia.2010.12.042
Baker-Henningham, H., Scott, Y., Bowers, M., and Francis, T. (2019). Evaluation of a violence-prevention programme with jamaican primary school teachers: A cluster randomised trial. Int. J. Environ. Res. Public Health 16:2797. doi: 10.3390/ijerph16152797
Banerjee, R., and Horn, E. (2013). Supporting classroom transitions between daily routines: Strategies and tips. Young Except. Children 16, 3–14. doi: 10.1177/1096250612446853
Barkley, R. A. (1997). Behavioral inhibition, sustained attention, and executive functions: Constructing a unifying theory of ADHD. Psychol. Bull. 121, 65–94. doi: 10.1037/0033-2909.121.1.65
Barkley, R. A. (2015). Attention-Deficit Hyperactivity Disorder: A Handbook for Diagnosis and Treatment, 4th Edn. New York: The Guilford Press.
Berch, D. B., Krikorian, R., and Huha, E. M. (1998). The corsi block-tapping task: Methodological and theoretical considerations. Brain Cogn. 38, 317–338. doi: 10.1006/brcg.1998.1039
Bernaerts, I., Magez, W., D’Haenens, E., Koomen, H., and Verschueren, K. (2015). Leerling Leerkracht Relatie Vragenlijst: Normering Vlaams Basisonderwijs. Houten: Bohn Stafleu van Loghum.
Best, J. R., and Miller, P. H. (2010). A developmental perspective on executive function. Child Dev. 81, 1641–1660. doi: 10.1111/j.1467-8624.2010.01499.x
Birch, S. H., and Ladd, G. W. (1998). Children’s interpersonal behaviors and the teacher-child relationship. Dev. Psychol. 34, 934–946. doi: 10.1037//0012-1649.34.5.934
Blackwell, K. A., Cepeda, N. J., and Munakata, Y. (2009). When simple things are meaningful: Working memory strength predicts children’s cognitive flexibility. J. Exp. Child Psychol. 103, 241–249. doi: 10.1016/j.jecp.2009.01.002
Bowlby, J. (1982). Attachment and loss: Retrospect and prospect. Am. J. Orthopsychiatry 52, 664–678. doi: 10.1111/j.1939-0025.1982.tb01456.x
Brady, K., Forton, M. B., and Porter, D. (2015). Rules in School: Teaching Discipline in the Responsive Classroom. Massachusetts: Center for Responsive Schools, Inc.
Brunetti, R., Del Gatto, C., and Delogu, F. (2014). eCorsi: Implementation and testing of the Corsi block-tapping task for digital tablets. Front. Psychol. 5:939. doi: 10.3389/fpsyg.2014.00939
Cabell, S. Q., Justice, L. M., McGinty, A. S., DeCoster, J., and Forston, L. D. (2015). Teacher–child conversations in preschool classrooms: Contributions to children’s vocabulary development. Early Childh. Res. Quart. 30, 80–92. doi: 10.1016/j.ecresq.2014.09.004
Capodieci, A., Ruffini, C., Frascari, A., Rivella, C., Bombonato, C., Giaccherini, S., et al. (2023). Executive functions in children with specific learning disorders: Shedding light on a complex profile through teleassessment. Res. Dev. Disabil. 142, 104621–104621. doi: 10.1016/j.ridd.2023.104621
Cappella, E., Hamre, B. K., Kim, H. Y., Henry, D. B., Frazier, S. L., Atkins, M. S., et al. (2012). Teacher consultation and coaching within mental health practice: Classroom and child effects in urban elementary schools. J. Consul. Clin. Psychol. 80, 597–610. doi: 10.1037/a0027725
Carlson, S. M. (2023). Let me choose: The role of choice in the development of executive function skills. Curr. Dir. Psychol. Sci. 32, 220–227. doi: 10.1177/09637214231159052
Carriedo, N., Corral, A., Montoro, P. R., Herrero, L., and Rucián, M. (2016). Development of the updating executive function: From 7-year-olds to young adults. Dev. Psychol. 52, 666–678. doi: 10.1037/dev0000091
Carter, D. R., and Pool, J. L. (2012). Appropriate social behavior: Teaching expectations to young children. Early Childh. Educ. J. 40, 315–321. doi: 10.1007/s10643-012-0516-y
Chevalère, J., Cazenave, L., Wollast, R., Berthon, M., Martinez, R., Mazenod, V., et al. (2022). The influence of socioeconomic status, working memory and academic self-concept on academic achievement. Eur. J. Psychol. Educ. 38, 287–309. doi: 10.1007/s10212-022-00599-9
Chorpita, B. F., Daleiden, E. L., and Weisz, J. R. (2005). Identifying and selecting the common elements of evidence based interventions: A distillation and matching model. Mental Health Serv. Res. 7, 5–20. doi: 10.1007/s11020-005-1962-6
Chuang, C., Reinke, W. M., and Herman, K. C. (2020). Effects of a universal classroom management teacher training program on elementary children with aggressive behaviors. School Psychol. 35, 128–136. doi: 10.1037/spq0000351
Cohen, J. (1988). Statistical Power Analysis for the Behavioral Sciences, 2nd Edn. New Jersey: Lawrence Erlbaum Associates, Publishers.
Colmar, S., Double, K., Davis, N., Sheldon, L., Phillips, N., Cheng, M., et al. (2020). Memory mates: An evaluation of a classroom-based, student-focused working memory intervention. J. Psychol. Counsellors Schools 30, 159–171. doi: 10.1017/jgc.2020.9
Conroy, M. A., Sutherland, K. S., Snyder, A. L., and Marsh, S. (2008). Classwide interventions: Effective instruction makes a difference. Teach. Except. Children 40, 24–30. doi: 10.1177/004005990804000603
Constantinidis, C., and Klingberg, T. (2016). The neuroscience of working memory capacity and training. Nat. Rev. Neurosci. 17, 438–449. doi: 10.1038/nrn.2016.43
Corkill, A. J. (1992). Advance organizers: Facilitators of recall. Educ. Psychol. Rev. 4, 33–68. doi: 10.1007/BF01322394
Corsi, P. (1972). Memory and the Medial Temporal Region of the Brain. Doctoral Thesis in Philosophy. Montreal: McGill University.
