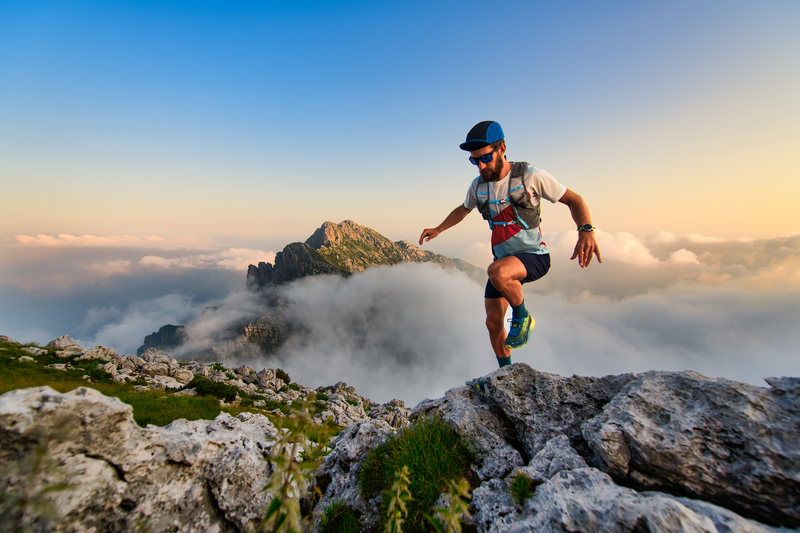
95% of researchers rate our articles as excellent or good
Learn more about the work of our research integrity team to safeguard the quality of each article we publish.
Find out more
ORIGINAL RESEARCH article
Front. Educ. , 19 March 2025
Sec. Digital Education
Volume 10 - 2025 | https://doi.org/10.3389/feduc.2025.1444544
This article is part of the Research Topic Promoting Innovative Learning-oriented Feedback Practice: Engagement, Challenges, and Solutions View all 7 articles
The growing integration of artificial intelligence (AI) in education has paved the way for innovative grading practices and assessment methods. This study uniquely explores GPT-4’s capabilities in handling essay-based exams in Norwegian across bachelor, master, and PhD levels, offering new insights into AI’s potential in educational assessment. Driven by the need to understand how AI can enhance assessment practices beyond traditional approaches, this case study research examines GPT-4’s performance on essay-based exams related to qualitative methods, case study research, qualitative meta-synthesis, and mixed method research, using chain-of-thought prompting. Unlike existing studies that primarily assess AI’s grading abilities, this research delves into GPT-4’s capability to both evaluate student responses and provide feedback, bridging a critical gap in integrating feedback theories with AI-assisted assessment. The study specifically investigates GPT-4’s ability to answer exam questions, grade student responses, and suggest improvements to those responses. A case study design was employed, with primary data sources derived from GPT-4’s performance on six exams, based on course learning goals and grading scale (feed up), GPT-4’s handling of main content in the exams (feedback), and GPT-4’s ability to critically assess its own performance and limitations (feed forward). The findings from this intrinsic case study revealed that GPT-4 performs well on these essay-based exams, effectively navigating different academic levels and the Norwegian language context. Fieldwork highlights GPT-4’s potential to significantly enhance formative assessment by providing timely, detailed, and personalized feedback that supports student learning. For summative assessment, GPT-4 demonstrated reliable evaluation of complex student essay exams, aligning closely with human assessments. The study advances understanding in the field by highlighting how AI can bridge gaps between traditional and AI-enhanced assessment methods, particularly in scaffolding formative and summative assessment practices. However, since this case study examines only the early phase of the intervention, it has several limitations. With an awareness of its limitations, the findings underscore the need for continuous innovation in educational assessment to prepare for future advancements in AI technology, while also addressing ethical considerations, such as bias. Vigilant and responsible implementation, along with ongoing refinement of AI tools, remains crucial.
The increasing integration of artificial intelligence (AI) in education presents a unique opportunity to improve assessment practices, but it also highlights a critical gap in how traditional feedback theories apply to AI-supported assessment. Established educational feedback theories—such as those proposed by Shute (2008), Wollenschläger et al. (2016), Wisniewski et al. (2020), and Hattie and Timperley (2007)—have laid valuable foundations for effective feedback processes. However, these frameworks were developed before the advent of advanced AI models and may not fully address the capabilities or limitations of AI in educational feedback.
This case study is positioned at the intersection of AI and educational assessment, aiming to explore GPT-4’s potential to enhance assessment by aligning with established feedback theories. There is currently a missing link in the literature regarding how these feedback theories can be integrated with AI-supported assessment in meaningful ways. Specifically, this study addresses this gap by examining GPT-4’s performance on essay-based exams in the Norwegian language, focusing on its ability to answer exam questions, grade student responses, and provide feedback for improvement. By investigating these dimensions, this research aims to establish a theoretical and practical foundation for integrating feedback theories with AI-supported assessment, bridging a crucial gap in current knowledge.
Building on prior research that demonstrated GPT-4’s capabilities with complex exams in fields such as medicine (Krumsvik, 2024a), nursing education (Krumsvik, 2024b), and doctoral education (Krumsvik, 2024c), this study further investigates how GPT-4 performs across six essay-based exams. The focus is on how GPT-4 provides feedback (through grading and suggestions for improvement) and feed forward (offering insights into potential enhancements in student responses). Drawing on our previous research on AI in education (Krumsvik et al., 2018, 2021; Krumsvik, 2023), this case study specifically examines GPT-4’s utility for both formative and summative assessment in Norwegian educational settings.
This study focuses on the following research questions:
1. What is GPT-4’s performance on the six exams (feed up)?
2. What is GPT-4’s capability of grading of six exams (feedback)?
3. What is the GPT-4’s capability to suggest measures of improvement on the six essay-based exams in the Norwegian language (feed forward)?
By addressing these questions, this case study seeks to illuminate GPT-4’s potential to redesign assessment practices, thereby bridging the gap between traditional feedback methods and AI-enhanced approaches and pinpointing areas for continued innovation in educational assessment.
The work on this article in 2022 led up to the launch of ChatGPT in November 2022 and GPT-4 in March 2023. In this context, the article “takes the temperature” on this technological paradigm shift as it appears in the fall of 2023 and spring of 2024, thematically focused on the journey between perceived affordances and real affordances (Norman, 1999) around AI in general and these language models specifically in higher education, highlighting the opportunities, challenges, dilemmas, and risks that emerge in this transitional period. Against this backdrop, we investigate GPT-4’s capability in light of the current state of knowledge:
What kind of contribution can AI make to education on a more general level?
