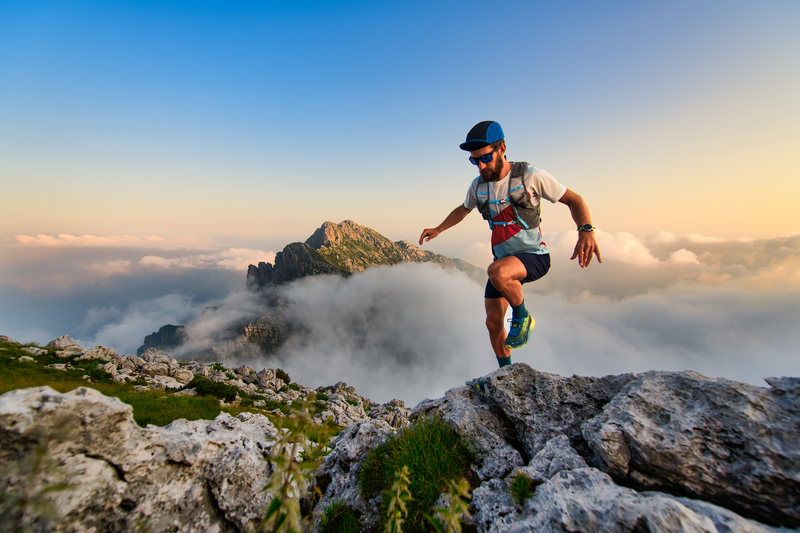
94% of researchers rate our articles as excellent or good
Learn more about the work of our research integrity team to safeguard the quality of each article we publish.
Find out more
ORIGINAL RESEARCH article
Front. Educ. , 26 March 2025
Sec. Digital Education
Volume 10 - 2025 | https://doi.org/10.3389/feduc.2025.1484904
Despite the recent increase in research on artificial intelligence in education (AIED), studies investigating the perspectives of academic staff and the implications for future-oriented teaching at higher education institutions remain scarce. This exploratory study provides initial insight into the perspectives of 112 academic staff by focusing on three aspects considered relevant for sustainable, future-oriented teaching in higher education in the age of AI: instructional design, domain specificity, and ethics. The results indicate that participants placed the greatest importance on AIED ethics. Furthermore, participants indicated a strong interest in (mandatory) professional development on AI and more comprehensive institutional support. Faculty who perceived AIED instructional design as important were more likely to use AI-based tools in their teaching practice. However, the perceived relevance of AIED domain specificity and ethics did not predict AI tool integration, which suggests an intention–behavior gap that warrants further investigation into factors such as AI literacy and structural conditions in higher education. The findings may serve as a basis for further discussion and development of adequate support services for higher education teaching and learning in the age of AI.
Given the rapid developments in the field of artificial intelligence (AI) in education (AIED), educators should be empowered to be “AI ready” (Chiu et al., 2024; Luckin et al., 2022). A prerequisite for this is to identify the perspectives of academic staff on aspects relevant for sustainable, future-oriented teaching in higher education in the age of AI. In this regard, the perspectives of academic staff on the use of AI are of great importance for the meaningful integration of AI into teaching and learning. However, research in this area is scarce. In contrast, numerous studies have been conducted lately to gain insight into students’ perceptions and utilization of AI in higher education, often with particular attention paid to the role of generative AI (Gašević et al., 2023; Hornberger et al., 2023; Johnston et al., 2024; von Garrel et al., 2023). Furthermore, different guidelines have been developed to serve as useful starting points for integrating AI into education (European Commission, 2022; Gimpel et al., 2023; Moorhouse et al., 2023; UNESCO, 2023), especially for novices. While guidelines may be regarded as providing a preliminary basis, successful and lasting digital transformation of higher education institutions requires systematic change management (McCarthy et al., 2023). It is therefore essential that the various perspectives and concerns of the multiple stakeholders involved (e.g., faculty, students, administration) be taken into account. Identification of the factors of acceptance, transparent communication, and professional development can facilitate the development of digital and AI literacy (Chiu et al., 2023; Ifenthaler and Yau, 2019; Pietsch and Mah, 2024; Redecker and Punie, 2017).
The field of AI literacy has emerged as an area that requires further exploration and development (Chiu et al., 2024; Knoth et al., 2024; Pinski and Benlian, 2023). This includes defining AI literacy (Long and Magerko, 2020; Ng et al., 2021) and delineating the roles of higher education institutions, lecturers, and students in acquiring digital and AI literacy. Indeed, the teaching and learning paradigms of the future in higher education call for a multitude of skills and perspectives. For instance, the capacity to provide instructions for teaching and learning and to integrate and adapt to new technological advances is necessary. In order to prepare students for the rigors and demands of their careers, it is crucial for universities to enable the acquisition of the necessary twenty-first-century skills (e.g., Redecker and Punie, 2017; Vuorikari et al., 2022). Hence, professional development is important for enhancing skills in emerging domains, such as technology and AI for teaching and learning. For example, Luckin et al. (2022) proposed an AI readiness training program in order to make educators AI ready. From an instructional design perspective, integrating educational technologies and AI-based tools to facilitate meaningful teaching and learning has become imperative. The technological pedagogical content knowledge (TPACK) framework (Mishra and Koehler, 2006) provides a comprehensive approach to understanding the interconnections between technology, pedagogy, and content. This framework aims to facilitate the effective integration of technology into teaching practices. Recently, discussions have emerged regarding the modification and extension of TPACK to incorporate AI components (e.g., including ethical considerations, impact of AI systems on one’s discipline leading to adaptation of content, didactical methods) and contextual knowledge (Brianza et al., 2024). Models such as Intelligent-TPACK (Celik, 2023) and AI-PACK (Lorenz and Romeike, 2023), as well as the discussion of the TPACK model by Mishra et al. (2023), represent an evolution of instructional design in response to the integration of AI.
A number of theoretical models have also been developed and applied with a focus on technology acceptance and use. Among the most prominent models in technology acceptance research are the Technology Acceptance Model (Davis et al., 1989) and the Unified Theory of Acceptance and Use of Technology (Kelly et al., 2023; Venkatesh et al., 2003). Both models suggest that actual technology use is influenced by an individual’s behavioral intention to use it. Furthermore, research indicates that perceived self-efficacy (Ajzen, 2002; Bandura, 1977) and proficiency in utilizing technologies affect the acceptance of AI and digital transformation and one’s intention to learn how to use AI (Ng et al., 2023; Sanusi et al., 2024; Wang et al., 2023). In addition, research has focused on general attitudes toward AI, particularly given the increasing integration of AI into everyday life (Schepman and Rodway, 2023; Sindermann et al., 2021). Despite the recent increase in research on AI in education, studies exploring the perspectives of academic staff remain limited. Furthermore, few studies have addressed the implications of recent advancements in AI for teaching in higher education, with a particular focus on instructional design considerations.
This paper presents an exploratory study designed to gain insights into the aspects of sustainable, future-oriented teaching that faculty consider relevant in higher education in the age of AI. Thus, our approach surveys the attitudes of academic staff toward important aspects of teaching related to the rapid developments and implications of AI in education. The findings may provide support for the implementation of effective change management strategies, such as the prioritization of professional development activities and curriculum development, and the integration of AI into higher education, focusing on instructional design, domain specificity, and ethics.
The paper is structured as follows: Section “2 Theoretical background” provides the theoretical background, giving an overview of AIED and focusing on the key perspectives of instructional design, domain specificity, and ethics (collectively referred to as AIED-IDDE). These perspectives inform the study’s three research questions. Section “3 Materials and methods” describes the methods, covering data collection, participant characteristics, the survey instrument, and instrument validation procedures. The results of the analyses addressing the research questions are presented in section “4 Results.” In section “5 Discussion”, we summarize and discuss the main findings and limitations of the study. Finally, section “6 Conclusion and future research” outlines implications and directions for future research.
