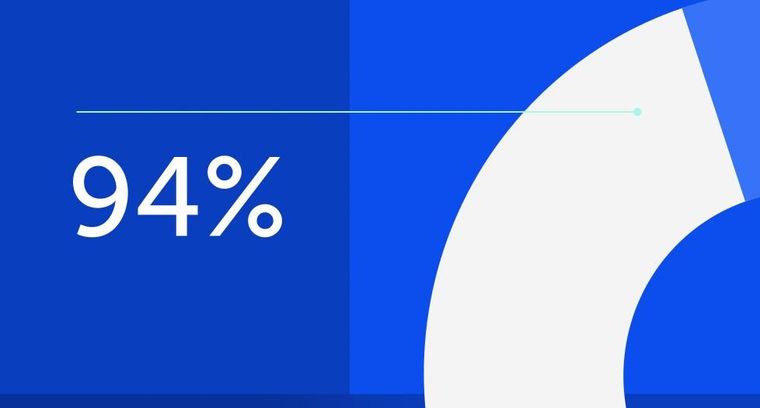
94% of researchers rate our articles as excellent or good
Learn more about the work of our research integrity team to safeguard the quality of each article we publish.
Find out more
ORIGINAL RESEARCH article
Front. Educ., 31 March 2025
Sec. Assessment, Testing and Applied Measurement
Volume 10 - 2025 | https://doi.org/10.3389/feduc.2025.1509904
This article is part of the Research TopicStudent Voices in Formative Assessment FeedbackView all 8 articles
This study explores students’ conceptions and experiences with feedback as integrated parts of a three draft writing process and group discussions in English as a foreign language (EFL) in three schools. The students (n = 106, six classes) were following the same draft writing process design during a full school day. The same assignment was given to all participating students, but different feedback contexts were assigned in each school. Half of the students received AI-generated feedback (context 1), while the remaining received peer feedback (context 2). Observations were conducted in all classes during the writing assignment. Individual interviews with students (n = 22) were used to investigate students’ experiences during the draft writing process, and the interviews were thematically analyzed. We find that while AI-generated feedback information supported students dialogic feedback interactions the peer feedback context allowed for students to rehearse their assessment and feedback strategies. The study also reveals that peer feedback for lower secondary school students is challenging, since the students function as both feedback givers, receivers and users during the draft writing process. Key aspects regarding how the students engaged with AI-generated feedback and peer feedback are discussed and we find that both feedback contexts have the potential to develop feedback literacy among lower secondary school students. Our study can contribute to the growing understanding of the relationship between feedback contexts, lower secondary students’ uptake of feedback, and how feedback literacy could be developed.
In this study we explore lower secondary students’ conceptions and experiences with formative feedback during a three draft writing process. Both AI-driven and peer assessment contexts are examined. We investigate student conceptions of feedback, how they engage with feedback, and whether they act upon feedback they receive, seek and engage with. In our study a writing task was designed specifically to involve students actively in feedback loops during the writing process. By examining how students experience integrated formative feedback loops, we wanted to explore their roles not just as receivers of feedback but also their roles as active participants (e.g., assessors and discussion participants) in the writing process. The study leverages a design-based approach, incorporating student voices through interviews and a thematic analysis to contribute to the understanding of how feedback influences students’ learning experiences and development of feedback literacy (Andrade et al., 2021; Sutton, 2012).
Hattie and Timperley (2007) suggest it is useful to consider a continuum of instruction and feedback and emphasize that feedback has no effect in a vacuum, and that its power must be related to the learning context in which feedback is addressed. This notion elucidates feedback processes as situated practices. Feedback has a powerful influence on student learning and achievement (Hattie and Timperley, 2007; Lipnevich and Panadero, 2021), and assessment for learning and formative feedback are found to be particularly effective in promoting learning (Black and Wiliam, 2009). Formative and summative feedback is often perceived as dichotomous, and mutually exclusive. However, summative assessment can enhance student learning if the embedded information is used formatively (Black et al., 2011; Gamlem et al., 2024). Assessment and feedback are formative when progress is elicited, interpreted and used to inform decisions about further steps likely to improve the learning process and hence also further progress (Sadler, 1989; William and Leahy, 2007). Formative feedback could be understood as information provided from various sources (e.g., from teachers, peers, or technology), and in addition to stemming from diverse sources feedback could also have different modes. Within assessment for learning feedback should be embedded in learning trajectories and take advantage of the critical moments where assessment makes learning change direction (moments of contingencies) (Black and Wiliam, 1998a,2009). However, classroom feedback practices have historically resisted change due to a focus on one-way information delivery to learners and students (Boud and Molloy, 2013). Such a one-way transmission model of feedback information is by Gamlem and Smith (2013), van der Kleij et al. (2019) argued for as questionable since it assumes a passive student role and thereby overlooks the importance of issues related to how feedback is received, interpreted and made use of by the learner. Feedback practices to enhance learning should therefore involve deliberate and purposeful transformations in classroom practices, content wise but also regarding learning processes, roles and relationships (Andrade et al., 2021). Dialogic feedback interactions might help students construct a path forward but is seldom employed in the classroom setting (Gamlem and Smith, 2013). Interactive dialogues between the teacher and the students or among the students can thus be seen as powerful for students’ learning.
Askew and Lodge (2000) uses the metaphors feedback as a gift and feedback as collaborative work. By doing so they emphasize the difference between being a passive recipient of feedback information (feedback as a gift) and the active two- or multiple way-involvement and engagement in feedback (feedback as collaborative work). When feedback is used in a formative process, students often become more active and engaged in regulating their learning (Andrade, 2010; Brandmo et al., 2020), and involving teachers and students in feedback loops, individually and collectively, is perceived to increase the quality of learning (Black and Wiliam, 1998b; Hattie and Timperley, 2007; Pedder and James, 2012). The importance of being an active participant in feedback processes is associated with the basic assumptions in self-regulated learning; That learners (ideally) gradually develop cognitive and affective strategies, empowering them to monitor and lead their own learning process (Andrade, 2010; Andrade et al., 2021). Emphasis on the active role of the learner is also reflected in an ongoing conceptual shift from analyzing feedback as external input (from a feedback giver) to analyzing the mechanisms involved in how feedback is received (within the feedback receiver) (Lui and Andrade, 2022).
The active feedback recipient role is by Carless and Boud (2018) elucidated as they describe feedback as «[a] process through which learners make sense of information from various sources and use it to enhance their work or learning strategies […] Information may come from different sources e.g., peers, teachers, friends, family members or automated computer-based systems to support student self-evaluation of progress» (Carless and Boud, 2018, p. 1315–1316). According to Carless and Boud (2018) a well-developed feedback literacy is a precondition for such a sense-making process to occur. Sutton (2012, p. 33) was the first to introduce the concept of feedback literacy and defined the concept in the following way:
“[…] a set of generic practices, skills and attributes which, like information literacy […] is a series of situated learning practices. Becoming feedback literate is part of the process that enables learners to reach the standards of disciplinary knowledge indicated in module and program learning outcomes […] that assists learners in forming judgments concerning what counts as valid knowledge within particular disciplines; and that helps them develop the ability to assess the quality of their own and others work.”
