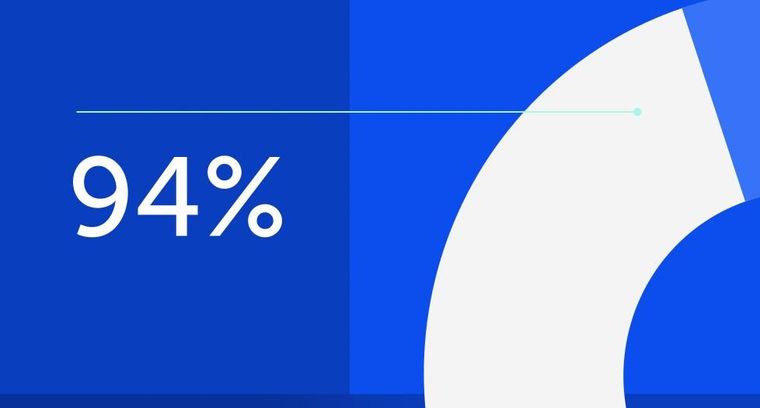
94% of researchers rate our articles as excellent or good
Learn more about the work of our research integrity team to safeguard the quality of each article we publish.
Find out more
REVIEW article
Front. Educ., 17 April 2025
Sec. Higher Education
Volume 10 - 2025 | https://doi.org/10.3389/feduc.2025.1518909
This article is part of the Research TopicEnhancing Public Health Workforce Competencies: AI Integration and Post-Pandemic Educational ReformsView all articles
Higher education institutions are accustomed to sudden and abrupt jolts that provoke poor enrollments, unviable courses, unsustainable practices, budget cuts, and job losses. Such a situation arose with the worldwide education crisis of COVID-19 and the global mandate to shift to online teaching and learning. Policies and guidelines were based on available solutions, often implemented by leaders with limited experience in online education. As a result, the focus was on transitioning to online teaching rather than creating pragmatic policy changes. This article explores the practices in higher education institutions during the COVID-19 pandemic and investigates how the crisis affected teaching and learning across different countries. It also offers insights into the adaptations made during and after the pandemic, particularly within public health education, workforce training, and healthcare, along with actionable suggestions for integrating artificial intelligence (AI) and lessons learned from COVID-19 into these sectors. To highlight the potential benefits of AI in public health education, we discuss how AI-driven epidemiological modeling could play a crucial role in future outbreak preparedness, using the ongoing monkeypox virus (Mpox) outbreaks as a case study. As Mpox continues to emerge as a potential global health threat, the shift to remote learning during the COVID-19 pandemic has demonstrated the importance of preparing the educational system for future uncertainties, including new outbreaks. The pandemic has shown how disruptions can catalyze reforms in public health education, workforce training, and healthcare systems. Looking ahead, AI holds significant potential in transforming epidemic and pandemic preparedness by predicting outbreaks, understanding their trajectories, and even forecasting the individual impact of diseases by analyzing immune responses. Integrating AI into pandemic response frameworks could save lives and strengthen readiness for future health crises.
Universities are bulwarks of a sustained base of acquired insight through decades of scholarly activity. Globally, the coronavirus pandemic affected educational institutions, enterprises, populations, and individuals. The upending of established practices and processes in higher education caused by COVID-19 were directly linked to many factors and considerations, such as the transition from in-person (face-to-face) classes to total remote or hybrid activities, various economic issues, disruption of family-life due to death, disease, distancing, and diminished support structures. Many diverse variables were encountered when implementing and delivering higher education learning online. These included the tight timescale for responding and the sheer number of staff, students and support workers involved. This was compounded by the absence of appropriate infrastructure, a paucity of online teaching experience, the disparity in perspectives on teaching online, and significant time zone differences for students. This transition from in-person to online learning highlighted the strengths and weaknesses of available educational technology. The shift from physical to online learning spaces was sudden and abrupt. It was necessary to review which aspects of the individual lessons and teaching sessions needed modification to make them fit for purpose in the new remote or hybrid environments.
Evaluating whether students had attained certain skill-levels and knowledge-levels required faculty to provide specifications of the defined skills and knowledge desired (i.e., criteria), what experiences to be employed to guarantee students’ learning (i.e., instruction), and determine the performance indicators and assessment of student achievement (i.e., assessment) (Kimmel et al., 2020). As faculty moved from the new classroom environment, the assessment issue became a significant challenge (Korkmaz and Toraman, 2020). Assessments should draw from multiple ways of demonstrating knowledge. It had to be determined if traditional assessments could be transferred to the remote learning space. Flexible assessments must be designed to maximize and demonstrate the student’s accurate learning by achieving specifying skills and knowledge without reducing the expectations for learners.
Teaching online is far from a new notion, and most of its implementation and services have been extensively examined, based on effective research-based teaching methods (Chun and Hargis, 2020; Cossovich and Ermacora, 2021; Hargis et al., 2020; Hargis, 2020; Le Zhou and Hargis, 2020; Shi and Hargis, 2020; Yuan et al., 2022). Believing that the COVID-19 pandemic situation was temporary, some universities did not consider contingency plans, nor did they commit to creating a 21st century method of education and preparing faculty and courses to be taught online. Throughout the COVID-19 pandemic, universities opened around the world offering different educational models, some full F2F, and others partially only. There were still several institutes that have opted not to offer all courses online, keeping students and faculty in academic limbo, and creating anxiety and stress.
This article provides insights views from academics, describing how the transferal to online platforms affected higher education establishments and the perspectives of institutions are drawn from as well as a diverse set of countries’ experiences, caught unaware of COVID-19 viral outbreak situation that is unprecedented and bound to be prolonged in duration. We also describe adaptations made during the COVID-19 and the post-COVID-19 times for public health education, workforce training, and the healthcare system, with objective recommendations for integrating artificial intelligence (AI) into public health education and healthcare. Effective and sustainable enhancements to public health education and healthcare should take account of health determinants from the societal and individual vantage points. Moreover, the dynamic interplay between them should also be assessed.
The ongoing Mpox global outbreak, which caused an excess of 100,000 confirmed cases in more than 122 countries. These include 115 countries with no previous history of Mpox, is discussed herein as an example to illustrate how pandemic preparedness and public health education could be affected and addressed. Advances and developments in AI may potentially complement established methods of preparation and may even generate novel epidemiological approaches, as well as transform many aspects of contemporary science with the possibility of radically disrupting the status quo of epidemiological techniques (Kraemer et al., 2025). On a global scale, AI can potentially solve pertinent epidemiological problems. It may become a crucial component in collecting surveillance data for the routine monitoring of infectious diseases (Kraemer et al., 2025). However, the public health benefits from AI will be predicated upon having free access to data in a representative and ethical manner.
The coronavirus pandemic and the resulting widespread disruptions were unprecedented. Its impact was massive for many private and public areas of the economy, including higher education institutions. As the academic calendar started in late February 2020, countries struggled to treat their citizens of the deadly virus, imposing travel bans to contain SARS-CoV-2 virus spread. Enforced social distancing restrictions and curtailed interpersonal interactions led to business closures and the shutdown of universities and colleges (Sahu et al., 2022). This came with colossal challenges regarding the shift from physical to online teaching spaces. The sudden transition required the successful integration of digital education tools, impacting the organizational response or students’ engagement in a learning environment accessed solely through a screen and a broadband connection. This all had manifest implications for economically disadvantaged teachers, learners and institutes.
Upon the lockdowns and restrictive movement acts declared by governments, the transition to online learning and teaching was less challenging for institutions that adopted the Blended Learning (BL) mode and utilized learning management systems and platforms as conduits between learning material and students. Parity was sought between the old and the new learning methods. Opportunities for “flexible-learning” and “personalized-learning” were now available in seamless learning experiences whilst continually maintaining professional development for academics through the vehicle of online technologies. Online learning had been part of BL, the definition of which became “a thoughtful integration of face-to-face learning experiences with online learning experiences” (Garrison and Kanuka, 2004). BL extrapolates innovation to eLearning based upon online learning material and the continual desire for interactive, personalized and appropriate learning experiences. Through BL, the student may self-regulate and calibrate their learning and help higher education institutions to tailor teaching to the individual learners (Giarla, 2024). The documented challenges of mixed-mode teaching were many; some academicians considered their duties and responsibilities increased bereft of the necessary increases in support. To facilitate faculty embracing online learning, institutions should innovate their pedagogy toward BL and create engaging learning experiences online (Wu et al., 2016). COVID-19 situation also affected many educators who had young children and needed extra effort to adapt their online classes with their family care.
Effective implementation of remote learning creates a pathway for providing a high-quality experience for students, along with practical approaches for assessing online learners. The foundation of successful online instruction lies in adopting the right teaching strategies and leveraging various technology tools that support both synchronous and asynchronous learning formats. There are two primary types of remote learning, each with distinct characteristics:
• Synchronous remote learning, or live-online education, enables students to interact in real-time with instructors and peers (Delgado, 2020). The shift to live online learning accelerated during the COVID-19 pandemic, with platforms like Zoom, WebEx, and Microsoft Teams being widely used. The content delivered in this mode is generally the same, regardless of whether the course is conducted in-person or remotely. Advantages of synchronous learning include minimal preparation for instructors familiar with the course material, a seamless transition to online formats, and the removal of physical social distancing, as all participants are remote. The main drawback, however, is the dependence on technology, internet speeds, and fixed scheduling, which can present challenges for some students.
• Asynchronous remote learning, delivered through platforms like Canvas and Blackboard, relies on the instructor creating and organizing course materials such as videos, readings, and resources in advance, allowing students to engage with the content on their own schedule. Students still learn the same material, but they have greater flexibility in deciding when and where they study. The main benefit of asynchronous learning is that it provides students with the flexibility to learn at their own pace and review content as needed. The disadvantage of asynchronous learning to the students is that they may not feel like they are attending school, and that they oversee their learning.
Assessments play a key role in documenting and demonstrating student progress. They offer valuable feedback to help students understand their strengths and areas for improvement. In the remote learning context, instructors can use traditional assessment methods to gauge students’ understanding of key concepts, skills, or material from lectures, discussions, readings, and assignments. The intended learning outcomes should clearly specify the knowledge (content) and skills students should acquire and be expressed in measurable terms to track student performance. Student feedback allows instructors to reflect on their teaching practices, adjust, and provide targeted feedback based on student progress. In addition to managing the learning experience, instructors must also assess student performance and assign grades. Since remote education lacks universally accepted grading standards, educational institutions must engage in further research and develop effective, authentic grading practices tailored to the online environment.
Distributed learning systems (DLS) represent a multi-media instructional delivery method that includes a combination of video conferencing, web-based instruction, in person contact, audio-video enabled distance learning and a mix of traditional and digital media (Kozlowski and Ilgen, 2006). DLS allows instructors to deliver teaching to learners in numerous localities and allows instructor-student interactions asynchronously or synchronously. Learners with inflexible schedules can use the recorded learning activity sessions and the associated discussions. Learners can also replay and revisit recordings, which was impossible in the legacy lecture format. The provision of supplementary real-time live video, web resources and traditional textbooks can augment the student’s learning; the instructor could provide a “roadmap” of suggestions on how to best use the material.
In most STEM (science, technology, engineering, and mathematics) subjects, there is a significant amount of content for students to absorb. Remote learning in STEM fields becomes more challenging due to the hands-on nature of science and engineering, which often involve laboratory work and team-based projects that require applying the engineering design process to solve complex, open-ended problems (De Jong et al., 2013). Engineering instructors must identify virtual activities that can replicate these hands-on experiences and develop methods for students to collaborate effectively in virtual teams. Instructors can support this by creating a “Quick Guide” that provides a concise summary of the lesson, allowing students to quickly grasp the key concepts before delving into the full material. Both theoretical and practical courses need to be adapted to a virtual format, which requires significant effort from both educators and students. This transition is further complicated by the psychological stress stemming from the broader health, economic, and educational challenges (Kimmel et al., 2020).
In the wake of the COVID-19 pandemic, numerous scholars have examined the impact on higher education teaching and learning. Many universities, particularly those in the US, UK, and Australia, heavily rely on international students, especially those from China. Over the past few years, the number of Chinese students studying abroad has consistently increased, with China becoming the largest source of international students in these countries. However, due to the pandemic, universities with significant Chinese student populations faced the challenge of adjusting academic calendars, postponing semester starts and offering alternative study plans in response to the anticipated loss of tuition revenue from canceled enrollments.
Several universities in the Australasian region are especially dependent on international students, with a significant proportion coming from China. According to data from Australia’s Federal Education Department, 212,000 Chinese students were enrolled in Australian institutions by late 2019, representing 28% of the country’s total international student population (Ziguras and Tran, 2020). The reduction in Chinese student numbers had a lasting impact on Australian higher education, as approximately 106,600 Chinese students were unable to enter the country due to the travel ban, resulting in an estimated loss of 8 billion Australian dollars in tuition fees and revenue for schools, colleges, and universities (Pearlman, 2020).
Australia was not the only country facing this challenge. The US also saw a rapid increase in Chinese students, which contributed to a significant rise in tuition-paying undergraduates. Many US universities began to depend on Chinese students for revenue and formed partnerships with Chinese institutions (Giridharan, 2020). Data from the Institute of International Education revealed that over 300,000 Chinese students were studying in the US during the 2018–2019 academic year, and more than 86,000 were enrolled in the UK in 2019 (Hu and Shuangyi). The pandemic disrupted many Chinese students’ plans to return to the US for the spring semester, as travel restrictions affected US universities that had invested heavily in student recruitment in China. Chinese students represent the largest group of international students in the US, making up just over one-third of all international students in US higher education institutions (Giridharan, 2020).
Spain entered a strict nationwide lockdown by mid-March 2020, as a result of the COVID-19 pandemic. A questionnaire-based study conducted by the University of Malaga highlighted the significant issue COVID-19 has thrust upon higher education, considering the demographic data, access to necessary technology needed to join online classes, the demotivation induced by the new approaches, the changes in the education modes, methods and evaluations, and the online learning as a global experience (Alba-Linero et al., 2020). Among 59 students of education, 56 students of engineering and 211 students of medicine, some of the learners were not living in their usual student homes and returned to their families’ homes, losing their usual student facilities such as libraries, computer labs, study rooms, etc. Other students lived in poor Internet access areas. Depending on their geographical location, a mere 21.5% of learners has “always-good” Internet, 39.2% of them had internet “often,” and 31.6% had “difficulties” with Internet access, whereas 7.7% “never” has the most basic connection to allow a class to be followed online. About one-third of the students had conflicting online learning when they needed to share their electronic equipment with a sibling with the same online education schedule, to study, or to work on projects (Alba-Linero et al., 2020). As reported by others, anxiety levels have been higher than pre-COVID-19 years (Unger and Meiran, 2020), the student stress affected their academic performance, more than 90% of students considered they are less motivated to study and needed to put more effort into their studies compared to their pre-pandemic situation (Alba-Linero et al., 2020). The most impacted areas has been the instruction of practical education components. The worst was for the students of medicine. 21.5% were deprived of any essential practical experience, a mere 48.6% could do a few, and the full quota of practical work could only be completed by 8.1%. Engineering students were less affected in general (Alba-Linero et al., 2020). Students considered that the workload and effort required was greater in the online delivery method relative to in-person teaching and complained about the diversity in online platforms and methods. In all of the groups that were investigated, most students considered that COVID-19 had a major impact on planning and performing group projects and assignments. As reported by another group (Paudel et al., 2021), face-to-face sessions were preferred by approximately 75% of the students, 2% prefer online classes, and 23% favored combining online with face-to-face classes (Alba-Linero et al., 2020).