Recently, research on AIED has increased, leading to growing discussion and adoption of AI in various educational practices (Chiu, 2023; Crompton and Burke, 2023; Grassini, 2023; Ouyang et al., 2022). One of the major drivers for the recent surge in research on AI in the field of education is rapid advancements in AI technology, including in generative AI. However, AI as a research field has existed for many years (Baker, 2000) and encompasses categories such as profiling and prediction, intelligent tutoring systems, assessment and evaluation, and adaptive systems and personalization (Bond et al., 2023; Celik et al., 2022; Crompton et al., 2022; Zawacki-Richter et al., 2019), and it includes prominent fields such as learning analytics (Ifenthaler, 2015; Márquez et al., 2023; Nouri et al., 2019; Siemens, 2013). Furthermore, the potential of AI to transform higher education has been increasingly recognized, with its main benefits including personalized learning, greater insight into student understanding, positive influences on learning outcomes, and reduced planning and administration time for educators (Bates et al., 2020; Bond et al., 2023). However, challenges such as a lack of ethical considerations, curriculum development, infrastructure, educators’ lack of technical knowledge, AI literacy, and the difficulty of integrating AI into educational systems remain (Bond et al., 2023; Ouyang et al., 2022; Southworth et al., 2023). Only a few studies have addressed these and related aspects from the perspective of academic staff. For instance, staff perceptions regarding the benefits and challenges of AI-based tools for teaching and learning (e.g., ethical considerations, curriculum development, AI literacy) may vary (optimistic, critical, critically reflected, and neutral) as indicated by a study that conducted latent profile analyses (Mah and Groß, 2024).
Understanding academic staff is crucial, as they play a vital role in preparing students for an increasingly digitalized world. In this regard, educational institutions (e.g., schools, higher education) should investigate how they can effectively integrate AI into the curriculum, both as a tool and as a subject of study (Southworth et al., 2023) and thereby empower students to navigate the digital landscape with competence. In this context, the concept of AI literacy (Long and Magerko, 2020; Ng et al., 2021) emerges as an area that warrants further exploration and development (Chiu et al., 2024; Walter, 2024). Long and Magerko (2020) define AI literacy as “a set of competencies that enable individuals to critically evaluate AI technologies, communicate and collaborate effectively with AI, and use AI as a tool online, at home, and in the workplace” (p. 2). However, forward-thinking higher education development includes not only defining AI literacy but also exploring how best to teach digital and AI literacy to students—as well as outlining the role of higher education institutions and faculty in the achievement of these competencies. Recent research on AI literacy has included the development of AI literacy assessment tools with a focus on self-assessment rather than knowledge-based or competency-based assessment (Carolus et al., 2023; Chiu, 2024; Knoth et al., 2024; Sperling et al., 2024; Wang et al., 2023). Indeed, the availability and use of valid AI literacy measurement tools is a fundamental prerequisite for the effective development of AI literacy. Knoth et al. (2024) distinguished between generic AI literacy (i.e., a basic understanding of AI that is domain-independent), domain-specific AI literacy (which refers to the specific field or discipline in which AI is implemented or used, such as education, medicine, or engineering), and AI ethics literacy (which focuses on the societal implications of AI). Following this, they developed a holistic AI literacy framework that encompasses these three forms of AI literacy and provides a matrix that can be used as a heuristic guide in the development of assessment and evaluation tools, or as a guide for constructive alignment (Biggs, 1996) in AI education. Following such an approach could address the domain-specific needs of different disciplines regarding AI literacy assessment and evaluation. However, in order to provide learners with opportunities to acquire AI literacy, including in a manner that respects domain specificity, educators need to become AI literate themselves.
With a focus on professional development in AI literacy, Laupichler et al. (2022), for example, discuss the importance of systematic reviews of AI courses tailored to educators. A general approach to AI literacy, such as the prominent course “Elements of AI” offered in Finland and many other countries, may provide a foundational introduction but may not suffice for the specialized requirements of higher education faculty. Regarding the education and training sector, Luckin et al. (2022) developed the EThICAL AI Readiness Framework encompassing seven steps: excite, tailor and hone, identify, collect, apply, learn, as well as iterate and iteration. The authors define AI readiness to be “an active, participatory training process and aims to empower people to be more able to leverage AI to meet their needs” (Luckin et al., 2022, p. 1). Such a form of AI readiness could then serve as a multiplier effect to transform higher education institutions toward systematic building of AI literacy. While some professional development courses are available, there is a need to explore more deeply the perspectives of faculty on their actual needs for competent integration of AI into teaching and learning practices in order to provide them with adequate and tailored support. For example, faculty members prefer online and digital self-paced formats for professional development in AI for teaching (Mah and Groß, 2024).
In light of the theoretical background outlined above, a more in-depth investigation of the instructional design implications of AI in education seems warranted, with a focus on the perspectives of academic staff in higher education. As previously indicated, recent studies on AIED have demonstrated the necessity for further research with regard to ethics (Bond et al., 2023; Celik, 2023; Laupichler et al., 2023; Nguyen et al., 2023), general instructional design (Celik, 2023; Deng and Zhang, 2023; Lorenz and Romeike, 2023; Mishra et al., 2023), and domain specificity (Long and Magerko, 2020; Ng et al., 2021; Schleiss et al., 2023). Following the demand to develop AI literacy for different professional domains (Almatrafi et al., 2024; Delcker et al., 2024; Knoth et al., 2024; Schleiss et al., 2023), this paper focuses on the domain of AI for teaching in higher education. In addition to domain-specific considerations, the generic aspects of teaching seen as a method (i.e., instructional design) and ethics are addressed. Hence, this section presents the three aspects that we intended to explore: AIED instructional design (AIED-ID), AIED domain specificity (AIED-D), and AIED ethics (AIED-E), collectively referred to as AIED-IDDE.
AIED instructional design (AIED-ID): Established instructional design models, such as Constructive Alignment (Biggs, 1996), the ADDIE model (analyze, design, develop, implement, and evaluate) for instructional design (Branch, 2009), and TPACK by Mishra and Koehler (2006), have played pivotal roles in planning lessons and guiding pedagogical strategies. It is noteworthy that discussions have recently emerged regarding the modification and extension of these models to incorporate AI components. Models such as AI-PACK (Lorenz and Romeike, 2023) and Celik’s (2023), as well as the discussion of the TPACK model itself (Mishra et al., 2023), represent the evolution of instructional design in response to the integration of AI. Furthermore, guides and templates have been developed to facilitate the integration of AI into educational practices, both as learning content and as a pedagogical tool (Schleiss et al., 2023). These developments emphasize the practical steps that institutions can take to harness the potential of AI for improved teaching and learning outcomes, which require further investigation and discussion (Abbas et al., 2024; Deng et al., 2024). Adapted strategies for teaching, learning, and assessment also require further discussion and investigation (Almatrafi et al., 2024; Hodges and Kirschner, 2024; Mao et al., 2023; Riegel, 2024). Thus, the AIED-ID was included as a factor in the survey.
AIED domain specificity (AIED-D): In current educational practice, there is a broad consensus on the importance of domain-specific knowledge, as the transmission of generic skills is not necessarily sufficient for holistic competence development (Tricot and Sweller, 2014). The central role of domain-specific aspects in teaching processes has been acknowledged since Shulman’s integrative concept of Pedagogical Content Knowledge (Shulman, 1986) and has continued to be a focus in the field of technology-based teaching and learning (see the TPACK model). The use of AI has profoundly impacted established practices in technology-based teaching and learning (Lorenz and Romeike, 2023), as the technical opportunities for teaching (and thus AI-related pedagogical knowledge) as well as content foundations (and thus AI-related content knowledge) are evolving. Given the diverse applications and adoption levels of AI in different subjects and fields, the latter can be considered highly domain-specific. Therefore, an analysis of AI-related learning processes in higher education should take into account domain-specific aspects such as potential use cases of AI in the domain, data in the domain, and implications of using AI in the domain (Schleiss et al., 2023). Because further research is necessary to explore the extent to which the perceived relevance of aspects of AIED and the use of AI in teaching vary among disciplines, AIED-D was included in the survey instrument.