In Sutton (2012) further conceptualization of feedback literacy, three dimensions are identified and unpacked: (1) The epistemological dimension (feedback on and for knowing), (2) the ontological dimension (developing educational being through feedback) and (3) the practical dimension (acting upon feedback). According to Sutton (2012) feedback literacy requires much more than students’ understanding and accepting why they have received a certain grade, and his distinction between feedback on- and for- knowing could be perceived parallel with the distinction between summative and formative assessment and feedback within the assessment for learning-domain. Whilst feedback on knowing (FoK) on one hand has potential to boost learners’ confidence in what they already know and do, it also tends to constitute learner identity as more or less successful. Feedback for knowing (FfK), on the other hand, is formative in character and indicate an openness to the “possibility of movement and development” (Sutton, 2012, p. 34). But engaging with FfK seems to be more challenging than FoK since it can require students to “change their mode of knowing the world and themselves” (Sutton, 2012, p. 35). In other words, developing Ffk will require the learner to understand their own potential for development and growth. Developing educational being through feedback is therefore the second dimension of feedback literacy conceptualization as proposed by Sutton (2012). The main point made is that feedback literacy development will change the relationship of learners to themselves and their educational world, implying that their confidence could both increase and decrease along the way. The third and final dimension unpacked by Sutton (2012) is the practical dimension: The ability to act upon, or feed forward, the feedback given and received. It is argued that learners cannot be assumed to possess skills needed to act on feedback. Therefore, explicit guidance concerning how to act and feed forward may be needed to various degrees. Sutton (2009, 2012) also highlight the need to address and overcome language barriers which could influence learners’ capacity to understand, interpret and act upon feedback. The third dimension thereby underline the necessity of learning to decode feedback information.
Carless and Boud (2018) have later expanded on Sutton (2012) dimensions, and they propose a set of four inter-related features, which could constitute a framework underpinning feedback literacy of students. These are: (1) appreciating feedback processes, (2) making judgments, (3) managing affect and (4) taking action (acting). The framework further supposes that maximizing the mutual interplay of first three features will maximize the potential for students to act on feedback provided. It should be noted that the framework describing feedback literacy has been developed in higher education and most research regarding feedback literacy have been conducted in higher educational contexts. For this reason, we use this framework as a starting point to investigate feedback literacy in other contexts (e.g., lower secondary school). However, in regard of our study, it should also be mentioned that feedback literacy might influence a student’s engagement and effectiveness in using feedback to improve writing. Graham (2018) emphasizes the potential to build writing confidence for the student, by providing feedback on techniques and language to develop their writing. Helping students develop self-generated feedback by providing concrete strategies for monitoring progress is crucial for fostering feedback literacy, as it creates opportunities for internal and external feedback to converge when the writer engages in text review (Fiskerstrand and Gamlem, 2023).
Peer assessment is alongside self-assessment by many advocated as a central part of formative assessment and assessment for learning practices, and peer feedback has been recognized as a meaningful approach to enhance student engagement (Andrade et al., 2021; Panadero, 2016). According to Gielen et al. (2010) peer assessment is an assessment form performed by equal status learners, which does not contribute to final grades. This type of feedback has a qualitative output aim, where strengths and weaknesses of a specific task- or activity performance (and recommendations for further improvement) are discussed. Peer assessment could be perceived as a learning tool for developing students’ judgment and ability to recognize quality work, and according to Nicol (2021) feedback designs could benefit from turning natural comparisons (that students are making anyway) into formal and explicit comparisons potential for learners. Gielen et al. (2010, p. 144) argue that students “peer assessment skills can be trained so that their feedback becomes as effective as teacher feedback in the end.” Gielen et al. (2010) found that peer feedback can substitute teacher feedback without a considerable loss of effectiveness in the long run. However, despite being optimistic around the use of peer assessment in classroom practices, studies investigating the efficiency and usefulness of peer feedback vary in their findings. While some studies have suggested that peer feedback can be as effective as traditional teacher feedback, the findings are not consistent. For instance, Double et al. (2020) concluded that there are positive effects of peer assessment compared to teacher assessment. But the study also pointed out that when grading is incorporated in peer feedback on students’ learning it is only beneficial for tertiary students but not primary or secondary school students. Cho and Schunn (2007) reported no significant difference in student performance between single peer feedback and teacher feedback, suggesting that peer assessment may be equally valid in certain contexts. However, Yang et al. (2006) found that teacher feedback led to greater performance improvements, indicating that the effectiveness of feedback methods may vary depending on the nature of the task and the students involved. It is also important to recognize the challenges associated with peer feedback (Panadero et al., 2023).
Peer assessment activates several motivational, cognitive and emotional processes with a potential to enhance the learning of the assessor and the assessee, and it thereby involves multiple social and human factors, since peer assessment does not happen in a vacuum (Panadero, 2016; Panadero et al., 2023). Peer assessment produces thoughts, actions, and emotions as part of the feedback interactions, and according to Panadero (2016) the traditional focus on the accuracy and reliability of peer assessment information has been too extensive and he thereby encourage a shift of focus to what happens during feedback interactions. Panadero (2016) further argues that peer assessment could not be perceived a specific concept, ready to be implemented in teaching and learning, but rather as a variety of practices, involving both human and social capacities. Gamlem and Smith (2013) highlighted that peer feedback can sometimes be perceived as damaging due to disrespectful behaviors exhibited by peers during the feedback process. These findings are in line with the complex relationships between students’ inner processes and the relation between participants during peer assessment processes, which according to Panadero (2016) entail both intra-individual factors and interpersonal aspects in addition to cognitive aspects. In their recent systematic review Panadero et al. (2023) elaborate on the characteristics of intrapersonal factors (variables within the learner) and interpersonal factors (relationships between learners) in peer assessment. Intrapersonal factors include motivation to perform, and emotions experienced as feedback is expected to increase learning and self-regulation strategies (e.g., Double et al., 2020). Interpersonal factors on the other hand include the relationships between the assessor and the assessee and the level of trust and psychological safety between participants during an assessment process (Panadero et al., 2023). The findings reveal poor reporting of peer assessment intervention characteristics and concluding remarks call for an increased focus on formative implementations to produce better intrapersonal and intrapersonal results (Panadero et al., 2023).
Peer assessment is itself a complex activity, since it activates several inter- and intrapersonal factors and processes. In addition, another layer of complexity is added, since the individual learner often must shift between roles during the feedback process. The different role characteristics as assessor and an assessee have different psychological and interpersonal consequences and activates different emotions and strategies (Panadero, 2016; Panadero et al., 2023). However, little research has distinguished between- and explored the contrasts between these two roles (Panadero, 2016). Despite adding another layer on complexity, an important argument for the use of peer assessment is that it enhances both learning and performance in two distinct ways: The assessee receives direct feedback on how to improve and the assessor become more aware of their own strengths and weaknesses (Panadero, 2016). Peer assessment therefore enhance self-assessment capability, and students self-regulated learning where peers act as co-regulators of assessee peers. Peer assessment is an opportunity to learn more, and most students have positive attitudes toward peer assessment and often report to have learning gains (Panadero, 2016).