Imran and Almusharraf (2023) conducted a systematic review of the literature to examine the role of ChatGPT as a writing assistant in higher education. The review found that ChatGPT has rapidly become a subject of debate among scholars and is being used by individuals across various fields. While opinions on the potential role of ChatGPT as a writing assistant vary, the study highlights its effectiveness in text generation, particularly for long essays and creative writing. It also emphasizes ChatGPT’s ability to produce human-like performance in various academic and professional tasks, suggesting its potential as a valuable tool in higher education writing. However, the review also identifies potential ethical concerns and challenges associated with the use of ChatGPT, underscoring the need for further research and careful implementation to ensure responsible and effective integration of this technology in educational settings.
Albadarin et al. (2024) conducted a systematic review of 14 empirical studies to investigate the use of ChatGPT in education. The review found that both learners and educators have utilized ChatGPT in various ways. Learners have used it as a virtual assistant for feedback, writing enhancement, and personalized learning, while educators have employed it for lesson planning, quiz generation, and resource provision. The study highlights the potential benefits of ChatGPT in education, such as improved learning outcomes and increased productivity. However, it also raises concerns about potential negative impacts on learners’ innovative capacities and collaborative skills, as well as ethical considerations and the need for structured training and guidelines for effective and responsible use.
Mai et al. (2024) conducted a systematic review titled “The use of ChatGPT in teaching and learning: a systematic review through SWOT analysis approach.” This review explores the integration of ChatGPT, an advanced AI-powered chatbot, into educational settings, highlighting the mixed reactions from educators. The review identified 32 topics related to ChatGPT’s use in education, categorized into 13 strengths, 10 weaknesses, five opportunities, and four threats and the findings were structured into three components: Presage, Process, and Product. In the Presage stage, the focus was on how ChatGPT adapts to diverse student characteristics and teaching contexts. In the Process stage, the impact of ChatGPT on teaching and learning activities was assessed, particularly its ability to provide personalized and adaptive instructional support. Finally, in the Product stage, the contribution of ChatGPT to student learning outcomes was evaluated. The authors conclude that by considering the application of ChatGPT in each stage of the teaching and learning process, educators can leverage its strengths and address its weaknesses to optimize its integration into educational practices.
Bhullar et al. (2024) conducted a comprehensive study titled “ChatGPT in higher education - a synthesis of the literature and a future research agenda,” and this study explores the impact of ChatGPT on higher education, highlighting key research articles, prominent journals, and leading countries in terms of citations and publications. The authors conducted a bibliometric analysis of 47 research papers from the Scopus database, identifying the United States as the most productive country in this field, with the highest volume of publications and citations. The study categorizes the findings into four thematic clusters: academic integrity, learning environment, student engagement, and scholarly research. These clusters represent the primary areas of focus in the research on ChatGPT in higher education, addressing critical issues such as student examinations, academic integrity, student learning, and field-specific research. A significant concern identified is plagiarism, as the use of ChatGPT may undermine students’ ability to produce original and creative work. This study provides valuable insights into the current state of ChatGPT in higher education literature, offering essential guidance for scholars, researchers, and policymakers on the integration and implications of ChatGPT in academic settings.
The systematic review, by Gao et al. (2024) investigates the use of text-based automatic assessment systems in post-secondary education, addressing the challenges of grading text-based questions in large courses. The review, screened 838 papers and synthesized 93 studies to explore the development and application of these systems in recent years. This review highlights the potential of AI and NLP, particularly Large Language Models like ChatGPT, to automate rapid assessment and feedback in higher education, offering valuable insights for researchers and educators integrating these technologies into their practices.
Guo et al. (2023) examined the extent to which ChatGPT’s responses resemble those of human experts, gathering 10,000 of comparison responses from both human experts and ChatGPT across various domains, including open-domain, finance, medicine, law, and psychology, in their article “How Close is ChatGPT to Human Experts?.” Their main finding is that “Compared with humans, we can imagine ChatGPT as a conservative team of experts. As a “team,” it may lack individuality but can offer a more comprehensive and neutral view toward questions” (p. 6).
Ray (2023) presents a comprehensive review titled “ChatGPT: a comprehensive review on background, applications, key challenges, bias, ethics, limitations and future scope,” which explores the transformative impact of ChatGPT in various fields through artificial intelligence (AI) and machine learning advancements. The review covers several critical aspects: The origins, development, and underlying technology of ChatGPT are examined, providing a detailed understanding of its foundation, the wide-ranging applications of ChatGPT across industries such as customer service, healthcare, and education are discussed, highlighting its versatility and utility. The paper identifies significant challenges faced by ChatGPT, including ethical concerns, data biases, and safety issues. It also discusses potential mitigation strategies to address these challenges. The review also examines various ethical issues and biases associated with ChatGPT, emphasizing the need to balance AI-assisted innovation with human expertise to ensure ethical use. The limitations of ChatGPT are acknowledged, and future research directions are suggested. These include integrating ChatGPT with other technologies, improving human-AI interaction, and addressing the digital divide. The paper explores how ChatGPT has been redefining scientific research, from data processing and hypothesis generation to collaboration and public outreach. Overall, Ray (2023) offers valuable insights for researchers, developers, and stakeholders, providing a thorough understanding of ChatGPT’s impact, challenges, and future potential in the ever-evolving landscape of AI-driven conversational agents. Despite ethical concerns and controversies, ChatGPT has garnered significant attention from academia, research, and industries in a short period.
The abovementioned current state of knowledge demonstrates that ChatGPT, GPT-4 and similar model and AI have a wide range of applications within education. However, there remains a missing link in the current state of knowledge regarding the integration of established feedback theories with AI-supported assessment and how capable GPT-4 is in languages as Norwegian in such feedback processes. This study seeks to address this gap by examining GPT-4’s performance on essay-based exams in the Norwegian language, focusing on its ability to answer exam questions, grade student responses, and provide feedback for improvement within educational practices.
This case study is exploratory and intrinsic (Stake, 1995, 2006), utilizing educational feedback theories by Shute (2007), Wollenschläger et al. (2016), Wisniewski et al. (2020), and especially Hattie and Timperley’s (2007) formative assessment model (Figure 1) with the concepts feed up, feedback and feed forward and the coherence between these regarding both the overall study, but also how these eclectic theories can be applied by GPT-4 itself within these assessment concepts.
Figure 1. Formative assessment model (Hattie and Timperley, 2007, p. 5).
Figure 1 illustrates the interconnected concepts of “feed up,” “feedback,” and “feed forward” and their role in bridging the theoretical foundations with the practical case study design of this research. Each concept is adapted to the context of GPT-4’s application, as follows:
1. Feed up: this concept addresses the question, “Where am I going?” It relates to the learning aims and objectives, specifically the descriptions of learning outcomes outlined in the course plan to which the exam belongs. In this study, feed up is represented by the alignment between the learning goals, the essay-based exam questions, and the criteria used to assess these goals. This ensures that GPT-4’s responses and evaluations are grounded in the intended learning outcomes of the course based on rubrics.