AIED ethics (AIED-E): Studies show that ethical considerations in the use of AI in education are among the most challenging and important (Bond et al., 2023; Ifenthaler et al., 2024; Laupichler et al., 2023). The field of research surrounding ethical principles for AI in education is rapidly expanding (Knoth et al., 2024; Nguyen et al., 2023) to encompass a variety of discussions. Depending on the perspective, discussions on AI ethics in educational institutions revolve around issues such as privacy rights, academic integrity, questions of power, and the responsibility of learners in their studies as well as the cultivation of an open yet critical mindset about the impact of AI on society at large. The importance of ethical considerations is also reflected in the number of educational and political institutions that have already published guidelines and recommendations on ethical considerations regarding AI and data for teaching and learning, including those of the European Commission and UNESCO (European Commission, 2022; UNESCO, 2022). In Europe, the Artificial Intelligence Act, as the first international legislation on AI, aims to harmonize rules on AI. It uses a risk-based approach to develop safe and trustworthy AI systems in Europe and beyond, and it considers fundamental rights, safety, and ethical principles (European Council, 2024). AI systems identified as high risk include AI technology used in educational contexts, such as AI systems that could determine someone’s access to education (e.g., in scoring exams) (European Commission, 2024). Moreover, research indicates that the adaptation of pedagogical frameworks and instructional design models is necessary, particularly with regard to incorporating ethical considerations and assessment, including aspects such as the privacy, transparency, and data bias (e.g., considering individual differences such as race or gender) of AI-based tools (Celik, 2023; Deng and Zhang, 2023; Ng et al., 2024). Subsequently, as an aspect of potentially high relevance, AIED-E was included in the questionnaire.
Against this background, the paper examines the perspectives of academic staff on aspects they consider to be relevant for sustainable, future-oriented teaching in higher education in the context of the emergence of AI. Hence, our study addresses the following research questions:
RQ1a: What aspects of the use of AI for teaching and learning do academic staff consider to be important to deliver future-oriented teaching and learning in higher education with regard to AIED-ID, AIED-D, and AIED-E? RQ1b: To what extent does the perceived relevance of AIED and the usage of AI in teaching differ across disciplines?
RQ2: Which additional aspects do academic staff consider important for future-oriented teaching in higher education?
RQ3: To what extent does faculty members’ perceived relevance of AIED-ID, AIED-D, and AIED-E relate to their self-reported use (frequency) of AI-based tools in their teaching?
An online questionnaire (Sofware LimeSurvey) was designed to collect data from academic staff from various higher education institutions (e.g., universities and universities of applied sciences) throughout Germany and one Austrian university. Academic staff were invited to voluntarily participate in the study through higher education events on AI in education (i.e., online and face-to-face presentations/talks and teaching days) and newsletter announcements from digital teaching and learning communities from March to June 2024. Informed consent was obtained from participants prior to their participation. Confidentiality and anonymity of data processing were also assured. The stated disciplines of the participants included the humanities (31.25%), engineering (4.46%), mathematics/natural sciences (7.14%), medicine/health sciences (14.29%), fine arts (1.79%), law, economics and social sciences (25.89%), sports (1.79%), and others (10.71%); and 2.68% preferred not to answer. While the sample cannot be generalized to the higher education landscape of Germany and Austria, its diversity allows for perspectives beyond a single domain. A total of N = 131 academic staff participated in the survey; N = 112 reported having taught at an institution of higher education or university in the previous 6 months. As this condition was necessary for our research questions, this sample was the basis for all subsequent analyses (age: M = 41.10 years, SD = 10.62; gender: n = 69 female, n = 34 male, n = 9 missing indication of gender).
The online questionnaire consisted of two parts. The first covered the three aspects in terms of their relevance for future-oriented teaching and learning in higher education in the age of AI. To determine the aspect of (1) AIED Domain Specificity, items were adapted from Celik (2023) [example item: “Lecturers… know how to utilize domain-specific AI-based tools (e.g., intelligent tutor for math)”] (six items, ω = 0.905, M = 3.84, SD = 0.91). Items for (2) AIED Instructional Design (example item: “Lecturers… teach lessons that appropriately combine their teaching content, AI-based tools, and teaching strategies”) were adapted from Schmidt et al. (2009) and Celik (2023) (six items, ω = 0.908, M = 3.72, SD = 0.92). Items for (3) AIED Ethics (example item: “Lecturers… can analyze AI-based applications for their ethical implications for teaching and learning”) were adapted from Celik (2023), Laupichler et al. (2023), and Ng et al. (2022) (five items, ω = 0.913, M = 4.21, SD = 0.97). McDonald’s omega values indicated excellent reliability of the respective constructs (see Table 1). All items were rated on a 5-point Likert scale ranging from 1 (not at all relevant) to 5 (very relevant). Participants were also asked if there were other aspects that should be considered important in using AI for teaching and learning for sustainable, high-quality higher education (open-ended question). Importantly, as another variable of interest, respondents were asked to indicate how often they had used AI-based applications in/for their teaching in the last 6 months (e.g., inspiration for course plans, group exercises, translations, literature processing, personalization, image creation, AI as a learning object) to capture the actual implementation of AI in their teaching. The second part of the questionnaire consisted of socio-demographic characteristics, encompassing the related disciplines, type of institution, employment relationship, federal state, age and gender. All in all, the survey instrument was considered appropriate for the research questions posed in section “2.3 Research questions,” as we were able both to observe indications of the perceived importance of various aspects of AIED, as well as the actual implementation of AI in teaching, and to explore differences between faculties in a descriptive manner. The following section discusses the validity of the questions posed and the resulting constructs.
Table 1. Item wordings, means (M), standard deviations (SD), factor loadings (λ), and reliabilities (ω) of all included scales.
Before we could conduct our analyses, we needed to psychometrically validate our instrument, as the items were derived from several other scales, and some were newly developed. To validate the scales used in the present study to capture the constructs of AIED-ID, AIED-D, and AIED-E, we conducted confirmatory factor analysis (CFA) using the lavaan R package (Rosseel, 2012). As the instrument was designed with these three constructs in mind, comparable to established technological-didactic models such as TPACK, CFA was performed instead of exploratory factor analysis. The model fit indices chosen were χ2, CFI, RMSEA, and SRMR (Hu and Bentler, 1999; Kline, 2015). The loadings of each item on the proposed latent factor, as well as McDonald’s omega as a reliability measure, are reported in Table 1. Most loadings are strong, confirming that the observed variables represent the latent constructs well. The model has an acceptable fit based on CFI and SRMR; therefore, the results reported here are based on the proposed three-factor solution. However, the RMSEA of > 0.10 indicates a potential model misfit, suggesting that the instrument should be refined in future research: χ2 = 268.123, df = 116, CFI = 0.904, RMSEA = 0.108, and SRMR = 0.066. That noted, inadequate RMSEA values can also result from small sample sizes and low degrees of freedom within the model (Kenny et al., 2014). Five potential improvements in model fit were indicated by modification indices (MIs) that exceeded a threshold of 10. In CFA, MIs indicate how much a model’s fit could improve with certain changes, such as adding a path between variables. However, blindly applying MIs risks overfitting, which reduces the generalizability of the model. For example, MIs suggested that the third item on the AIED-ID scale may be more appropriate for the AIED-E scale, which showcases the difficulty in disentangling issues of AI responsibility and sensitivity in instructional design per se from general AI ethics education at a more fine-grained level. There are also several high covariances between items on the AIED-D scale, indicating that there are redundant items. Although the model fit was not optimal, we proceeded with our specified model because it best reflected our theoretical assumptions, and all items loaded substantially on their respective factors. In support of this decision, McDonald’s omega indicated excellent reliability for all scales (see Table 1). Still, a comparable CFA should be validated on a larger dataset, as the sample used in this study is hardly satisfactory for conducting a robust CFA.
Software R-Studio and the programming language R (v. 4.2.3) were used for data analysis. For RQ1, an ANOVA with post hoc t-tests was used. Differences between disciplines were explored descriptively, as the subgroups were not large enough for inferential statistics. RQ2 was approached through a qualitative analysis with the aim of identifying aspects that were not present in our survey. To analyze the open-ended responses in our questionnaire, we followed Mayring’s (2021) approach. Thus, we employed inductive category development, consolidating the statements in a two-step, low-inference process and ultimately assigning them to seven distinct categories. For RQ3, we conducted a linear regression, with the perceived importance of the three measured aspects as the predictor variables and the reported use of AI in teaching as the criterion.
Following the validation of our survey instrument, we analyzed the collected data in relation to our proposed research questions.
As RQ1a investigated which aspects of AI for teaching and learning academic staff consider most important for providing a future-oriented higher education in the age of AI, the three constructs of perceived relevance of AIED-ID, AIED-D, and AIED-E were compared using a within-subjects repeated measures ANOVA to test whether any construct received significantly higher relevance ratings. The means and standard deviations for each construct are presented in Table 3. Interestingly, some scales’ responses appeared to create ceiling effects, as 72.32% of participants responded with a mean score of between 4 and 5 on the AIED-E scale (M = 4.21, SD = 0.97; see also Figure 1).