Over the recent decades, numerous forms of digital feedback systems have emerged in the educational field, and technology-enhanced assessment (TEA) and technology-enhanced feedback (TEF) has become growing areas of development and research (Munshi and Deneen, 2018). In accordance with general assessment research, early development and studies focused on the efficiency and accuracy of automated scoring systems (associated with summative assessment). But as the field of assessment shifted focus toward formative feedback and assessment for learning, the growing awareness of what constitutes quality feedback revealed limitations regarding how technology could support and enhance assessment and feedback processes (Munshi and Deneen, 2018). Still, the last two decades of rapid technology development has brought far more sophisticated technologies, as technologies have emerged and converged. Digital technologies now enable communication through multiple combinations of modality (e.g., text, images, and sound), and the internet infrastructure have enabled such multi modal information and communication to overcome previous limitations regarding the co-location and synchronic participation in feedback processes. The development of more sophisticated algorithms has also enabled personalized, individualized, and timely feedback to customize and facilitate student activity while continuous feedback is offered.
Munshi and Deneen (2018, p. 341) identified three distinct processes that could enhance feedback during feedback provision across different feedback technologies. These are: “(1) acquiring information from the student during some learning activity, (2) transforming the acquired information into a feedback message, and (3) conveying the feedback message to the student.” In their analysis they found that four out of ten TEF-systems in the literature enhanced feedback by acquiring information, transforming information, and conveying information, and these four systems were: e-learning applications, automated marking systems, intelligent tutoring systems (such as AI-generated feedback) and computer games. Huang et al. (2023) did a similar review focusing on how technology could assist the feedback process, and they found that technology could support (1) generating feedback, (2) delivering feedback and (3) using feedback. However, in their concluding remarks both Munshi and Deneen (2018), Huang et al. (2023) point at important blind spots: Research on student uptake of technology-based feedback and the characteristics of quality feedback in technology remains limited. This is concerning since many educational- and feedback technologies claim to contribute to bolster and enhance metacognition and self-regulated learning (e.g., Knight and Buckingham Shum, 2017; Pardo et al., 2017).
Despite challenges to provide formative feedback in educational settings, utilization of automated- and artificial intelligence (AI) technologies in assessment practices has been perceived an important contribution to increase reliability and reduce human bias and the risk of making mistakes (Chen et al., 2020). The functionalities of automated feedback- and scoring systems (including artificial intelligence technologies) are expected to serve as means to improve assessment practices as it speeds up marking time, reduces or removes human bias and increase the accuracy and reliability of assessment (Richardson and Clesham, 2021). Automated feedback- and scoring systems are already applied in various educational contexts across educational levels but are mostly studied in computer science- and online courses in higher education. When studied, automated- and AI applications are found to perform assessment at a high accuracy and efficiency level as long as large and relevant datasets are available for training the systems (machine learning) (Zawacki-Richter et al., 2019). In addition, automated- and AI technologies have the potential to increase reliability and validity of assessment and could thus assist both student learning trajectories and teachers’ overall assessment practices (Chen et al., 2020). But debates regarding the role of automated- and intelligent feedback systems in formal education still fuel a fear of machines taking over the role of humans as nuanced examiners (Baker, 2016; Richardson and Clesham, 2021). Issues related to the role of judgment in assessment and feedback practices thereby also seem to influence an emerging field of research aiming to reduce the risk of bias and human mistakes in assessment practices. This clearly demonstrates that ongoing debates about the importance of summative accuracy vs. the quality of formal feedback live side by side also in research revolving technology assisted assessment and feedback. According to Liu et al. (2016) it is necessary to evaluate the applicability of traditional learning theories in contexts infused with computer technology. Strengths associated with educational use of tablets and computers are also related to shifts of roles in the classroom, frequent transitions, and the facilitation of varied activities including collaboration and discussion. This is in line with previous mentioned debates regarding the importance of active participation in both formal and informal feedback processes during a learning process.
AI-generated feedback have been found to offer diverse benefits in language learning, primarily through personalized and efficient responses. According to Barrot (2023), Engeness and Mørch (2016), AI tools adapt feedback to each student’s needs, providing targeted support that addresses specific areas of improvement. These systems also process large volumes of writing quickly, making them scalable for educational settings (Giannakos et al., 2024). Additionally, AI tools can support formative assessment, offering immediate feedback that fosters self-directed learning and helps students independently address their errors (Shadiev and Feng, 2023). However, AI-generated feedback has certain limitations. For example, it may struggle with understanding the context and nuances of student writing, leading to generic or misaligned responses (Barrot, 2023). There is also a risk of students becoming overly reliant on AI corrections, potentially weakening their self-editing and critical thinking skills (Giannakos et al., 2024). While effective for surface-level errors, AI feedback may not address more complex aspects of writing, like creativity and argumentation (Hopfenbeck et al., 2023). Furthermore, ethical concerns, including data privacy, need careful consideration (Giannakos et al., 2024). In summary, while AI-driven feedback is valuable for its personalized, immediate support, it should be balanced with traditional teaching methods to address its limitations and maximize its educational impact.
While previous research has offered useful and detailed conceptualizations of feedback, more knowledge is needed on how students perceive and engage with diverse types of feedback, particularly in the complex context of digital learning environments. This study specifically investigates lower secondary students’ conceptions and experiences with feedback information from an AI-driven feedback system and per assessment within a pre-planned and structured writing process. To do so, we interviewed students in how they engage with feedback information to improve their own text (three paragraph essay) throughout a three draft writing process. The group of participating classes were split in two, and two different feedback contexts were investigated. The design of the writing trajectory was the same in both contexts, but in one context the students received feedback information from an AI-driven computer-based system, and in the other the students received peer feedback. While existing research acknowledges the importance of feedback literacy (Andrade et al., 2021; Sutton, 2012), our study contributes to the emerging knowledge of issues regarding feedback literacy development in lower secondary school contexts. By employing two different feedback information sources we can describe some similarities and differences regarding how the participating students act on feedback according to their assigned context.
The aim of this study is to explore lower secondary students’ experiences with feedback as integrated parts of their three draft writing process and group discussions. By exploring the student’s experiences with AI-generated feedback (context 1) and peer assessment and feedback (context 2) our study contributes with nuanced and situational knowledge regarding how students perceive feedback in two different contexts. Understanding how students view and experience both peer feedback and automated feedback during a writing process is essential for identifying strategies that might enhance their learning experiences and foster engagement in an increasingly digital and collaborative educational environment. In addition, we explore and discuss similarities and differences between receiving automated AI-driven feedback (context 1) and peer-feedback (context 2). We take a closer look at students’ uptake of feedback, dialogic feedback interactions and feed forward actions in response to the feedback (Carless and Boud, 2018) in the two contexts. The following research question (RQ) drive the study: How did lower secondary students from two feedback contexts (peer feedback and AI-generated feedback) experience the process of receiving-, discussing- and acting on feedback during a three draft writing process?