2. Feedback: this process addresses the question “How am I going?” and relates to the direct, criterion-based responses provided by GPT-4 when grading the six essay-based exams. In this context, feedback involves GPT-4’s capacity to evaluate student answers against established rubric criteria, offering detailed insights and assessments that align closely with human grading standards where possible. We will examine if GPT-4 is capable in delivering immediate, structured feedback, and if it can be a grading tool, capable of providing summative assessment that reflects the expectations of traditional evaluative practices.
3. Feed forward: this concept addresses “Where to go next?” Feed forward refers to GPT-4’s capacity, based on its initial grading (feedback), to suggest specific, actionable improvements in student responses. By indicating ways students can enhance their work, GPT-4 supports future learning processes and demonstrates its potential for formative assessment.
The theoretical foundation for this study draws on established feedback theories from Shute (2008), Wollenschläger et al. (2016), Wisniewski et al. (2020), and Hattie and Timperley (2007). These frameworks are used to guide the study’s approach to integrating GPT-4 into assessment practices, ensuring that its application is theoretically justified and pedagogically sound.
Another theoretical underpinning of the study is the concept of rubrics. In line with Wollenschläger et al. (2016), rubrics can be defined as structured scoring tools that outline specific criteria for evaluating the quality of student performance on various tasks. Rubrics provide a framework that breaks down complex assessment tasks into distinct, measurable components, each with clearly defined performance levels. This approach allows educators to assess student work consistently and transparently, supporting both formative and summative assessment by offering students clear expectations and actionable feedback.
Wollenschläger et al. (2016) emphasize that rubrics not only guide grading but also serve as learning tools, helping students understand the specific areas of strength and improvement in their work. By making assessment criteria explicit and transparent, rubrics facilitate a shared understanding between students and educators, which can enhance learning outcomes and support the development of targeted skills.
But how do these frameworks align with each other? Rubrics, as defined by Wollenschläger et al. (2016), provide a structured scoring tool that aligns assessment tasks with learning goals. This closely connects with the “feed up” concept in Hattie and Timperley’s model, as rubrics make learning expectations explicit and transparent, forming the foundation for both feedback and feed forward processes.
By aligning these frameworks, the study ensures theoretical coherence and pedagogical soundness. Rubrics serve as a practical tool to connect theoretical constructs with assessment practices, enabling GPT-4 to operationalize “feed up,” “feedback,” and “feed forward” in a systematic and measurable way. Furthermore, the exploratory nature of this study highlights the potential of GPT-4 to bridge these frameworks within both formative and summative assessment contexts.
In summary, this case study investigates whether GPT-4 can effectively integrate these three feedback processes and rubrics in essay exams and other educational contexts, offering both formative and summative assessment capabilities grounded in established educational frameworks.
This exploratory and intrinsic case study (Stake, 1995, 2006) investigates GPT-4’s potential for assessing Norwegian-language essay exams across various academic levels. GPT-4 was chosen due to its advanced language capabilities, making it uniquely suited to handle nuanced, open-ended questions that are characteristic of essay-based assessments. Furthermore, as GPT-4 has demonstrated proficiency in a range of academic tasks, its application to Norwegian essay exams offers a meaningful context to explore both its formative and summative assessment capabilities within a non-English language setting. This setting is particularly relevant for testing AI’s adaptability to diverse linguistic and cultural contexts, directly addressing the study’s research questions.
The data collection and analysis process were cumulative (Creswell and Guetterman, 2021), with three main phases. In the first phase, GPT-4’s performance on six essay-based exams was assessed using chain-of-thought prompting. Prompts included exact wording from the course plan, grading guidelines, and the Norwegian exam questions to ensure alignment with academic expectations. This methodology, based on Wollenschläger et al. (2016), involved using established rubrics to structure GPT-4’s responses according to educational standards and expectations.
The second phase focused on GPT-4’s grading capabilities, applying the rubric criteria consistently to evaluate the responses across the six exams. This phase was designed to test GPT-4’s accuracy and reliability in a grading role, examining its consistency with established academic grading practices in the Norwegian context. The third phase evaluated GPT-4’s ability to offer constructive feedback and feed forward by suggesting measures for improvement based on previous chain-of-thought prompting, again guided by Wollenschläger et al. (2016) rubrics.
For supplementary data collection, blue arrows in the study design represent additional steps that included integrating the research questions directly in (A) dialogue with GPT-4 to explore how it synthesized and responded to findings from phases 1, 2, and 3. Two external researchers then participated in a validation community to review preliminary findings and assess their validity (B). Lastly, additional fieldwork (C) was conducted to identify potential biases or misinterpretations in GPT-4’s responses, ensuring the findings were robust and grounded in current understanding of AI-supported assessment.
This methodological approach not only allowed for a comprehensive examination of GPT-4’s abilities across formative and summative assessments but also provided a framework to evaluate its performance in a specific language and educational context, directly addressing the study’s research questions.
The main test period was carried out from March 25, 2023–August 5, 2023 and the six essay-based exams set consisted of three essay-exam questions in ordinary exams on bachelor, and master level where the grading is from 1.0–6.0 (where 1.0 is the best). Next, the 3 essay-based exams on doctoral level (PhD) are more extensive and require an academic paper of 7–10 pages where the grading is “Approved” or “Not approved.” All the six essay-based exams were in the Norwegian language and consisted of only text. Scoring of all the six exams were based on the grading guidelines (“sensorveiledning”) based on the course plans. Interaction with GPT-4 was conducted based on a starting prompt (see attachment 1) and the six essay exam questions which were posed to GPT-4, and responses were recorded (each response was considered final). This was also based on Wollenschläger et al. (2016) rubrics-process.
Given the exploratory nature of this case study and the early stage of research into AI-assisted assessment in Norwegian educational settings, a purposive sampling approach was employed (Maxwell, 2009). This sampling method was selected to ensure the inclusion of a representative range of essay-based exams that could provide meaningful insights into GPT-4’s capabilities in handling academic tasks across multiple levels and subjects. The choice of purposive sampling was also influenced by GDPR requirements and research ethics, prioritizing data security and participant anonymity throughout the study.
The exams were selected from courses covering various academic levels (bachelor, master, and PhD) and focused on topics such as qualitative methods, case study research, qualitative meta-synthesis, and mixed-methods research. These subjects were chosen for their emphasis on critical thinking and written expression, characteristics that align well with the competencies required in essay-based exams. By selecting exams in these areas, the study could examine how effectively GPT-4 handles complex, open-ended questions in a language-specific (Norwegian) context, addressing the study’s core research questions.
Purposive sampling enabled the selection of exams that required high levels of analytical and interpretive skills, which are critical for testing the model’s capacity for formative and summative assessment. This approach ensured that the sample would yield valuable insights into GPT-4’s ability to align with educational objectives, provide coherent grading, and offer actionable feedback.