Cortés Pascual, A., Moyano, N., and Robres, A. Q. (2019). The relationship between executive functions and academic performance in primary education: Review and meta-analysis. Front. Psychol. 10:1582. doi: 10.3389/fpsyg.2019.01582
Cowan, N. (1999). “An embedded-processes model of working memory,” in Models of Working Memory, eds A. Miyake and P. Shah (Cambridge: Cambridge University Press), 62–101. doi: 10.1017/CBO9781139174909.006
Cowan, N. (2001). The magical number 4 in short-term memory: A reconsideration of mental storage capacity. Behav. Brain Sci. 24, 87–114. doi: 10.1017/S0140525X01003922
Croce, K. M., and Salter, J. S. (2022). Beyond the walls: Establishing classroom expectations in a virtual classroom. Front. Educ. 7:816007. doi: 10.3389/feduc.2022.816007
Crosnoe, R., Morrison, F., Burchinal, M., Pianta, R. C., Keating, D. P., Friedman, S. L., et al. (2010). Instruction, teacher–student relations, and math achievement trajectories in elementary school. J. Educ. Psychol. 102, 407–417. doi: 10.1037/a0017762
Daniel, K. (2005). Advance Organizers: Activating and Building Schema for More Successful Learning in Students with Disabilities. Lynchburg, VA: University of Lynchburg.
Davidson, C., Shing, Y. L., McKay, C., Rafetseder, E., and Wijeakumar, S. (2023). The first year in formal schooling improves working memory and academic abilities. Dev. Cogn. Neurosci. 60, 101205–101205. doi: 10.1016/j.dcn.2023.101205
Davis, E. A., and Linn, M. C. (2000). Scaffolding students’ knowledge integration: Prompts for reflection in KIE. Int. J. Sci. Educ. 22, 819–837. doi: 10.1080/095006900412293
Davis, N., Sheldon, L., and Colmar, S. (2013). Memory mates: A classroom-based intervention to improve attention and working memory. Aust. J. Guidance Counsell. 24, 111–120. doi: 10.1017/jgc.2013.23
de Wilde, A., Koot, H. M., and van Lier, P. A. (2015). Developmental links between children’s working memory and their social relations with teachers and peers in the early school years. J. Abnormal Child Psychol. 44, 19–30. doi: 10.1007/s10802-015-0053-4
Dekker, M. C., Ziermans, T. B., Spruijt, A. M., and Swaab, H. (2017). Cognitive, parent and teacher rating measures of executive functioning: Shared and unique influences on school achievement. Front. Psychol. 8:48. doi: 10.3389/fpsyg.2017.00048
Demetriou, E. A., Lampit, A., Quintana, D. S., Naismith, S. L., Song, Y. J. C., Pye, J. E., et al. (2018). Autism spectrum disorders: A meta-analysis of executive function. Mol. Psychiatry 23, 1198–1204. doi: 10.1038/mp.2017.75
Diamond, A. (2013). Executive functions. Annu. Rev. Psychol. 64, 135–168. doi: 10.1146/annurev-psych-113011-143750
Diamond, A., and Ling, D. S. (2016). Conclusions about interventions, programs, and approaches for improving executive functions that appear justified and those that, despite much hype, do not. Dev. Cogn. Neurosci. 18, 34–48. doi: 10.1016/j.dcn.2015.11.005
Diamond, A., and Ling, D. S. (2020). “Review of the evidence on, and fundamental questions about, efforts to improve executive functions, including working memory,” in Cognitive and Working Memory Training: Perspectives from Psychology, Neuroscience, and Human Development, eds J. M. Novick, M. F. Bunting, M. R. Dougherty, and R. W. Engle (Oxford: Oxford University Press), 143–431. doi: 10.1093/oso/9780199974467.003.0008
Dörr, L., and Perels, F. (2020). Improving young children’s self-regulated learning using a combination of direct and indirect interventions. Early Child Dev. Care 190, 2581–2593. doi: 10.1080/03004430.2019.1595608
Downer, J., Sabol, T. J., and Hamre, B. (2010). Teacher–child interactions in the classroom: Toward a theory of within- and cross-domain links to Children’s developmental outcomes. Early Educ. Dev. 21, 699–723. doi: 10.1080/10409289.2010.497453
Dweck, C. S. (2000). Self-theories: Their Role in Motivation, Personality, and Development. Hove: Psychology Press.
Elliott, J. G., Gathercole, S. E., Alloway, T. P., Holmes, J., and Kirkwood, H. (2010). An evaluation of a classroom-based intervention to help overcome working memory difficulties and improve long-term academic achievement. J. Cogn. Educ. Psychol. 9, 227–250. doi: 10.1891/1945-8959.9.3.227
Emmer, E. T., and Stough, L. M. (2001). Classroom management: A critical part of educational psychology, with implications for teacher education. Educ. Psychol. 36, 103–112. doi: 10.1207/S15326985EP3602_5
Evanovich, L. L., and Kern, L. (2018). Precorrection: Preventing predictable problem behaviors in school settings. Beyond Behav. 27, 90–98. doi: 10.1177/1074295618769892
Faul, A., Stepensky, K., and Simonsen, B. (2011). The effects of prompting appropriate behavior on the off-task behavior of two middle school students. J. Positive Behav. Interventions 14, 47–55. doi: 10.1177/1098300711410702
Ferguson, H. J., Brunsdon, V. E. A., and Bradford, E. E. F. (2021). The developmental trajectories of executive function from adolescence to old age. Sci. Rep. 11, 1382–1382. doi: 10.1038/s41598-020-80866-1
Ford, A. (2019). Examining and improving classroom environments through the lens of self-determination theory. Crit. Questions Educ. 10:65.
Frewen, P. A., Evans, B., Goodman, J., Halliday, A., Boylan, J., Moran, G., et al. (2013). Development of a Childhood Attachment and Relational Trauma Screen (CARTS): A relational-socioecological framework for surveying attachment security and childhood trauma history. Eur. J. Psychotraumatol. 4, 1–17. doi: 10.3402/ejpt.v4i0.20232
García-Rodríguez, L., Iriarte Redín, C., and Reparaz Abaitua, C. (2023). Teacher-student attachment relationship, variables associated, and measurement: A systematic review. Educ. Res. Rev. 38:100488. doi: 10.1016/j.edurev.2022.100488
Gathercole, S. E., and Alloway, T. P. (2008). Working Memory and Learning: A Practical Guide. Thousand Oaks, CA: Sage Press.
Gathercole, S. E., Pickering, S. J., Ambridge, B., and Wearing, H. (2004). The structure of working memory from 4 to 15 years of age. Dev. Psychol. 40, 177–190. doi: 10.1037/0012-1649.40.2.177
Gerst, E. H., Cirino, P. T., Fletcher, J. M., and Yoshida, H. (2017). Cognitive and behavioral rating measures of executive function as predictors of academic outcomes in children. Child Neuropsychol. 23, 381–407. doi: 10.1080/09297049.2015.1120860
Gioia, G. A., Isquith, P. K., Guy, S. C., and Kenworthy, L. (2015). Behavior Rating Inventory Of Executive Function®, 2nd Edn. Florida: PAR Inc.