In Turkey, the COVID-19 pandemic was officially declared on March 10, 2020. In response to the pandemic, universities shifted to a distance education model for the Spring semester of the 2019–2020 academic year. At Isparta University of Applied Sciences, Alba-Linero et al. (2020) conducted a study to gather the perspectives of faculty members on distance education during the Spring 2020 pandemic period. When synchronous courses began on March 30, 2020, a total of 855 faculty members uploaded 13,245 course materials to the student information system, and 2,895 live lessons were conducted (İnce et al., 2020). When comparing distance education to traditional in-person learning, participants expressed a preference for the latter. However, they also acknowledged that distance education could be beneficial for certain courses if the infrastructure and curriculum were well-developed, with adequate training for both instructors and students. The academics reported several drawbacks, such as the inappropriateness of distance education for workshops and laboratory-based courses (noted by 19% of participants), issues with fairness in assessments (18%), and its potential to make students feel isolated (7%), reduce motivation (7%), and negatively impact their personal development (4%). Other concerns included internet connectivity problems (12%), inequality of access among students (10%), and insufficient preparation levels (4%) that hindered socialization.
Regarding future preferences for education delivery, 49% of participants expressed a desire to discontinue distance learning, 23% favored continuing it, and 22% preferred using distance education for the theoretical components of their courses (İnce et al., 2020).
In South Korea, the COVID-19 pandemic prompted higher education institutions and stakeholders to reconsider existing assumptions about higher education and learning. The country’s technological progress has played a crucial role in shaping new visions for education in the post-COVID-19 era, particularly for future generations. In 2015, South Korea launched its Massive Open Online Courses (MOOCs), and currently, 86% of its higher education institutions are private. The widespread use of Information and Communication Technology (ICT) in education could potentially lead to a major transformation in the Korean higher education model, shifting from traditional university education to a system focused on lifelong learning. This would allow learners to access higher education content online, regardless of time or location (Peters et al., 2022).
The Spring 2020 semester served as a testing period for the adaptability and flexibility of South Korea’s higher education system in terms of online teaching and learning. Many universities quickly adopted platforms like Google Classroom, Webex, and Zoom. However, while faculty and staff responded rapidly by offering online classes, this swift transition did not necessarily reflect the readiness, capacity, and financial resources of all institutions. The current state of university-level online learning shows that many institutions continue to face challenges in delivering synchronous online courses, providing appropriate pedagogical support, and offering adequate technical assistance to students. The post-COVID-19 era will require significant changes in South Korea’s higher education system to ensure its survival and transformation, necessitating institutional and educational reforms that question and reevaluate the foundational principles of teaching, learning, and global engagement (Peters et al., 2022).
Due to the COVID-19 pandemic, many universities in the US, including top institutions like MIT, Stanford, Caltech, Chicago, Princeton, Cornell, Pennsylvania, Yale, Columbia, Johns Hopkins, and Duke, shifted from in-person teaching to online learning (Elers, 2020). The disruption caused by the pandemic prompted Boston University (BU) to reevaluate nearly all aspects of its academic and operational structure. In April 2021, BU unveiled its COVID-19 Recovery Plan, which formed multiple working groups to examine every facet of the university’s return to on-campus life. These groups identified the necessary steps for safely resuming academic, research, and residential programs.
Starting with the fall semester of 2021, BU introduced a new hybrid teaching format called “Learn from Anywhere” (LfA), allowing both incoming freshmen and returning undergraduate students to choose between attending classes in person or remotely. The LfA format, approved by a university committee overseeing the campus reopening, provided flexibility and supported the health and safety of students and faculty during the pandemic. It also ensured that all students, regardless of location, had access to the same high-quality education BU is known for. The LfA format was designed to comply with safety measures required by public health officials and to accommodate travel restrictions and other challenges BU students faced. It allowed students to participate in the same classroom discussions, whether they were in a classroom, a BU residence, or another country.
As a result of delays related to COVID-19, many BU students were unable to travel to Boston for the start of the fall 2022 semester. In response, BU offered remote learning options for certain graduate programs. Unlike the spring 2021 semester, when remote learners were graded on a Credit/No Credit basis, grading for the fall 2022 semester returned to the standard format. International students unable to arrive on campus had the option to begin their studies remotely and transition to in-person classes when they arrived at BU.
To facilitate the LfA format, BU upgraded its classrooms with the necessary technology to accommodate remote learning. This initiative was part of a broader effort to safely repopulate the residential campus following strict public health guidelines. The transition to this new learning format varied in difficulty depending on the course, with labs, clinical work, and hands-on instruction posing the greatest challenges. Since then, BU has worked to create a safe environment where students can continue their studies alongside faculty and staff.
During the COVID-19-remote education at the Normal Superior School of Tetouan in Morocco, for 76 students’ first-year University “fluid mechanics” course, a study was conducted to assess the influence of employing the Google Classroom (a learning platform) on the success of students, their motivation and their scientific thinking skills (Sofi and Laafon, 2020). All the students installed Google Classroom on their mobile phones to access their teachers’ distributed educational documents (courses, homework, links, videos, assignments, etc.), comments and exchanges with their teachers. Among the students, 48% have a laptop, 97.5% have a cell phone, 27% have a digital table, and 94.80% have Internet, Wi-Fi or 4G connection. It was easy to incorporate Google Classroom into teaching because all the students had accomplished competency with Information and Communication Technologies. Most (66.2%) students found that each of the task’s processes was user-friendly, and 7.8% reported the procedural steps for using Google Classroom lacked clarity for appropriate monitoring (Sofi and Laafon, 2020). Students reported that Google Classroom had flexibility, ease of access, use from any device (smartphones, desktop, etc.), and operating system, and with the capacity to study outside core class times anywhere at any time, and downloading files in different formats that contributed significantly to their satisfaction with their remote knowledge acquisition. Positive attitudes were expressed by students toward mobile technology; 76.62% reported that Google Classroom helped them improve their problem-solving skills and allowed them to keep in touch with their teachers outside the classroom. This research showed that learning with mobile technology is a workable solution for incorporating technology into remote learning (Sofi and Laafon, 2020).
In Bahrain, the first COVID-19 case was announced on 24 February 2020. The first death was declared on 16 March 2020, the Ministry of Health officially recognized a total of 1,394 deaths, and 276,627 recoveries by December 2021 (World Health Organization, 2021). Besides affecting the economy and society, the pandemic majorly interrupted education. The educational activities of thousands of students and teachers had changed with closures affecting the 11 higher education institutes in Bahrain during the COVID-19 pandemic. To ensure continuity of learning during COVID-19 and due to the closures of institutes, distance and digital learning platforms have become a sharp focus. The new COVID-19 measures mandated that all in-class university activities be abolished at the Royal College of Surgeons in Ireland (RCSI) in Bahrain. Higher education institutions have adopted nationwide, solely remote and distance education. At the RCSI in Bahrain, the university management decided to perform distance education via the HyFlex platform, live courses, and electronic sources.
India implemented a nationwide lockdown in response to the COVID-19 pandemic, impacting nearly every sector of the country, the daily lives of approximately 1.37 billion people, and the entire education system (Mathur, 2020). Mathur (2020) assessed several factors, including the effectiveness of online learning technologies, the challenges faced, the role of institutions, future prospects, and teacher satisfaction with the shift to digital education. Their study revealed that while teachers acknowledged the benefits of online learning—such as helping complete the curriculum, providing richer content, diversifying knowledge, making better use of time, and addressing various challenges, the lack of proper communication infrastructure hindered the smooth transition from traditional in-person education to online formats. The study found that about 68% of teachers had no prior experience with remote teaching before the pandemic, but by the time the lockdown occurred, approximately 84.8% had transitioned to online platforms (Mathur, 2020). In general, teachers expressed satisfaction with the use of digital learning tools and supported their continued use in the future.
The success of online learning during the COVID-19 pandemic was primarily due to the teachers’ adaptability and tenacity (Gokuladas and Sam, 2020). Educators moved more core content online, reserving the discussions and discourse for in-person interaction. General COVID-19 restrictions, limitations in hardware and software availability, and support structures highlighted the desperate imperative for in-person teaching by reestablishing physical schools (Hebebci, 2021). Colleges and universities were dealing with reopening campuses as they considered possible learning models and plans for instruction. COVID-19 brought opportunities to reevaluate the issues and challenges of inequalities, such as access to teaching and learning spaces. The outcome of these reassessments could and should be extended to the post-pandemic period and beyond. It has become common place for educational institutions to have continued remote learning in some guise or another, be that face to face, asynchronous, synchronous or a series of combinations and permutation of hybrids (Burke, 2020; Hooker, 2020). The hybrid model and approach allow for a combination of in-person learning (for selected sections of semesters) supplemented with virtual teaching (for selected sections of semesters). Additionally, it offers small-group collaboration, allowing student teams to start working on a project in the classroom and continue their efforts remotely. Effective assessment methods will be able to show that students have gained the skills and knowledge outlined in the course’s learning outcomes.
The post-modern University in the 21st century, subject to “infinite interpretability” and “super complexity”, needs to prepare students for the challenges faced in professional lives. This includes managing vast amounts of data and theories within various frameworks of understanding, as well as addressing conflicting foundational structures. It is essential that the university prepares students to tackle these challenges with innovative and sustainable solutions for the long term (Barnett, 2000). In the aftermath of the COVID-19 pandemic, many experts and world leaders predict that life will not return to pre-pandemic norms anytime soon, and the world as we once knew it will change (Giridharan, 2020). The future is expected to bring a new economic model that benefits all and foster greater international cooperation on global issues such as climate change (Schifferes, 2020).
In recent years, Open Educational Resources (OER) have gained significant attention, with educators from kindergarten through graduate school increasingly turning to these resources for free textbooks and materials. OERs benefit not only students but also instructors, educational institutions, and curriculum developers, helping to level the playing field for students from low socio-economic backgrounds who cannot afford the high costs of traditional textbooks (Usinski, 2018). As schools and universities have transitioned to online learning, OERs have become an invaluable resource for both students and teachers, providing access to textbooks and supplementary materials. OERs will continue to play a critical role in supporting students who are studying online for extended periods, especially for institutions that remain closed or face the possibility of reclosures (McMurtrie, 2020).
To foster a sense of community, it is recommended that educators establish social media channels for their classes and create brief videos explaining weekly learning topics. However, student anxiety and discomfort may increase in online learning environments, as students may struggle with responding to discussion threads or experience disruptive home environments, such as sharing space with siblings or family members. Regardless of the organizational structure of educational institutions, a successful reopening strategy must be implemented through collaborative efforts and shared responsibility among all stakeholders—students, parents, faculty, administration, and management—who must work together to create a transparent and effective communication system. At the national level, the lack of unified reopening plans has further contributed to confusion and uncertainty among schools regarding the approach to physical reopening (ElSaheli-Elhage, 2020). To ensure that all stakeholders in higher education share equal responsibility and the opportunity to contribute to students’ well-being, and to make the physical reopening of education (along with effective blended learning) a success, higher education institutions and stakeholders must (Gokuladas and Sam, 2020):
• Provide strategic direction and unconditional support for students to return to their education more confidently and enthusiastically and institutionalize a robust Learning Management System to provide blended learning, as it is expected to be the primary pedagogical approach for many higher education institutions. Educators must recognize that blended learning is the future of education and create lesson plans that distinguish which sessions are best suited for in-person and online teaching separately.
• Ensure that both synchronous and asynchronous learning options are available for in-person and online instruction, and that all educators are thoroughly trained in the pedagogical strategies needed to manage the blended learning environment effectively.
• Innovate ways of delivering education that are inclusive of all students and embrace technology-assisted learning to ensure that educators remain accessible beyond the classroom. Ensure that the entire campus infrastructure is prepared for blended learning by providing the necessary hardware and networking resources.
• Maintain regular, transparent, and clear communication with parents, teachers, and students through multiple channels, addressing any concerns related to the reopening of higher education institutions. Update them on the learning environment and its safety measures. Share only accurate and verified information regarding the reopening process and address any misinformation to alleviate public concerns.
• Gather feedback from teachers and staff regarding their preferences for various duties, and train educators to identify students who may face difficulties when returning to campus. Prepare students to engage in peer counseling for those who may have been psychologically affected by the pandemic, empowering them to support their peers and nurture their creativity.
• Shift from a prescriptive approach to an inductive teaching method, helping students connect theoretical concepts to real-world applications. Implement specific classroom activities designed to enhance students’ psychomotor and social skills.
• Use both top-down and bottom-up communication strategies to keep stakeholders informed about classroom activities and outcomes. Listen attentively to their concerns, strive to resolve issues amicably, remain open to constructive feedback, and take the necessary actions to strengthen the reopening process.
• Encourage open discussions about concerns with friends, teachers, and family members to manage mental stress related to the pandemic. Show compassion toward others, approach challenges with patience, and foster unity by developing a positive outlook on life. Follow all safety guidelines and adhere to instructions to ensure a secure and supportive environment at the higher education institution.
Global challenges such as terrorism, pandemics, climate change, and other borderless issues require collaborative, interdisciplinary solutions. Throughout history, pandemics have compelled humanity to break from tradition and reimagine the world. The COVID-19 pandemic presents an opportunity to reshape our universities, which should be spaces for deep, holistic, and transdisciplinary thinking. It provides a chance to reconsider global interconnectedness, interdependence, the fundamental aims of education, and pedagogical models that are better equipped to address the constant uncertainty, risk, change, and complexity of our world. In particular, AI-driven models for healthcare and public health education, along with relevant policy actions, teaching methods, and remote learning platforms, must be further developed and refined, especially in developing nations. These countries often face challenges such as limited internet access, fewer connection points, and high data costs, which restrict both connectivity and affordability.
The global COVID-19 pandemic forced us to reassess previously accepted norms in various areas of life, including home, work, and society. This disruption led to significant changes in how we learn, teach, work, communicate, and collaborate. It also prompted an unprecedented transition from in-person healthcare to remote consultations (Sara, 2022; Uscher-Pines et al., 2021). Additionally, the pandemic created disruptions in medical education for residents and fellows by limiting in-person meetings, which required both learners and educators to adapt to remote learning methods (Rose, 2020; Wayne et al., 2020). These challenges, however, became opportunities for rapid innovation and the effective use of technology to maintain personal safety while preserving, and potentially enhancing, the quality of medical education (Sara, 2022). Technology has increasingly played a pivotal role in teaching clinical skills, with simulation centers and computerized anatomy labs becoming more common (Uchida et al., 2019).
Various academic institutions have reported successful experiences implementing virtual education programs for medical students in specialties such as diagnostic radiology and surgery (Calhoun et al., 2020; Ehrlich et al., 2020; Gomez et al., 2020). A critical component in the success of these educational strategies has been the rise in the use of widely available videoconferencing and remote sharing tools such as Zoom, WebEx, and Skype. These platforms have enabled administrators to record conferences for later viewing, facilitate live chat interactions, and offer educational lectures both internally and globally in an open-access format.
The COVID-19 pandemic presented escalating challenges for healthcare professionals around the world (Razu et al., 2021). Despite these obstacles, healthcare workers have adapted to manage the health crisis. However, providing sufficient support to address the difficulties faced by healthcare professionals was essential for improving overall health outcomes during the pandemic. While social distancing proved to be an effective strategy for containing the spread of COVID-19, implementing it was particularly challenging for healthcare workers who needed to be in direct contact with COVID-19 patients, putting them at high risk of infection (Liu Q. et al., 2020). Frontline healthcare workers were especially vulnerable due to their dedication to controlling the disease (Kola et al., 2021). In addition to physical threats, this public health emergency also took a psychological toll on healthcare professionals, causing stress, fear of infection, and feelings of helplessness (O’Boyle et al., 2006).
Healthcare professionals in many developing countries faced significant informational challenges during the pandemic. For example, Bangladesh, a country with many similarities to the other South Asian Association for Regional Cooperation (SAARC) nations, struggled to address these challenges. The SAARC region consists of South Asian countries such as Afghanistan, Bangladesh, Bhutan, India, Maldives, Nepal, Pakistan, and Sri Lanka, covering 3% of the world’s land area and home to 21% of the global population. As of 2021, SAARC nations represented 5.21% of the global economy, valued at US$4.47 trillion (Fund, 2021).