Data collection was conducted in three main phases, each aimed at assessing different aspects of GPT-4’s performance:
1. Answering exam questions: in the first phase, exam questions were entered into GPT-4 in a structured sequence, using chain-of-thought prompting to simulate how a student might approach answering the questions. GPT-4’s responses were recorded directly into a secure, GDPR-compliant data storage system to ensure data integrity and compliance with ethical standards.
2. Grading student responses: in the second phase, the same exam questions were paired with student responses, which GPT-4 was then prompted to grade according to the specific rubric criteria for each course. Each grading prompt was recorded, including GPT-4’s evaluative comments, scores, and any relevant observations. To comply with GDPR, no personal data from the students was included, and the grading outputs were stored securely.
3. Providing feedback and feed forward: in the third phase, GPT-4 was prompted to offer feedback and feed forward on student responses based on the rubric. This phase focused on identifying areas where students could improve and providing actionable suggestions for future performance. Feedback outputs were recorded in the same GDPR-compliant system, ensuring that all data were handled according to strict ethical guidelines.
The further digital field work from August 2023–March 2024 consisted of interactions with GPT-4 to check for possible biases and misinterpretations, in light of the abovementioned knowledge summary within this field and dialogues with Gemini Advanced (Google, 2024) as a validation of GPT-4’s performance. This process revealed that GPT-4 had improved in some areas, probably because of an ongoing fine tuning of GPT-4 by OpenAI.
Data analysis, step 1, was based on GPT-4’s performance on the six exams (feed up). Data analysis, step 2, was based on GPT-4’s ability of grading of the six exams (feedback) (one of the essay exam on PhD-level will be used as an example and “lens” regarding this phase 2 and feed up, feedback and feed forward). Data analysis, step 3, was based on 3, GPT’s exam-measures of improvement (feed forward). The supplemental data was collected from august 2023 – March 2024 and consisted of comprehensive interactions with GPT-4, the use of validation community and digital field work (described above).
The 3 essay-based exams on bachelor and master level were based on the University of Bergen’s grading scale (University of Bergen (UiB), 2018) as a letter by scale A, B, C, D, E, F. Below you find the general, qualitative descriptions of the criteria used in the assessment of the 3 essay-based exams.
For the 3 essay-based exams on doctoral level the grade was “Approved” or “Not Approved” based on the grading guidelines (“sensorveiledning”) based on the course plans. This was based on Wollenschläger et al. (2016) rubrics-process.
The validation community examined the GPT-4’s performance, feed up (1), grading abilities, feedback (2) and feed forward abilities (3), and found that GPT-4 handled these three areas very good. But they also mentioned the limitations with the few essay exams in the sample, etc. and several other concerns about the use of GPT-4 in student learning contexts (especially ethical issues, plagiarism, etc.). The dialogue with another LLM, Gemini Advanced (Google, 2024) as validation of GPT-4’s performance retrospectively during the autumn 2023 and spring 2024 confirmed strength and the weaknesses with this case study. Gemini Advanced also raised several limitations with the case study.
The “chain-of-thought prompting” methodology was employed as a structured approach to guide GPT-4 in providing coherent, contextually accurate responses across all phases of the study. This methodology involved presenting prompts that sequentially aligned with course objectives, grading criteria, and exam expectations, ensuring that GPT-4’s outputs were grounded in the academic standards relevant to each exam. By structuring prompts in a step-by-step manner (see Supplementary material), this approach supported GPT-4 in producing responses that demonstrated clear reasoning and alignment with educational expectations.
In the first phase, chain-of-thought prompting was applied to guide GPT-4 in answering exam questions accurately and in detail. Each prompt was constructed to follow a logical sequence that mirrored the structure of the course plan and exam expectations. For example, prompts included specific instructions that referenced the course’s learning outcomes and thematic focus areas. This helped ensure that GPT-4’s responses were relevant, comprehensive, and directly aligned with the knowledge and skills expected in the course. By consistently using structured prompts, the study aimed to mitigate response variability and maintain alignment with educational goals.
The second phase used chain-of-thought prompting to instruct GPT-4 on grading student responses according to established criteria. Here, prompts included detailed grading guidelines derived from the course rubrics, which specified various grading dimensions (e.g., comprehension, argumentation, coherence, and use of evidence). GPT-4 was sequentially guided through each rubric criterion, enabling it to evaluate responses systematically and produce grades that reflected the criteria comprehensively. This approach ensured consistency in grading by prompting GPT-4 to address each criterion in a step-by-step manner, closely following the course’s expectations for assessment.
In the third phase, chain-of-thought prompting was applied to enable GPT-4 to generate constructive feed forward for students. Prompts were designed to encourage GPT-4 to review the graded responses and suggest targeted areas for improvement. Each prompt focused on aspects identified in the grading phase that could be enhanced, such as argument clarity, depth of analysis, or alignment with course themes. GPT-4 was guided to offer actionable feed forward, helping students understand specific areas where they could improve their performance. This structured approach allowed GPT-4 to produce relevant, concrete feed forward that aligned with both the course objectives and grading criteria.
To ensure consistent application of the chain-of-thought prompting methodology across all phases, prompts were standardized and applied in a systematic order, with each prompt referencing established guidelines, rubrics, and course expectations. By maintaining this structured approach throughout the study, the methodology helped GPT-4 to provide responses, grades, and feedback that were coherent, accurate, and reflective of academic standards. This consistency was crucial in evaluating GPT-4’s capacity to handle both formative and summative assessment tasks within a structured framework.
In summary, the chain-of-thought prompting methodology enabled a cohesive approach to guiding GPT-4’s responses across various assessment functions. By using structured prompts that were consistently aligned with course expectations, this methodology supported the reliability of GPT-4’s outputs and allowed for a thorough exploration of its potential as an assessment tool in Norwegian-language essay exams.
However, there are some limitations we had to consider during this process. For one, GPT-4’s responses often benefit from prompts that break down complex exam questions into more manageable parts. This can enhance clarity but may also lead to fragmented grading insights that differ from holistic human evaluations. Additionally, while chain-of-thought prompting (COTP) helps structure GPT-4’s responses, it may sometimes produce overly precise, segmented feedback that lacks the broader context an educator might provide. Future applications could explore fine-tuning GPT-4’s prompts to balance detailed assessment with a more cohesive grading perspective.
Rubrics played a central role in guiding GPT-4’s performance across all phases of this study, providing a structured framework for assessment and feedback. Rubrics were adapted to suit the capabilities of GPT-4, ensuring clarity and alignment with the learning objectives and grading standards of the Norwegian essay exams. By structuring prompts and evaluations around these rubrics, the study aimed to foster consistency and reliability in GPT-4’s responses. Below is a description of how rubrics were specifically utilized and adapted in each phase.