Gonzalez-DeHass, A. R., and Willems, P. P. (2016). Nurturing self-regulated learners: Teacher, peer, and parental support of strategy instruction. Educ. Forum 80, 294–309. doi: 10.1080/00131725.2016.1173751
Graziano, P. A., Garb, L. R., Ros, R., Hart, K., and Garcia, A. (2016). Executive functioning and school readiness among preschoolers with externalizing problems: The moderating role of the student–teacher relationship. Early Educ. Dev. 27, 573–589. doi: 10.1080/10409289.2016.1102019
Gregoriadis, A., Grammatikopoulos, V., Tsigilis, N., and Zachopoulou, E. (2020). “Assessing teacher-child relationships: A cultural context perspective,” in Handbook of Research on the Education of Young Children, 4th Edn, ed. O. N. Saracho (Milton Park: Routledge), 322–332. doi: 10.4324/9780429442827-24
Grossman, H. (2004). Classroom Behavior Management for Diverse and Inclusive Schools, 3rd Edn. New York, NY: Rowman and Littlefield Publishers, Inc.
Gus, L., Rose, J., and Gilbert, L. (2015). Emotion Coaching: A universal strategy for supporting and promoting sustainable emotional and behavioural well-being. Educ. Child Psychol. 32, 31–41. doi: 10.53841/bpsecp.2015.32.1.31
Hagger, M. S., Hankonen, N., Chatzisarantis, N. L. D., and Ryan, R. M. (2020). “Changing behavior using self-determination theory,” in The Handbook of Behavior Change, eds M. S. Hagger, L. D. Cameron, K. Hamilton, N. Hankonen, and T. Lintunen (Cambridge: Cambridge University Press), 104–119.
Hajovsky, D. B., Chesnut, S. R., and Jensen, K. M. (2020). The role of teachers’ self-efficacy beliefs in the development of teacher-student relationships. J. School Psychol. 82, 141–158. doi: 10.1016/j.jsp.2020.09.001
Hall, J. A., Horgan, T. G., and Murphy, N. A. (2019). Nonverbal communication. Annu. Rev. Psychol. 70, 271–294. doi: 10.1146/annurev-psych-010418-103145
Hampshire, A., Sandrone, S., and Hellyer, P. J. (2019). A large-scale, cross-sectional investigation into the efficacy of brain training. Front. Hum. Neurosci. 13:221. doi: 10.3389/fnhum.2019.00221
Hamre, B. K., and Pianta, R. C. (2001). Early teacher-child relationships and the trajectory of children’s school outcomes through eighth grade. Child Dev. 72, 625–638. doi: 10.1111/1467-8624.00301
Hamre, B. K., and Pianta, R. C. (2005). Can instructional and emotional support in the first-grade classroom make a difference for children at risk of school failure? Child Dev. 76, 949–967. doi: 10.1111/j.1467-8624.2005.00889.x
Hamre, B. K., and Pianta, R. C. (2007). “Learning opportunities in preschool and early elementary classrooms,” in School Readiness and the Transition to Kindergarten in the Era of Accountability, eds R. C. Pianta, M. J. Cox, and K. L. Snow (Baltimore, MA: Paul H. Brookes Publishing Co), 49–83. doi: 10.1007/s11121-015-0570-y
Hamre, B. K., Pianta, R. C., Downer, J. T., DeCoster, J., Mashburn, A. J., Jones, S. M., et al. (2013). Teaching through interactions: Testing a developmental framework of teacher effectiveness in over 4,000 classrooms. Elementary School J. 113, 461–487. doi: 10.1086/669616
Hamre, B., Hatfield, B., Pianta, R., and Jamil, F. (2014). Evidence for general and domain-specific elements of teacher-child interactions: Associations with preschool children’s development. Child Dev. 85, 1257–1274. doi: 10.1111/cdev.12184
Hattie, J., and Timperley, H. (2007). The power of feedback. Rev. Educ. Res. 77, 81–112. doi: 10.3102/003465430298487
Hawthorne, B. S., Vella-Brodrick, D. A., and Hattie, J. (2019). Well-being as a cognitive load reducing agent: A review of the literature. Front. Educ. 4:121. doi: 10.3389/feduc.2019.00121
Hendry, A., and Scerif, G. (2023). Moulding environmental contexts to optimise neurodiverse executive function performance and development: A goodness-of-fit account. Infant Child Dev. 32:e2448. doi: 10.1002/icd.2448
Holmes, J., Gathercole, S. E., and Dunning, D. L. (2009). Adaptive training leads to sustained enhancement of poor working memory in children. Dev. Sci. 12, F9–F15. doi: 10.1111/j.1467-7687.2009.00848.x
Horner, S. L., Bhattacharyya, S., and O’connor, E. A. (2008). Modeling: It’s more than just imitation. Childh. Educ. 84, 219–222. doi: 10.1080/00094056.2008.10523010
Howe, G. W., Beach, S. R. H., and Brody, G. H. (2010). Microtrial methods for translating gene-environment dynamics into preventive interventions. Prevent. Sci. 11, 343–354. doi: 10.1007/s11121-010-0177-2
Howes, C., Burchinal, M., Pianta, R., Bryant, D., Early, D., Clifford, R., et al. (2008). Ready to learn? Children’s pre-academic achievement in pre-Kindergarten programs. Early Childh. Res. Quart. 23, 27–50. doi: 10.1016/j.ecresq.2007.05.002
Huizinga, M., and Smidts, D. P. (2020). BRIEF-2 Vragenlijst Executieve Functies Voor 5- tot En Met 18-Jarigen. Newburyport, MA: Hogrefe.