A recent study by Razu et al. (2021) examined the challenges faced by healthcare workers (doctors and nurses) during the COVID-19 pandemic in Bangladesh. The authors conducted qualitative research at various hospitals and clinics between May and August 2020, which involved 15 in-depth telephone interviews. These interviews were recorded, transcribed, and analyzed using an interview guide. The study identified seven key themes, highlighting several challenges, including increased workloads, psychological stress, inadequate personal protective equipment (PPE), social stigma, lack of incentives, and poor coordination and management in healthcare services. The findings emphasized the urgent need for better safety protocols, adequate protective equipment, and support for both the physical and mental health of healthcare professionals (Razu et al., 2021). In Bangladesh, the ratio of doctors to the population is low, with only 5.26 doctors per 10,000 people, and many healthcare workers were working up to 17 hours per day, including long shifts for tele-counseling (Razu et al., 2021).
During COVID-19, the authors reported (Razu et al., 2021):
• Increased workload: Due to a shortage of medical staff and equipment, healthcare workers faced intense pressure, resulting in mental distress, insomnia, physical weakness, and a fear of contracting the virus (Greenberg et al., 2020; Razu et al., 2021). |
• Lack of resources and coordination: There was insufficient knowledge about the virus, and a lack of coordination among different healthcare administration sections created a chaotic environment. The absence of standardized COVID-19 protocols left both healthcare professionals and patients uncertain about safety measures, heightening the risk of infection. Healthcare workers with children faced emotional distress due to the need to distance themselves from their families to avoid transmitting the virus. Furthermore, there was a severe shortage of masks, gloves, and PPE, and many healthcare professionals were not adequately trained in using them, contributing to a high infection rate among the medical workforce (Mahmood et al., 2020; Shammi et al., 2020). |
• Mental and emotional strain: Healthcare workers suffered from insomnia, loneliness, sleep disturbances, and depression, all of which were compounded by the workload and stress. They experienced anxiety, frustration, and fear of infecting their families. Additionally, they faced stigma from neighbors and relatives who believed healthcare workers were at a higher risk of exposure to COVID-19. Nurses, in particular, expressed dissatisfaction with the lack of recognition for their contributions, despite often providing high-quality care similar to doctors (Laurant et al., 2018; Su et al., 2007). |
When comparing their findings with those from other SAARC countries, Razu et al. (2021) noted significant similarities. The SAARC region, which comprises nations like Bangladesh, India, and Pakistan, already struggles with fragile economies and inadequate healthcare infrastructure (Banerjee et al., 2020). Health workers are unevenly distributed globally, with countries that are least in need having the highest number of healthcare workers, while those most burdened with disease have a much smaller workforce (World Health Organization, 2024). Over 40% of World Health Organization (WHO) member states report having fewer than 10 doctors per 10,000 people, and more than a quarter have fewer than three. In addition, while the African region accounts for 22% of the global disease burden, it has access to only 3% of healthcare workers and less than 1% of global financial resources (World Health Organization, 2024).
Density of doctors (physicians) per 10 000 population for developing and developed countries (World Health Organization, 2024)
Country | Density of Doctors (physicians) per 10 000 population |
Egypt | It improved from 5.1 in 2003 to 6.8 in 2020. |
Morocco | It improved from 5.3 in 2004 to 7.3 in 2017. |
Algeria | It worsened from 11.2 in 2002 to 9.9 in 2019. |
United States of America | It improved from 24.3 in 1995 to 36.1 in 2021. |
China | It improved from 11.3 in 1990 to 25.2 in 2021. |
India | It worsened from 12.2 in 1991 to 7.3 in 2020. |
Australia | It improved from 21.7 in 1990 to 39.8 in 2021. |
Canada | It improved from 24.3 in 2018 to 25 in 2022. |
The Russian Federation | It worsened from 45.1 in 1990 to 38.3 in 2020. |
Denmark | It improved from 24.9 in 1990 to 43.8 in 2020. |
France | It improved from 31.2 in 1990 to 33.4 in 2021. |
Malaysia | It improved from 6.8 in 2000 to 23.2 in 2021. |
Traditional healthcare models often require in-person evaluations, which can involve long visits and expensive testing. In contrast, telemedicine offers a more timely and cost-effective way to provide healthcare services. It presents an opportunity for healthcare providers to conduct evaluations in a simple, affordable, and non-invasive manner, while also minimizing the risk of disease transmission between patients and providers (Sara, 2022). Numerous studies have highlighted the potential of telemedicine to improve healthcare delivery, but the broader implications of a large-scale shift to remote healthcare, and its impact on patient care and outcomes, still require further investigation (Merriel et al., 2014; Sana et al., 2020). During the COVID-19 pandemic, the use of telemedicine in remote clinics surged, establishing it as a more permanent aspect of healthcare delivery (Contreras et al., 2020; Dorsey and Topol, 2016; Hunt and Hooten, 2020).
Given the absence of established guidelines for remote healthcare, there is a real possibility of unintended consequences and limitations. These concerns are particularly relevant for specific patient groups, such as the elderly, racial and ethnic minorities, and those with multiple comorbidities, who may struggle with remote care due to issues like lack of internet access, low technology literacy, or the inability to undergo physical examinations (Sara, 2022). To ensure telemedicine becomes an ethical and secure mode of healthcare delivery, education systems and care providers must establish guidelines that promote accessibility and inclusivity. Some necessary steps include (Farooqi et al., 2021; Sara, 2022):
• Training in digital healthcare tools: This would improve remote patient care and standardize remote healthcare delivery protocols. Guidelines should be developed for regulatory agencies, insurance companies, and private companies that collaborate on telemedicine infrastructure.
• Systematic evaluation of telemedicine: There is a need for thorough assessment of telemedicine practices to evaluate their safety, effectiveness, and feasibility, including clinical trials that involve diverse populations across different income levels.
• Privacy and data protection: Strong measures should be implemented to ensure the safeguarding of patient privacy and data protection in telemedicine systems.
During pandemics, it is critical to implement effective coping strategies, especially in both developed and developing nations. Strengthening healthcare governance can improve response outcomes, by providing:
1. Support for healthcare workers: Providing adequate resources for healthcare workers’ physical and mental well-being is crucial. Reducing their workloads through proper coordination and access to information is essential for ensuring quality care.
2. Healthcare training and facility distribution: Training healthcare professionals and distributing healthcare resources effectively between urban and rural areas, and across public and private sectors, is necessary. Media’s role in this effort should also be enhanced.
3. Increased recruitment and protection for healthcare workers: Recruiting more healthcare workers and ensuring they have access to necessary protective equipment like PPE, oxygen supplies, and intensive care units is crucial.
4. Incentives for healthcare workers: Offering financial and emotional support, as well as sufficient staffing, can motivate healthcare workers to contribute more during health crises.
The demand for precision, speed, and digital interaction, particularly in critical sectors like healthcare, has pushed us toward advanced technological solutions. AI has the potential to revolutionize public health education and healthcare by providing tools that allow practitioners to perform tasks more efficiently (Smith, 2024). The COVID-19 pandemic accelerated the adoption of AI in sectors such as retail, education, and healthcare (Forum, 2021). Forty-one percent of companies expedited their AI strategies to improve remote work, enhance user experiences, and reduce operational costs. Healthcare institutions, in particular, have focused on incorporating AI for virtual interactions (Forum, 2021).
Achieving AI full potential in public health education and healthcare demands strategic investment, interdisciplinary collaboration, and careful consideration of ethical and equity implications. Incorporating and adapting effective AI into the health education programs and training can empower medical students and healthcare workers in general, with the knowledge and skills needed to effectively utilize AI tools and technologies in addressing public health challenges, including the predictive modeling in epidemiology and training of healthcare workers in pandemic responses. In public health practice, AI presents an opportunity to use both established and new sources of large, highly complex data (“big data”) to develop and tailor population-level interventions. Planning for AI applications requires a Systems Thinking approach and infrastructure to support the public, research, and policy. Because public health often handles several interrelated or interdependent issues with practitioners interacting with many different sectors, AI applications will need to support collaborations especially between health and social services. Furthermore, regulation and oversight are necessary steps to support AI adoption (Kueper et al., 2023). Despite the excitement and perceived opportunities for AI to support public health, a key barrier is that many local public health units do not currently have the capacity or resources for dedicated AI-related work.
During the pandemic, AI-powered learning technologies helped educational institutions move to online programs, creating digital content that closely mimics human-created material. AI also played a significant role in the healthcare sector, particularly by enhancing telehealth services.
Many healthcare organizations now use AI-powered chatbots for tasks such as appointment scheduling, symptom assessment, and medication reminders. AI was particularly crucial in diagnosing COVID-19, aiding doctors in analyzing MRI scans and other imaging systems to detect the virus. As social distancing measures continue, the need for contactless patient interactions has grown, with AI helping create safe, virtual healthcare environments for patients (Forum, 2021; Kueper et al., 2023).
AI advancements helped keep the public safe through the flow of informed during the global pandemic. One exciting case study involves Translators Without Borders (TWB), an organization committed to distributing essential information globally in a multitude of languages (Forum, 2021). TWB partnered with technologists and language professionals from several academic and industry partners (Amazon, Appen, Carnegie Mellon University, Facebook, George Mason University, Google, Johns Hopkins University, Microsoft and Translated) to form the Translation Initiative for COVID-19 (TICO-19). Together, they have developed COVID-19 materials in numerous world’s languages to train professional translators and researchers on Machine Translation (MT) models, ensuring the virus-related information reaches people across the globe. The initiative has produced translation benchmarks comprising 30 documents with nearly 70,000 words related to COVID-19 terminology. These documents were translated from English into 38 languages, including those with limited technological resources. The resulting content is publicly available on TICO-19’s GitHub, ensuring its continued accessibility for machine learning (ML) researchers and professional translators (Forum, 2021).
Disease threats from infections are numerous, diverse in presentation and usually unexpected. Medical applications of AI have predominantly focused on individual patient care, facilitating diagnostic processes, prescribing precision medicine, and supporting clinical decision making. AI and related technologies can potentially revolutionize the power and possibilities of infectious disease epidemiology. Spanning public health and individual outcomes through new AI approaches has the potential to radically change responses to infections, allowing for targeted treatments with appropriate and reasonable interventions (Kraemer et al., 2025). Amidst increasing global debate on AI investment and regulation, in a very recent study, Kraemer et al. (2025) particularly emphasizing on safe and ethical usage of AI with transparency and accountability (combining ML, computational statistics, information retrieval and data science) in infectious disease research. Here are a few actionable recommendations for public health education and the healthcare system, based on knowledge gained from COVID-19 and AI development. The public health practitioners, AI researchers and providers should discuss and determine how AI can fit into public health education and healthcare, risks of AI (such as racial bias), and how to bring fair AI and public health goals together. They also need to engage, collaborate and organize symposiums, workshops and retreats to Kueper et al. (2023):
• Identify: (1) The public health challenges and support or relevance of AI by identifying the tasks and goals of public health practitioners and identifying the broad areas of public health practice where AI could add value, including the most pressing issues in public health. And (2) appropriate cases for AI technologies that were not recognized or considered by AI developers, such as upstream prevention and earlier detection of threatening events, and how to best match resources with communities most in need of support.
• Foster trust in public health by addressing misinformation as a key obstacle, drawing lessons from current examples and the experiences of others to identify both facilitators and barriers to using AI in public health. Strengthening new and existing collaborations between public health professionals and AI researchers or providers. Online misinformation, including disinformation efforts, poses a significant threat to the effectiveness and success of public health strategies in combating epidemics (Gradoń et al., 2021). AI could detect and respond to dis- and mis-information, thus allowing for genuine public health communication during epidemics and pandemics. Generative AI may provide real-time views and options on relevant information and how public opinion shifts amid an epidemic or a pandemic (Chen and Stadler, 2023; Gradoń et al., 2021). The use of AI to inform or support public health communication can come with several risks. Careful attention is needed to avoid or reduce bias, leading to ethical mistakes or misunderstandings and damaging public trust in public health information (Morley et al., 2020).
• Build partnerships between universities and AI companies to co-develop training modules and identify future directions for integrating AI into public health practice with the concern about the risks of AI, such as racial bias. More recently, Kraemer et al. (2025) outlined how AI developments may hasten breakthroughs in research and responses to infectious outbreaks. The study is a partnership between scientists from the University of Oxford and other academics, industry, and policy organizations across the globe. The authors suggest rigorous benchmarks to evaluate AI models, advocating strong collaborations between government, society, industry and academia for sustainable and practical development of models for improving human health (Kraemer et al., 2025).
• Define and determine equity and social determinants of health for consideration in any AI application. And identify a potential opportunity to use AI to demonstrate the value of public health interventions in general, e.g., by simulating counterfactual scenarios or documenting program outcomes.
• Collect data based on its use, deficiencies, its availability, quality, and accessibility, in a timely fashion. The public and community health is under-resourced in general, with concerns about how the infrastructure needed to create and maintain improved public health data would be funded. It was unclear whose responsibility it is to improve data and where resources would come from. A key factor contributing to the slower adoption of AI in infectious disease epidemiology is the difficulty in obtaining large, standardized, and representative datasets necessary for training and evaluating AI/ML models with multiple parameters. However, recent developments in AI techniques are showing promising results even with limited data, creating new opportunities for AI to enhance health outcomes in both high-income and low-income countries. These advancements are improving the integration and collection of diverse data sources, allowing AI to play a role in policy-making and decision-making processes aimed at enhancing population health (Kraemer et al., 2025).
• Offer professional development programs to train public health educators in AI and digital tools and build partnerships between universities and AI companies to co-develop training modules, forge and sustain quality- communication and collaboration with AI end users and beneficiaries, including the “public health education - health workforce training – healthcare” professionals, data engineers, and AI developers.
• Discuss and determine how: (1) The potential barriers to AI integration (e.g., lack of digital infrastructure, ethical concerns) and how they can be addressed. Education and training in public health ethics need to adapt to address challenges in the post- COVID-19 and AI era, by balancing patient privacy with the need for data sharing in AI-powered systems and raising concerns and questions around data linkage and better data infrastructure (including its privacy, ethical, legal, social, and technical considerations). (2) Data created for public health activities may not necessarily be suitable for research and application development. (3) To use data generated by the public (e.g., through social media), highlighting some of the privacy, ethical and potentially even legal challenges of data that are compatible with AI technologies. The question of who is responsible for newer data types (such as social media) as they interact with catchment areas, was identified as a source of complexity for early detection systems. (4) Sociotechnical barriers are due to the possibility of data linkage caused by data sharing. (5) Data trust (from gathering to analysis to knowledge translation) and a need for data can support targeted interventions that work within larger socio-technical systems.
• Ensure equitable access to AI tools in low-resource settings. Across countries, the data landscape for infectious disease modeling is fragmented, thus, the harmonization of the data surveillance for infectious disease remains a significant challenge (Xu et al., 2020). The integration of digital health opens new possibilities for the use of AI. However, it is crucial to understand how international, sensitive, and multimodal data can be effectively combined with innovative AI models within secure and distributed hardware systems, as well as how AI computing can be made more environmentally sustainable (Kraemer et al., 2025).
• Recognize and consider respect for individuals and diverse populations, as public health interventions must be tailored to meet the unique needs of various groups. The challenges related to data sharing and access go beyond health data, extending to other critical data used for predicting health outcomes, such as individual behavior data (e.g., human mobility data), which is often owned by companies and can hold commercial value (Barreras and Watts, 2024).
• Define: (1) The accountability gap and the AI risk assessment, as many initiatives already exist with unclear future directions and sustainability. And (2) all the challenges and facilitators including: data foresight/availability, privacy and security, trust issues with evaluation, political cycle influence, lack of capacity for data engineers and data scientists in public health leading to data gaps, quality improvement versus continuous learning, standardization across settings, education around what AI is and the role it would play in evaluation, need to understand what situations are (not) amenable to AI solutions/benefit, and mistrust in AI and/or in data.
• Use data to evaluate programs and advocate for resources for successful public health interventions and delivery, detect early the key events, and tailor responses to needs. Example applications may include monitoring outbreak detection, identifying substance-related harm before overdoses, identifying emergency department visit spikes (e.g., opioid overdoses or new compounds/medications), and monitoring child health and development screening.