In the initial phase, rubrics served as a reference for GPT-4’s responses to exam questions, providing a clear standard for the depth, scope, and focus expected in each answer. The rubric criteria were used to structure the chain-of-thought prompting, encouraging GPT-4 to answer each question in alignment with course objectives and expected outcomes. Key rubric elements, such as relevance, coherence, analytical depth, and integration of course themes, were integrated directly into the prompts. No major modifications were necessary in this phase, as the rubrics primarily guided GPT-4 in producing complete, well-structured answers to open-ended questions.
During the grading phase, rubrics were utilized in a criterion-based format to ensure that GPT-4’s grading aligned with the course’s established standards. Each rubric criterion—such as content accuracy, analytical depth, coherence, structure, and use of evidence—was broken down into specific prompts that instructed GPT-4 to assess each aspect of a student’s response. Modifications were made to adjust the level of detail in the rubric criteria, with additional descriptors provided for each grading level [e.g., the content of the grading scale (University of Bergen (UiB), 2018) as well as “excellent,” “good,” “satisfactory” on doctoral level] to ensure that GPT-4 could interpret the standards accurately.
To further aid GPT-4 in applying the rubrics effectively, examples and explanatory notes were added where necessary, helping the model recognize subtle distinctions between performance levels. These modifications aimed to bridge the gap between rubric language, which may be abstract (and in Norwegian), and GPT-4’s interpretive capabilities, ensuring that grading was consistent and aligned with academic expectations.
In the final phase, rubrics were used to guide GPT-4 in generating constructive feedback, or feed forward, to suggest specific improvements in student responses. The rubrics provided a structured framework for identifying areas where students could enhance their work, focusing on criteria such as argument clarity, depth of analysis, adherence to course themes, and overall coherence. Prompts based on rubric criteria guided GPT-4 to highlight specific areas for improvement, such as offering more in-depth analysis or better structuring their arguments.
To adapt rubric usage for this phase, prompts were rephrased to encourage constructive feedback rather than evaluative grading. Rubric criteria were reframed to guide GPT-4 in offering suggestions rather than assigning scores, emphasizing positive reinforcement and actionable advice. For instance, instead of simply indicating weaknesses in coherence or argument structure, GPT-4 was prompted to recommend practical ways for students to improve in these areas, aligning the feedback with formative assessment principles.
Throughout all phases, rubrics were applied consistently to ensure that GPT-4’s outputs were cohesive and aligned with the study’s objectives. By adapting rubrics to suit each phase’s unique requirements—whether for answering, grading, or providing feed forward—this approach enabled GPT-4 to operate within a structured, pedagogically sound framework. These adaptations might be crucial for maximizing GPT-4’s effectiveness as an assessment tool, allowing the model to produce outputs that met academic standards and supported student learning. However, it is important to be aware that rubrics can sometimes have a reductionist effect, being too narrow and goal-oriented. This is especially important to avoid in essay-based exams, which—unlike multiple-choice exams—are underpinned by a broader epistemology and require a more holistic approach. Another challenge is that certain distinctive Norwegian concepts in the rubrics may be difficult for GPT-4 to interpret and should be carefully considered in such processes.
There are several limitations when it comes to GPT-4’s grading of the exams. The grading was based on an alignment with the grading guidelines and grading scale specified for the exams. These guidelines provide criteria for evaluating the quality of responses, including accuracy, relevance, depth of understanding, and coherence. It is important to acknowledge some limitations in this approach. The alignment between GPT-4’s comments and the grading criteria does not necessarily ensure a comprehensive understanding of all nuances in the exam responses. And the evaluation process relies on the assumption that the grading scale fully captures the intended competencies, which may not account for all possible variances in performance. To address these limitations, we used a validity community of researchers and Gemini Advanced (Google, 2024) to mitigate biases and ensure better trustworthiness.
Throughout the data collection process, GDPR guidelines and research ethics were rigorously adhered to, particularly concerning the anonymization of data and secure storage of responses. Only exam questions and rubric criteria, which contained no personal identifiers, were used in the prompts to GPT-4, ensuring compliance with data protection regulations. All responses generated by GPT-4 were recorded in a secure environment, accessible only to authorized researchers involved in this study.
In summary, the data collection process was designed to ensure a secure, ethically sound approach to exploring GPT-4’s capabilities in educational assessment, using purposive sampling to provide a relevant sample of exam questions. This approach allowed the study to examine the practical applications of GPT-4 within the constraints of GDPR and research ethics, ensuring that the findings could inform further research in AI-supported assessment without compromising data security or participant confidentiality.
A key limitation of this study is that it represents an early phase of the research intervention, with findings based on a small sample of only six essay-based exams. This limited scope restricts the generalizability of the results, as a broader and more diverse sample across different subjects and academic levels would provide a more comprehensive assessment of GPT-4’s capabilities. Additionally, as an initial investigation and because of the GDPR, this study had no real students participating in the study (only the anonymized papers they had submitted). Thus, the study relies heavily on simulating the student role within these three feedback domains. This is, of course, a limitation, as we have not yet fully explored the potential challenges and complexities that may arise in real-world educational settings with actual students interacting directly with GPT-4, but this is particularly concerning GDPR and other ethical considerations (as AI can still be an “ethical minefield” for students in higher education given that directives, rules, and regulations are not yet fully established).
The results of the main data sources in the case study are presented below.
Data analysis, step 1, was based on GPT-4’s performance on the six exams (feed up) and the assessment of the GPT-4’s responses is based on the grading guidelines (“sensorveiledning”) for the different courses. Interaction with GPT-4: the six essay-based exams questions/assignments were posed to GPT-4 based on chain of thought prompting, and responses were analyzed (each response was considered final) (see Figure 2).
Figure 2. The research process of the intrinsic case study. The yellow arrows show the main data sources, and the blue arrows show the supplemental data in this article.
The GPT-4’s performance was six out of six exam questions correctly, which gives an accuracy overall rate of approximately 100% (96/110 * 100). On PSYK102, GPT-4 received A, on PSYK202 B, on Qual. method on master level A. On PhD-level the GPT-4 performed high on qualitative study research and mixed method research, and slightly above average on qualitative meta synthesis (Figure 3).
GPT-4’s good performance indicates a capability/understanding of the material covered in the essay-based exams. The GPT-4’s performance reflects insights and knowledge about the topic, and it is interesting that GPT-4 are capable to respond so good to Norwegian essay-exam questions (only few weeks after it was launched in March 2023). While the performance is strong, we should keep in mind that there are several limitations in the study.
The study focused on only six essay-based exams within a specific field (methodology). This limits the generalizability of the findings to other subjects or exam formats (e.g., multiple-choice, short answer).
1. Single iteration of responses: the study considered each GPT-4 response as final, without allowing for iterative improvement through re-prompting. This might not reflect how GPT-4 would be used in a real-world setting, where students might refine their work.