Huizinga, M., Baeyens, D., and Burack, J. A. (2018). Editorial: Executive function and education. Front. Psychol. 9:1357. doi: 10.3389/fpsyg.2018.01357
Huizinga, M., Dolan, C. V., and van der Molen, M. W. (2006). Age-related change in executive function: Developmental trends and a latent variable analysis. Neuropsychologia 44, 2017–2036. doi: 10.1016/j.neuropsychologia.2006.01.010
Jaeger, A. J., Shipley, T. F., and Reynolds, S. J. (2017). The roles of working memory and cognitive load in geoscience learning. J. Geosci. Educ. 65, 506–518. doi: 10.5408/16-209.1
Jones, S. M., Bub, K. L., and Raver, C. C. (2013). Unpacking the black box of the chicago school readiness project intervention: The mediating roles of teacher-child relationship quality and self-regulation. Early Educ. Dev. 24, 1043–1064. doi: 10.1080/10409289.2013.825188
Kamins, M. L., and Dweck, C. S. (1999). Person versus process praise and criticism: Implications for contingent self-worth and coping. Dev. Psychol. 35, 835–847. doi: 10.1037/0012-1649.35.3.835
Karasova, J., and Nehyba, J. (2023). Student-centered teacher responses to student behavior in the classroom: A systematic review. Front. Educ. 8:1156530. doi: 10.3389/feduc.2023.1156530
Kattner, F. (2021). Transfer of working memory training to the inhibitory control of auditory distraction. Psychol. Res. 85, 3152–3166. doi: 10.1007/s00426-020-01468-0
Katz, I., and Assor, A. (2007). When choice motivates and when it does not. Educ. Psychol. Rev. 19, 429–442. doi: 10.1007/s10648-006-9027-y
Keilow, M., Holm, A., Friis-Hansen, M., and Kristensen, R. M. (2019). Effects of a classroom management intervention on student selective attention: Results from a randomized controlled trial. School Effect. School Improvement 30, 194–211. doi: 10.1080/09243453.2018.1557701
Kessels, R. P. C., van Zandvoort, M. J. E., Postma, A., Kappelle, L. J., and de Haan, E. H. F. (2000). The corsi block-tapping task: Standardization and normative data. Appl. Neuropsychol. 7, 252–258. doi: 10.1207/S15324826AN0704_8
Kincade, L., Cook, C., and Goerdt, A. (2020). Meta-analysis and common practice elements of universal approaches to improving student-teacher relationships. Rev. Educ. Res. 90, 710–748. doi: 10.3102/0034654320946836
Kirk, M., Tytler, R., and White, P. (2023). Critical thinking in primary science through a guided inquiry pedagogy: A semiotic perspective. Teach. Teach. Theory Pract. 29, 615–637. doi: 10.1080/13540602.2023.2191181
Klingberg, T., Fernell, E., Olesen, P. J., Johnson, M., Gustafsson, P., Dahlström, K., et al. (2005). Computerized training of working memory in children with ADHD-A randomized, controlled trial. J. Am. Acad. Child Adolesc. Psychiatry 44, 177–186. doi: 10.1097/00004583-200502000-00010
Kokko, A. K., and Hirsto, L. (2021). From physical spaces to learning environments: Processes in which physical spaces are transformed into learning environments. Learn. Environ. Res. 24, 71–85. doi: 10.1007/s10984-020-09315-0
Kolk, S. M., and Rakic, P. (2022). Development of prefrontal cortex. Neuropsychopharmacology 47, 41–57. doi: 10.1038/s41386-021-01137-9
Koomen, H. M. Y., Verschueren, K., and Pianta, R. C. (2007). Leerling Leerkracht Relatie Vragenlijst, Handleiding. Houten: Bohn Stafleu van Loghum.
Koomen, H. M. Y., Verschueren, K., van Schooten, E., Jak, S., and Pianta, R. C. (2012). Validating the student-teacher relationship scale: Testing factor structure and measurement invariance across child gender and age in a dutch sample. J. School Psychol. 50, 215–234. doi: 10.1016/j.jsp.2011.09.001
Korzeniowski, C., Ison, M. S., and Difabio de Anglat, H. (2021). “A summary of the developmental trajectory of executive functions from birth to adulthood,” in Psychiatry and Neuroscience Update, ed. H. L. M. Arroyo (Berlin: Springer International Publishing), 459–473. doi: 10.1007/978-3-030-61721-9_33
Li, D., and Zhang, L. (2023). Contextualizing feedback in L2 writing: The role of teacher scaffolding (vol 31, p. 328, 2022). Lang. Awareness 32, 385–385. doi: 10.1080/09658416.2023.2176584
Liu, H., Liu, Q., Du, X., Liu, J., Hoi, C. K. W., and Schumacker, R. E. (2023). Teacher-student relationship as a protective factor for socioeconomic status, students’ self-efficacy and achievement: A multilevel moderated mediation analysis. Curr. Psychol. 42, 3268–3283. doi: 10.1007/s12144-021-01598-7
Lombardi, L., Mednick, F. J., De Backer, F., and Lombaerts, K. (2021). Fostering critical thinking across the primary school’s curriculum in the european schools system. Educ. Sci. 11:505. doi: 10.3390/educsci11090505
Longobardi, C., Prino, L. E., Settanni, M., and Fabris, M. A. (2024). Attachment to teacher, internalizing and externalizing symptoms: The mediating role of executive functions. Early Child Dev. Care 194, 134–146. doi: 10.1080/03004430.2023.2286900
Mattera, S., Rojas, N. M., Morris, P. A., and Bierman, K. (2021). Promoting EF with preschool interventions: Lessons learned from 15 years of conducting large-scale studies. Front. Psychol. 12:640702. doi: 10.3389/fpsyg.2021.640702
Mayer, R. E. (2002). Rote versus meaningful learning. Theory Into Pract. 41, 226–232. doi: 10.1207/s15430421tip4104_4
McIntosh, K., Herman, K., Sanford, A., McGraw, K., and Florence, K. (2004). Teaching transitions: Techniques for promoting success between lessons. Teach. Except. Children 37, 32–38. doi: 10.1177/004005990403700104
Melby-Lervåg, M., and Hulme, C. (2013). Is working memory training effective? A meta-analytic review. Dev. Psychol. 49, 270–291. doi: 10.1037/a0028228
Melby-Lervåg, M., Redick, T. S., and Hulme, C. (2016). Working memory training does not improve performance on measures of intelligence or other measures of “Far Transfer”: Evidence from a meta-analytic review. Perspect. Psychol. Sci. 11, 512–534. doi: 10.1177/1745691616635612
Miles, J., and Shevlin, M. (2001). Applying Regression and Correlation: A Guide for Students and Researchers. Thousand Oaks, CA: Sage.
Miller, P. W. (1988). Non Verbal Communication. What Research Says to the Teacher, 3rd edn. Washington, DC: National Education Association.