Traditional teaching methods have long been the cornerstone of healthcare education, relying heavily on didactic instruction, in-person interactions, and standardized curricula (Wang and Li, 2024). However, these approaches face significant limitations regarding scalability and the swift dissemination of information, particularly during global health crises like the COVID-19 pandemic (Liu X. et al., 2020). Furthermore, these methods often fail to account for the diverse demographic and socio-economic backgrounds of students, resulting in several challenges for public health education today. These challenges hinder its effectiveness, contributing to gaps in health literacy, practical skills among public health students and professionals, and the reliance on conventional lecture-based teaching (Woodruff et al., 2008).
AI, referring to hard- or soft-ware that can carry out tasks typically requiring genuine intelligence, e.g., language interpretation, pattern recognition, and decision making, is more and more applied in various fields, such as economy, science, healthcare and healthcare education (Wang and Li, 2024). Public health practitioners, AI researchers and providers should explore how AI could be integrated into public health education to improve competencies, AI-driven simulations for training healthcare workers in pandemic responses, and develop interdisciplinary curricula that combine public health, AI and ethics. AI-driven predictive analytics and tailored learning platforms can forecast epidemiological trends, healthcare service needs, and emerging public health risks. For instance, during the COVID-19 pandemic, Google AI created a model that could predict the number of COVID-19 cases in a specific region up to two weeks ahead (Devaraj et al., 2021; Stolerman et al., 2023).
Developing AI systems, trained in a broad range of health care data, may anticipate unknown challenges and trends that may emerge. Curricula can be developed in advance to provide medical students with the essential knowledge and skills, necessitating a sophisticated combination of data science and teaching expertise. Additionally, learning content can be tailored to adapt dynamically, aligning with the current state of public health and anticipating future needs. To appropriately integrate AI into public health education, a recent study by Wang & Li proposed a blended curriculum that includes (1) theoretical courses (covering the fundamental science of data management, academic elements of public health and the application of AI to public health), and (2) training, through advanced simulation platforms of genuine public health data in a controlled but authentic environment, e.g., outbreak and vaccine distribution simulations (Wang and Li, 2024). The authors suggested incorporating peer reviews and self-assessments to evaluate students’ progress and their capacity to apply AI technologies in practical scenarios. This approach allows for a thorough understanding of students’ innovative skills and critical thinking abilities when addressing and solving real-world public health challenges.
With its substantial potential for future growth, AI utilizes advanced computational techniques to support and enhance the learning experience for medical and public health students. For medical education, AI enhances the quality of medical education and improves educational outcomes, by and through (Chen et al., 2017; Erduran, 2023; Kolachalama, 2022; Masters, 2019; Wang and Li, 2024):
• Simulation training, personalized learning, and adaptive systems: AI-driven simulation platforms provide students with a risk-free environment to enhance their clinical skills. For example, virtual reality surgical training enables students to practice and master complex medical procedures in a fully simulated setting.
• Supporting medical professionals: AI technologies assist medical students and healthcare professionals by facilitating tasks such as disease diagnosis, clinical reasoning, data analysis, and informed decision-making. AI is now being applied across various medical specialties, including rheumatology, neurology, endocrinology, orthopedics, and surgery.
• Machine learning (ML) in education: ML, a subset of AI that enables predictions and decisions through data training without explicit programming proves transformative in educational settings. AI/ML can personalize learning by analyzing large data sets, tailoring content to individual needs, enhancing comprehension and retention, and providing interactive training through simulations. This process allows students to access and apply the latest learning resources while improving learning outcomes.
• Personalizing content and learning experiences: AI-enabled platforms adjust to students’ learning pace, comprehension levels, and preferred teaching styles, thus creating personalized learning pathways. These platforms have been shown to improve student engagement and learning efficiency by analyzing individual performance and adapting educational content accordingly. AI’s ability to customize learning materials brings a new level of personalization, enhancing education through real-time feedback. However, the risk arises when students use AI prompts to generate assignments, challenging the essence of learning and authentic assessment of knowledge.
• Dynamic learning modules: AI can create dynamic learning modules that automatically adjust content based on student interactions and progress, offering tailored educational experiences in real-time (Wang and Li, 2024).
• (1) To help them overcome learning challenges, AI can offer students struggling with a medical topic additional resources, such as video tutorials, interactive simulations, and personalized practice questions.
• (2) The use of AI-powered simulation technology in education provides medical students with hands-on experience and instant feedback. This method proved especially beneficial during the COVID-19 pandemic, as it allowed students to practice and enhance their clinical skills in a secure virtual setting, minimizing physical contact and reducing health risks.
AI/ML can be widely integrated into public health education and various public health scenarios, from automating administrative tasks to aiding in the resolution of complex issues and decision-making, ultimately improving the quality and effectiveness of education. Below is a list of some ML algorithms and their applications in medical education to improve learning efficiency and educational quality and offer new insights and tools for medical research (e.g., optimizing disease diagnosis and treatment strategies) (Wang and Li, 2024).
Algorithm name | Application |
Few-Shot Learning | Diagnosis of rare diseases. |
Transfer Learning | Inter-specialties transference of information. |
Meta-Learning | Developing diagnostic reasoning that adjusts dynamically to clinical outcomes. |
Reinforcement Learning | Simulation of diagnostic reasoning processes. |
Self-supervised Learning | Analysis of diagnostic imaging data. |
Generative Adversarial Networks (GANs) | To generate diagnostic imaging data to train students and hone interpretation skills. |
To ensure that the future public health education and public health workforce are well-equipped to chart a path through the complex realities of modern healthcare, shaped and affected by AI, academic, medical and public health programs must integrate AI education and guidance into their curriculum with a combination and appliance with other cornerstone technologies such as (Smith, 2024; Wang and Li, 2024):
• Data analytics: is the process of examining raw data to draw conclusions and extract meaningful insights. It involves analyzing large datasets to uncover hidden patterns, correlations, and trends. This process has been automated into mechanical systems and algorithms that process raw data for human use. Different types of data analytics include descriptive analytics, diagnostic analytics, predictive analytics, and prescriptive analytics. A variety of software tools, such as spreadsheets, data visualization platforms, reporting tools, data mining applications, and open-source programming languages are used in data analytics. In the context of education, data analytics can (1) help educators understand student learning behaviors and outcomes, (2) support better decision-making regarding teaching methods and interventions, and (3) enhance adaptive learning platforms, intelligent tutoring systems that offer real-time feedback and assistance, and predictive analytics to inform curriculum development and adjustments (Daniel, 2015). The use of big data analytics and AI can aid in the early identification of epidemics and other public health threats, helping to implement focused and effective public health strategies (Wang and Li, 2024). Strengthening the medical students’ and healthcare professionals’ preparedness strategies to healthcare crisis is vital for ensuring effective emergency responses. Simulation creates immersive scenarios that reflect real-world crises, it rests as an essential part of preparedness in attending to public health issues by departments of health and other agencies (Rega and Fink, 2014).
• To enable medical students to adapt scenarios based on their decisions, creating a dynamic learning experience and engaging in problem-solving exercises that mirror real world situations, several AI-powered platforms utilizing ML algorithms have been introduced in public health education to simulate public health crises (Wang and Li, 2024). AI-driven predictive analytics and tailored learning platforms can forecast epidemiological trends, medical service needs, and emerging health threats. In a recent study, Li et al. (2020) explored the challenges and potential strategies for simulation and technology-enhanced learning during the COVID-19 crisis in China. The authors highlighted that simulations played a crucial role in providing practical training for frontline healthcare workers, ensuring both patient safety and a secure environment for medical professionals. They recommended the development of specialized simulation courses to better prepare medical staff for both current and future pandemics.
• Pre-training: A neural network that involves initially training a model on a vast amount of general data before focusing on a specific task or dataset is known as pre-training. This approach allows the model to build a broad knowledge foundation, which can then be fine-tuned to suit specific applications. Afterward, the parameters or models from the initial training are used to train another model for a different task or dataset. In the context of medical education, pre-trained models can be utilized to interpret complex medical texts or assist in diagnosing conditions, thereby improving the efficiency and accuracy of subsequent tasks (Chen et al., 2023).
• Prompt engineering: is a rapidly developing field focused on creating and optimizing prompts (like questions and instructions) to maximize the efficiency of Language Models (LMs) across various applications and research areas, including enhancing AI model outputs. By improving the capacity of Large Language Models (LLMs) to perform tasks like question answering and arithmetic reasoning, prompt engineering helps design robust techniques that effectively interact with LLMs and other tools. Effective, prompt engineering in medical education can enable educators to develop more impactful AI-driven teaching tools, such as simulated dialogue systems, which can guide students more effectively through targeted and precise prompts (Meskó, 2023).
• Augmented Reality and Virtual Reality: Augmented Reality (AR) is an interactive experience that blends the real world with computer-generated 3D content, creating a synthetic environment where digital elements are superimposed onto the physical world. Virtual Reality (VR), on the other hand, is an immersive experience that uses 3D near-eye displays and pose-tracking systems, combining computers and sensory devices (like headphones and gloves) to offer users a sense of presence in a virtual environment. When AI is integrated into VR and AR simulations, it can: (1) Improve the adaptability and interactivity of educational tools, providing students with a more personalized, responsive learning experience and enabling them to engage in realistic medical scenarios without physical constraints. (2) Create highly customized, dynamically adaptive learning environments, making education more inclusive and accessible (Barteit et al., 2021; Yeung et al., 2021).
• Natural Language Processing (NLP)/Generative AI: The use of AI in education is growing, offering a range of benefits, especially in large-scale teaching settings, by providing real time and personalized feedback to students (Gao et al., 2024). NLP, a branch of AI, has significantly progressed in text analysis. The development of powerful Large Language Models (LLMs), such as ChatGPT, indicates a bright future for these technologies in educational settings (Kasneci et al., 2023). AI-powered assessment and evaluation tools are increasingly used for automated grading, giving feedback, gauging student understanding and engagement, ensuring academic integrity, and evaluating teaching effectiveness (Huang et al., 2023). A recent study by Chan et al. (2024) explored the impact of feedback generated by LLM on the essay writing skills of university students in Hong Kong. This randomized controlled trial evaluated how generative AI might improve student revisions of essays among 918 first-year language students, examining the effects on student writing tasks and their experiences during the revision process (Chan et al., 2024).
• Game-Based Learning (GBL): By investigating the influence of GBL in higher education in Hong Kong, a mixed-methods exploration of the effect of GBL on the experiences of students Lo and Chan (2024) provide valuable insights into how GBL influences student motivation and learning outcomes. The primary aims of the study are to assess the educational impacts of immersive competitive GBL, examine student experiences with online GBL, and investigate factors such as student motivation, engagement, and learning outcomes. The study also seeks to interpret these experiences within the framework of social learning theory, which highlights the role of observational learning, where individuals acquire knowledge, skills, attitudes, and beliefs by observing the behaviors of others and the resulting consequences, leading to adopting these behaviors. Additionally, the authors compare GBL to traditional classroom learning and analyze students’ reactions to gamification and digital learning tools introduced during the COVID-19 pandemic. The study provides valuable insights and evidence on the effects and potential of GBL, offering guidance for academics, policymakers, and educators considering the integration of GBL into educational programs.
• Maker Culture (MC) and digital literacy: MC is a collaborative educational method that encourages self-paced, autonomous learning and applies this knowledge to problem-solving and innovation, which are essential for sustainability education. It is a promising tool for developing green digital skills and offers an alternative to traditional performance-based learning. A recent study explores the implementation and impact of MC and digital literacy, focusing on self-directed learning in higher education institutions in Hong Kong (Lo, 2024). The findings offer important insights into the advantages and challenges of incorporating MC into traditional education systems to cultivate green digital skills. This integration promotes a future of education that is innovative, creative, and student-driven, helping to develop sustainability competencies. Successful implementation of the MC approach relies on sufficient resources, skilled teachers, and improved administrative support.
Drawing from a broader empirical foundation, as previously mentioned, and given that both GBL and MC focus on structured instructional design rather than rigid policies, we suggest that well-crafted educational strategies and flexible, student-centered teaching methods are more effective than broad policy mandates.
Men who engage in sex with men (MSM) are particularly vulnerable to HIV and sexually transmitted diseases (STDs). However, there is a shortage of reliable and accessible tools for evaluating HIV risk, especially in countries with low endemic rates and globally. To allow clinicians to quantify predictions, present the risk of diseases’ infections, and offer intervention options to the MSM population, risk prediction models have been used in the prevention system of diseases and clinical scenarios. AI-based risk stratification models and web applications can assist clinicians in accurately evaluating the infection risk of individuals at high risk, MSM with concealed behaviors and help them monitor their own risk. AI and ML methods offer adaptability and scalability, making them well-suited for tasks like risk stratification, especially when handling large datasets (Ngiam and Khor, 2019). A study utilizing data from a longitudinal cohort study of high-risk individuals for syphilis infection in Peru aimed to predict future syphilis occurrences among MSM and transgender women. The researchers developed a predictive model and an online risk calculator for syphilis, which showed moderate accuracy and could serve as a foundation for future studies on syphilis prediction (Allan-Blitz et al., 2018).
Here we present a case study that highlights the effectiveness of ML methods in predicting the risk of HIV and STDs among MSM. In a study by Lin et al. (2025), ML models were developed to assess the risk of HIV and STDs in MSM by conducting a cross-sectional survey of 1,999 MSM with unknown or negative HIV serostatus in Western China from 2013 to 2023. The study found that: (1) the ML models performed well in predicting HIV and STD risk among the MSM population, (2) 72 (3.6%) of the 1,999 MSM tested positive for HIV, and 146 (7.3%) reported a history of STDs, (3) the highest combined predictive performance scores for the HIV and STD models were 33 and 39, respectively, and (4) high-risk groups exhibited significantly higher rates of HIV and STDs, with higher predicted probabilities. Sensitivity analyses also showed that the models were effective in predicting risks for MSM subgroups with varying infection risks. The authors found that the XGBoost algorithm (an enhanced version of gradient boosting) performed the best in predicting HIV and STD risk in this population. Previous studies have also reported the effectiveness of XGBoost in predicting HIV infections in Zimbabwe on timely clinic attendance and acceptance of HIV or STD testing (Birri Makota and Musenge, 2023; Xu et al., 2022). XGBoost is recommended as a key algorithm for future risk prediction models. The study also identified several risk factors for HIV infection and STDs, including low education, inconsistent condom use, limited HIV knowledge, multiple male partners, and internet dating. To protect privacy and promote self-management and monitoring, the authors suggest that MSM use web applications for self-assessment of their infection risks. These applications can help clinicians and MSM make more informed decisions about prevention and intervention by offering predictive results through web-based calculators for probability estimation and interactive computation (Xu et al., 2022).
The COVID-19 pandemic, caused by the SARS-CoV-2 virus, has had a profound impact on global health and economies, leading to substantial morbidity and mortality worldwide (Abdelouahed et al., 2023). The SARS-CoV-2 virus utilizes its “spike protein” to bind with the Angiotensin-Converting Enzyme 2 (ACE2) on the surface of host cells, allowing it to enter and infect them (Abdelouahed et al., 2023). Despite global efforts to limit transmission through measures such as testing symptomatic individuals and enforcing social distancing, the virus spread rapidly due to presymptomatic and asymptomatic transmission via airborne respiratory droplets and aerosols. This posed a significant challenge in curbing the pandemic (Huff and Singh, 2020; Pollock and Lancaster, 2020). Traditional contact tracing methods, while important, were slow and raised privacy concerns, especially as patients were hesitant to share personal activity details. In contrast, digital contact tracing methods, leveraging Bluetooth communication between smartphones, offered a more efficient, precise, and timely alternative. These digital solutions could notify individuals who might have been exposed through automated messages, along with instructions for follow-up testing and quarantine if a close contact tested positive. However, privacy issues regarding the use of smartphones for such tracing still posed barriers to widespread adoption.
Tang et al. (2021) suggested that effective healthcare contact tracing technologies should possess specific features and meet certain requirements to address these challenges.