2. Potential bias in assessment: the assessment of GPT-4’s responses was based on the grading scale and grading guidelines (“sensorveiledning”). However, these scales and guidelines may not perfectly capture all nuances of good essay writing, and there could be some subjectivity in their application.
3. Chain-of-thought prompting: while chain-of-thought prompting was used, it’s possible that other prompting strategies might elicit different levels of performance from GPT-4. The study did not explore the impact of varying prompts on the results.
4. Lack of human benchmark: the study employed a validity community of two researchers, but did not directly compare GPT-4’s performance to that of human students on the same exams. This makes it difficult to definitively assess how GPT-4’s abilities stack up against those of real test-takers.
5. Evolving technology: GPT-4 is a rapidly evolving technology. The specific version used in the study might have different capabilities than later versions, limiting the long-term relevance of the findings.
However, the good results in section 3.1. are in line with the current state of knowledge internationally (e.g., Ray, 2023) and raises several questions about whether we can perceive GPT-4 as a reliable source and sparring partner in learning processes.
This grading of the six essay exams is based on the answer key/grading guidelines (“sensorveiledning”) for the bachelor-and master exams, and the guidelines for the academic paper on PhD-level. Interaction with GPT-4: the six essay-exams answers were posed to GPT-4 based on chain of thought prompting, and responses were recorded (each response was considered final). In all six essay exams answers GPT-4 had a good assessment performance and demonstrated sound judgment and a high degree of analytical abilities in the analysis of the essay texts. This may be partly due to the quality and specificity of the chain-of-thought prompting, but also because 15 of the essay texts were at the bachelor’s level, 4 at the master’s level, and 8 at the PhD level. But how did this grading unfold in the process? One of the essay exams will be used as an example of GPT’s grading ability (see below).
You are evaluating a PhD student’s mixed methods research paper. Assess the paper based on the following criteria, referencing specific examples from the text to support your evaluation:
1 Mixed methods design clarity and justification:
• Is the chosen mixed methods design (e.g., convergent, explanatory sequential, exploratory sequential, transformative) clearly stated and well-justified?
• Does the rationale for using a mixed methods approach align with the research questions and overall research goals?
• Are the strengths and limitations of the chosen design discussed in relation to the specific research context?
2 Philosophy of science and alignment:
• Are the philosophy of science (ontology, epistemology, axiology) underlying the mixed methods approach explicitly stated and discussed?
• Is there a clear connection between the philosophy of science foundations and the chosen research design and methods?
• Does the paper engage with relevant philosophy of science literature (e.g., Hesse-Biber et al., 2015) to support the discussion?
3 Research design model (Fetters et al., 2013):
• Are the main components of Fetters et al.’s (2013) model (or a similar framework) clearly identified and applied to the research design?
• Does the paper explain how each component (e.g., purpose, timing, weighting, mixing) is operationalized in the study?
• Are any adaptations or modifications to the model explained and justified?
4 Literature review and forefront of knowledge:
• Does the literature review demonstrate a comprehensive understanding of existing mixed methods research in the relevant field?
• Are the most relevant and up-to-date studies identified and discussed?
• Does the paper clearly articulate how the proposed research will build upon and extend the current state of knowledge?
5 Research questions (clarity, precision, justification):
• Are the research questions clearly stated, focused, and answerable using a mixed methods approach?
• Do the research questions align with the chosen mixed methods design and philosophical foundations?
• Are the research questions justified based on the literature review and theoretical framework?
• Do the research questions adhere to guidelines provided by Johnson and Christensen (2017) or other relevant sources?
6 Methodological discussion in synopsis:
• Does the synopsis (kappe) provide a more in-depth discussion of methodological considerations than the individual articles?
• Are the rationale, strengths, and limitations of the chosen methods thoroughly discussed?
• Is there a clear explanation of how the mixed methods approach will be implemented in each article of the thesis?
7 Data collection, analysis, and quality:
• Are the data collection procedures (including instruments and sampling strategies) for both quantitative and qualitative phases clearly described?
• Are the data analysis techniques appropriate for the types of data collected and the research questions?
• Are issues of data quality, validity, reliability, and trustworthiness addressed?
8 Quality assurance and alignment with assessment guidelines:
• Does the paper demonstrate a clear plan for ensuring the coherence and alignment of research questions, literature review, articles, and synopsis?
• Are the assessment guidelines for doctoral committees (e.g., Faculty’s guidelines) explicitly addressed?
• Are there any areas where the paper falls short of meeting these guidelines?
Overall assessment: based on your evaluation of the above criteria, provide an overall assessment of the PhD student’s mixed methods research paper. Highlight the strengths and weaknesses of the paper, and offer constructive feedback for improvement.
1 Mixed methods design clarity and justification
NN clearly identifies her research as an explanatory sequential mixed methods design (QUAN - > qual), where a quantitative survey will be followed by qualitative interviews to elaborate and clarify the survey results. The rationale for this choice is well-articulated, emphasizing the need to understand both what leadership competencies are important (quantitative) and how they are understood in context (qualitative). The paper effectively links this design to the complexity of the research problem, citing Fetters et al. (2013) to support the appropriateness of mixed methods for investigating complex phenomena in healthcare. However, the discussion of the design’s limitations is brief and could be expanded to address potential challenges in integrating the quantitative and qualitative phases.
2 Philosophy of science foundations and alignment
The paper demonstrates a good understanding of the philosophical underpinnings of mixed methods research, referencing pragmatism as the guiding philosophy. The discussion of post-positivism and constructivism is informative, but a more explicit link between these paradigms and the specific ontological, epistemological, and axiological assumptions of the research could strengthen the paper. While the references to Johnson and Christensen (2017) are relevant, incorporating additional philosophical literature (e.g., Greene, 2007) could provide a more nuanced discussion of the philosophical alignment.
3 Research design model (Fetters et al., 2013)
NN effectively utilizes Fetters et al.’s (2013) model to outline the main components of her mixed methods study. The discussion of purpose, timing, and building is particularly strong, clearly articulating how the quantitative survey will inform the subsequent qualitative interviews. However, the paper could delve deeper into the weighting aspect of the model, explaining the relative importance of the quantitative and qualitative phases in addressing the research questions.
4 Literature review and forefront of knowledge
The literature review provides a solid foundation for the research, demonstrating familiarity with key studies on health leadership competencies. The paper identifies a gap in the Norwegian context and positions the research as a valuable contribution to the field. However, the discussion of the forefront of knowledge could be strengthened by more explicitly identifying the specific ways in which this research will extend or challenge existing findings.