Moore, T. C., Alpers, A. J., Rhyne, R., Coleman, M. B., Gordon, J. R., Daniels, S., et al. (2018). Brief prompting to improve classroom behavior: A first-pass intervention option. J. Posit. Behav. Interv. 21, 30–41. doi: 10.1177/1098300718774881
Morgan, P. L., Farkas, G., Hillemeier, M. M., Pun, W. H., and Maczuga, S. (2019). Kindergarten children’s executive functions predict their second-grade academic achievement and behavior. Child Development 90, 1802–1816. doi: 10.1111/cdev.13095
Morra, S., and Borella, E. (2015). Working memory training: From metaphors to models. Front. Psychol. 6:1097. doi: 10.3389/fpsyg.2015.01097
Mueller, C. M., and Dweck, C. S. (1998). Praise for intelligence can undermine children’s motivation and performance. J. Pers. Soc. Psychol. 75, 33–52. doi: 10.1037/0022-3514.75.1.33
Murray, D. W., Rabiner, D. L., Kuhn, L., Pan, Y., and Sabet, R. F. (2018). Investigating teacher and student effects of the Incredible years classroom management program in early elementary school. J. Schl. Psychol. 67, 119–133. doi: 10.1016/j.jsp.2017.10.004
Myers, D., Freeman, J., Simonsen, B., and Sugai, G. (2017). Classroom management with exceptional learners. Teach. Except. Children 49, 223–230. doi: 10.1177/0040059916685064
Nguyen, T. D., Pham, L. D., Crouch, M., and Springer, M. G. (2020). The correlates of teacher turnover: An updated and expanded meta-analysis of the literature. Educ. Res. Rev. 31:100355. doi: 10.1016/j.edurev.2020.100355
Nordling, J. K., Boldt, L. J., O’Bleness, J., and Kochanska, G. (2016). Effortful control mediates relations between children’s attachment security and their regard for rules of conduct. Soc. Dev. 25, 268–284. doi: 10.1111/sode.12139
Nyberg, L., Brocki, K., Tillman, C., and Bohlin, G. (2009). The proposed interaction between working memory and inhibition. Eur. J. Cogn. Psychol. 21, 84–111. doi: 10.1080/09541440701862133
Nye, E., Gardner, F., Hansford, L., Edwards, V., Hayes, R., and Ford, T. (2016). Classroom behaviour management strategies in response to problematic behaviours of primary school children with special educational needs: Views of special educational needs coordinators. Emot. Behav. Difficulties 21, 43–60. doi: 10.1080/13632752.2015.1120048
O’Reilly, C., Devitt, A., and Hayes, N. (2022). Critical thinking in the preschool classroom - A systematic literature review. Think. Skills Creativity 46:101110. doi: 10.1016/j.tsc.2022.101110
Paas, F., and van Merriënboer, J. J. G. (2020). Cognitive-load theory: Methods to manage working memory load in the learning of complex tasks. Curr. Dir. Psychol. Sci. J. Am. Psychol. Soc. 29, 394–398. doi: 10.1177/0963721420922183
Paas, F., van Gog, T., and Sweller, J. (2010). Cognitive load theory: New conceptualizations, specifications, and integrated research perspectives. Educ. Psychol. Rev. 22, 115–121. doi: 10.1007/s10648-010-9133-8
Park, H., Doh, H., Lee, E., Park, H., and Ahn, W. Y. (2023). The neurocognitive role of working memory load when Pavlovian motivational control affects instrumental learning. PLoS Comput. Biol. 19:e1011692. doi: 10.1371/journal.pcbi.1011692
Pascoe, L., Spencer-Smith, M., Giallo, R., Seal, M. L., Georgiou-Karistianis, N., Nosarti, C., et al. (2018). Intrinsic motivation and academic performance in school-age children born extremely preterm: The contribution of working memory. Learn. Individ. Differ. 64, 22–32. doi: 10.1016/j.lindif.2018.04.005
Paul, R., and Elder, L. (2008). The Miniature Guide to Critical Thinking Concepts and Tools. Santa Barbara, CA: Foundation for Critical Thinking Press.
Payne, R. (2015). Using rewards and sanctions in the classroom: Pupils’ perceptions of their own responses to current behaviour management strategies. Educ. Rev. 67, 483–504. doi: 10.1080/00131911.2015.1008407
Peng, P., and Kievit, R. A. (2020). The development of academic achievement and cognitive abilities: A bidirectional perspective. Child Dev. Perspect. 14, 15–20. doi: 10.1111/cdep.12352
Peng, P., Barnes, M., Wang, C., Wang, W., Li, S., Swanson, H. L., et al. (2018). A meta-analysis on the relation between reading and working memory. Psychol. Bull. 144, 48–76. doi: 10.1037/bul0000124
Peng, P., Namkung, J., Barnes, M., and Sun, C. (2016). A meta-analysis of mathematics and working memory: Moderating effects of working memory domain, type of mathematics skill, and sample characteristics. J. Educ. Psychol. 108, 455–473. doi: 10.1037/edu0000079
Pergher, V., Shalchy, M. A., Pahor, A., Van Hulle, M. M., Jaeggi, S. M., and Seitz, A. R. (2019). Divergent research methods limit understanding of working memory training. J. Cogn. Enhanc. Towards Integr. Theory Pract. 4, 100–120. doi: 10.1007/s41465-019-00134-7
Pianta, R. C. (2001). Student-Teacher Relationship Scale: Professional Manual. Odessa, FL: Psychological Assessment Resources, Inc.
Pianta, R. C., Hamre, B. K., and Allen, J. P. (2012). “Teacher-student relationships and engagement: Conceptualizing, measuring, and improving the capacity of classroom interactions,” in Handbook of Research on Student Engagement, eds S. L. Christenson, A. L. Reschly, and C. Wylie (Berlin: Springer Science + Business Media), 365–386. doi: 10.1007/978-1-4614-2018-7_17
Pianta, R. C., Hamre, B. K., Downer, J. T., Burchinal, M., Williford, A. P., LoCasale-Crouch, J., et al. (2017). Early childhood professional development: Coaching and coursework effects on indicators of children’s school readiness. Early Educ. Dev. 28, 956–975. doi: 10.1080/10409289.2017.1319783
Pianta, R. C., La Paro, K. M., and Hamre, B. K. (2008). Classroom Assessment Scoring System [CLASS] Manual: Pre-K. Baltimore, MA: Brookes Publishing.
Ponitz, C. C., McClelland, M. M., Matthews, J. S., and Morrison, F. J. (2009). A structured observation of behavioral self-regulation and its contribution to kindergarten outcomes. Dev. Psychol. 45, 605–619. doi: 10.1037/a0015365
Powers, K. L., Brooks, P. J., Aldrich, N. J., Palladino, M. A., and Alfieri, L. (2013). Effects of video-game play on information processing: A meta-analytic investigation. Psychon. Bull. Rev. 20, 1055–1079. doi: 10.3758/s13423-013-0418-z
Ramdass, D., and Zimmerman, B. J. (2011). Developing self-regulation skills: The important role of homework. J. Adv. Acad. 22, 194–218. doi: 10.1177/1932202X1102200202
Randall, L., and Tyldesley, K. (2016). Evaluating the impact of working memory training programmes on children—A systematic review. Educ. Child Psychol. 33, 34–50.