Figure 1. Violin plots of the perceived relevance of AIED domain specificity, AIED instructional design, and AIED ethics.
Regarding the proposed within-subjects ANOVA, Mauchly’s test indicated that the assumption of sphericity had been violated, χ2(2) = 0.655, p < 0.001. Therefore, the degrees of freedom were corrected using Greenhouse-Geisser estimates of sphericity (ε = 0.744). The results of the repeated measures ANOVA with Greenhouse-Geisser correction showed a significant effect of scale type on perceived relevance scores, F(1.49, 165.10) = 34.08, p < 0.001, partial η2 = 0.23.
Following this initial effect, post hoc pairwise comparisons with Bonferroni correction for multiple comparisons revealed a significant directional ordering of the perceived relevance of the different constructs. The AIED-E construct (M = 4.21, SD = 0.97) was perceived as significantly more relevant than both the AIED-D construct (M = 3.84, SD = 0.91), t(111) = −5.06, p < 0.001, and the AIED-ID construct (M = 3.72, SD = 0.92), t(111) = −7.20, p < 0.001, which indicated AIED-E the aspect perceived as most relevant to future-oriented higher education. AIED-D was perceived as significantly more relevant than AIED-ID, t(111) = 3.15, p = 0.006, indicating that AIED education that respects domain specificity is perceived as more relevant than changing pedagogy and didactics through the use of AI. The differences between constructs are illustrated in Figure 1 via violin plots.
In order to gain a more fine-grained perspective, we proposed with RQ1b to conduct descriptive analyses of the differences between disciplines for all target variables regarding the perceived importance of aspects of AIED as well as actual AI use in teaching. The results obtained are highly exploratory in nature and not generalizable, as no balance of disciplinary backgrounds could be achieved within the data, and some subgroups are extremely small. Therefore, no inferential statistics were performed; instead, all means and standard deviations are presented as descriptive statistics in Table 2. Taking into account the descriptive values, several trends could be indicated that would need to be replicated with a more representative sample. Such trends could be a higher relevance of AIED-D for the arts, which could reflect the extensive implications of generative AI for image generation, or a lower perceived relevance of AIED-E for engineering, as this domain might have a more pragmatic and technical perspective on AI in education. In addition, it is interesting to note that the actual frequency of AI use in education was medium to low in all disciplines, while the perceived relevance of AI in education was largely high. This is discussed further in section “6 Conclusion and future research.”
Out of the sample of N = 112 participants, 26 provided insights regarding additional relevant aspects. From their open-ended responses, 32 individual statements were identified. Following Mayring’s (2021) approach to inductive category development, the statements were consolidated in a two-stage, low-inference process and ultimately assigned to categories.
The majority of mentions (n = 8) fell under the category of Knowledge & Training. The educators, for instance, deemed “regular mandatory training on current findings” (ID37), “practical workshops” (ID175), and the “development of personal competencies in handling AI” (ID190) necessary.
Another significant aspect was institutional support (n = 6). They considered “standardized guidelines provided by the university” (ID234) important and asked for “support for pioneers and multipliers” (ID61), a short-term “reduction of teaching assignments” to engage with AIED (ID82), and the establishment of a “community of practice for dealing with AI in higher education” (ID204).
The use of AI in exams and assessments was highlighted as a aspect relevant for future-oriented higher education (n = 4). Educators must be able to “navigate the possibilities and challenges of AI in the examination context with confidence” (ID66) and “critically evaluate their own teaching and examination concepts” (ID186).
The remaining mentions fell into the categories of Reflection, Application, Promotion of Student Competencies, and a miscellaneous category.
Our RQ3 examined whether faculty members’ subjective perceptions of the importance of these aspects were related to their self-reported frequency of actually integrating AI-based tools into their teaching.
To explore this research question in more detail, bivariate Pearson correlations were calculated for each variable of interest, revealing some substantial associations between variables (Table 3). In terms of RQ3, of particular interest is the positive correlation between the perceived importance of AIED-ID and the actual implementation of AI in teaching contexts, r(110) = 0.19, p = 0.047. Interestingly, the perceived importance of AIED-E did not correlate significantly with actual implementation of AI in the classroom, even though this aspect was rated the most important (see Table 3). This will be discussed in section “6 Conclusion and future research.” Another notable significant correlation was found between gender and the perceived importance of AIED-E, r(110) = −0.23, p = 0.022, indicating that females rated the perceived importance of AIED-E higher than males.
Table 3. Bivariate Pearson correlations between perceived relevance of AI for the future of teaching and learning, frequency of actual AI usage in teaching, and remaining demographic variables.
Following the calculation of the correlation matrix, a multiple linear regression was conducted to predict the use of AI in teaching, using participant ratings of the relevance of the three proposed AIED aspects as predictors. The regression model was not statistically significant, F(3, 108) = 1.70, p = 0.172, and explained only a small amount of the variance in AI usage in teaching, R2 = 0.045, Radj2 = 0.018. The intercept was significantly different from zero, b = 2.054, SE = 0.556, t = 3.693, p < 0.001. However, none of the predictors—AIED-ID (b = 0.428, SE = 0.301, t = 1.424, p = 0.157), AIED-D (b = −0.050, SE = 0.289, t = −0.172, p = 0.864), or AIED-E (b = −0.172, SE = 0.173, t = −0.996, p = 0.322)—significantly predicted the frequency of AI usage in the classroom. Notably, the predictor AIED-ID was significant when AIED-D was excluded from the model (see also the Pearson correlation in Table 3). This suggests that AIED-ID and AIED-D suffered from multicollinearity, which occurs when two or more independent variables in a regression model are highly correlated, making it difficult to determine their individual effects on the dependent variable. This is also supported by their intercorrelations (see Table 3), as well as by the fact that both predictors yielded a variance inflation factor (VIF) of around 5, indicating highly intercorrelated variables. Thus, these constructs may not actually represent two unique constructs, or the wording of the items and the operationalization of the constructs may have resulted in a methodological artifact. This is discussed further in section “6 Conclusion and future research.”
Despite the recent increase in research on AI in education, studies investigating the perspectives of academic staff and the implications of future-oriented teaching at higher education institutions remain scarce (Mah and Groß, 2024). This paper offers first insights into the perspectives of academic staff on the question of which aspects they consider important for sustainable, future-oriented teaching in the age of AI. In response to calls for the development of AI literacy in various professional domains (Almatrafi et al., 2024; Knoth et al., 2024; Schleiss et al., 2023), research on the modification of pedagogical models and instructional design considerations with regard to AI teaching (Celik, 2023; Mishra et al., 2023), as well as ethical aspects of using AI in education (Deng and Zhang, 2023; Nguyen et al., 2023), we intended to further explore AIED-ID, AIED-D, and AIED-E (acronym AIED-IDDE). The study employed an exploratory approach to examine the attitudes of lecturers toward the aspects perceived as relevant for teaching in higher education, with a focus on the emergence of AI. Overall, the study should be viewed within the context of twenty-first-century skills for teaching and learning. Digital competencies for teaching and learning are a prerequisite, and with corresponding digital competence frameworks, such as DigCompEdu (Redecker and Punie, 2017), they provide a basis for the adaptation of AI technologies for education (Ng et al., 2023).
The findings of the study indicate that participants assigned the greatest importance to ethical considerations related to AI in higher education and teaching, compared to AIED-ID and AIED-D considerations (RQ1a). This is consistent with research indicating that ethical considerations in the use of AI in education are among the most challenging and important (Bond et al., 2023; Ifenthaler et al., 2024; Laupichler et al., 2023). This result is also aligned with the discourse on the modification and extension of instructional design frameworks (e.g., TPACK), including ethical concerns and limitations (Celik, 2023; Deng and Zhang, 2023; Lorenz and Romeike, 2023). Therefore, it is prudent to employ practical examples such as the EThICAL AI Readiness Framework (Luckin et al., 2022) and the comprehensive AI policy education framework for university teaching and learning (Chan, 2023) to enhance AI literacy in higher education, which includes ethics. Moreover, higher education institutions should develop policies to support AI and data literacy among academic staff and students through curriculum development (Ifenthaler et al., 2024). We sought to examine the extent to which differences in the perceived relevance of our AIED aspects and AI usage in disciplines existed (RQ1b). However, our sample was imbalanced with respect to disciplines. Consequently, we conducted descriptive analyses and did not perform inferential statistics with significance tests.