5 Research questions (clarity, precision, justification)
The overall research questions are clear and relevant to the study of health leadership competencies. However, the paper could benefit from articulating more specific sub-questions for each phase of the research (quantitative and qualitative). These sub-questions would help guide the data collection and analysis processes and ensure alignment with the overall research goals. Additionally, while the questions are justified based on the literature review, a stronger connection to the theoretical framework and philosophical foundations could enhance their clarity and precision.
6 Methodological discussion in synopsis
The paper acknowledges the importance of discussing methodological considerations in the synopsis (kappe) but does not provide specific details on how this will be done. A more elaborate discussion of the rationale for the chosen methods, potential challenges, and strategies for addressing them would be beneficial. This would demonstrate a deeper understanding of the methodological complexities of mixed methods research.
7 Data collection, analysis, and quality
The paper outlines the data collection procedures for the survey and interviews, including the use of a translated and adapted competency assessment tool. However, more details on the sampling strategy, sample size, and data analysis techniques would be helpful. Additionally, a discussion of potential biases or limitations in the data collection and analysis processes would demonstrate a critical awareness of the challenges inherent in mixed methods research.
8 Quality assurance and alignment with assessment guidelines
The paper briefly mentions the importance of quality assurance and alignment with assessment guidelines but does not provide a detailed plan for achieving this. A more comprehensive discussion of how the researcher and supervisors will ensure coherence between the research questions, literature review, articles, and synopsis would be valuable. This could include specific strategies for addressing feedback from supervisors, annual progress reports, and the doctoral committee.
Overall assessment: NN’s paper demonstrates a good understanding of mixed methods research principles and a clear rationale for using a mixed methods approach to study health leadership competencies. The research design is well-structured and aligns with the research questions. However, the paper could be strengthened by providing more specific details on the research questions, methodological considerations, data analysis techniques, and quality assurance procedures. Additionally, a more in-depth discussion of the philosophy of science foundations and their alignment with the research design would enhance the paper’s theoretical rigor. Overall, this paper provides a solid foundation for a mixed methods doctoral thesis and, with some refinements, has the potential to make a significant contribution to the field of health leadership research (GPT-4, OpenAI, 2023).
NN, your paper demonstrates a solid understanding of mixed methods research (MMR) and a clear rationale for its application to your research on health leadership competencies. To further strengthen your work and align it more closely with the assessment criteria, consider the following feedback, drawing on key assessment theories:
1 Task-specific feedback:
• Research questions: refine your research questions to be more specific and measurable for both the quantitative and qualitative phases. Consider how each question will be addressed by your chosen methods.
• Methodological discussion: expand the methodological discussion in your synopsis to include a more detailed rationale for your chosen methods, potential challenges, and mitigation strategies.
• Data analysis: elaborate on the specific data analysis techniques you will use for both quantitative and qualitative data, ensuring they align with your research questions.
2 Process-oriented feedback:
• Philosophy of science foundations: deepen your discussion of the philosophy of science underpinnings of your research by explicitly linking your ontological, epistemological, and axiological assumptions to your chosen mixed methods design.
• Integration: provide a more detailed plan for integrating your quantitative and qualitative findings. Consider using a joint display to visually represent the convergence or divergence of your results.
3 Feed-Up:
• Literature review: while your literature review is comprehensive, consider incorporating more recent studies on mixed methods research in health leadership to demonstrate your engagement with the latest developments in the field.
• Theoretical framework: strengthen the connection between your theoretical framework and your research questions. Ensure that your framework explicitly guides your methodological choices and data analysis.
4 Feedback:
• Quality assurance: develop a more detailed plan for quality assurance, outlining specific steps you and your supervisors will take to ensure coherence and alignment between your research components.
• Assessment guidelines: explicitly address the assessment guidelines for doctoral committees in your paper, demonstrating your awareness of and commitment to meeting these standards.
5 Feedback on self-regulation:
• Reflective practice: engage in regular reflective practice throughout your research process. Document your reflections on methodological choices, challenges encountered, and lessons learned. This will help you refine your approach and make informed decisions.
• Goal setting: set clear, achievable goals for each phase of your research. Regularly review and adjust these goals as needed to ensure you stay on track and make progress toward your overall research objectives.
6 Feedback to close the gap:
• Integration: focus on strategies for integrating your quantitative and qualitative findings to achieve a deeper understanding of the research problem. Consider using a weaving or merging approach to combine your data sources.
• Validity and trustworthiness: elaborate on the specific techniques you will use to ensure the validity and trustworthiness of your mixed methods study. Discuss how you will address potential biases and limitations in your data collection and analysis.
By incorporating this feedback into your revisions, you can further enhance the rigor, clarity, and impact of your mixed methods research paper. Remember, feedback is a valuable tool for growth and improvement, so embrace it as an opportunity to refine your work and achieve your research goals.
Overall assessment: Based on the assessment of NN’s mixed methods research paper, I would grade it as a B (Very good) and “Approved.”1
The paper demonstrates a very good performance in most areas, showcasing a sound understanding of mixed methods research principles and a well-structured research design. The rationale for using a mixed methods approach is clearly articulated, and the chosen design aligns with the research questions. The literature review is comprehensive, and the paper identifies a relevant gap in the existing knowledge base.
While the paper demonstrates a high degree of independent thinking in conceptualizing the research and choosing an appropriate methodology, there are some areas where the depth and clarity could be improved:
• Research questions: while the overall research questions are clear, the paper would benefit from articulating more specific sub-questions for each research phase.
• Methodological discussion: the synopsis could provide a more in-depth discussion of the rationale for the chosen methods, potential challenges, and mitigation strategies.
• Data analysis: more specific details on the data analysis techniques for both quantitative and qualitative data would strengthen the paper.
• Quality assurance: a more comprehensive plan for ensuring alignment with assessment guidelines and maintaining quality throughout the research process would be beneficial.
Despite these areas for improvement, the paper’s overall quality and potential contribution to the field of health leadership research warrant a B grade. With some refinements, this paper has the potential to be an excellent piece of scholarship (GPT-4, OpenAI, 2023).
One key focus of this study was to evaluate GPT-4’s performance in Norwegian, a language with a relatively small number of speakers globally and presents unique challenges in terms of vocabulary, syntax, and context. Although GPT-4 has been trained on a large, multilingual dataset, its proficiency can vary across languages, potentially impacting the accuracy and cultural relevance of its responses. Norwegian is characterized by specific linguistic structures and idiomatic expressions that differ significantly from English, and these nuances may affect GPT-4’s interpretive capabilities, particularly in essay-based exams that demand high levels of comprehension and context sensitivity. Norwegian is based on two official written forms, Bokmål and Nynorsk, both used in everyday life, education, media, and official documents. In addition, Norway recognizes three minority languages: Sami, Kven, and Romani. These minority languages have official status in certain regions and are protected to support cultural and linguistic diversity.