Raver, C. C., Jones, S. M., Li-Grining, C., Zhai, F., Metzger, M. W., and Solomon, B. (2009). Targeting children’s behavior problems in preschool classrooms: A cluster-randomized controlled trial. J. Consult. Clin. Psychol. 77, 302–316. doi: 10.1037/a0015302
Reeve, J. (2012). “A self-determination theory perspective on student engagement,” in Handbook of Research on Student Engagement, eds S. L. Christenson, A. L. Reschly, and C. Wylie (Berlin: Springer Science + Business Media), 149–172. doi: 10.1007/978-1-4614-2018-7_7
Riggs, N. R., Blair, C. B., and Greenberg, M. T. (2003). Concurrent and 2-year longitudinal relations between executive function and the behavior of 1st and 2nd grade children. Child Neuropsychol. 9, 267–276. doi: 10.1076/chin.9.4.267.23513
Rimm-Kaufman, S. E., Curby, T. W., Grimm, K. J., Nathanson, L., and Brock, L. L. (2009). The contribution of children’s self-regulation and classroom quality to children’s adaptive behaviors in the kindergarten classroom. Dev. Psychol. 45, 958–972. doi: 10.1037/a0015861
Rimm-Kaufman, S. E., Larsen, R. A., Baroody, A. E., Curby, T. W., Ko, M., Thomas, J. B., et al. (2014). Efficacy of the responsive classroom approach: Results from a 3-year, longitudinal randomized controlled trial. Am. Educ. Res. J. 52, 567–603. doi: 10.3102/0002831214523821
Ripp, I., Emch, M., Wu, Q., Lizarraga, A., Udale, R., von Bastian, C. C., et al. (2022). Adaptive working memory training does not produce transfer effects in cognition and neuroimaging. Transl. Psychiatry 12, 512–512. doi: 10.1038/s41398-022-02272-7
Robbie, K., Santiago-Rosario, M., Yanek, K., Kern, L., Meyer, B., Morris, K., et al. (2022). Creating a Classroom Teaching Matrix. Center on Positive Behavioral Interventions and Supports. Eugene, OR: University of Oregon.
Roberts, G., Quach, J., Spencer-Smith, M., Anderson, P. J., Gathercole, S., Gold, L., et al. (2016). Academic outcomes 2 years after working memory training for children with low working memory: A randomized clinical trial. JAMA Pediatr. 170:e154568. doi: 10.1001/jamapediatrics.2015.4568
Rodas, J. A., Asimakopoulou, A. A., and Greene, C. M. (2024). Can we enhance working memory? Bias and effectiveness in cognitive training studies. Psychon. Bull. Rev. 31, 1891–1914. doi: 10.3758/s13423-024-02466-8
Roorda, D., Koomen, H. M., Spilt, J. L., and Oort, F. J. (2011). The influence of affective teacher-student relationships on students’ school engagement and achievement: A meta-analytic approach. Rev. Educ. Res. 81, 493–529. doi: 10.3102/0034654311421793
Rosenshine, B. (2012). Principles of instruction: Research-based strategies that all teachers should know. Educ. Digest 78:30.
Rowe, A., Titterington, J., Holmes, J., Henry, L., and Taggart, L. (2019). Interventions targeting working memory in 4–11 year olds within their everyday contexts: A systematic review. Dev. Rev. 52, 1–23. doi: 10.1016/j.dr.2019.02.001
Rusk, N., and Rothbaum, F. (2010). From stress to learning: Attachment theory meets goal orientation theory. Rev. General Psychol. 14, 31–43. doi: 10.1037/a0018123
Ruzek, E. A., Hafen, C. A., Allen, J. P., Gregory, A., Mikami, A. Y., and Pianta, R. C. (2016). How teacher emotional support motivates students: The mediating roles of perceived peer relatedness, autonomy support, and competence. Learn. Instruction 42, 95–103. doi: 10.1016/j.learninstruc.2016.01.004
Ryan, R. M., and Deci, E. L. (2000). Self-determination theory and the facilitation of intrinsic motivation, social development, and well-being. Am. Psychol. 55, 68–78. doi: 10.1037/0003-066X.55.1.68
Ryan, R. M., and Deci, E. L. (2017). Self-Determination Theory: Basic Psychological Needs in Motivation, Development, and Wellness. New York, NY: The Guilford Press.
Ryan, R. M., Deci, E. L., and Vansteenkiste, M. (2016). “Autonomy and autonomy disturbances in self-development and psychopathology: Research on motivation, attachment, and clinical process,” In Developmental Psychopathology: Theory and Method, 3rd edn, D. Cicchetti ed. 385–438. John Wiley & Sons, Inc. doi: 10.1002/9781119125556.devpsy109
Sala, G., and Gobet, F. (2017). Working memory training in typically developing children: A meta-analysis of the available evidence. Dev. Psychol. 53, 671–685. doi: 10.1037/dev0000265
Sala, G., and Gobet, F. (2020). Cognitive and academic benefits of music training with children: A multilevel meta-analysis. Memory and Cognition 48, 1429–1441. doi: 10.3758/s13421-020-01060-2
Sankalaite, S., Huizinga, M., Dewandeleer, J., Xu, C., de Vries, N., Hens, E., et al. (2021). Strengthening executive function and self-regulation through teacher-student interaction in preschool and primary school children: A systematic review. Front. Psychol. 12:718262. doi: 10.3389/fpsyg.2021.718262
Sankalaite, S., Huizinga, M., Pollé, S., Xu, C., De Vries, N., Hens, E., et al. (2023). A qualitative study into teacher–student interaction strategies employed to support primary school children’s working memory. Educ. Sci. 13:1149. doi: 10.3390/educsci13111149
Schwaighofer, M., Fischer, F., and Bühner, M. (2015). Does working memory training transfer? A meta-analysis including training conditions as moderators. Educ. Psychol. 50, 138–166. doi: 10.1080/00461520.2015.1036274
Shabiralyani, G., Shahzad Hasan, K., Hamad, N., and Iqbal, N. (2015). Impact of visual aids in enhancing the learning process case research. J. Educ. Pract. 6, 226–234.
Shenhav, A., Musslick, S., Lieder, F., Kool, W., Griffiths, T. L., Cohen, J. D., et al. (2017). Toward a rational and mechanistic account of mental effort. Annu. Rev. Neurosci. 40, 99–124. doi: 10.1146/annurev-neuro-072116-031526
Shute, V. J. (2008). Focus on formative feedback. Rev. Educ. Res. 78, 153–189. doi: 10.3102/0034654307313795
Skinner, B. F. (1938). The Behavior of Organisms: An Experimental Analysis. New York, NY: Appleton-Century.