Statements on additional aspects that faculty consider important for using AI for teaching and learning in future-oriented higher education (open-ended question, RQ2), included, for example, mandatory professional development for academic staff on the use of AI as well as institutional support and guidelines. In response to generative AI, numerous higher education institutions have had to develop guidelines, most encompassing three main areas: academic integrity, advice on assessment design, and communicating with students (Moorhouse et al., 2023). Professional development activities may be developed and offered in an interactive and participatory manner. A comprehensive approach that facilitates the overarching integration of AI within the curriculum for student programs—along with qualifying lecturers accordingly to meet the demands of future-oriented teaching and learning—also appears to be a promising avenue. The University of Florida, for instance, implemented a comprehensive framework for AI integration across the curriculum with the objective of transforming the higher education landscape through innovation in AI literacy (Southworth et al., 2023), and so have some K–12/16 curricula (Bellas et al., 2022; Chiu and Chai, 2020; Ng et al., 2022). Gašević et al. (2023) highlight the necessity of extensive collaboration between researchers, technology developers, and policymakers to assist educators in navigating the use of AI in education.
The present study also observed a positive correlation between AIED-ID and the actual implementation of AI in teaching (RQ3), which corresponds to similar relationships between attitudes and AI usage among first-year students (Delcker et al., 2024). This finding suggests that lecturers who perceive instructional design considerations regarding AI to be important are more likely to use AI-based tools in their teaching practice. Given that there has been an ongoing debate and interest in AI in education, individual educational institutions are developing and providing information sessions and courses on the topic (Luckin et al., 2022; Sperling et al., 2024). A focus is placed on the implications of AI for teaching, learning, and assessment. Thus, many academic staff are investigating the potential of AI-based tools for their own teaching practices, which recent studies on utilization have shown (Mah and Groß, 2024; Masley et al., 2024; Shaw et al., 2023). This is probably particularly the case for those who consider instructional design for teaching, including educational technologies, to be of high importance. This suggestion is in line with research showing that teachers’ participation in professional development was related to their teaching practices, e.g., regarding educational technologies (Fütterer et al., 2023; Konstantinidou and Scherer, 2022; Luckin et al., 2022).
However, AIED-D and AIED-E did not predict the actual use of AI in educational contexts, even though these aspects were rated as most important by the participants. This observation is corroborated by comparing the response distributions of the AIED-D and AIED-E scales, which both show left-skewed distributions, whereas the frequency of AI use shows a more right-skewed distribution. This may be indicative of the well-researched phenomenon of the “intention–behavior gap” (Sheeran and Webb, 2016). While the faculty perceived the integration of aspects of AI in education as very important, which likely shaped their intention to do so (An et al., 2023), very few participants actually integrate AI-based tools into their teaching on a regular basis. There are several possible explanations for this gap, including internal reasons like a lack of AI literacy on the part of the faculty themselves (Sperling et al., 2024), or external reasons, such as structural conditions that inhibit experimentation with and integration of AI into teaching contexts. Both point to important areas for future research.
Collaboration between researchers and educators would be helpful in refining and substantiating the constructs of AIED-ID, AIED-D, and AIED-E. As our analysis has shown, it is not entirely clear whether the proposed model is better fit with three or two factors. There are several indications that AIED-ID and AIED-D are not separable factors in our analyses. First, these factors yielded strong intercorrelations, further supported by substantial VIF values indicating multicollinearity. Furthermore, the inspected MIs suggest a common covariance of error between some instructional design and domain-specificity scale items. To put this in a theoretical context, respondents may have found it difficult to respond to the AIED-ID items at a “general” level that is independent of their own disciplinary background. After all, all the respondents were situated in a particular discipline from which they view and think about higher education issues, including AI. This argument could also be supported by the violin plots (see Figure 1), which show very similar response distributions for the two constructs. As the distinction between generic instructional design and those pedagogies that respect domain specificity is important in the context of professional development, future research should explore this aspect in more depth, hopefully leading to important insights that can guide improvement of such assessment instruments. One approach would be to conduct interviews with academic staff to determine where faculty differentiate instructional design from domain specificity.
In addition to conceptual and psychometric clarification of the AIED-ID and AIED-D factors, the AIED-E factor could also be refined, as, within the AIED-ID category, participants ranked the item “thinking critically about how to use AI-based tools in their classroom” as the most important aspect for future-oriented teaching in higher education in the age of AI. At the same time, this is the item with the lowest loading on the proposed factor (see Table 1). When examining the recommended MIs after CFA, it was suggested that this item might be better suited to the AIED-E factor. To clarify the distinctiveness and practical utility of the proposed constructs, future research including interviews with educators and other participatory methods may be helpful.
Following our discussion of implications for theory and educational practice, it should be noted that this study has limitations. The sample size of 131 academic staff, 112 of whom reported having taught in the previous 6 months, from higher education institutions was relatively small. Furthermore, there was an imbalance among the disciplines. Therefore, caution should be exercised in generalizing the findings beyond the current sample. The survey was promoted through newsletters and events on digital teaching/learning and AI in education. Therefore, the sample consisted of respondents who were already interested in the topic, and it may not reflect the general view of faculty on the integration of AI for future-oriented teaching in higher education. Thus, the data collected may suffer from self-selection bias, as faculty who are already interested in AI may be more likely to participate in such a survey. In addition, generalizable implications cannot be drawn from the differences between disciplines because these subgroups were unbalanced and too small. Nevertheless, they are presented descriptively for the sake of transparency and to stimulate other research questions. The small sample size may also have significantly affected the mixed goodness of fit of our proposed factorial structure of the survey instrument, leaving open whether the model is better fit with three or two factors. Finally, the assessment approach chosen here may have led to results that are difficult to interpret, which may also affect the factorial structure of the survey instrument. What the items were intended to capture were perceptions of what academic staff consider relevant aspects of AI in education for a future-oriented higher education system. This is neither an assessment of self-reported competencies in the respective areas nor an objective measure of what the most relevant aspects actually are. This form of operationalization could also have led to the observed skewness and non-normality of the responses, resulting in the intention–behavior gap, because on a practical level, there is a distinct difference between asking someone what they think is relevant and whether they think they are able to fulfill the purposes they deem relevant (i.e., self-efficacy). Nevertheless, these subjective perspectives of faculty allow insight into the needs of academic staff and can inform participatory design of curricula and the sustainable development of higher education systems, as these voices need to be recognized and represented.
The findings of this exploratory research provide valuable insights into the perspectives of academic staff regarding relevant aspects of future-oriented, sustainable teaching in higher education in the age of AI, particularly considering our proposed AIED-IDDE factors—instructional design considerations, domain specificity, and ethics—as these factors are considered critical in light of the rapid developments in the field of AI and its potential to transform higher education. As such, the results can serve as a preliminary basis for understanding the relative perceived importance of these issues among higher education faculty. While instructional design adaptations in the face of AI were perceived as somewhat important, domain-specific considerations were perceived as significantly more important. However, ethical considerations in the face of AI in teaching and learning were rated as the most important aspect to consider for future-oriented higher education. Interestingly, none of these aspects significantly predicted actual use of AI in teaching contexts, suggesting an intention–behavior gap in which faculty already consider certain aspects of AIED to be very important but may struggle to implement them in their own teaching commitments. These findings provide fruitful insights for the use of AI in higher education and for the development of appropriate professional development services, e.g., increasing AI literacy among faculty and changing structural conditions in higher education. First insights into concrete requirements for this were extracted from the participants’ free-text responses. Taking into account the findings of the present study as well as its limitations, several future research avenues can be outlined. First, similar surveys should be conducted with larger sample sizes, balanced disciplinary backgrounds, and in several countries. In this way, our preliminary findings could be replicated in a more robust manner, in particular by investigating whether there are currently systematic differences in AI tool integration between different domain disciplines and countries. Second, future research should take into account the AI literacy of respondents, as these competencies may serve as important mediators between the perceived importance of AIED aspects and intentions to use AI-based tools in teaching and the actual ability to fully integrate them. Third, in addition to further quantitative surveys and assessments, future research should consider using qualitative methods, such as interviews or focus groups. This could provide a more fine-grained picture of what faculty perceive as critical aspects of future-oriented higher education in the age of AI, while also considering the diverse disciplinary backgrounds and institutional cultures of the faculty. Such approaches should not only serve the purpose of clustering aspects of relevance but ideally also capture specific needs and requirements of academic staff to enable participatory design of future-oriented curricula that provide opportunities for adaptive teaching strategies, support targeted institutional support for faculty professional development, and thus enhance faculty’s ability to teach with didactic purpose and newfound capabilities of AI-based tools overall. Looking forward, it is essential to synthesize the perspectives of all relevant stakeholders (e.g., academic staff, students, policymakers, and researchers) in order to meaningfully and sustainably transform teaching and learning in higher education in the age of AI.