• Promptness: through automation and a streamlined response process. It is needed to reduce the cases numbers that spread from one index case (medical staff or patient). |
• Simplicity: through an effortless management system (via well-developed user interfaces and unobstructed communication), it reduces human errors. It has economic implications and increases the likelihood of take-up among medical staff and patients. |
• High precision: is needed for effective automation in contact-tracing solutions enabling dynamic responses to information. It must accurately measure distance and time to determine interactions between individuals. |
• Integrable: robust contact-tracing systems should be integrated seamlessly into existing digital healthcare records. |
• Privacy: the systems should ensure medical staff and patient privacy. Limits and boundaries should be placed only for hospital and other healthcare facilities activities. |
• Fairness: current methods rely heavily on mobile phone technology. These have existing concerns over privacy and access. Many patients may not use smartphones for various social and economic reasons. |
To implement COVID-19 contact tracing systems in healthcare environments with the desired features, a Real-Time Location System (RTLS) utilizing Bluetooth Low Energy (BLE) and AI was developed and deployed (Tang et al., 2020). This system involved installing sensors throughout the hospital to detect signals from small BLE tags worn by patients, visitors, and healthcare workers. Using a Deep Learning (DL) algorithm, the system was able to accurately determine interpersonal distances and the duration of contacts by comparing signals from the sensors on two different tags. To further improve risk assessment and estimate the potential severity of exposure, the system could integrate with electronic health records and hospital surveillance systems, allowing it to gather additional data such as demographic details, medical history, and mask compliance. Privacy concerns were addressed by restricting the system’s use to specific hospital areas and designated individuals (patients, visitors, and healthcare workers). This technology offers a highly accurate, efficient, and equitable solution for contact tracing in healthcare settings, with minimal privacy issues. It is also accessible to all patients and visitors, regardless of smartphone ownership, and it is strongly recommended for healthcare workers to maximize its effectiveness in controlling the spread of COVID-19. Additionally, this system is adaptable for use with other infectious diseases.
At the University of Texas Southwestern Medical Center, a BLE sensor network consisting of 142 sensors (costing $50 each) was installed in the storage radiation oncology building, and a DL algorithm using Long Short-Term Memory (LSTM) models was used. This setup achieved perfect zone-localization accuracy. The total cost of implementing the system, including hardware, construction, and software development, was approximately $10,000 for a 63,000-square-foot facility (Tang et al., 2020).
In recent years, Knowledge, breakthrough discoveries and development in the fields of biomedicine and biotechnology (including medicine, biology, biotech, biochemistry, proteomics, immunology, microbiology and bioinformatics). These have increased intensively, with a paralleled dramatic increase in development, innovation and growth data science and information technology along with AI. All of these are essential to manage and leverage immense biomedicine, biotechnology and pharmaceutical data flow.
AI, the mimicking of actual intelligence in machines is applicable to any device or process that exhibits characteristics of human behaviors, e.g., the trait of recognizing patterns and solving problems. Cross-talks and cross-applications among biomedicine biotechnology sciences and informatics/Informatic field led to the creation of new sub-fields in biology, e.g., bioinformatics, and immunoinformatic. AI allows diverse and new applications for biomedicine-biotechnology research and biotech-pharma drugs and diagnostics’ development. Such applications include the use of AI for protein modeling, drug screening, vaccine and drug design, and the prediction of complex processes found in the human immune systems (e.g., the forecasting recognition and binding sites in immune cell and molecules).
As previously mentioned, ML refers to computer programs that can automatically learn from and adapt to new data, either under the guidance of a teacher (supervised learning) or independently (unsupervised learning). One type of ML utilizes Artificial Neural Networks (ANNs), which have distinct capabilities that enable them to solve problems that traditional rote learning models cannot address. DL, a subset of ML, uses multilayered ANNs trained on large datasets to enable automatic learning. Within each layer of the neural network, DL algorithms continuously perform calculations and predictions. Over time, as the volume of data increases, these algorithms learn and enhance the accuracy of outcomes without requiring further human input (Thomas et al., 2022).
The accurate three-dimensional structure of a protein (or its recombinant peptide derivative), governed by its primary sequence of amino acids sequence and post-translational molecular changes, defines the function of the targeted protein (or its recombinant peptide derivative) and is needed to define key neutralizing epitopes needed to design and produce effective new vaccines or drugs. To protect against infectious disease and design effective vaccines or monoclonal antibodies, it is essential to define and understand the overall all molecular special arrangement and structure of the chosen and targeted protein/peptide derivative. Defining the three-dimensional structure and resolution of a protein by experimental crystallography is a long and tedious process, it rests ineffective for many large or complex proteins. For biomedical and healthcare applications, AI-based approaches can learn to identify subtle patterns with masses of datapoints and generate models that predict paradigms to be studied biologically (Bishop, 2013). The advances in AI can allow innovative and novel vaccines, diagnostics, drugs, and personalized treatments to be produced more efficiently than with traditional methods.
In recent years, by leveraging genetic information, considerable progress has been made by scientists to analyze the co-variation of homologous sequences, to deduce which amino acid residues are in contact, and predict the structure of the chosen protein (or its recombinant peptide derivative). Senior et al. (2020) demonstrated that a neural network called AlphaFold could predict protein structure and shape with remarkable accuracy, even for sequences with limited homologous data. Using data from the Protein Data Bank, AlphaFold employed the input sequence and multiple-sequence alignments to predict distances and torsion angles between residues. The enhanced accuracy of protein structure predictions by AI models like AlphaFold marks a significant breakthrough in the field, providing scientists with a deeper understanding of both the function and dysfunction of targeted proteins, particularly when no homologous proteins have been experimentally characterized (Senior et al., 2020).
Drug discovery has entered a new phase characterized by the integration of “big data”, AI, innovative ML and DL techniques (Zhang et al., 2017). Through artificial neural networks (ANNs), such as DL networks and recurrent neural networks (RNNs), AI is significantly enhancing drug discovery efforts. These technologies help evaluate candidate molecules for various critical properties, including potency, selectivity, and ADMET (absorption, distribution, metabolism, excretion, and toxicity) (Hessler and Baringhaus, 2018). In silico methods and ML technologies, such as Support Vector Machines, Random Forests, and Bayesian Learning, are commonly utilized in drug design (Thomas et al., 2022). To explore new targets for existing drugs, large public datasets are leveraged to develop ML models that predict cross-target activities.
ML-based methods, like Kronecker-Regularized Least Squares (KronRLS), assess the similarity between drugs and protein molecules to predict drug-target binding affinities. AI and ML models are trained to analyze vast libraries of compounds to identify potential biological activities, quickly and cost-effectively validating and optimizing drug targets (Thomas et al., 2022). Additionally, Deep Neural Networks (DNNs) have demonstrated superior performance in predicting compound properties, outperforming other ML techniques in areas like biological activity, ADMET properties, and physicochemical parameters. DL models, such as DeepDTA, PADME, WideDTA, and DeepAffinity, have improved drug-target binding affinity predictions, using network-based approaches that do not rely on 3D protein structures (Thomas et al., 2022).
In recent years, AI and RNNs have been applied to de novo drug design, a computational technique that generates a variety of protein structures and modifies existing proteins to create novel functional proteins (Huang et al., 2016). Once a potential drug candidate is identified, it undergoes toxicity assessments through in vitro cell-based assays, followed by further evaluations in animal models. Regulatory bodies, such as government drug agencies, require extensive phase 3 studies to establish the safety and efficacy of AI-generated drug candidates.
Vaccines, which protect against pathogens by stimulating immune memory responses to critical pathogen proteins, have been transformative in global health by reducing the spread of infectious diseases. Traditional vaccine development is often a lengthy process. Additionally, the virus can mutate into an immune-escape variant, which may compromise vaccine effectiveness during pandemics or endemic outbreaks.
AI-based approaches may quicken vaccine development processes. Many AI algorithms and ML methods have been developed to:
• Enhance R&D in immunology and infection diseases, by assisting immune epitope detection, disease prognosis, and treatment outcome prediction, predicting genotypes associated with a poor prognosis, detecting the phenotypes of particular diseases, predicting the immunopeptidome (large collection of peptides associated to Human Leukocyte Antigens or HLA), and determining which peptides can be presented by Major Histocompatibility Complex (MHC)-II molecules, paving the way toward personalized vaccine development (Jabbari and Rezaei, 2019). ML can predict the tertiary structure of a protein based on its amino acid sequence and predict its binding to MHC molecules. (Moghram et al., 2018). Although the epitope prediction for MHC-II molecules is challenging, due to varying peptide length, AI-based algorithms have been effective in designing vaccines targeting the MHC-II immunopeptidome (Jabbari and Rezaei, 2019).
• Determine oligonucleotides with immunomodulatory properties and create in silico cell models that help design vaccines, to find new candidate antigens using reverse vaccinology (Thomas et al., 2022).
• Facilitate the rapid design and evaluation of new and emerging vaccines, identifying individuals unlikely to be protected by vaccine, and guide the development of improved vaccines to fight emerging infections and cancer and improve monitoring for poor responses in the elderly, infants, or patients with weakened immune systems.
• Develop personalized HIV vaccines. McGowan et al. (2021) used the diversity metric of the HLA profile of individuals within a population and sequence diversity of an individual’s CD8 T cell immune repertoire to assess the HIV proteome for regions of immunogenicity, allowing an expedited and rational CD8 T cell vaccine design for HIV that could potentially be applied to other pathogens.
• In 2019, a clinical trial [NCT03945825] was commenced in the USA of a seasonal influenza vaccine whose adjuvant was identified by AI. In the development of this vaccine, a combination of in silico AI programs were used to predict an oligonucleotide sequence that could act in the vaccine formulation as a potent agonist of human Toll-like receptor (TLR-9), which plays important roles in activating the innate immune system, resulting in induction of type I Interferon expression and other cytokines that enhance adaptive immune responses. Using conventional wet lab-based techniques would have taken decades to screen many different oligonucleotide sequences, whereas the in silico AI-based screening approach was able to identify the best TLR-9 agonist adjuvant candidate in just weeks (Thomas et al., 2022)
• AI/DL can be deployed also as a prognostic diagnostic tool for COVID-19. Wang et al. (2020) developed a DL-based system to analyze thoracic computed tomography (CT) images to detect and monitor COVID-19 patients. Poongodi et al. (2022) proposed a hybrid DL method to diagnose COVID-19 by measuring the symptom level of the patient with an Oura smart ring. AI/DL approaches have also been applied in early triage, medical imaging (X-ray and CT), data acquisition, and analysis techniques to control the spread of the SARS-CoV2 virus (Shi et al., 2021).
Effective disease preparedness and response depend on the prompt identification of emerging zoonotic pathogens or new variants of existing ones. Standard genomic surveillance, which monitors the genetic diversity of pathogens and helps determine their epidemiological and disease phenotypes, typically requires extensive time and resources for experimental work (Kraemer et al., 2025). However, AI-driven high-throughput predictions of protein structures and pathogen phenotypes from genomic data can facilitate quick initial assessments of new phenotypes, ultimately reducing the amount of experimental work needed to achieve accurate predictive results (Hie et al., 2021). AI methods and models can improve the precision of phylogenetic analysis, offering a more accurate characterization of the infection process. They can also help trace the evolutionary path of circulating pathogens, potentially predict escape variants, and explore previously unrecognized pathogen diversity through sequence-based AI approaches (Thadani et al., 2023).
In this article, we chose and gave the ongoing monkeypox virus (Mpox) outbreak as an example to illustrate how pandemic preparedness and public health education that incorporate AI-driven epidemiological modeling could address future outbreaks.
Since the first human case was identified in Central Africa in 1970, monkeypox (Mpox), caused by the monkeypox virus (MPXV), has been recognized as a viral zoonotic disease, with endemic areas mainly located in West and Central Africa (Breman et al., 1980). The monkeypox virus belongs to the Ortho poxvirus genus within the Poxviridae family and is closely related to the variola virus, which causes smallpox (Di Giulio and Eckburg, 2004). There are two main clades of the virus responsible for Mpox: clade I and clade II, with subclades within each—clade Ia, clade Ib, and clade IIb (CDC, 2024). Mpox is transmitted through direct skin-to-skin contact with infected individuals, their contaminated clothing or bedding, sexual contact, or consumption of infected meat (Kozlov, 2024). Additionally, Mpox is recognized as a sexually transmitted disease, primarily affecting MSM (Deng et al., 2024). The clade 1b viral strain responsible for the Mpox outbreaks in Central Africa is more deadly than the strain associated with the global 2022 Mpox outbreak, which was caused by the subclade IIb. Cameroon is the only country in West Africa that has reported cases of both clade I and clade II Mpox, with a few travel-related clade I cases recently reported in Germany, India, Sweden, and Thailand (CDC, 2024; Kozlov, 2024).
Since early May 2022, Mpox expanded into a global outbreak as countries outside Africa endemic regions reported new Mpox cases. Following the confirmed mpox case in the UK in May 2022, the outbreak spread to other European countries, and further afield (Deng et al., 2024). By June 2023, Japan had 181 confirmed whilst South Korea reported 102 cases and Taiwan confirmed more than over 50 cases (Deng et al., 2024). As of February 2, 2025, a total of 21,085 confirmed Mpox cases (involving clades I and II) and 70 deaths among those cases, have been reported by 21 countries in Africa since the beginning of 2024 (Department of Health, 2024). While confirmed cases have been predominantly concentrated within Democratic Republic of the Congo (DRC) (68.9% of all cases), activity has been increasing in Burundi since July 2024 (15.9% of cases) and in Uganda since mid-September 2024 (11.8% of cases). Cases of clade I Mpox have been reported outside of Africa in countries that included Thailand China, US, and UK (Department of Health, 2024). All imported cases have traveled to areas with community transmission of clade I mpox or the United Arab Emirates.
On August 14, 2024, WHO declared the ongoing Mpox outbreaks in DRC and other parts of Africa a global emergency, urging immediate measures to prevent further transmission of the virus. This announcement came 15 months after the conclusion of WHO’s previous Mpox emergency declaration. By July 2024, there had been 102,997 confirmed cases and 223 deaths reported across 121 countries. By August 2024, 15 countries had identified Mpox cases, with more than 17,000 cases and 590 fatalities (∼3.5% fatality rate), nearly all of which were in DRC, one of the world’s poorest nations, has seen its healthcare system severely strained by malnutrition, cholera, and measles outbreaks. Children are especially vulnerable, with approximately two-thirds of the infections in the DRC affecting individuals under 15 years old. By September 18, 2024, over 21,000 suspected cases of clade I Mpox had been reported in the DRC, marking the highest annual number ever recorded. This includes over 700 deaths in a population of more than 99 million people (Cheng, 2024; Jadhav et al., 2025; Kozlov, 2024).
In the US, Clade II Mpox remains at low levels, with no cases of Clade I Mpox reported. The CDC recommends the JYNNEOS vaccine, which requires two doses, for individuals at risk of Mpox in the US. This FDA-approved vaccine is anticipated to offer protection against both Clade I and Clade II, as well as all their subclades. In June 2024, health authorities in DRC authorized the emergency use of the JYNNEOS vaccine (manufactured by Bavarian Nordic) and the LC16 vaccine (produced by KM Biologics). JYNNEOS was also granted emergency use approval in Nigeria. Effective surveillance and collaboration between affected nations are crucial for managing the outbreak. However, despite the widespread use of treatments and vaccines during the 2022 global outbreak in high-income countries, these resources remain largely inaccessible to African nations (CDC, 2024; Kozlov, 2024).