Smid, C. R., Karbach, J., and Steinbeis, N. (2020). Toward a science of effective cognitive training. Curr. Dir. Psychol. Sci. J. Am. Psychol. Soc. 29, 531–537. doi: 10.1177/0963721420951599
Smithers, L. G., Sawyer, A. C. P., Chittleborough, C. R., Davies, N. M., Davey Smith, G., and Lynch, J. W. (2018). A systematic review and meta-analysis of effects of early life non-cognitive skills on academic, psychosocial, cognitive and health outcomes. Nat. Hum. Behav. 2, 867–880. doi: 10.1038/s41562-018-0461-x
Soenens, B., and Vansteenkiste, M. (2020). “Understanding the complexity of praise through the lens of self-determination theory,” In Psychological Perspectives on Praise, E. Brummelman ed. 27–35. Milton Park: Routledge. doi: 10.4324/9780429327667-5
Spiegel, J. A., Goodrich, J. M., Morris, B. M., Osborne, C. M., and Lonigan, C. J. (2021). Relations between executive functions and academic outcomes in elementary school children: A meta-analysis. Psychol. Bull. 147, 329–351. doi: 10.1037/bul0000322
Stefanou, C. R., Perencevich, K. C., DiCintio, M., and Turner, J. C. (2004). Supporting autonomy in the classroom: ways teachers encourage student decision making and ownership. Educ. Psychol. 39, 97–110. doi: 10.1207/s15326985ep3902_2
Stichting Innovatie and Arbeid (2024). Werkbaar Werk in Het Onderwijs 2023. SECTORALE Analyse op de Vlaamse Werkbaarheidsmonitor 2004-2023. Brussel: SERV - Sociaal-Economische Raad van Vlaanderen.
Summers, J. J., Davis, H. A., and Hoy, A. W. (2017). The effects of teachers’ efficacy beliefs on students’ perceptions of teacher relationship quality. Learn. Individ. Diff. 53, 17–25. doi: 10.1016/j.lindif.2016.10.004
Sweller, J. (2011). “Cognitive load theory,” in The Psychology of Learning and Motivation: Cognition in Education, eds J. P. Mestre and B. H. Ross (Amsterdam: Elsevier), 37–76. doi: 10.1016/B978-0-12-387691-1.00002-8
Tamnes, C. K., Walhovd, K. B., Grydeland, H., Holland, D., Østby, Y., Dale, A. M., et al. (2013). Longitudinal working memory development is related to structural maturation of frontal and parietal cortices. J. Cogn. Neurosci. 25, 1611–1623. doi: 10.1162/jocn_a_00434
ten Braak, D., Lenes, R., Purpura, D. J., Schmitt, S. A., and Størksen, I. (2021). Why do early mathematics skills predict later mathematics and reading achievement? The role of executive function. J. Exp. Child Psychol. 214:105306. doi: 10.1016/j.jecp.2021.105306
Tepper, D. L., Howell, T. J., and Bennett, P. C. (2022). Executive functions and household chores: Does engagement in chores predict children’s cognition? Australian Occup. Therapy J. 69, 585–598. doi: 10.1111/1440-1630.12822
Thorndike, E. L. (1935). The Psychology of Wants, Interests and Attitudes. New York, NY: Appleton-Century.
Tobia, V., Sacchi, S., Cerina, V., Manca, S., and Fornara, F. (2022). The influence of classroom seating arrangement on children’s cognitive processes in primary school: The role of individual variables. Curr. Psychol. 41, 6522–6533. doi: 10.1007/s12144-020-01154-9
Toplak, M. E., West, R. F., and Stanovich, K. E. (2013). Practitioner review: Do performance-based measures and ratings of executive function assess the same construct? J. Child Psychol. Psychiatry 54, 131–143. doi: 10.1111/jcpp.12001
Torok, M., Rasmussen, V., Wong, Q., Werner-Seidler, A., O’Dea, B., Toumbourou, J., et al. (2019). Examining the impact of the good behaviour game on emotional and behavioural problems in primary school children: A case for integrating well-being strategies into education. Aust. J. Educ. 63, 292–306. doi: 10.1177/0004944119878480
Ullman, H., Almeida, R., and Klingberg, T. (2014). Structural maturation and brain activity predict future working memory capacity during childhood development. J. Neurosci. 34, 1592–1598. doi: 10.1523/JNEUROSCI.0842-13.2014
Ursache, A., Blair, C., Bierman, K., and Nix, R. (2011). Executive Function as a Mediator of Effects on Kindergarten Learning Behaviors one Year After the pre-K Head Start REDI Intervention. Paper presented Paper presented at the Society for Research on Educational Effectiveness conference. Washington, DC.