This project is a design-based research (DBR) study (Brown, 1992, The Design-Based Research Collective, 2003). The requirement to develop practical design principles through iterations of testing and development is considered a key strength of DBR (Anderson and Shattuck, 2012). However, design-based research should not merely focus on enhancing classroom practices and learning processes in real life-context. It should also address theoretical questions and issues (Collins et al., 2004). In the present study, we employ data procured from the initial development phase (first iteration) of the AI-driven technology Essay Assessment Technology (EAT). EAT is specifically designed to facilitate assessment and feedback for learning during students writing processes and is programmed to provide feedback based on predetermined sets of criteria. The EAT system acquires information from learners texts and generates, transforms and delivers feedback to the students in a one-step process. It offers various functionalities, the most relevant to the present study being its focus on writing mechanics, such as spelling, punctuation, missing commas, and capitalization errors. Instead of providing the correct answers, the feedback highlights that the text requires improvement and suggests how revisions should be made. This feedback signals to students that a specific word or sentence has an issue needing attention. In this way, the EAT distinguishes itself from many other AI tools, such as Grammarly or Word. These tools predominantly suggest changes to the text, requiring users to either accept or reject the modifications. In contrast, the EAT moves beyond static corrections by offering developmental feedback designed to encourage reflection and learning about the reasoning behind the errors. This approach promotes a deeper understanding of the writing process.
The experiences of the students, as captured through interviews conducted immediately following their writing and feedback interactions, were subjected to rigorous coding and thematic analysis. Consequently, this research adopts a qualitative interview study methodology. Considering that the researchers have been actively involved in the design of the learning and feedback trajectories, in addition to observing the writing process throughout the data collection day(s), they possess an in-depth understanding of the context to which the students refer. This facet of the research enhances its ecological validity (Gehrke, 2018).
The sample in this study consists of students from six classes at three different schools in a municipality in Eastern Norway. The students were lower secondary students in the eighth grade (12–13 years old). As visually represented in Table 1 below, a total of 106 students participated. One class from each school were using AI-technology (context 1) where the students received feedback on their written work from the AI-driven EAT software. Simultaneously, another class from the same school used peer assessment (context 2). Peer feedback was grounded in same predetermined assessment criteria that EAT utilize in its automated feedback. This ensured consistency in the study design.
Table 1. Overview of the participants, participating schools, context 1, context 2 and days of data collection.
The project was approved by the Norwegian Agency for Shared Services in Education and Research (SIKT) and informed consent was provided by all participating students and their legal guardians.
In the current study we focus on a three draft writing process. The lower secondary students completed a writing task over the course of one school day, starting in the morning in their own classrooms. The participating students were part of their normal classes which made the classroom environment similar to their everyday learning context. To ensure comparable consistency a group of three researchers from the research team was present alongside the teacher in all classes (at all three schools), to observe during the students’ writing process. The physical classroom setup was pre-arranged in the same way for context one (AI-generated feedback) and context two (peer feedback) and across all three schools. The students were placed in groups (4–5 students) by their teachers and the students’ desks were grouped beforehand by the teachers so the students would not have to spend time forming groups when discussing the feedback provided. The task assignment was printed for all students and was handed out during the introduction to the task itself and the assigned feedback context trajectory (Table 2). The time allocated for each activity during the writing process was pre-planned by the team of researchers in collaboration with the teachers. The wording of the assignment was as follows: “Write a story about someone you consider to be a hero. It can be a fictional or a real person doing something heroic. The length of your essay should be approximately 400 words.”
All students across the two contexts had 40 min to write their first drafts. Students from context 1 wrote their texts in the EAT text editor while students from context 2 wrote their texts in Microsoft Word. As the first step of the feedback loop, the students from context 1 pressed the feedback button in EAT, which immediately submitted their drafts to the automated feedback process (driven by AI). The software technology in turn generated and delivered feedback on students’ language (spelling, punctuation, grammar). The automated feedback loop was followed by a 10 min review where students were expected to select three feedback points from EAT to address in the following group discussion. Students from context 1 then participated in a 20 min group discussion, before revising their drafts for 40 min and submitted a second version for a second feedback loop. The corresponding first step of the feedback loop students assigned to context 2 reviewed a peer’s draft for about 15 min by using an assessment rubric. The rubric was developed and printed by the researchers and handed out by the teachers. The rubric was designed to ensure that the feedback would address the same language categories as EAT (thus focusing on spelling, punctuation, and grammar). The students in the peer feedback context were then expected to provide feedback to their assigned peer before they engaged in a group discussion to support the revision process of their peer’s work for about 15 min. Like the students in context one the students further revisited their drafts for another 40 min before a second feedback loop. Feedback information could be given in English or Norwegian to ensure clarity and reduce language barriers. Both groups from each school completed two feedback loops, producing a third draft for final submission. The entire three draft writing process spanned 180 min, and the students submitted their final draft to a Microsoft Teams folder created by the class teacher. Throughout the process, teachers were available in all classes to address questions, and they were otherwise expected to assist and facilitate the process like they would normally have done. The full writing process and integrated feedback loops are summarized in Table 2.
During the day of the three draft writing process the project group of researchers collected data (students’ essays, recorded video for observation, student- and teacher interviews etc.) from both feedback contexts. Of the 106 participants, 22 students (11 from each context) were interviewed by the researchers who had been present in their classroom during the day. All student interviews were conducted according to a semi-structured interview guide, developed by the team of researchers. The questions in the interview guide were designed to initiate a conversation about students’ experiences with receiving, giving and engaging with feedback during the draft writing process, but also at school in general. However, the interviewing researchers also asked follow-up questions if they wanted the students to elaborate on aspects initiated by the students or if they felt the need to rephrase one or more question(s). The student interviews could therefore be described as semi-structured interviews (e.g., Kvale and Brinkmann, 2009). The student interviews were conducted in the end of the day, after finishing the writing process, this to ensure consistency in the context and environment in which the data were collected. This approach minimizes variations that may arise from differing circumstances, thereby enhancing the reliability of the findings. Conducting the interviews at the end of the process allows for a more coherent comparison of responses. This ensures that the experiences discussed remain fresh in the students’ memories providing a clearer understanding of the students’ experiences as opposed to delayed interviews.
The initial transcription of the student interviews was conducted by an AI-driven “voice to text” software tool. However, the quality of some of the transcripts were quite poor and the researchers therefore had to thoroughly compare the raw data sound files with the transcripts and manually correct them, resulting in what Da Silva (2021) describe as semi-automated transcripts. Since the aim of this study was to explore students’ conceptions of feedback and their experiences with feedback loops as integrated parts of their three draft writing process we applied a thematic analysis. The thematic analysis is by Braun and Clarke (2006) described as a foundational method for qualitative analysis and it is characterized by the flexibility needed to describe patterns across qualitative data. In the initial coding process in vivo- and preliminary coding was applied to recognize main patterns in students’ conceptions and experiences (Silverman, 2019; Saldaña, 2013).
The codes reflected students’ general conceptions of feedback, and their experiences with receiving (and giving) feedback, discussing feedback and acting upon feedback during the writing process. Reflecting the students’ own perspectives and voices was an important part of the study, and the initial coding of the transcripts therefore prioritized inductive coding over theoretical coding and lifeworld experiences over theoretical coding categories [e.g., Braun and Clarke, 2006; Rapley in Silverman (2021, p. 341–356)]. During the initial coding, patterns of similarities and differences between how the students in the two different context experienced the feedback were revealed (saturation). Student interviews were therefore further analyzed according to theoretically informed categories related to the contextual factors embedded in the assigned feedback contexts. The final categories applied to transcripts from the two contexts are presented in Table 3.