COVID-19 | Mpox |
• COVID-19 spreads extremely rapidly dissimilar to Mpox. Soon after the identification of coronavirus, the number of cases climbed rapidly from a few hundred to a few thousand, in a one week in January 2020. When WHO described COVID-19 (March 2020) as a pandemic, there were already greater than 126,000 infections and 4,600 COVID fatalities, approximately 3 months after the initial identification of the virus. Unlike Mpox, there were no vaccines and no antivirals available for the COVID-19 pandemic in its early days. |
• Mpox spreads at a much slower rate compared to the coronavirus. The first confirmed case of Mpox in the US was reported on May 17, 2022, in Massachusetts (Centers for Disease Control and Prevention, 2023). The epidemic in the US reached its peak on August 1, 2022, before experiencing a significant decline. By January 2023, the US had reported over 30,000 new cases and 32 deaths (Owens et al., 2023). • Unlike the early days of the COVID-19 pandemic, vaccines and treatments for Mpox are now available. The 2022 Mpox outbreak, which spread to over 70 countries, was significantly reduced within a few months, primarily due to vaccination campaigns and the availability of treatments for at-risk populations in wealthier nations. • Despite promising results of Mpox treatments’ case-control studies, there is a need to investigate evidence on safety and effectiveness of Mpox treatments in humans through randomized controlled trials. Currently, there are no licensed therapeutics for treating Mpox, and no efficacy and safety therapeutic options have been studied in humans with Mpox, still some medications may be authorized for use in an outbreak, such as: Mpox (Fox et al., 2023). |
The trajectory of the 2022-23 Mpox pandemic began to flatten before the large-scale vaccination campaign was implemented. Using detailed Mpox parameter estimates derived from national surveys, case data, and vaccine distribution records, Lin et al. (2024) conducted a dynamic modeling study to assess the impact of the two-dose JYNNEOS vaccination campaign and the changes in behavior resulting from increased awareness among high-risk groups during the 2022–2023 Mpox outbreak in the US. Their model revealed that the two-dose vaccination campaign alone prevented 21.2% of cases, while the increased awareness in high-risk groups led to a 15.4% reduction in cases. The combination of both the vaccination campaign and behavior changes due to heightened awareness resulted in a 64.0% reduction in Mpox cases, suggesting that the US Mpox epidemic was effectively managed through these two strategies, along with sexual risk reduction efforts (Lin et al., 2024). The combined effects observed are important for countries dealing with Mpox outbreaks, as they can help guide preparedness strategies for potential future Mpox spread. These findings may also be applicable to other emerging or re-emerging infectious diseases and to those that are preventable through vaccines or influenced by behavioral changes. A modeling study of the 2022 Mpox outbreak in the UK indicated that the decrease in Mpox cases was primarily due to increased population immunity following infections and reduced exposure from behavioral changes, rather than the impact of a single-dose vaccination (Brand et al., 2023).
Here are tangible AI’s opportunities and applications for outbreaks/pandemic preparedness (e.g., the ongoing Mpox) to (Kraemer et al., 2025):
• Enhance existing disease transmission models and identify areas with high potential for transmission. |
• Forecast the emergence of new variants and strengthen genetic surveillance to support vaccine development, while helping to determine the characteristics of new pathogens. |
• Improve outbreak detection and monitoring by utilizing AI to integrate population-level data with individual-level information from sources like wearable technologies, including heart rate and step counts. |
• Develop an interface that bridges advanced scientific research with healthcare professionals who have limited technical training, thereby increasing capacity in areas that require these tools the most. |
In education, particularly in public health education, AI poses several well-known and significant risks, such as biases, privacy and security concerns, discrimination, lack of transparency, insufficient oversight, job displacement, depersonalization, and the inappropriate use of context-specific algorithms (Wang and Li, 2024). Educators must specifically address these potential biases and their impact on decision-making, using all available technical and methodological tools to identify and mitigate them. To better understand how to detect and correct AI biases in real-world and healthcare settings, medical students can benefit from simulation games that demonstrate how AI functions (including its limitations and biases), while also helping them develop and refine their critical thinking and decision-making skills to effectively address challenges associated with AI usage.
Implementing AI systems in medical education and public health involves managing sensitive patient health and personal data, which requires strict privacy measures and the use of robust encryption systems within the AI educational framework to prevent data breaches. To guide immediate actions, plan long term strategies, and build human capacities for ensuring that Generative AI remains human-centered, UNESCO has issued “Guidance for the Use of Generative AI in Education and Research”. This guidance highlights concerns that AI models may perpetuate or amplify existing health data biases due to the use of datasets with historical biases or unrepresentative samples during algorithmic training, which could result in biased educational content and health recommendations (Holmes and Miao, 2023; Wesson et al., 2022). A recent study by Obermeyer et al. (2019) uncovered significant racial bias against black patients in a commonly used AI algorithm.
Cultural backgrounds can have a significant impact on the effectiveness of AI-driven educational programs and learning methods across various regions. To foster the globalization and personalization of public health education, while ensuring fairness and inclusivity on a global scale, AI can better serve diverse populations by incorporating cross-cultural sensitivity into algorithm development and data collection. This can be achieved by creating health education materials that are culturally adaptable and ensuring that health data captures a broad range of demographic characteristics and health needs (Wang and Li, 2024). For responsible and effective AI implementation in public health education, in alignment with societal norms and legal standards, the following are necessary: (1) Establishing robust policy frameworks and guiding principles to address ethical concerns related to AI, and (2) Encouraging collaboration among educational institutions, policymakers, and AI developers to participate in ongoing discussions and promote sustainable, well-funded programs for fair AI development. Additionally, and as cited below, there are several other significant limitations regarding the use and effectiveness of AI (Kraemer et al., 2025).
• Modern AI/ML algorithms leverage the frequency of mutations in pathogen genomes to forecast the timing of infections, identify nucleotide signatures that link epidemiologically related individuals, and determine the directionality of transmission events (Skums et al., 2018). During the COVID-19 pandemic, pathogen genome datasets expanded significantly (from a few thousand genomes in past outbreaks to tens of millions), leading to the development of tools for swiftly analyzing large genomic datasets, which will be valuable for managing future pandemics. However, not all aspects of pandemic preparedness and response will be equally influenced by AI advancements. For instance, while protein language models show great potential for accelerating our understanding of how viral mutations affect disease spread and severity, advances in foundational models may only yield modest improvements over current methods for modeling the speed of pathogen transmission and infection (Turakhia et al., 2021; Turakhia et al., 2022). |
• Scientists caution against assuming that AI alone will resolve the challenges posed by infectious diseases. Incorporating human feedback into AI modeling processes could help address current limitations. There are concerns regarding the quality and representativeness of training data, the limited availability of AI models to the broader community, and the potential risks associated with using black-box models for decision-making. |
• Infectious disease outbreaks continue to pose a significant threat, but AI provides policymakers with a powerful toolset to make informed decisions on when and how to act. Although AI has immense potential to transform pandemic response, its effectiveness relies on widespread global collaboration and continuous, comprehensive surveillance data. |
Higher education has faced numerous challenges in the past but has consistently emerged stronger due to its unwavering dedication to maintaining high-quality learning, supporting students, and advancing scholarship, research, and innovation. Worldwide, universities have gone through, endured and are still conning with the COVID-19 pandemic situation and crisis. Our paper highlights some of the major challenges faced by institutions, faculty, students and healthcare professionals who adapted and created a semblance of education and healthcare work normally in the COVID-19 turbulent times. The changes to remote education and healthcare work generated challenges for educators, students and healthcare professionals (Korkmaz and Toraman, 2020). Having been prepared for potential disruptions due to technological issues during the COVID-19 pandemic, institutions that adopted distributed learning models, along with their faculty and students, gained experience in synchronous learning with peers and instructors from offshore campuses. Universities, including those offering healthcare education and training, should conduct thorough benchmark testing to assess how remote or hybrid learning environments have impacted the learning of students and healthcare professionals, and develop strategies for addressing any challenges. Many instructors had to navigate unfamiliar technologies while adapting their teaching methods to remote formats to continue meeting students’ academic needs. The challenge was to collaborate with faculty members to integrate technology effectively, enhancing the learning experience through course design and a thoughtful approach to selecting appropriate, relevant, and impactful technological platforms.
To avoid having underprepared college students and healthcare professionals succeed for their following future college courses, training and careers, they might need specific support for their following education and training through reviewing skills and content. Educational technologies, including augmented reality, AI, the “Internet of Things”, Cloud Computing, Mobile Learning, etc. are emerging. To create engaging learning environments, more research and efforts are needed to support, reinforce, and integrate technology, including the use of AI-driven technologies for education in general, higher education and healthcare education in particular. Amid the COVID-19 crisis, open educational applications, AI- driven technologies and many more platforms such as: Google Meet, Web Ex, MS-Teams, Zoom, and Education 4.0 were used to bridge the gap between teachers and learners. Added to its delivery and conduct of videoconferencing, sharing PowerPoint slides, videos, and documents can be effective, online education and AI-driven technologies are likely to be more impactful if they foster active learning. This can be achieved by providing opportunities for students to read, write, discuss, think critically, ask questions, solve problems, analyze, and create, based on the learning content (Zayapragassarazan, 2020). Online teaching and AI-driven resources should be streamlined and engaging to address the challenges of non-attendance, motivating both students and healthcare professionals. Since workshops and laboratory work are difficult to conduct during epidemics or pandemics, integrating virtual and remote laboratories, along with augmented reality, into distance education management systems should be explored and adapted. Converting students’ and healthcare professionals’ outcomes into measurable performance indicators offers valuable feedback on their progress. The data collected from these indicators should aim to enhance students’ achievement of specific skills and knowledge.
The COVID-19 pandemic provided an opportunity to establish the foundation for digital learning and AI-driven technologies, fostering unity, truth, and the holistic development of all students. It also encouraged the critical evaluation of science, knowledge, and wisdom within a framework of learning, love, empathy, and compassion. There remains a pressing need for innovation and the adoption of alternative educational and healthcare systems, as well as new evaluation methods. To offer more inclusive and accessible knowledge and wisdom to all students, healthcare professionals, universities and healthcare institutions, according to each specific institutional missions; need to engage in complete rethinking about the 21st century - post-COVID-19 education era by benefiting from what was learned from the COVID-19 pandemic, extensive research and literature on effective online teaching, and the use of AI-driven technologies. Online learning and AI-driven tools became widely adopted and established as a new standard for delivering education, integrating technology into existing educational systems to enhance syllabus completion, offer improved content to learners, diversify knowledge, and optimize time management while addressing various challenges (Ananga, 2020). After the pandemic, universities and colleges were expected to keep using distance education, all university boards must be prepared to adapt to changes in context of changing education environment, and should be ready to shift not only their strategic goals but to their understanding of their own role.
Pandemics, including the most recent ones of swine flu and COVID-19, are typically sparked by airborne viruses that spread quickly, including by people who may not be showing symptoms. More recently Deng et al. (2024) demonstrated that the reduced international air-travel volume and stringent border entry policy during the COVID-19 pandemic reduced Mpox importations prominently in China. The Mpox importation risk could be much higher now that air travel has returned to normal levels. The new post-COVID-19 world educative focus, possibilities and opportunity, including the ongoing Mpox outbreaks, are for universities and healthcare institutions to become places where acknowledgment of “diversity, equality and inclusion” are the prerequisites for education in the modern era.
During global health crises, such as the outbreak of a new infectious disease, nations encounter significant and complex decision-making challenges across their healthcare, economic, and education systems, as well as in ensuring the social well-being of their citizens. AI-driven solutions that aim to create generalized systems could offer valuable decision support in such situations (Bengio et al., 2024). Integrating AI with physics-based models can enhance: (1) the gathering and integration of historical, current, and future climate data into epidemiological models, (2) our comprehension of critical epidemic dynamics and the accuracy of predicted short- and long-term epidemic trends, and (3) the assessment of how climate influences infectious diseases (Kraemer et al., 2025). AI has the potential to enhance efforts in preventing and controlling infectious diseases, potentially saving lives and alleviating the impact of these diseases. However, the effectiveness of AI tools will largely depend on the thorough identification, analysis, and resolution of critical ethical issues, such as transparency, accountability, fairness, privacy, and preventing the exacerbation of existing inequalities (Guidance, 2021). AI-driven epidemiological modeling and AI-driven healthcare education systems should be incorporated to address future outbreaks, such as using AI tools to support Mpox, or any outbreak, for surveillance, diagnosis, prevention, and rapid vaccine development, on site and/or online higher education per se, particularly in healthcare and public health.
As the world continues to adapt to the changes brought about by the COVID-19 pandemic, we are increasingly transitioning to a virtual reality. AI played a significant role in facilitating safe social distancing, detecting COVID-19, and simplifying various aspects of our lives. However, further investment in AI is essential for a future where AI is deeply integrated into our daily routines. To ensure that AI is accessible to everyone, including underserved communities, it will be necessary to foster expertise through collaboration and improve access to computational resources. The growing scale and cost of training foundational AI models remain a significant barrier for most (Achiam et al., 2023). Globally, for AI to be successful in the future, close and effective collaborations between research, policy and society should be seen, as well as growth and sustainability (Burki, 2024). Our article aims to make a stronger contribution to the ongoing discourse on the post- COVID-19 pandemic educational reforms in public health, and to participate in the ongoing and expanding dialogue on how AI can be utilized to empower healthcare and public health education in responsible, effective, ethical, inclusive and equitable manners and approaches, thereby improving the quality, accessibility, and impact of health education initiatives worldwide.
MA: Conceptualization, Writing – original draft, Writing – review and editing. DY: Writing – review and editing. CA: Writing – review and editing. IA: Writing – review and editing. EA: Writing – review and editing. SF: Writing – review and editing.
The author(s) declare that no financial support was received for the research and/or publication of this article.
The authors declare that the research was conducted in the absence of any commercial or financial relationships that could be construed as a potential conflict of interest.
The authors declare that no Generative AI was used in the creation of this manuscript.
All claims expressed in this article are solely those of the authors and do not necessarily represent those of their affiliated organizations, or those of the publisher, the editors and the reviewers. Any product that may be evaluated in this article, or claim that may be made by its manufacturer, is not guaranteed or endorsed by the publisher.
Abdelouahed, M., Yateem, D., and Fredericks, S. (2023). Fc γ RIIa - dependent platelet activation identified in COVID-19 vaccine-induced immune thrombotic thrombocytopenia-, heparin-induced thrombocytopenia, streptokinase- and anisoylated plasminogen-streptokinase activator complex-induced platelet activation. Front. Cardiovasc. Med. 10:1282637. doi: 10.3389/fcvm.2023.1282637
Achiam, J., Adler, S., and Agarwal, S. (2023). Gpt-4 technical report. arXiv [Preprint] doi: 10.48550/arXiv.2303.08774
Alba-Linero, C., Moral-Sanchez, S., and Gutierrez-Castillo, P. (2020). Impact of COVID-19 on Education in a Spanish University: What Should we Change. Educational Practices During the COVID-19 Viral Outbreak: International Perspectives. Washington, DC: U.S. Department of Education, 81–106.
Allan-Blitz, L., Konda, K., Vargas, S., Wang, X., Segura, E., Fazio, B., et al. (2018). The development of an online risk calculator for the prediction of future syphilis among a high-risk cohort of men who have sex with men and transgender women in Lima. Peru. Sex Health 15, 261–268. doi: 10.1071/SH17118
Ananga, P. (2020). Pedagogical considerations of E-Learning in education for development in the face of COVID-19. Int. J. Technol. Educ. Sci. 4, 310–321. doi: 10.46328/ijtes.v4i4.123
Banerjee, D., Vaishnav, M., Rao, T., Raju, M., Dalal, P., Javed, A., et al. (2020). Impact of the COVID-19 pandemic on psychosocial health and well-being in South-Asian (World Psychiatric Association zone 16) countries: A systematic and advocacy review from the Indian Psychiatric Society. Indian J. Psychiatry 62, S343–S353. doi: 10.4103/psychiatry.IndianJPsychiatry_1002_20
Barnett, R. (2000). University knowledge in an age of supercomplexity. High. Educ. 40, 409–422. doi: 10.1023/A:1004159513741
Barreras, F., and Watts, D. (2024). The exciting potential and daunting challenge of using GPS human-mobility data for epidemic modeling. Nat. Comput. Sci. 4, 398–411. doi: 10.1038/s43588-024-00637-0
Barteit, S., Lanfermann, L., Bärnighausen, T., Neuhann, F., and Beiersmann, C. (2021). Augmented, mixed, and virtual reality-based head-mounted devices for medical education: Systematic review. JMIR Serious Games 9:e29080. doi: 10.2196/29080
Bengio, Y., Hinton, G., Yao, A., Song, D., Abbeel, P., Darrell, T., et al. (2024). Managing extreme AI risks amid rapid progress. Science 384, 842–845. doi: 10.1126/science.adn0117
Birri Makota, R., and Musenge, E. (2023). Predicting HIV infection in the decade (2005-2015) pre-COVID-19 in Zimbabwe: A supervised classification-based machine learning approach. PLoS Digit. Health 2:e0000260. doi: 10.1371/journal.pdig.0000260
Bishop, C. (2013). Model-based machine learning. Philos. Trans. A Math. Phys. Eng. Sci. 371:20120222. doi: 10.1098/rsta.2012.0222
Brand, S., Cavallaro, M., Cumming, F., Turner, C., Florence, I., Blomquist, P., et al. (2023). The role of vaccination and public awareness in forecasts of Mpox incidence in the United Kingdom. Nat. Commun. 14:4100. doi: 10.1038/s41467-023-38816-8
Breman, J., Steniowski, M. V., Zanotto, E., Gromyko, A. I., and Arita, I. (1980). Human monkeypox, 1970-79. Bull. World Health Organ. 58, 165–182.