van Aar, J., Leijten, P., Orobio, de Castro, B., and Overbeek, G. (2017). Sustained, fade-out or sleeper effects? A systematic review and meta-analysis of parenting interventions for disruptive child behavior. Clin. Psychol. Rev. 51, 153–163. doi: 10.1016/j.cpr.2016.11.006
van de Pol, J., Volman, M., and Beishuizen, J. (2010). Scaffolding in teacher—Student interaction: A decade of research. Educ. Psychol. Rev. 22, 271–296. doi: 10.1007/s10648-010-9127-6
van Nooijen, C. C. A., de Koning, B. B., Bramer, W. M., Isahakyan, A., Asoodar, M., Kok, E., et al. (2024). A cognitive load theory approach to understanding expert scaffolding of visual problem-solving tasks: A scoping review. Educ. Psychol. Rev. 36:12. doi: 10.1007/s10648-024-09848-3
Van Stockum, C. A., and DeCaro, M. S. (2020). When working memory mechanisms compete: Predicting cognitive flexibility versus mental set. Cognition 201, 104313–104313. doi: 10.1016/j.cognition.2020.104313
Vandenbroucke, L., Spilt, J., Verschueren, K., and Baeyens, D. (2017a). Keeping the spirits up: The effect of teachers’ and parents’ emotional support on children’s working memory performance. Front. Psychol. 8:512. doi: 10.3389/fpsyg.2017.00512
Vandenbroucke, L., Spilt, J., Verschueren, K., Piccinin, C., and Baeyens, D. (2018a). The classroom as a developmental context for cognitive development: A meta-analysis on the importance of teacher-student interactions for children’s executive functions. Rev. Educ. Res. 88, 125–164. doi: 10.3102/0034654317743200
Vandenbroucke, L., Verschueren, K., and Baeyens, D. (2017b). The development of executive functioning across the transition to first grade and its predictive value for academic achievement. Learn. Instruct. 49, 103–112. doi: 10.1016/j.learninstruc.2016.12.008
Vandenbroucke, L., Verschueren, K., Desoete, A., Aunio, P., Ghesquiere, P., and Baeyens, D. (2018b). ‘Crossing the bridge to elementary school : The development of children’s working memory components in relation to teacher-student relationships and academic achievement’. Early Childh. Res. Quart. 42, 1–10. doi: 10.1016/j.ecresq.2017.08.004
Verburgh, L., Königs, M., Scherder, E. J., and Oosterlaan, J. (2014). Physical exercise and executive functions in preadolescent children, adolescents and young adults: A meta-analysis. Br. J. Sports Med. 48, 973–979. doi: 10.1136/bjsports-2012-091441
Verschueren, K., and Koomen, H. M. Y. (2012). Teacher–child relationships from an attachment perspective. Attachment Hum. Dev. 14, 205–211. doi: 10.1080/14616734.2012.672260
Vervoort, E., Doumen, S., and Verschueren, K. (2015). Children’s appraisal of their relationship with the teacher: Preliminary evidence for construct validity. Eur. J. Dev. Psychol. 12, 243–260. doi: 10.1080/17405629.2014.989984
Vijapur, D., Candido, C., Göçer, Ö, and Wyver, S. (2021). A ten-year review of primary school flexible learning environments: Interior design and ieq performance. Buildings 11:183. doi: 10.3390/buildings11050183
Vygotsky, L. S. (1987). The Collected Works of L. S. Vygotsky, Problems of General Psychology, Vol. 1. New York, NY: Plenum Press.
Wannarka, R., and Ruhl, K. (2008). Seating arrangements that promote positive academic and behavioural outcomes: A review of empirical research. Support Learn. 23, 89–93. doi: 10.1111/j.1467-9604.2008.00375.x
Webster-Stratton, C. (2005). The Incredible Years: A Trouble-Shooting Guide for Parents of Children Aged 2 – 8 Years. Seattle, WA: Seattle: Incredible Years Inc.
Webster-Stratton, C. (2012). Incredible Teachers: Nurturing Children’s Social, Emotional and Academic Competence. Seattle, WA: Seattle: Incredible Years Inc.
Webster-Stratton, C. (2020). “The incredible years parent, teacher and child programs: Foundations and future,” in Designing Evidence-Based Public Health and Prevention Programs: Expert Program Developers Explain the Science and Art, ed. M. E. Feinberg (England: Routledge).
Weisner, T. S. (2001). Children investing in their families: The importance of child obligation in successful development. New Dir. Child Adolesc. Dev. 2001, 77–84. doi: 10.1002/cd.32
Wisniewski, B., Zierer, K., and Hattie, J. (2020). The power of feedback revisited: A meta-analysis of educational feedback research. Front. Psychol. 10:3087. doi: 10.3389/fpsyg.2019.03087
Wolf, S. (2019). Year 3 follow-up of the ‘Quality Preschool for Ghana’ interventions on child development. Dev. Psychol. 55, 2587–2602. doi: 10.1037/dev0000843
Wolf, S., Aber, J. L., Behrman, J. R., and Peele, M. (2019). Longitudinal causal impacts of preschool teacher training on Ghanaian children’s school readiness: Evidence for persistence and fade-out. Dev. Sci. 22:e12878. doi: 10.1111/desc.12878
Wolf, S., Aber, J. L., Behrman, J. R., and Tsinigo, E. (2018). Experimental impacts of the “Quality Preschool for Ghana” interventions on teacher professional well-being, classroom quality, and children’s school readiness. J. Res. Educ. Effect. 12, 10–37. doi: 10.1080/19345747.2018.1517199
Xu, C., Huizinga, M., Tekelia Ekubagewargies, D., Soetaert, J., Van Den Noortgate, W., and Baeyens, D. (2024). The relation between teacher–student interaction and executive function performance in children: A cross-cultural meta-analysis. Educ. Psychol. 59, 195–215. doi: 10.1080/00461520.2024.2315527
Zajac, K., and Kobak, R. (2006). “Attachment,” in Children’s Needs III: Development, Prevention, and Intervention, eds G. G. Bear and K. M. Minke (Bathesda, MA: National Association of School Psychologists), 379–389.
Zeki, C. P. (2009). The importance of non-verbal communication in classroom management. Proc. Soc. Behav. Sci. 1, 1443–1449. doi: 10.1016/j.sbspro.2009.01.254
Zelazo, P. D., and Carlson, S. M. (2020). The neurodevelopment of executive function skills: Implications for academic achievement gaps. Psychol. Neurosci. 13, 273–298. doi: 10.1037/pne0000208
Zelazo, P. D., Blair, C., and Willoughby, M. T. (2016). Executive Function: Implications for Education. Washington, DC: U.S. Department of Education, Institute of Education Sciences.
Keywords: classroom intervention, microtrials, study protocol, working memory, executive functions, teacher-student interactions, primary school
Citation: Pollé S, Sankalaite S, Huizinga M, McClelland M, Spilt JL and Baeyens D (2025) Teacher-student interactions as a pathway to strengthen working memory in primary school students: a microtrial study protocol. Front. Educ. 10:1528805. doi: 10.3389/feduc.2025.1528805
Received: 15 November 2024; Accepted: 26 March 2025;
Published: 11 April 2025.
Edited by:
Aloysius Henry Sequeira, National Institute of Technology Karnataka, IndiaReviewed by:
Vanessa Arán Filippetti, National Scientific and Technical Research Council (CONICET), ArgentinaCopyright © 2025 Pollé, Sankalaite, Huizinga, McClelland, Spilt and Baeyens. This is an open-access article distributed under the terms of the Creative Commons Attribution License (CC BY). The use, distribution or reproduction in other forums is permitted, provided the original author(s) and the copyright owner(s) are credited and that the original publication in this journal is cited, in accordance with accepted academic practice. No use, distribution or reproduction is permitted which does not comply with these terms.
*Correspondence: Sophie Pollé, c29waGllLnBvbGxlQGt1bGV1dmVuLmJl
Disclaimer: All claims expressed in this article are solely those of the authors and do not necessarily represent those of their affiliated organizations, or those of the publisher, the editors and the reviewers. Any product that may be evaluated in this article or claim that may be made by its manufacturer is not guaranteed or endorsed by the publisher.
Research integrity at Frontiers
Learn more about the work of our research integrity team to safeguard the quality of each article we publish.