Across both contexts, the students were positive about receiving feedback on their schoolwork in general. They emphasized that receiving feedback helped them improve their work and their texts and they all appeared to have a formative view of feedback aligned with assessment for learning. The students linked their conceptions of feedback usefulness to being able to receive and act upon information that could help them uncover blind spots in their work or understanding:
When you have done an assignment for example, it’s a bit fun or exciting to get feedback. On what you have done and such […] If I have done something wrong, and they say it’s wrong without me knowing it myself, it’s easier to change it (Student 4, context 2).
Students also described their perceptions in ways associated with task- or process level performance, indicating that they find feedback on self-level less useful: “[…] because then you know what to change. What they think of your story. It’s fun, and much better than just a thumbs up. It’s better that they write what they think instead of just a thumbs up. Then you get a lot more answers.” (Student 1, context 2). However, even if all students said they valued feedback on a general level, patterns regarding their assigned contexts during the three draft writing process seemed to shape parts of their further reasoning. Students assigned to the AI generated-feedback context mainly pointed at feedback from both teachers and fellow peers as useful: “If I need help in a subject I ask a student. The one sitting next to me. So, he says that maybe you should change this and this, and then you get the correct answer. Like that.” (Student 3, context 1). As opposed to students from context 1 (AI-feedback), students from context 2 (peer feedback) explicitly addressed feedback from peers as less useful despite being asked about general experiences of receiving feedback. Some explicitly linked their view to the formal education of a teacher: “Maybe the teacher knows more. For example, about grammar. And not everyone knows what to assess. So maybe it is easier to trust the teacher then. If you understand” (Student 9, context 2). Others expressed doubting feedback given by fellow students since they might be wrong or not taking the feedback process seriously: “Not everyone takes it seriously, and it’s [feedback] not so good from other students. At least I don’t take it very much in” (Student 6, context 2).
During the three draft writing process students assigned to feedback context 1 received AI-generated and transformed feedback. The students described process writing with integrated feedback loops and writing in the EAT system and receive AI- generated as new to them. “It was a bit difficult and confusing at first but got better during the day” (Student 3, context 1). The interviewed students enjoyed working in the program, and many of them explicitly explained how they interacted with the technology and engaged in the received feedback to improve their work: “I thought it was incredibly good. I think you get much more feedback than in word. I think this writing program is better than word. [.] I saw where the errors were, and then I corrected where there was an error” (Student 2, context 1). “I received feedback that said: (quotes program feedback) here you have three sentences in a row that start with the same word (ends program feedback quoting). It was genuinely like (student makes wow-sound), because I don’t usually do that. So that was exceptionally good, because it annoys me when I read other people’s texts and they start with the same word several times” (Student 3, context 1). Some students also experienced confusion when the AI-algorithm provided some generic and misaligned feedback information. One student described how the feedback identified a name as a repeating word: “I wrote about Nelson Mandela in the first sentence and had Nelson Mandela as a headline. The others got errors on similar things too. I was a bit confused by that” (Student 3, context 1). The above quote reflects yet another pattern visible: By comparing the generated, transformed and conveyed feedback received, the students were able to detect and reveal generic and misaligned response from the AI- generated feedback.
During the three draft writing process students assigned to feedback context 2 received peer feedback. The students described process writing with integrated feedback loops during a school day as new to them, and both giving and receiving peer feedback was something they had to get used to throughout the day: “It was difficult the first time, to figure out how to assess the different ones. It went fine the second time. Then I knew a little more about what it was like to have to assess” (Student 9, context 2). The interviewed students varied in their description of the feedback they received. Some of them perceived feedback from their peers merely as differences in personal preferences and would not act on the feedback unless feeling sure it could give a higher grade. A common pattern among students from the peer-feedback context was that they differentiated between their fellow peers regarding whether they trusted the feedback to be valuable or not. Their arguments were mainly related to the perceived competence of the peer, the perceived value of the feedback, or both. One student said: “(student name A) wrote wrong on things, and it wasn’t so useful, so I didn’t take it all in. But (student name B) who sits next to me gave genuinely good feedback. That was very good.” (Student 10, context 2). Some students from the peer feedback context also differentiated regarding who they trusted as recipients of their feedback when they were at the giving end of the feedback process. One student described how they normally don’t find it difficult to give feedback to other students but also added: I did’t dare to give to (student name), because (they) was a bit high on (themselves) (Student 12, context 2). One student from the peer feedback context ended up in a group with what they describe as “the smart ones in class,” and experienced the feedback provided as particularly useful: “They know where to put commas and full stops. If I had done something wrong, they also showed how to correct it and what it was and such things” (Student 11, context 2). The student further described how fellow students pointed out errors and that they then assisted the understanding of how to correct the errors that were identified. On the other hand, the student also experienced difficulties as the giver of feedback to the smart ones: “It was a bit difficult because I’m not that good, and then I was a bit unsure about what to give feedback on. It is difficult t to give feedback because I’m not that good in grammar and such things, and then it can be difficult to find errors […] it’s easier to give feedback on things you’re good at yourself” (Student 11, context 2). The student further described the feedback giving process as vulnerable.
I’m unsure of myself and think a lot about myself. It becomes a bit personal in a way. And then the others are going to sit and listen. And all eyes are on me. If everything is good, then it’s easier. I think it’s easy (to give feedback) as long as I understand the text, and then I know what to say (Student 11, context 2).
Another student from the peer feedback context used the inherent spelling tool in Microsoft Word to describe their difficulties verbalizing peer feedback information: “It’s easy to understand that red line means wrong word and blue line means that it is correct, but that the spelling is a bit wrong, or it needs a comma or full stop.”(Student 13, context 2). The student further said that they wrote too short and could have asked for tips for more content but chose not to do anything with the feedback they got. The student said that it was not so difficult to understand the feedback, but at the same time they pointed out that they found it difficult to read and hence interpret and make use of feedback. “I didn’t do anything, really. I just continued to do my things, but I listened a bit to it. […] I have reading difficulties and struggle a bit to keep up, really” (Student 13, context 2).
When exploring student experiences regarding dialogic feedback interactions the students who received AI- generated feedback (context 1) were generally positive toward the group discussions. Many of the students described and exemplified how the collaborative discussions supported the process of identifying, interpreting, and making use of the feedback and comparisons: “It was better with group work than if we had just worked one by one. Because then you can talk together and give each other feedback and such. It helps a lot when for example I told them things and then they gave me tips on what I could change.” (Student 14, context 1). Some students found the discussions useful beyond correcting existing errors. By taking part in group discussions, they experienced being reminded of what not to do in their further draft-writing-process:
I talked to the others at my table. Some of us had the same errors and others not. And then we went through the text and corrected what was wrong. And then we went through the text a few times and moved on to the next stage. It was a good reminder, because when I saw what the others had done wrong - which I might not have done wrong this time, it helped me remember when I was going to write my next draft (Student 3, context 1).
However, the dialogic feedback intentions of the group discussions were experienced to be far less fruitful by students from the peer feedback context (context 2). Some of the students didn’t discuss or explore the feedback received at all and their experiences were limited to merely reading aloud from the assessment rubric sheet without discussing. Others simply exchanged assessment rubric sheets. One student described how the lack of feedback and feedback interactions made them seek feedback elsewhere: “I didn’t get much feedback from the group, so I sought out three others and they gave me feedback and it became easier” (Student 11, context 2). The student further described how their peers emphasized potential grades above providing useful feedback information: “At first there wasn’t much useful information that could help me improve other than that I was believed to be at a [grade] four or five then” […] But at the end I realized that I needed more paragraphs, so it would have been nice if someone had brought it up before” (Student 11, context 2). As previously stated, students from the peer feedback context seemed to struggle even more with the assessor role than with interpreting and making use of feedback they had received. One student summed up their experience of the peer feedback and the following group discussion in the following way: “We haven’t done this before, so the feedback wasn’t long.” (Student 15, context 2).