The raw data supporting the conclusions of this article will be made available by the authors, without undue reservation.
D-KM: Conceptualization, Investigation, Writing – original draft, Writing – review and editing. NK: Formal Analysis, Methodology, Writing – original draft, Writing – review and editing. ME: Writing – original draft, Writing – review and editing.
The authors declare that financial support was received for the research and/or publication of this article. This publication was funded by the German Research Foundation (DFG).
During the preparation of this work the authors used DeepL in order to improve readability and language of the work. After using the tool, the authors reviewed and edited the content as needed and take full responsibility for the content of the publication.
The authors declare that the research was conducted in the absence of any commercial or financial relationships that could be construed as a potential conflict of interest.
All claims expressed in this article are solely those of the authors and do not necessarily represent those of their affiliated organizations, or those of the publisher, the editors and the reviewers. Any product that may be evaluated in this article, or claim that may be made by its manufacturer, is not guaranteed or endorsed by the publisher.
Abbas, M., Jam, F. A., and Khan, T. I. (2024). Is it harmful or helpful? Examining the causes and consequences of generative AI usage among university students. Int. J. Educ. Technol. High. Educ. 21:10. doi: 10.1186/s41239-024-00444-7
Ajzen, I. (2002). Perceived behavioral control, self-efficacy, locus of control, and the theory of planned behavior. J. Appl. Soc. Psychol. 32, 665–683. doi: 10.1111/J.1559-1816.2002.TB00236.X
Almatrafi, O., Johri, A., and Lee, H. (2024). A systematic review of AI literacy conceptualization, constructs, and implementation and assessment efforts (2019–2023). Comput. Educ. Open 6:100173. doi: 10.1016/J.CAEO.2024.100173
An, X., Chai, C. S., Li, Y., Zhou, Y., Shen, X., Zheng, C., et al. (2023). Modeling English teachers’ behavioral intention to use artificial intelligence in middle schools. Educ. Information Technol. 28, 5187–5208. doi: 10.1007/S10639-022-11286-Z/FIGURES/2
Baker, M. (2000). The roles of models in artificial intelligence and education research: A prospective view. Int. J. Artificial Intell. Educ. 11, 122–143.
Bandura, A. (1977). Self-efficacy: Toward a unifying theory of behavioral change. Psychol. Rev. 84, 191–215. doi: 10.1037/0033-295X.84.2.191
Bates, T., Cobo, C., Mariño, O., and Wheeler, S. (2020). Can artificial intelligence transform higher education? Int. J. Educ. Technol. High. Educ. 17:42. doi: 10.1186/s41239-020-00218-x
Bellas, F., Guerreiro-Santalla, S., Naya, M., and Duro, R. J. (2022). AI curriculum for European high schools: An embedded intelligence approach. Int. J. Artificial Intell. Educ. 33, 399–426. doi: 10.1007/s40593-022-00315-0
Biggs, J. (1996). Enhancing teaching through constructive alignment. High. Educ. 32, 347–364. doi: 10.1007/BF00138871
Bond, M., Khosravi, H., De Laat, M., Bergdahl, N., Negrea, V., Oxley, E., et al. (2023). A meta systematic review of artificial intelligence in higher education: A call for increased ethics, collaboration, and rigour. Int. J. Educ. Technol. High. Educ. 21:4. doi: 10.1186/s41239-023-00436-z
Brianza, E., Schmid, M., Tondeur, J., and Petko, D. (2024). Is contextual knowledge a key component of expertise for teaching with technology? A systematic literature review. Comput. Educ. Open 7:100201. doi: 10.1016/J.CAEO.2024.100201
Carolus, A., Koch, M., Straka, S., Latoschik, M. E., and Wienrich, C. (2023). MAILS - meta AI literacy scale: Development and testing of an AI literacy questionnaire based on well-founded competency models and psychological change- and meta-competencies. Comput. Hum. Behav. Artificial Huma. 1:100014. doi: 10.48550/arXiv.2302.09319
Celik, I. (2023). Towards intelligent-TPACK: An empirical study on teachers’ professional knowledge to ethically integrate artificial intelligence (AI)-based tools into education. Comput. Hum. Behav. 138:107468. doi: 10.1016/j.chb.2022.107468
Celik, I., Dindar, M., Muukkonen, H., and Järvelä, S. (2022). The promises and challenges of artificial intelligence for teachers: A systematic review of research. TechTrends 66, 616–630. doi: 10.1007/s11528-022-00715-y
Chan, C. K. Y. (2023). A comprehensive AI policy education framework for university teaching and learning. Int. J. Educ. Technol. High. Educ. 20:38. doi: 10.1186/s41239-023-00408-3
Chiu, T. K. F. (2023). The impact of generative AI (GenAI) on practices, policies and research direction in education: A case of ChatGPT and Midjourney. Interactive Learn. Environ. 32, 6187–6203. doi: 10.1080/10494820.2023.2253861
Chiu, T. K. F. (2024). Future research recommendations for transforming higher education with generative AI. Comput. Educ. Artificial Intell. 6:100197. doi: 10.1016/j.caeai.2023.100197
Chiu, T. K. F., Ahmad, Z., Ismailov, M., and Sanusi, I. T. (2024). What are artificial intelligence literacy and competency? A comprehensive framework to support them. Comput. Educ. Open 6:100171. doi: 10.1016/J.CAEO.2024.100171
Chiu, T. K. F., and Chai, C. S. (2020). Sustainable curriculum planning for artificial intelligence education: A self-determination theory perspective. Sustainability 12:5568. doi: 10.3390/SU12145568
Chiu, T. K. F., Xia, Q., Zhou, X., Chai, C. S., and Cheng, M. (2023). Systematic literature review on opportunities, challenges, and future research recommendations of artificial intelligence in education. Comput. Educ. Artificial Intell. 4:100118. doi: 10.1016/j.caeai.2022.100118
Crompton, H., and Burke, D. (2023). Artificial intelligence in higher education: The state of the field. Int. J. Educ. Technol. High. Educ. 20:22. doi: 10.1186/s41239-023-00392-8
Crompton, H., Jones, M. V., and Burke, D. (2022). Affordances and challenges of artificial intelligence in K-12 education: A systematic review. J. Res. Technol. Educ. 56, 248–268. doi: 10.1080/15391523.2022.2121344
Davis, F. D., Bagozzi, R. P., and Warshaw, P. R. (1989). User acceptance of computer technology: A comparison of two theoretical models. Manag. Sci. 35, 982–1003. doi: 10.1287/MNSC.35.8.982
Delcker, J., Heil, J., Ifenthaler, D., Seufert, S., and Spirgi, L. (2024). First-year students AI-competence as a predictor for intended and de facto use of AI-tools for supporting learning processes in higher education. Int. J. Educ. Technol. High. Educ. 21, 1–13. doi: 10.1186/S41239-024-00452-7
Deng, G., and Zhang, J. (2023). Technological pedagogical content ethical knowledge (TPCEK): The development of an assessment instrument for pre-service teachers. Comput. Educ. 197:104740. doi: 10.1016/J.COMPEDU.2023.104740
Deng, R., Jiang, M., Yu, X., Lu, Y., and Liu, S. (2024). Does ChatGPT enhance student learning? A systematic review and meta-analysis of experimental studies. Comput. Educ. 227:105224. doi: 10.1016/J.COMPEDU.2024.105224
European Commission (2022). Ethical Guidelines on the Use of Artificial Intelligence (AI) and data in Teaching and Learning for Educators (P. O. of the European Union, Ed.). Brussels: European Commission, doi: 10.2766/127030
European Council (2024). Artificial Intelligence (AI) Act: Council Gives Final Green Light to the First Worldwide Rules on AI. Brussels: Council of the European Union.