Burke, L. (2020). Fall Comes Into View. Available online at: https://www.educationaladvisors.com/fall-comes-into-view/ (accessed March 1, 2025).
Burki, T. (2024). “Crossing the frontier: The first global AI safety summit,” in Lancet Digital Health, 6, e91–e92 (accessed March 1, 2025).
Calhoun, K., Yale, L., Whipple, M., Allen, S., Wood, D., and Tatum, R. (2020). The impact of COVID-19 on medical student surgical education: Implementing extreme pandemic response measures in a widely distributed surgical clerkship experience. Am J Surg. 220, 44–47. doi: 10.1016/j.amjsurg.2020.04.024
Centers for Disease Control and Prevention. (2023). US Mpox Case Trends Reported to CDC. Washington, DC: US Department of Health and Human Services.
Chan, S., Lo, N., and Wong, A. (2024). Enhancing university level English proficiency with generative AI: Empirical insights into automated feedback and learning outcomes. Contemp. Educ. Technol. 16:e541. doi: 10.30935/cedtech/15607
Chen, C., and Stadler, T. (2023). Genspectrum chat: Data exploration in public health using large language models. arXiv [Preprint] doi: 10.48550/arXiv.2305.13821
Chen, J., Han, K., Zhang, D., Li, Z., Li, Y., and Hou, L. (2017). Presurgical Planning for Supratentorial Lesions with Free Slicer Software and Sina App. World Neurosurg. 106, 193–197. doi: 10.1016/j.wneu.2017.06.146
Chen, W., Li, C., Chen, D., and Luo, X. (2023). A knowledge-based learning framework for self-supervised pre-training towards enhanced recognition of biomedical microscopy images. Neural Netw. 167, 810–826. doi: 10.1016/j.neunet.2023.09.001
Cheng, M. (2024). WHO Declares Mpox Outbreaks in Africa a Global Health Emergency as a New Form of the Virus Spreads. Geneva: WHO.
Chun, H., and Hargis, J. (2020). Linear algebra online learning objects: Rapid development while still focusing on pedagogy. Online J. New Horizons Educ. 10:206.
Contreras, C., Metzger, G., Beane, J., Dedhia, P., Ejaz, A., and Pawlik, T. (2020). Telemedicine: Patient-provider clinical engagement during the COVID-19 pandemic and beyond. J. Gastrointest. Surg. 24, 1692–1697. doi: 10.1007/s11605-020-04623-5
Cossovich, R., and Ermacora, G. (2021). “Interactive technology workshop as an activity for social-emotional competence in a post-pandemic scenario,” 13th International Conference on Education and New Learning Technologies 5–6 July, 2021, IATED, 7290–7298.
Daniel, B. (2015). Big Data and analytics in higher education: Opportunities and challenges. Br. J. Educ. Technol. 46, 904–920. doi: 10.1111/bjet.12230
De Jong, T., Linn, M., and Zacharia, Z. (2013). Physical and virtual laboratories in science and engineering education. Science (New York, NY) 340, 305–308. doi: 10.1126/science.1230579
Delgado, P. (2020). Synchronous and Asynchronous Learning: Definition, Advantages, and Disadvantages. Available online at: https://observatory.tec.mx/edu-news/synchronous-and-asynchronous-learning-definitions/ (accessed September 24, 2024).
Deng, X., Tian, Y., Zou, J., Yang, J., Sun, K., and Yu, H. (2024). The risk of mpox importation and subsequent outbreak potential in Chinese mainland: A retrospective statistical modelling study. Infect. Dis. Poverty 13:21. doi: 10.1186/s40249-024-01189-1
Devaraj, J., Madurai Elavarasan, R., Pugazhendhi, R., Shafiullah, G., Ganesan, S., Jeysree, A., et al. (2021). Forecasting of COVID-19 cases using deep learning models: Is it reliable and practically significant? Results Phys. 21:103817. doi: 10.1016/j.rinp.2021.103817
Di Giulio, D., and Eckburg, P. (2004). Human monkeypox: An emerging zoonosis. Lancet Infect. Dis. 4, 15–25. doi: 10.1016/s1473-3099(03)00856-9
Dorsey, E., and Topol, E. (2016). State of telehealth. N. Engl. J. Med. 375, 154–161. doi: 10.1056/NEJMra1601705
Ehrlich, H., McKenney, M., and Elkbuli, A. (2020). We asked the experts: Virtual learning in surgical education during the COVID-19 pandemic-shaping the future of surgical education and training. World J. Surg. 44, 2053–2055. doi: 10.1007/s00268-020-05574-3
Elers, S. (2020). Education Institutes Around the world Scramble to Prepare for Online Teaching. Available online at: https://www.stuff.co.nz/national/health/coronavirus/120400677/education-institutes-around-the-world-scramble-to-prepare-for-online-teaching (accessed March 1, 2025).
ElSaheli-Elhage, R. (2020). Access to students and parents and levels of preparedness of educators during the COVID-19 emergency transition to e-learning. Int. J. Stud. Educ. 3, 61–69. doi: 10.46328/ijonse.35
Erduran, S. (2023). AI is transforming how science is done. Science education must reflect this change. Science 382:eadm9788. doi: 10.1126/science.adm9788
Farooqi, M., Ullah, I., Irfan, M., Taseer, A., Almas, T., Hasan, M., et al. (2021). The revival of telemedicine in the age of COVID-19: Benefits and impediments for Pakistan. Ann. Med. Surg (Lond). 69:102740. doi: 10.1016/j.amsu.2021.102740
Forum, W. E. (2021). COVID-19 Increased the Use of AI. Here’s Why it’s Here to Stay. Cologny: The World Economic Forum.
Fox, T., Gould, S., Princy, N., Rowland, T., Lutje, V., and Kuehn, R. (2023). Therapeutics for treating mpox in humans. Cochrane Database Syst. Rev. 3:CD015769. doi: 10.1002/14651858.CD015769
Fund, I. (2021). International Monetary Fund. Available online at: https://www.imf.org/en/Publications/SPROLLS/world-economic-outlook-databases#sort=%40imfdate%20descending (accessed March 1, 2025).
Gao, R., Merzdorf, H., Anwar, S., Hipwell, M., and Srinivasa, A. (2024). Automatic assessment of text-based responses in post-secondary education: A systematic review. Comput. Educ. Artificial Intell. 6:100206. doi: 10.1016/j.caeai.2024.100206
Garrison, D., and Kanuka, H. (2004). Blended learning: Uncovering its transformative potential in higher education. Int. High. Educ. 7, 95–105. doi: 10.1016/j.iheduc.2004.02.001
Giarla, A. (2024). The Benefits Of Blended Learning. Available online at: https://www.teachthought.com/technology/benefits-of-blended-learning/ (accessed September 25, 2024).
Giridharan, B. (2020). Engaging with students and faculties online in the era of the corona virus pandemic: A higher education perspective. Horizon J. Hum. Soc. Sci. 2, 103–110. doi: 10.37534/bp.jhssr.2020.v2.nS.id1037.p103
Gokuladas, V., and Sam, S. (2020). Challenges and Responsibilities During School Reopening post-COVID-19: A Strategic Approach. Educational Practices During the COVID-19 Viral Outbreak: International Perspectives. Washington, DC: U.S. Department of Education, 199–220.
Gomez, E., Azadi, J., and Magid, D. (2020). Innovation born in isolation: Rapid transformation of an in-person medical student radiology elective to a remote learning experience during the COVID-19 pandemic. Acad. Radiol. 27, 1285–1290. doi: 10.1016/j.acra.2020.06.001
Gradoń, K., Hołyst, J., Moy, W., Sienkiewicz, J., and Suchecki, K. (2021). Countering misinformation: A multidisciplinary approach. Big Data Soc. 8:20539517211013848. doi: 10.1177/2053951721101384
Greenberg, N., Docherty, M., Gnanapragasam, S., and Wessely, S. (2020). Managing mental health challenges faced by healthcare workers during covid-19 pandemic. BMJ 368:m1211. doi: 10.1136/bmj.m1211
Guidance, W. (2021). Ethics and Governance of Artificial Intelligence for Health. Geneva: World Health Organization.
Hargis, J. (2020). What is effective online teaching and learning in higher education. Acad. Lett. 13, 1–6. doi: 10.20935/AL13
Hargis, J., Yuan, Y., and Wu, T. (2020). Rapid course migration: Comparing the changes of instructor selfefficacy. Glokalde Global Local Distance Educ. J. 6, 40–57. doi: 10.20935/AL13
Hebebci, M. (2021). The bibliometric analysis of studies on distance education. Int. J. Technol. Educ. 4, 796–817. doi: 10.46328/ijte.199
Hessler, G., and Baringhaus, K. (2018). Artificial intelligence in drug design. Molecules 23:2520. doi: 10.3390/molecules23102520
Hie, B., Zhong, Berger, B., and Bryson, B. (2021). Learning the language of viral evolution and escape. Science 371, 284–288. doi: 10.1126/science.abd7331
Holmes, W., and Miao, F. (2023). Guidance for Generative AI in Education and Research. Paris: UNESCO Publishing.
Hooker, C. (2020). “New learning models for fall 2020,” in Tech Learning, 40, 4–12. Available online at: https://www.techlearning.com/how-to/new-learning-models-for-fall-2020 (accessed March 1, 2025).
Hu, Y., and Shuangyi, L. Impact of COVID-19 on Overseas Chinese Students. Available online at: https://news.cgtn.com/news/2020-02-25/Impact-of-COVID-19-on-overseas-Chinese-students-OlLLLXMC0o/index.html (accessed March 1, 2025).
Huang, A., Lu, O., and Yang, S. (2023). Effects of artificial Intelligence–enabled personalized recommendations on learners’ learning engagement, motivation, and outcomes in a flipped classroom. Comput. Educ. 194:104684. doi: 10.1016/j.compedu.2022.104684
Huang, P., Boyken, S., and Baker, D. (2016). The coming of age of de novo protein design. Nature 537, 320–327. doi: 10.1038/nature19946
Huff, H., and Singh, A. (2020). Asymptomatic transmission during the coronavirus disease 2019 pandemic and implications for public health strategies. Clin. Infect Dis. 71, 2752–2756. doi: 10.1093/cid/ciaa654
Hunt, T., and Hooten, W. (2020). The effects of COVID-19 on telemedicine could outlive the virus. Mayo Clin. Proc. Innov. Qual. Outcomes 4, 583–585. doi: 10.1016/j.mayocpiqo.2020.07.001
İnce, E., Kabul, A., and Diler, I. (2020). The Opinions of Academicians on Distance Education During the COVID-19 Pandemic. Educational Practices During the COVID-19 viral Outbreak: International Perspectives. Washington, DC: U.S. Department of Education, 107.
Jabbari, P., and Rezaei, N. (2019). Artificial intelligence and immunotherapy. Expert. Rev. Clin. Immunol. 15, 689–691. doi: 10.1080/1744666X.2019.1623670
Jadhav, V., Paul, A., Trivedi, V., Bhatnagar, R., Bhalsinge, R., and Jadhav, S. (2025). Global epidemiology, viral evolution, and public health responses: A systematic review on Mpox (1958-2024). J. Glob. Health 15:04061. doi: 10.7189/jogh.15.04061
Kasneci, E., Seßler, K., Küchemann, S., Bannert, M., Dememtieva, D., Fischer, F., et al. (2023). ChatGPT for good? On opportunities and challenges of large language models for education. Learn. Individ. Diff. 103:102274. doi: 10.1016/j.lindif.2023.102274
Kimmel, H., Carpinelli, J., Spak, G., and Rockland, R. H. (2020). A Methodology for Retaining Student Learning During the Pandemic. Educational Practices During the COVID-19 viral Outbreak: International Perspectives (San Antonio, TX: ISTES).
Kola, L., Kohrt, B., Hanlon, C., Naslund, J., Sikander, S., Balaji, M., et al. (2021). COVID-19 mental health impact and responses in low-income and middle-income countries: Reimagining global mental health. Lancet Psychiatry 8, 535–550. doi: 10.1016/S2215-0366(21)00025-0
Kolachalama, V. (2022). Machine learning and pre-medical education. Artif. Intell. Med. 129:102313. doi: 10.1016/j.artmed.2022.102313
Korkmaz, G., and Toraman, Ç. (2020). Are we ready for the post-COVID-19 educational practice? An investigation into what educators think as to online learning. Int. J. Technol. Educ. Sci. 4, 293–309. doi: 10.46328/ijtes.v4i4.110
Kozlov, M. (2024). Growing mpox outbreak prompts WHO to declare global health emergency. Nature 632, 718–719. doi: 10.1038/d41586-024-02607-y
Kozlowski, S., and Ilgen, D. (2006). Enhancing the effectiveness of work groups and teams. Psychol. Sci. Public Interest 7, 77–124. doi: 10.1111/j.1529-1006.2006.00030.x
Kraemer, M., Tsui, J., Chang, S., Lytras, S., Khurana, M., Vanderslott, S., et al. (2025). Artificial intelligence for modelling infectious disease epidemics. Nature 638, 623–635. doi: 10.1038/s41586-024-08564-w
Kueper, J., Rosella, L., Booth, R., Davis, D., Nayani, S., Smith, N., et al. (2023). Inaugural Artificial Intelligence for Public Health Practice (AI4PHP) Retreat: Ontario, Canada. London: Western University.
Laurant, M., van der Biezen, M., Wijers, N., Watananirun, K., Kontopantelis, E., and van Vught, A. (2018). Nurses as substitutes for doctors in primary care. Cochrane Database Syst. Rev. 7:CD001271. doi: 10.1002/14651858.CD001271.pub3
Le Zhou, M. S., and Hargis, J. (2020). Integrating live coding and interactive syllabus to engage students in an interactive media arts course. Online J. New Horizons Educ. 10:106. doi: 10.13140/RG.2.2.29465.57440
Li, L., Lin, M., Wang, X., Bao, P., and Li, Y. (2020). Preparing and responding to 2019 novel coronavirus with simulation and technology-enhanced learning for healthcare professionals: Challenges and opportunities in China. BMJ Simul. Technol. Enhanc. Learn. 6, 196–198. doi: 10.1136/bmjstel-2020-000609
Lin, B., Liu, J., Li, K., and Zhong, X. (2025). Predicting the risk of HIV infection and sexually transmitted diseases among men who have sex with men: Cross-sectional study using multiple machine learning approaches. J. Med. Internet Res. 27:e59101. doi: 10.2196/59101
Lin, Y., Wen, T., Shih, W., Vermund, S., and Fang, C. (2024). Impact of vaccination and high-risk group awareness on the mpox epidemic in the United States, 2022-2023: A modelling study. EClinicalMedicine 68:102407. doi: 10.1016/j.eclinm.2023.102407
Liu, Q., Luo, D., Haase, J., Guo, Q., Wang, X., Liu, S., et al. (2020). The experiences of health-care providers during the COVID-19 crisis in China: A qualitative study. Lancet Glob. Health 8, e790–e798. doi: 10.1016/S2214-109X(20)30204-7
Liu, X., Liu, C., Liu, G., Luo, W., and Xia, N. (2020). COVID-19: Progress in diagnostics, therapy and vaccination. Theranostics 10, 7821–7835. doi: 10.7150/thno.47987
Lo, N. (2024). The confluence of digital literacy and eco-consciousness: Harmonizing digital skills with sustainable practices in education. Platforms 2, 15–32. doi: 10.3390/platforms2010002
Lo, N., and Chan, S. (2024). “Chapter 25 gamification for higher education applications: A social learning theory approach,” in Handbook of research in online learning, eds T. Martindale, T. B. Amankwatia, L. Cifuentes, and A.A. Piña (Leiden: Brill). doi: 10.1163/9789004702813_025
Mahmood, S., Crimbly, F., Khan, S., Choudry, E., and Mehwish, S. (2020). Strategies for rational use of personal protective equipment (PPE) Among healthcare providers during the COVID-19 crisis. Cureus 12:e8248. doi: 10.7759/cureus.8248
Masters, K. (2019). Artificial intelligence in medical education. Med. Teach. 41, 976–980. doi: 10.1080/0142159X.2019.1595557
Mathur, S. (2020). “A. The perception of teachers on unlocking technology by redesigning education system during and after CO VID 19 pandemic lockdown,” in Educational Practices During the COVID 19 Viral Outbreak: International perspectives, ed. I. Sahin (India: ISTES Organization).