All student informants across the two contexts agreed that writing a text through a three draft writing process (with integrated feedback loops) was helpful and offered a structured approach to writing. However, they expressed their viewpoints differently, likely influenced by the feedback context they were assigned. All the interviewed students who got AI- generated feedback (context 1) said that they had tried their best to act on the feedback they received:
It was actually very good. The first time I made many mistakes, but after I received feedback, I made fewer and fewer mistakes. Because then you get to check first if what you are doing is correct, and then correct it (Student 16, context 1).
I thought it was nice because then I got to go through the text several times and be completely sure that there were no mistakes. So that you don’t get any grammar mistakes. I would have chosen several drafts again if I could. I thought it was a good way to do it. When I’ve made a mistake, I get the opportunity to correct it (Student 2, context 1).
Many of the students said that the draft writing process with integrated feedback loops helped them to identify and correct mistakes and some explicitly said that it felt like “a safe way to write.” All the students who received and gave peer feedback (context 2) also described the three draft writing process as a good way to write. However, few of them explicitly pointed at the integrated feedback loops as an important aspect of the writing process. Some of them merely stated that they enjoyed getting two breaks during the process of writing, while others were more explicit about the value of the feedback: “If I had only had one draft then there would”t have been any change, and I would”t have known what was wrong - or what I could change”(Student 11, context 2). “I became more motivated to write, and then it was nice that we got breaks in between and didn’t need to be completely quiet. So, we could talk about the story. That was genuinely nice.” (Student 17, context 2). “Some words I have been spelling wrong for a long time, because I have been unsure how they are spelled. And now I got answers to some. So that helped me a lot.” (Student 24, context 2).
In this study we explore how lower secondary students from two feedback contexts (peer feedback and AI-generated feedback) experience the process of receiving-, discussing- and acting on language feedback (spelling, punctuation and grammar) during a three draft writing process. The writing trajectory was designed to embed formative feedback loops after the first and second draft, before the third (final) draft was submitted. One class from each of the three participant schools were assigned to the AI-generated feedback context while the other class from each school was assigned to the peer feedback context.
A common argument for implementing peer feedback and dialogic feedback interactions in classroom practices is that active participation in feedback processes could model feedback literacy for learners and thereby support students’ development of self-regulated learning (Andrade, 2010; Andrade et al., 2021). This argument rests on the premise that tacit feedback knowledge and feedback literacy might in some contexts be acquired and emerge through observation, imitation, participation and dialogue (Carless and Boud, 2018; Sutton, 2012). In our findings, we see a clear pattern where students who receive AI-feedback express to be more actively engaged in the group discussions than students who receive peer (generated) feedback. Students who received AI-generated feedback found the group discussions both useful and educational whilst the students who received peer feedback generally did not make use of the time set aside for group discussions. Factors embedded in the two feedback contexts could assist our understanding of this finding; Students assigned to the AI feedback context received generated and transformed feedback information (Huang et al., 2023; Munshi and Deneen, 2018) from a software technology they trusted. By pressing the feedback button, they received immediate feedback informed by the software who had acquired activity data about their draft. Students who received feedback from EAT were equipped with automated feedback (including numbers of errors) and examples from their own text before they were expected to discuss their feedback. This makes the feedback information available and understandable, or to use the words of Askew and Lodge (2000): They received “feedback as a gift” before they moved on to discussing the feedback (“feedback as collaborative work”).
Students assigned to the peer feedback context faced various challenges. As assessors they looked for language quality in their peers’ texts according to an assessment rubric, and some of them were more equipped to identify errors (and point at potential for improvement) than others. The peer assessors also had to actively generate feedback information in written mode and/or oral mode (Brookhart, 2008). Thus, these students were (in various degrees) cognitively and emotionally challenged in the assessor-role before they received feedback on their own texts from their peers (Panadero, 2016; Panadero et al., 2023). Panadero (2016) and Panadero et al. (2023) describe many inter- and intra-personal factors that could influence peer feedback processes, and quote examples in our result section illustrate at least two key aspects regarding the peer feedback context. The first aspect being: Understanding and realizing the inherent potential for engagement, and acquisition of tacit knowledge through modeling and comparisons can be demanding for 12–13 years old lower secondary students. One cannot take for granted that collaboration and active engagement will occur. The second aspect is: Feeling like a less competent student in a group of more competent fellow students could be a vulnerable situation. And when students are expected to be at both the giving and receiving end of feedback interactions at the same time, the cognitive overload added to the emotional stress could be overwhelming.
As evident in the result section, both groups of students were eager to get feedback which enabled them to correct their errors and improve their work. They all knew that their third draft (final version) of the text would be submitted to their teacher. The students also appreciated the ability to find and correct their mistakes during the three draft writing process, but the group of students who received AI- generated feedback seemed far more satisfied with the integrated feedback loops overall. The very intention of AI- generated and automated feedback technology is its ability to offer feedback that is both immediate and personalized, adapted to each student’s needs, providing targeted support that addresses specific areas of improvement (Barrot, 2023; Engeness and Mørch, 2016). This means that technology enhanced feedback should be easy to act on Huang et al. (2023), Munshi and Deneen (2018). All the interviewed students from the AI-generated feedback context agreed that they had acted upon the provided feedback by the best of their ability. Despite some examples of generic or misaligned responses (Barrot, 2023) in the AI-generated feedback, the students from context 1 trusted the feedback they received. This aligns with the view that AI- generated and automated feedback systems have the potential to increase reliability and validity of feedback, reduce the risk of bias and human mistakes, and that they could thus assist both student learning trajectories and teachers’ overall assessment practices (Chen et al., 2020; Richardson and Clesham, 2021).
Students from the peer feedback context did not trust the feedback they received in the same way as students from the AI- generated feedback context did. Some of them talked about peer feedback merely as differences in individual opinions, emphasizing that they would only use the received feedback if they believed it would somehow influence their grade. We also found examples of students experiencing that their peers did not take the feedback process seriously. According to Gielen et al. (2010) peer feedback is an assessment form performed by equal status learners. However, in our study we find many examples of students not perceiving themselves to be equal to the other students in their group. Some students note that they do not trust the peer feedback they receive from fellow students because they do not trust their level of competence. We also see examples of some students having experienced good support from what they call “the brightest students in class” in the receiver end of the feedback loop, whilst simultaneously do not feel that they are able to contribute as feedback givers the other way around. They express uncertainty about what aspects to provide feedback on and how to use feedback to improve their texts, making the situation challenging for them.