Fütterer, T., Scherer, R., Scheiter, K., Stürmer, K., and Lachner, A. (2023). Will, skills, or conscientiousness: What predicts teachers’ intentions to participate in technology-related professional development? Comput. Educ. 198:104756. doi: 10.1016/J.COMPEDU.2023.104756
Gašević, D., Siemens, G., and Sadiq, S. (2023). Empowering learners for the age of artificial intelligence. Comput. Educ. Artificial Intell. 4:100130. doi: 10.1016/J.CAEAI.2023.100130
Gimpel, H., Hall, K., Decker, S., Eymann, T., Lämmermann, L., Mädche, A., et al. (2023). Unlocking the Power of Generative AI Models and Systems such as GPT-4 and ChatGPT for Higher Education: A Guide for Students and Lecturers. Stuttgart: University of Hohenheim.
Grassini, S. (2023). Shaping the future of education: Exploring the potential and consequences of AI and ChatGPT in educational settings. Educ. Sci. 13:692. doi: 10.3390/educsci13070692
Hodges, C. B., and Kirschner, P. A. (2024). Innovation of instructional design and assessment in the age of generative artificial intelligence. TechTrends 68, 195–199. doi: 10.1007/s11528-023-00926-x
Hornberger, M., Bewersdorff, A., and Nerdel, C. (2023). What do university students know about artificial intelligence? Development and validation of an AI literacy test. Comput. Educ. Artificial Intell. 5:100165. doi: 10.1016/J.CAEAI.2023.100165
Hu, L. T., and Bentler, P. M. (1999). Cutoff criteria for fit indexes in covariance structure analysis: Conventional criteria versus new alternatives. Struct. Equ. Modeling 6, 1–55. doi: 10.1080/10705519909540118
Ifenthaler, D. (2015). “Learning analytics,” in The SAGE Encyclopedia of Educational Technology, ed. J. M. Spector (Thousand Oaks, CA: SAGE Publications Inc), 448–451. doi: 10.4135/9781483346397.n187
Ifenthaler, D., and Yau, J. Y.-K. (2019). Higher education stakeholders’ views on learning analytics policy recommendations for supporting study success. Int. J. Learn. Anal. Artificial Intell. Educ. 1, 28–42. doi: 10.3991/IJAI.V1I1.10978
Ifenthaler, D., Majumdar, R., Gorissen, P., Judge, M., Mishra, S., Raffaghelli, J., et al. (2024). Artificial intelligence in education: Implications for policymakers, researchers, and practitioners. Technol. Knowledge Learn. 29, 1693–1710. doi: 10.1007/S10758-024-09747-0/TABLES/4
Johnston, H., Wells, R. F., Shanks, E. M., Boey, T., and Parsons, B. N. (2024). Student perspectives on the use of generative artificial intelligence technologies in higher education. Int. J. Educ. Integrity 20, 1–21. doi: 10.1007/S40979-024-00149-4/TABLES/10
Kelly, A., Sullivan, M., and McLaughlan, P. (2023). ChatGPT in higher education: Considerations for academic integrity and student learning. J. Appl. Learn. Teach. 6, 1–10. doi: 10.37074/jalt.2023.6.1.17
Kenny, D. A., Kaniskan, B., and McCoach, D. B. (2014). The performance of RMSEA in models with small degrees of freedom. 44, 486–507. doi: 10.1177/0049124114543236
Kline, R. B. (2015). Principles and Practice of Structural Equation Modeling, 4th Edn. New York, NY: Guilford Publications.
Knoth, N., Decker, M., Laupichler, M. C., Pinski, M., Buchholtz, N., Bata, K., et al. (2024). Developing a holistic AI literacy assessment matrix – Bridging generic, domain-specific, and ethical competencies. Comput. Educ. Open 6:100177. doi: 10.1016/J.CAEO.2024.100177
Konstantinidou, E., and Scherer, R. (2022). Teaching with technology: A large-scale, international, and multilevel study of the roles of teacher and school characteristics. Comput. Educ. 179:104424. doi: 10.1016/J.COMPEDU.2021.104424
Laupichler, M. C., Aster, A., and Raupach, T. (2023). Delphi study for the development and preliminary validation of an item set for the assessment of non-experts’ AI literacy. Comput. Educ. Artificial Intell. 4:100126. doi: 10.1016/J.CAEAI.2023.100126
Laupichler, M. C., Aster, A., Schirch, J., and Raupach, T. (2022). Artificial intelligence literacy in higher and adult education: A scoping literature review. Comput. Educ. Artificial Intell. 3:100101. doi: 10.1016/j.caeai.2022.100101
Long, D., and Magerko, B. (2020). “What is AI literacy? Competencies and design considerations,” in Proceedings of the 2020 CHI Conference on Human Factors in Computing Systems, (ACM), 1–16. doi: 10.1145/3313831.3376727
Lorenz, U., and Romeike, R. (2023). “What is AI-PACK? – outline of AI competencies for teaching with DPACK,” in SSEP 2023: Informatics in Schools. Beyond Bits and Bytes: Nurturing Informatics Intelligence in Education, eds J. P. Pellet and G. Parriaux (Berlin: Springer), 13–25. doi: 10.1007/978-3-031-44900-0_2
Luckin, R., Cukurova, M., Kent, C., and Du Boulay, B. (2022). Empowering educators to be AI-ready. Comput. Educ. Artificial Intell. 3:100076. doi: 10.1016/j.caeai.2022.100076
Mah, D.-K., and Groß, N. (2024). Artificial intelligence in higher education: Exploring faculty use, self-efficacy, distinct profiles, and professional development needs. Int. J. Educ. Technol. High. Educ. 21:58. doi: 10.1186/s41239-024-00490-1
Mao, J., Chen, B., and Liu, J. C. (2023). Generative artificial intelligence in education and its implications for assessment. TechTrends. 68, 58–66. doi: 10.1007/s11528-023-00911-4
Márquez, L., Henríquez, V., Chevreux, H., Scheihing, E., and Guerra, J. (2023). Adoption of learning analytics in higher education institutions: A systematic literature review. Br. J. Educ. Technol. 55, 439–459. doi: 10.1111/bjet.13385
Masley, N., Fattorini, L., Perrault, R., Parli, V., Reuel, A., Brynjolfsson, E., et al. (2024). The AI Index 2024 Annual Report. Stanford, CA: Stanford University.
Mayring, P. (2021). Qualitative Content Analysis: A Step-by-Step Guide. Thousand Oaks, CA: SAGE Publications.
McCarthy, A. M., Maor, D., McConney, A., and Cavanaugh, C. (2023). Digital transformation in education: Critical components for leaders of system change. Soc. Sci. Human. Open 8:100479. doi: 10.1016/j.ssaho.2023.100479
Mishra, P., and Koehler, M. J. (2006). Technological pedagogical content knowledge: A framework for teacher knowledge. Teach. Coll. Rec. Voice Scholarsh. Educ. 108, 1017–1054. doi: 10.1177/016146810610800610
Mishra, P., Warr, M., and Islam, R. (2023). TPACK in the age of ChatGPT and generative AI. J. Digit. Learn. Teach. Educ. 39, 235–251. doi: 10.1080/21532974.2023.2247480
Moorhouse, B. L., Yeo, M. A., and Wan, Y. (2023). Generative AI tools and assessment: Guidelines of the world’s top-ranking universities. Comput. Educ. Open 5:100151. doi: 10.1016/j.caeo.2023.100151
Ng, D. T. K., Leung, J. K. L., Chu, S. K. W., and Qiao, M. S. (2021). Conceptualizing AI literacy: An exploratory review. Comput. Educ. Artificial Intell. 2:100041. doi: 10.1016/j.caeai.2021.100041
Ng, D. T. K., Leung, J. K. L., Su, J., Ng, R. C. W., and Chu, S. K. W. (2023). Teachers’ AI digital competencies and twenty-first century skills in the post-pandemic world. Educ. Technol. Res. Dev. 71, 137–161. doi: 10.1007/s11423-023-10203-6
Ng, D. T. K., Leung, J. K. L., Su, M. J., Yim, I. H. Y., Qiao, M. S., and Chu, S. K. W. (2022). AI Literacy in K-16 Classrooms. Berlin: Springer International Publishing, doi: 10.1007/978-3-031-18880-0
Ng, D. T. K., Wu, W., Leung, J. K. L., Chiu, T. K. F., and Chu, S. K. W. (2024). Design and validation of the AI literacy questionnaire: The affective, behavioural, cognitive and ethical approach. Br. J. Educ. Technol. 55, 1082–1104. doi: 10.1111/BJET.13411
Nguyen, A., Ngo, H. N., Hong, Y., Dang, B., and Nguyen, B. P. T. (2023). Ethical principles for artificial intelligence in education. Educ. Information Technol. 28, 4221–4241. doi: 10.1007/S10639-022-11316-W/TABLES/1
Nouri, J., Ebner, M., Ifenthaler, D., Saqr, M., Malmberg, J., Khalil, M., et al. (2019). Efforts in Europe for data-driven improvement of education – A review of learning analytics research in seven countries. Int. J. Learn. Anal. Artificial Intell. Educ. 1, 8–27. doi: 10.3991/IJAI.V1I1.11053
Ouyang, F., Zheng, L., and Jiao, P. (2022). Artificial intelligence in online higher education: A systematic review of empirical research from 2011 to 2020. Educ. Information Technol. 27, 7893–7925. doi: 10.1007/s10639-022-10925-9
Pietsch, M., and Mah, D.-K. (2024). Leading the AI transformation in schools: It starts with a digital mindset. Educ. Technol. Res. Dev. doi: 10.1007/s11423-024-10439-w [Epub ahead of print].