McGowan, E., Rosenthal, R., Fiore-Gartland, A., Macharia, G., Balinda, S., Kapaata, A., et al. (2021). Utilizing computational machine learning tools to understand immunogenic breadth in the context of a CD8 T-Cell mediated HIV response. Front. Immunol. 12:609884. doi: 10.3389/fimmu.2021.609884
McMurtrie, B. (2020). How to Reconnect with Students and Strengthen your Remote Course. Available online at: https://www.chronicle.com/article/How-to-Reconnect-With-Students/248461? (accessed March 1, 2025).
Merriel, S., Andrews, V., and Salisbury, C. (2014). Telehealth interventions for primary prevention of cardiovascular disease: A systematic review and meta-analysis. Prev. Med. 64, 88–95. doi: 10.1016/j.ypmed.2014.04.001
Meskó, B. (2023). Prompt engineering as an important emerging skill for medical professionals: Tutorial. J. Med. Internet Res. 25:e50638. doi: 10.2196/50638
Moghram, B., Nabil, E., and Badr, A. (2018). Ab-initio conformational epitope structure prediction using genetic algorithm and SVM for vaccine design. Comput. Methods Programs Biomed. 153, 161–170. doi: 10.1016/j.cmpb.2017.10.011
Morley, J., Machado, C., Burr, C., Cowls, J., Joshi, I., Taddeo, M., et al. (2020). The ethics of AI in health care: A mapping review. Soc. Sci. Med. 260:113172. doi: 10.1016/j.socscimed.2020.113172
Ngiam, K., and Khor, I. (2019). Big data and machine learning algorithms for health-care delivery. Lancet Oncol. 20, e262–e273. doi: 10.1016/S1470-2045(19)30149-4
Obermeyer, Z., Powers, B., Vogeli, C., and Mullainathan, S. (2019). Dissecting racial bias in an algorithm used to manage the health of populations. Science 366, 447–453. doi: 10.1126/science.aax2342
O’Boyle, C., Robertson, C., and Secor-Turner, M. (2006). Nurses’ beliefs about public health emergencies: Fear of abandonment. Am. J. Infect. Control. 34, 351–357. doi: 10.1016/j.ajic.2006.01.012
Owens, L., Currie, D., Kramarow, E., Siddique, S., Swanson, M., Carter, R., et al. (2023). JYNNEOS vaccination coverage among persons at risk for Mpox - United States, May 22, 2022-January 31, 2023. MMWR Morb. Mortal Wkly Rep. 72, 342–347. doi: 10.15585/mmwr.mm7213a4
Paudel, P., Sapkota, S., Gyanwali, K., and Adhikari, B. (2021). Comparison of vehicular fuel consumption and CO2 emission before and during the COVID-19 pandemic in Kathmandu Valley. J. Innov. Eng. Educ. 4, 10–17. doi: 10.3126/jiee.v4i1.34628
Pearlman, J. (2020). Australian Colleges Plan Deferrals as Chinese Students Face Travel Ban. Hong Kong: Caixinglobal.
Peters, M., Rizvi, F., McCulloch, G., Gibbs, P., Gorur, R., Hong, M., et al. (2022). Reimagining the new pedagogical possibilities for universities post-Covid-19: An EPAT collective project. Educ. Philos. Theory 54, 717–760. doi: 10.1080/00131857.2020.1777655
Pollock, A., and Lancaster, J. (2020). Asymptomatic transmission of covid-19. BMJ 371:m4851. doi: 10.1136/bmj.m4851
Poongodi, M., Hamdi, M., Malviya, M., Sharma, A., Dhiman, G., and Vimal, S. (2022). Diagnosis and combating COVID-19 using wearable Oura smart ring with deep learning methods. Pers. Ubiquitous Comput. 26, 25–35. doi: 10.1007/s00779-021-01541-4
Razu, S., Yasmin, T., Arif, T., Islam, M., Islam, S., Gesesew, H., et al. (2021). Challenges faced by healthcare professionals during the COVID-19 pandemic: A qualitative inquiry from bangladesh. Front. Public Health 9:647315. doi: 10.3389/fpubh.2021.647315
Rega, P., and Fink, B. (2014). Immersive simulation education: A novel approach to pandemic preparedness and response. Public Health Nurs. 31, 167–174. doi: 10.1111/phn.12064
Rose, S. (2020). Medical student education in the time of COVID-19. JAMA 323, 2131–2132. doi: 10.1001/jama.2020.5227
Sahu, P., Dalcik, H., Dalcik, C., Gupta, M., Chattu, V., and Umakanthan, S. (2022). Best practices for effective implementation of online teaching and learning in medical and health professions education: During COVID-19 and beyond. AIMS Public Health 9, 278–292. doi: 10.3934/publichealth.2022019
Sana, F., Isselbacher, E., Singh, J., Heist, E., Pathik, B., and Armoundas, A. (2020). Wearable devices for ambulatory cardiac monitoring: Jacc state-of-the-art review. J. Am. Coll. Cardiol. 75, 1582–1592. doi: 10.1016/j.jacc.2020.01.046
Sara, J. (2022). Lessons from a crisis-opportunities for lasting public health change from the COVID-19 pandemic. Front. Public Health 10:893871. doi: 10.3389/fpubh.2022.893871
Schifferes, S. (2020). Will Coronavirus be the Turning Point for Globalisation?. Available online at: theconversation.com/will-coronavirus-be-the-turning-point-for-globalisation-134739 (accessed March 1, 2025).
Senior, A., Evans, R., Jumper, J., Kirkpatrick, J., Sifre, L., Green, T., et al. (2020). Improved protein structure prediction using potentials from deep learning. Nature 577, 706–710. doi: 10.1038/s41586-019-1923-7
Shammi, M., Bodrud-Doza, M., Towfiqul Islam, A., and Rahman, M. M. (2020). COVID-19 pandemic, socioeconomic crisis and human stress in resource-limited settings: A case from Bangladesh. Heliyon 6:e04063. doi: 10.1016/j.heliyon.2020.e04063
Shi, F., Wang, J., Shi, J., Wu, Z., Wang, Q., Tang, Z., et al. (2021). Review of artificial intelligence techniques in imaging data acquisition, segmentation, and diagnosis for COVID-19. IEEE Rev. Biomed. Eng. 14, 4–15. doi: 10.1109/RBME.2020.2987975
Shi, H., and Hargis, J. (2020). Elevate instruction to an online environment. Glokalde Glob. Local Distance Educ. J. 6, 12–19.
Skums, P., Zelikovsky, A., Singh, R., Gussler, W., Dimitrova, Z., Knyazev, S., et al. (2018). QUENTIN: Reconstruction of disease transmissions from viral quasispecies genomic data. Bioinformatics 34, 163–170. doi: 10.1093/bioinformatics/btx402
Sofi, A., and Laafon, M. (2020). Effect of Using the Online Learning Platform in Teaching During the COVID-19 Pandemic. Educational Practices During the COVID-19 Viral outbreak: International Perspectives. Washington, DC: U.S. Department of Education, 167–180.
Stolerman, L., Clemente, L., Poirier, C., Parag, K., Majumder, A., Masyn, S., et al. (2023). Using digital traces to build prospective and real-time county-level early warning systems to anticipate COVID-19 outbreaks in the United States. Sci. Adv. 9:eabq0199. doi: 10.1126/sciadv.abq0199
Su, T., Lien, T., Yang, C., Su, Y., Wang, J., Tsai, S., et al. (2007). Prevalence of psychiatric morbidity and psychological adaptation of the nurses in a structured SARS caring unit during outbreak: A prospective and periodic assessment study in Taiwan. J. Psychiatr. Res. 41, 119–130. doi: 10.1016/j.jpsychires.2005.12.006
Tang, G., Westover, K., and Jiang, S. (2021). Contact tracing in healthcare settings during the COVID-19 pandemic using bluetooth low energy and artificial intelligence-a viewpoint. Front. Artif. Intell. 4:666599. doi: 10.3389/frai.2021.666599
Tang, G., Yan, Y., Shen, C., Jia, X., Zinn, M., Trivedi, Z., et al. (2020). Development of a real-time indoor location system using bluetooth low energy technology and deep learning to facilitate clinical applications. Med. Phys. 47, 3277–3285. doi: 10.1002/mp.14198
Thadani, N., Gurev, S., Notin, P., Youssef, N., Rollins, N., Ritter, D., et al. (2023). Learning from prepandemic data to forecast viral escape. Nature 622, 818–825. doi: 10.1038/s41586-023-06617-0
Thomas, S., Abraham, A., Baldwin, J., Piplani, S., and Petrovsky, N. (2022). Artificial intelligence in vaccine and drug design. Methods Mol. Biol. 2410, 131–146. doi: 10.1007/978-1-0716-1884-4_6
Turakhia, Y., Thornlow, B., Hinrichs, A., De Maio, N., Gozashti, L., Lanfear, R., et al. (2021). Ultrafast sample placement on Existing tRees (UShER) enables real-time phylogenetics for the SARS-CoV-2 pandemic. Nat. Genet. 53, 809–816. doi: 10.1038/s41588-021-00862-7
Turakhia, Y., Thornlow, B., Hinrichs, A., McBroome, J., Ayala, N., Ye, C., et al. (2022). Pandemic-scale phylogenomics reveals the SARS-CoV-2 recombination landscape. Nature 609, 994–997. doi: 10.1038/s41586-022-05189-9
Uchida, T., Park, Y., Ovitsh, R., Hojsak, J., Gowda, D., Farnan, J., et al. (2019). Approaches to teaching the physical exam to preclerkship medical students: Results of a national survey. Acad. Med. 94, 129–134. doi: 10.1097/ACM.0000000000002433
Unger, S., and Meiran, W. (2020). Student attitudes towards online education during the COVID-19 viral outbreak of 2020: Distance learning in a time of social distance. Int. J. Technol. Educ. Sci. 4, 256–266. doi: 10.46328/ijtes.v4i4.107
Uscher-Pines, L., Sousa, J., Jones, M., Whaley, C., Perrone, C., McCullough, C., et al. (2021). Telehealth use among safety-net organizations in california during the COVID-19 pandemic. JAMA 325, 1106–1107. doi: 10.1001/jama.2021.0282
Usinski, D. (2018). Disrupting Education: The Case For Open Educational Resources. San Diego, CA: University of California San Diego.
Wang, J., and Li, J. (2024). Artificial intelligence empowering public health education: Prospects and challenges. Front. Public Health 12:1389026. doi: 10.3389/fpubh.2024.1389026
Wang, S., Zha, Y., Li, W., Wu, Q., Li, X., Niu, M., et al. (2020). A fully automatic deep learning system for COVID-19 diagnostic and prognostic analysis. Eur. Respir. J. 56:2000775. doi: 10.1183/13993003.00775-2020
Wayne, D., Green, M., and Neilson, E. (2020). Medical education in the time of COVID-19. Sci. Adv. 6:eabc7110. doi: 10.1126/sciadv.abc7110
Wesson, P., Hswen, Y., Valdes, G., Stojanovski, K., and Handley, M. (2022). Risks and opportunities to ensure equity in the application of big data research in public health. Annu. Rev. Public Health 43, 59–78. doi: 10.1146/annurev-publhealth-051920-110928
Woodruff, J., Angelos, P., and Valaitis, S. (2008). Medical professionalism: One size fits all? Perspect. Biol. Med. 51, 525–534. doi: 10.1353/pbm.0.0056
Wu, H.-C., Shen, P. D., Chen, Y.-F., and Tsai, C.-W. (2016). Effects of web-based cognitive apprenticeship and time management on the development of computing skills in cloud classroom: A quasi-experimental approach. Int. J. Inf. Commun. Technol. Educ. 12, 1–12. doi: 10.4018/IJICTE.2016070101
Xu, B., Gutierrez, B., Mekaru, S., Sewalk, K., Goodwin, L., Loskill, A., et al. (2020). Epidemiological data from the COVID-19 outbreak, real-time case information. Sci. Data 7:106. doi: 10.1038/s41597-020-0448-0
Xu, X., Fairley, C., Chow, E., Lee, D., Aung, E., Zhang, L., et al. (2022). Using machine learning approaches to predict timely clinic attendance and the uptake of HIV/STI testing post clinic reminder messages. Sci. Rep. 12:8757. doi: 10.1038/s41598-022-12033-7
Yeung, A., Tosevska, A., Klager, E., Eibensteiner, F., Laxar, D., Stoyanov, J., et al. (2021). Virtual and augmented reality applications in medicine: Analysis of the scientific literature. J. Med. Internet Res. 23:e25499. doi: 10.2196/25499
Yuan, Y., Hargis, J., Lu, H., Lian, J., Huang, X., and Song, Y. (2022). “Embrace it, enjoy it, and work hard for it!”: A qualitative investigation into instructors’ reflections on rapid migration to online teaching,” in Transformative Dialogues: Teaching and Learning Journal, 14. Available online at: https://journals.kpu.ca/index.php/td/index (accessed March 1, 2025).
Zayapragassarazan, Z. (2020). COVID-19: Strategies for engaging remote learners in medical education. Online Submission 9, 1–18. doi: 10.7490/f1000research.1117846.1
Zhang, L., Tan, J., Han, D., and Zhu, H. (2017). From machine learning to deep learning: Progress in machine intelligence for rational drug discovery. Drug Discov. Today 22, 1680–1685. doi: 10.1016/j.drudis.2017.08.010
Keywords: monkeypox (Mpox), COVID-19, artificial intelligence, education, higher education
Citation: Abdelouahed M, Yateem D, Amzil C, Aribi I, Abdelwahed EH and Fredericks S (2025) Integrating artificial intelligence into public health education and healthcare: insights from the COVID-19 and monkeypox crises for future pandemic readiness. Front. Educ. 10:1518909. doi: 10.3389/feduc.2025.1518909
Received: 03 December 2024; Accepted: 25 March 2025;
Published: 17 April 2025.
Edited by:
Zhaohui Su, Southeast University, ChinaReviewed by:
Ahmet Cagkan Inkaya, Hacettepe University, TürkiyeCopyright © 2025 Abdelouahed, Yateem, Amzil, Abdelwahed and Fredericks. This is an open-access article distributed under the terms of the Creative Commons Attribution License (CC BY). The use, distribution or reproduction in other forums is permitted, provided the original author(s) and the copyright owner(s) are credited and that the original publication in this journal is cited, in accordance with accepted academic practice. No use, distribution or reproduction is permitted which does not comply with these terms.
*Correspondence: Salim Fredericks, c2ZyZWRlcmlja3NAcmNzaS1tdWIuY29t
Disclaimer: All claims expressed in this article are solely those of the authors and do not necessarily represent those of their affiliated organizations, or those of the publisher, the editors and the reviewers. Any product that may be evaluated in this article or claim that may be made by its manufacturer is not guaranteed or endorsed by the publisher.
Research integrity at Frontiers
Learn more about the work of our research integrity team to safeguard the quality of each article we publish.