According to Carless and Boud (2018) developing feedback literacy involves appreciating feedback processes, making judgments, managing affect and taking action (acting), and their framework further supposes that maximizing the mutual interplay of first three features will maximize the potential for students to act on feedback provided. By comparing the two feedback contexts in our study, we have demonstrated that peer feedback processes and engaging in feedback through dialogic feedback interactions require a certain level of feedback literacy or at least supplementary support. Our study indicate that that AI-generated feedback and peer assessment could support lower secondary students’ development of feedback literacy in different ways: Students who receive AI-generated feedback information seem to be provided with a starting point for dialogic feedback interactions. The feedback information is easy to understand and could easily be compared. Our study also demonstrate that peer feedback is inherently complex for lower secondary school students, when they are both feedback givers and receivers during a feedback loop. Adding another layer of feedback mode (group discussions) during a three draft writing process seems to overwhelm the students, and especially students with limited feedback literacy. However, by rehearsing the processes of giving and receiving feedback – as provided in the peer feedback context, students must actively involve their judgment and practice how to manage affect. These elements could be key factors in strengthening students’ feedback literacy.
As noted in the results section the draft writing process with integrated feedback loops (both feedback contexts) was a new experience for the students. The study provides descriptive insights regarding how the students experienced the feedback process in the two contexts but should not be interpreted as a comparative study.
The datasets presented in this article are not readily available as participants did not give consent to share interview transcripts. Requests to access the datasets should be directed to SM, moltudal@hivolda.no.
The studies involving humans were approved by the Norwegian Agency for Shared Services in Education and Research (SIKT). The studies were conducted in accordance with the local legislation and institutional requirements. Written informed consent for participation in this study was provided by the participants’ legal guardians/next of kin. Written informed consent was obtained from the minor(s)’ legal guardian/next of kin for the publication of any potentially identifiable images or data included in this article.
SM: Formal Analysis, Writing – original draft, Writing – review and editing. SG: Data curation, Formal Analysis, Writing – review and editing. MS: Data curation, Writing – review and editing. IE: Data curation, Writing – review and editing.
The author(s) declare that financial support was received for the research and/or publication of this article. This is a sub-project of the AI4AfL-project (2022–2025) that is funded by The Research Council of Norway, Grant number 326607. Publication is funded by Volda University College.
The authors declare that the research was conducted in the absence of any commercial or financial relationships that could be construed as a potential conflict of interest.
The authors declare that no Generative AI was used in the creation of this manuscript.
All claims expressed in this article are solely those of the authors and do not necessarily represent those of their affiliated organizations, or those of the publisher, the editors and the reviewers. Any product that may be evaluated in this article, or claim that may be made by its manufacturer, is not guaranteed or endorsed by the publisher.
Anderson, T., and Shattuck, J. (2012). Design-based research: A decade of progress in education research? Educ. Res. 41, 16–25. doi: 10.3102/0013189X11428813
Andrade, H. (2010). “Students as the definitive source of formative assessment,” in Handbook of Formative Assessment, eds H. Andrade and G. Cizek (New York: Routledge), 90–105.
Andrade, H., Brookhart, S., and Yu, E. C. (2021). Classroom assessment as co-regulated learning: A systematic review. Front. Educ. 6:751168. doi: 10.3389/feduc.2021.751168
Askew, S., and Lodge, C. (2000). “Gifts, ping-pong and loops: Linking feedback and learning,” in Feedback for Learning, ed. S. Askew (London: Routledge), 1–17.
Baker, R. S. (2016). Stupid tutoring systems, intelligent humans. Int. J. Artificial Intell. Educ. 26, 600–614. doi: 10.1007/s40593-016-0105-0
Barrot, J. S. (2023). Using ChatGPT for second language writing: Pitfalls and potentials. Assessing Writing 57:100745. doi: 10.1016/j.asw.2023.100745
Black, P., and Wiliam, D. (1998a). Assessment and classroom learning. Assess. Educ. Principles Policy Pract. 5, 7–74. doi: 10.1080/0969595980050102
Black, P., and Wiliam, D. (1998b). Inside the black box. raising standards through classroom assessment. Phi Delta Kappan 80, 139–148.
Black, P., and Wiliam, D. (2009). Developing the theory of formative assessment. Educ. Assess. Eval. Accountability 21, 5–31.
Black, P., Harrison, C., Hodgen, J., Marshall, B., and Serret, N. (2011). Can teachers’ summative assessments produce dependable results and also enhance classroom learning? Assess. Educ. Principles Policy Pract. 18, 451–469. doi: 10.1080/0969594X.2011.557020
Boud, D., and Molloy, E. (2013). Rethinking models of feedback for learning: The challenge of design. Assess. Eval. High. Educ. 38, 698–712. doi: 10.1080/02602938.2012.691462
Brandmo, C., Panadero, E., and Hopfenbeck, T. (2020). Bridging classroom assessment and self-regulated learning. Assess. Educ. Principles Policy Pract. 27, 319–331. doi: 10.1080/0969594X.2020.1803589
Braun, V., and Clarke, V. (2006). Using thematic analysis in psychology. Qualitative Res. Psychol. 3, 77–101. doi: 10.1191/1478088706qp063oa
Brown, A. L. (1992). Design experiments: Theoretical and methodological challenges in creating complex interventions in classroom settings. J. Learn. Sci. 2, 141–178.
Carless, D., and Boud, D. (2018). The development of student feedback literacy: Enabling uptake of feedback. Assess. Eval. High. Educ. 43, 1315–1325. doi: 10.1080/02602938.2018.1463354
Chen, L., Chen, P., and Lin, Z. (2020). Artificial intelligence in education: A review. IEEE Access. 8, 75264–75278. doi: 10.1109/ACCESS.2020.2988510
Cho, K., and Schunn, C. D. (2007). Scaffolded writing and rewriting in the discipline. Comput. Educ. 48, 409–426.
Collins, A., Joseph, D., and Bielaczyc, K. (2004). Design research: Theoretical and methodological issues. J. Learn. Sci. 13, 15–42. doi: 10.1207/s15327809jls1301_2
Da Silva, J. (2021). Producing ‘good enough’ automated transcripts securely: Extending Bokhove and Downey (2018) to address security concerns. Methodol. Innov. 14, 1–11. doi: 10.1177/2059799120987766
Double, K. S., McGrane, J. A., and Hopfenbeck, T. N. (2020). The impact of peer assessment on academic performance: A meta-analysis of control group studies. Educ. Psychol. Rev. 32, 481–509. doi: 10.1007/s10648-019-09510-3
Engeness, I., and Mørch, A. (2016). Developing writing skills in english using content-specific computer-generated feedback with essaycritic. Nordic J. Digital Literacy 10, 118–135. doi: 10.18261/issn.1891-943x-2016-02-03
Fiskerstrand, P., and Gamlem, S. M. (2023). Instructional feedback to support self regulated writing in primary school. Front. Educ. 8:1232529. doi: 10.3389/feduc.2023.1232529
Gamlem, S. M., and Smith, K. (2013). Student perceptions of classroom feedback. Assess. Educ. Principles Policy Pract. 20, 150–169. doi: 10.1080/0969594X.2012.749212
Gamlem, S. M., Segaran, M., and Moltudal, S. (2024). Lower secondary school teachers’ arguments on the use of a 26-point grading scale and gender differences in use and perceptions. Assess. Educ. Principles Policy Pract. 31, 56–74. doi: 10.1080/0969594X.2024.2325365
Gehrke, P. J. (2018). “Ecological validity,” in The SAGE Encyclopedia of Educational Research, Measurement, and Evaluation, ed. B. B. Frey (Thousand Oaks, CA: SAGE Publications), 563–565.