Pinski, M., and Benlian, A. (2023). “AI literacy - towards measuring human competency in artificial intelligence,” in Proceedings of the 56th Hawaii International Conference on System Sciences, (AIS), 165–174.
Redecker, C., and Punie, Y. (2017). European Framework for the Digital Competence of Educators: DigCompEdu. Brussels: European Commission.
Riegel, C. (2024). “Leveraging online formative assessments within the evolving landscape of artificial intelligence in education,” in Assessment Analytics in Education, eds M. Sahin and D. Ifenthaler (Cham: Springer), 355–371. doi: 10.1007/978-3-031-56365-2_18
Rosseel, Y. (2012). lavaan: An R package for structural equation modeling. J. Stat. Softw. 48, 1–36. doi: 10.18637/JSS.V048.I02
Sanusi, I. T., Ayanwale, M. A., and Tolorunleke, A. E. (2024). Investigating pre-service teachers’ artificial intelligence perception from the perspective of planned behavior theory. Comput. Educ. Artificial Intell. 6:100202. doi: 10.1016/j.caeai.2024.100202
Schepman, A., and Rodway, P. (2023). The General Attitudes towards Artificial Intelligence Scale (GAAIS): Confirmatory validation and associations with personality, corporate distrust, and general trust. Int. J. Hum. Comput. Interaction 39, 2724–2741. doi: 10.1080/10447318.2022.2085400
Schleiss, J., Laupichler, M. C., Raupach, T., and Stober, S. (2023). AI course design planning framework: Developing domain-specific AI education courses. Educ. Sci. 13:954. doi: 10.3390/educsci13090954
Schmidt, D. A., Baran, E., Thompson, A. D., Mishra, P., Koehler, M. J., and Shin, T. S. (2009). Technological pedagogical content knowledge (TRACK): The development and validation of an assessment instrument for preservice teachers. J. Res. Technol. Educ. 42, 123–149. doi: 10.1080/15391523.2009.10782544
Shaw, C., Yuan, L., Brennan, D., Martin, S., Jason, N., Fox, K., et al. (2023). GenAI in Higher Education. Fall 2023 Update. Time for Class Study. Available online at: https://tytonpartners.com/app/uploads/2023/10/GenAI-IN-HIGHER-EDUCATION-FALL-2023-UPDATE-TIME-FOR-CLASS-STUDY.pdf (accessed February 13, 2024).
Sheeran, P., and Webb, T. L. (2016). The intention–behavior gap. Social and Pers. Psychol. Compass 10, 503–518. doi: 10.1111/SPC3.12265
Siemens, G. (2013). Learning analytics: The emergence of discipline. Am. Behav. Sci. 57, 1380–1400. doi: 10.1177/0002764213498851
Sindermann, C., Sha, P., Zhou, M., Wernicke, J., Schmitt, H. S., Li, M., et al. (2021). Assessing the attitude towards artificial intelligence: Introduction of a short measure in German, Chinese, and English language. Künstliche Intelligenz 35, 109–118. doi: 10.1007/s13218-020-00689-0
Southworth, J., Migliaccio, K., Glover, J., Glover, J., Reed, D., McCarty, C., et al. (2023). Developing a model for AI Across the curriculum: Transforming the higher education landscape via innovation in AI literacy. Comput. Educ. Artificial Intell. 4:100127. doi: 10.1016/j.caeai.2023.100127
Sperling, K., Stenberg, C.-J., McGrath, C., Åkerfeldt, A., Heintz, F., and Stenliden, L. (2024). In search of artificial intelligence (AI) literacy in teacher education: A scoping review. Comput. Educ. Open 6, 100169. doi: 10.1016/J.CAEO.2024.100169
Tricot, A., and Sweller, J. (2014). Domain-specific knowledge and why teaching generic skills does not work. Educ. Psychol. Rev. 26, 265–283. doi: 10.1007/S10648-013-9243-1
UNESCO (2023). Guidance for Generative AI in Education and Research. Paris: UNESCO, doi: 10.54675/EWZM9535
Venkatesh, V., Morris, M. G., Davis, G. B., and Davis, F. D. (2003). User acceptance of information technology: Toward a unified view. MIS Quarterly: Manag. Information Syst. 27, 425–478. doi: 10.2307/30036540
von Garrel, J., Mayer, J., and Mühlfeld, M. (2023). Künstliche Intelligenz im Studium. Eine quantitative Befragung von Studierenden zur Nutzung von ChatGPT & Co. Darmstadt: Hochschule Darmstadt, doi: 10.48444/h
Vuorikari, R., Kluzer, S., and Punie, Y. (2022). DigComp 2.2: The Digital Competence Framework for citizens - with New Examples of Knowledge, Skills and Attitudes. Luxembourg: European Union.
Walter, Y. (2024). Embracing the future of artificial intelligence in the classroom: The relevance of AI literacy, prompt engineering, and critical thinking in modern education. Int. J. Educ. Technol. High. Educ. 21:29. doi: 10.1186/S41239-024-00448-3/METRICS
Wang, B., Rau, P. L. P., and Yuan, T. (2023). Measuring user competence in using artificial intelligence: Validity and reliability of artificial intelligence literacy scale. Behav. Information Technol. 42, 1324–1337. doi: 10.1080/0144929X.2022.2072768
Keywords: artificial intelligence in higher education, academic staff perspective, instructional design, domain specificity, ethics, AI literacy
Citation: Mah D-K, Knoth N and Egloffstein M (2025) Perspectives of academic staff on artificial intelligence in higher education: exploring areas of relevance. Front. Educ. 10:1484904. doi: 10.3389/feduc.2025.1484904
Received: 22 August 2024; Accepted: 14 March 2025;
Published: 26 March 2025.
Edited by:
Tom Crick, Swansea University, United KingdomReviewed by:
Isaiah T. Awidi, University of Southern Queensland, AustraliaCopyright © 2025 Mah, Knoth and Egloffstein. This is an open-access article distributed under the terms of the Creative Commons Attribution License (CC BY). The use, distribution or reproduction in other forums is permitted, provided the original author(s) and the copyright owner(s) are credited and that the original publication in this journal is cited, in accordance with accepted academic practice. No use, distribution or reproduction is permitted which does not comply with these terms.
*Correspondence: Dana-Kristin Mah, ZGFuYS1rcmlzdGluLm1haEBsZXVwaGFuYS5kZQ==
Disclaimer: All claims expressed in this article are solely those of the authors and do not necessarily represent those of their affiliated organizations, or those of the publisher, the editors and the reviewers. Any product that may be evaluated in this article or claim that may be made by its manufacturer is not guaranteed or endorsed by the publisher.
Research integrity at Frontiers
Learn more about the work of our research integrity team to safeguard the quality of each article we publish.