Giannakos, M., Azevedo, R., Brusilovsky, P., Cukurova, M., Dimitriadis, Y., Hernandez-Leo, D., et al. (2024). The promise and challenges of generative AI in education. Behav. Information Technol. 1–27.
Gielen, S., Tops, L., Dochy, F., Onghena, P., and Smeets, S. (2010). A comparative study of peer and teacher feedback and of various peer feedback forms in a secondary school writing curriculum. Br. Educ. Res. J. 36, 143–162. doi: 10.1080/01411920902894070
Graham, S. (2018). “Instructional feedback in writing,” in The Cambridge Handbook of Instructional Feedback, eds A. A. Lipnevich and J. K. Smith (Cambridge: Cambridge University Press), 145–168. doi: 10.1017/9781316832134.009
Hopfenbeck, T. N., Zhang, Z., Sun, S., Robertson, P., and McGrane, J. A. (2023). Challenges and opportunities for classroom-based formative assessment and AI: A perspective article. Front. Educ. 8:1270700. doi: 10.3389/feduc.2023.1270700
Huang, W., Brown, G. T. L., and Stephens, J. M. (2023). “How technology assists the feedback process in a learning environment: A review,” in Proceedings of the 2023 IEEE International Conference on Advanced Learning Technologies (ICALT), (Piscataway, NJ), 326–328. doi: 10.1109/ICALT58122.2023.00101
Knight, S., and Buckingham Shum, S. (2017). “Theory and Learning Analytics,” in Handbook of Learning Analytics, eds C. Lang, G. Siemens, A. Wise, and D. Gasevic (Alberta: Society for Learning Analytics Research (SoLAR)), 17–22. doi: 10.18608/hla17.001
Kvale, S., and Brinkmann, S. (2009). InterViews: Learning the Craft of Qualitative Research Interviewing. Thousand Oaks, CA: Sage Publications.
Lipnevich, A. A., and Panadero, E. (2021). A review of feedback models and theories: Descriptions, definitions, and conclusions. Front. Educ. 6:720195. doi: 10.3389/feduc.2021.720195
Lui, A., and Andrade, H. (2022). The next black box of formative assessment: A model of the internal mechanisms of feedback processing. Front. Educ. 7:751548. doi: 10.3389/feduc.2022.751548
Liu, W. C., Wang, J. C. K., and Ryan, R. M. (2016). “Understanding motivation in education: Theoretical and practical considerations,” in Building Autonomous Learners, eds W. C. Liu, J. C. K. Wang, and R. M. Ryan (Singapore: Springer), 1–7.
Munshi, C., and Deneen, C. C. (2018). “Technology-enhanced feedback,” in The Cambridge Handbook of Instructional Feedback, eds J. K. Smith and A. A. Lipnevich (Cambridge: Cambridge University Press), 335–356. doi: 10.1017/9781316832134.017
Nicol, D. (2021). The power of internal feedback: Exploiting natural comparison processes. Assess. Eval. High. Educ. 46, 756–778. doi: 10.1080/02602938.2020.1823314
Panadero, E. (2016). “Is it safe? Social, interpersonal, and human effects of peer assessment: A review and future directions,” in Handbook of Human and Social Conditions in Assessment, eds G. T. L. Brown and L. R. Harris (Milton Park: Routledge), 247–266.
Panadero, E., Alqassab, M., Fernández Ruiz, J., and Ocampo, J. C. (2023). A systematic review on peer assessment: Intrapersonal and interpersonal factors. Assess. Eval. High. Educ. 48, 1053–1075. doi: 10.1080/02602938.2023.2164884
Pardo, A., Poquet, O., Martinez-Maldonado, R., and Dawson, S. (2017). “Provision of data-driven student feedback in LA & EDM,” in Handbook of Learning Analytics, eds C. Lang, G. Siemens, A. Wise, and D. Gasevic (Alberta: Society for Learning Analytics Research (SoLAR)), 163–174. doi: 10.18608/hla17.001
Pedder, D., and James, M. (2012). “Professional learning as a condition for assessment for learning,” in Assessment and Learning, 2nd Edn, ed. J. Gardner (London: SAGE), 33–48.
Richardson, M., and Clesham, R. (2021). Rise of the machines? The evolving role of AI technologies in high-stakes assessment. London Rev. Educ. 19, 1–13. doi: 10.14324/LRE.19.1.09
Sadler, D. R. (1989). Formative assessment and the design of instructional systems. Instructional Sci. 18, 119–144.
Saldaña, J. (2013). The Coding Manual for Qualitative Researchers. Thousand Oaks, CA: Sage Publications.
Shadiev, R., and Feng, Y. (2023). Using automated corrective feedback tools in language learning: A review study. Interactive Learn. Environ. 21, 2538–2566.
Sutton, P. (2012). Conceptualizing feedback literacy: Knowing, being, and acting. Innov. Educ. Teach. Int. 49, 31–40. doi: 10.1080/14703297.2012.647781
The Design-Based Research and Collective. (2003). Design- based research: An emerging paradigm for educational inquiry. Educ. Res. 32, 5–8. doi: 10.3102/0013189X032001005
van der Kleij, F. M., Adie, L. E., and Cumming, J. J. (2019). A meta-review of the student role in feedback. Int. J. Educ. Res. 98, 303–323. doi: 10.1016/j.ijer.2019.09.005
William, D., and Leahy, S. (2007). “A theoretical foundation for formative assessment,” in Formative Classroom Assessment. Theory into Practice, ed. J. McMillan (New York: Teachers College Press), 29–42.
Yang, M., Badger, R., and Yu, Z. (2006). A comparative study of peer and teacher feedback in a Chinese EFL writing class. J. Sec. Lang. Writing 15, 179–200.
Keywords: formative feedback, automated feedback, AI-feedback, peer feedback, feedback literacy, writing process
Citation: Moltudal SH, Gamlem SM, Segaran M and Engeness I (2025) Same assignment—two different feedback contexts: lower secondary students’ experiences with feedback during a three draft writing process. Front. Educ. 10:1509904. doi: 10.3389/feduc.2025.1509904
Received: 11 October 2024; Accepted: 17 March 2025;
Published: 31 March 2025.
Edited by:
Gavin T. L. Brown, The University of Auckland, New ZealandReviewed by:
Luqman Rababah, Jadara University, JordanCopyright © 2025 Moltudal, Gamlem, Segaran and Engeness. This is an open-access article distributed under the terms of the Creative Commons Attribution License (CC BY). The use, distribution or reproduction in other forums is permitted, provided the original author(s) and the copyright owner(s) are credited and that the original publication in this journal is cited, in accordance with accepted academic practice. No use, distribution or reproduction is permitted which does not comply with these terms.
*Correspondence: Synnøve Heggedal Moltudal, moltudal@hivolda.no
Disclaimer: All claims expressed in this article are solely those of the authors and do not necessarily represent those of their affiliated organizations, or those of the publisher, the editors and the reviewers. Any product that may be evaluated in this article or claim that may be made by its manufacturer is not guaranteed or endorsed by the publisher.
Research integrity at Frontiers
Learn more about the work of our research integrity team to safeguard the quality of each article we publish.