This study used only Norwegian Bokmål and observed that while GPT-4 performed well in grading and feedback in Norwegian, certain language-specific challenges did arise, such as difficulties in interpreting regional expressions or specialized terminology within academic writing. Addressing this limitation will be essential as AI applications in education expand into more diverse linguistic contexts. Future iterations of language models could benefit from more targeted training on Norwegian academic texts, ensuring that models like GPT-4 can better capture the nuances of the language.2 Additionally, further research is warranted to explore how AI can be adapted to support a range of languages in educational assessment, enhancing both the model’s applicability and reliability.
GPT-4 was selected for this study due to its advanced language processing capabilities, including handling open-ended, complex questions that require critical thinking and detailed analysis. While alternative AI models, such as XLNet, BERT, T5, or earlier versions of GPT, (as well as Gemini Advanced and Claude) have shown strengths in certain areas of language processing, GPT-4’s enhanced ability to generate coherent, contextually aware responses makes it particularly suitable for essay-based exams that assess higher-order thinking skills.
However, it is important to consider that other models might yield different insights, particularly in cases where simpler, factual assessments or multiple-choice formats are involved. For example, models like XLNet, BERT, with its focus on sentence embeddings, could provide efficient performance in recognizing patterns or classifying responses but may lack the depth and coherence required for open-ended tasks. Exploring these models in future studies could offer a comparative analysis, assessing whether simpler models might achieve comparable results in specific educational contexts. In the context of this study, however, GPT-4’s advanced processing abilities were deemed essential for handling the complex demands of Norwegian essay exams.
This study was limited to six essay-based exams in Norwegian, focusing specifically on qualitative and methodological subjects across bachelor, master, and PhD levels. While this scope allowed for an in-depth exploration of GPT-4’s performance in a structured, culturally specific context, it also limits the generalizability of the findings. The small sample size and narrow focus may not fully represent GPT-4’s capabilities across diverse subjects, languages, or exam formats, potentially restricting the broader applicability of the results. Future research should expand the sample to include exams from various academic fields and languages, allowing for a more comprehensive understanding of GPT-4’s performance in different educational settings.
We included a validity check involving two researchers (validity community) in this study; however, this did not provide the same level of insight as a full-scale human benchmark. A more thorough and systematic full-scale comparison with human grading and feedback would provide valuable context for evaluating GPT-4’s relative performance. Such human benchmarks would allow for a deeper assessment of GPT-4’s alignment with human evaluators, offering insights into the accuracy, fairness, and reliability of AI-generated feedback. While such a benchmark was not incorporated in this study, future research should consider including both expert and peer assessments as comparison points to gauge the model’s effectiveness and identify areas for improvement.
This study employed a single prompting strategy—chain-of-thought prompting—to guide GPT-4’s responses, grading, and feedback generation. While chain-of-thought prompting was chosen to structure GPT-4’s outputs in a coherent, step-by-step manner, other prompting strategies might yield different results. For example, zero-shot or few-shot prompting approaches could offer alternative insights into GPT-4’s responses by allowing it more flexibility or by reducing bias associated with structured prompts. Investigating these approaches in future studies could provide a more comprehensive assessment of GPT-4’s potential in educational assessment, enabling educators to select the most effective prompting strategy for their specific needs.
To broaden the study’s evaluative scope, it would be beneficial to incorporate additional feedback sources, such as expert evaluations or peer reviews (in addition to the validity community). This would offer a more balanced view of GPT-4’s performance, capturing potential strengths and weaknesses that may not be fully revealed through AI-driven assessment alone. A more thorough framework for the use of expert feedback in the study, for example, could provide critical insights into GPT-4’s interpretation of nuanced exam content, while peer reviews could help identify practical implications for student engagement and learning.
The study’s focus on Norwegian exams poses challenges for generalizing the results across languages and educational systems. GPT-4’s performance may vary significantly in other languages due to linguistic and cultural nuances that influence interpretation and feedback quality. Furthermore, the model’s alignment with educational standards in Norway may not translate directly to other educational systems, which might have different grading standards, feedback expectations, and assessment frameworks. Future studies should test GPT-4 in diverse linguistic and educational contexts to evaluate the model’s adaptability and effectiveness across various academic settings.
As a large language model, GPT-4 carries inherent biases that may influence its assessment and feedback. These biases stem from the model’s training data, which may contain cultural or linguistic biases that could affect its interpretation of exam content and feedback generation. Additionally, given the rapid evolution of AI technology, newer models may outperform GPT-4, offering improvements in interpretive accuracy and fairness. This study, therefore, represents a preliminary exploration into AI-assisted assessment, and ongoing advancements in AI may lead to models that better align with educational objectives while minimizing biases.
Strengths:
• High performance: GPT-4 demonstrated strong alignment with human assessments, performing well in grading and providing detailed, structured feedback on essay-based exams.
• Detailed feedback: the use of chain-of-thought prompting and rubrics enabled GPT-4 to provide precise, thorough feedback, supporting both formative and summative assessment.
Limitations:
• Sample size and generalizability: the limited scope of six Norwegian-language exams restricts the study’s generalizability, and a broader sample would be needed to confirm GPT-4’s applicability in other academic and linguistic contexts.
• Lack of student feedback: insights from students on the usefulness and clarity of GPT-4’s feedback was not included, leaving a gap in understanding its practical impact.
• Prompting strategy limitations: sole reliance on chain-of-thought prompting may have constrained GPT-4’s performance, and exploring other strategies could yield additional insights.
• Absence of human benchmark: even if we had a validity community, a comparison with a more systematic and thorough human evaluations would provide context for GPT-4’s effectiveness relative to traditional grading practices.
In summary, while this study presents promising results for GPT-4’s role in AI-supported educational assessment, future research must address these limitations. Incorporating a broader sample, diverse prompting strategies, and human benchmarks will be essential to advancing our understanding of AI’s potential and limitations in academic assessment.
Building on our previous research on AI in education (Krumsvik et al., 2018, 2021; Krumsvik, 2023), this case study aims to examine GPT-4’s capabilities for formative and summative assessment in Norwegian educational settings. In the following part we will sum up the findings of the study and conclude based on the research questions of the study:
1 What is the capability of GPT on grading six essay-based exams in the Norwegian language?
In response to the first research question, this study found that GPT-4 demonstrates a promising capacity for accurately assessing responses to Norwegian essay-based exams, with the potential to serve as an effective sparring partner for students preparing for such assessments. Through its formative assessment capabilities, GPT-4 can provide personalized feedback that closely aligns with course objectives, making it a valuable tool in students’ learning processes. Consistent with established theories on feedback (Hattie and Timperley, 2007; Shute, 2008; Wollenschläger et al., 2016; Wisniewski et al., 2020), GPT-4 can offer students detailed, individualized insights into their performance, analyzing responses to pinpoint errors and guide students toward a clearer understanding of their mistakes, similar to the benefits of one-to-one tutoring (Bloom, 1984).
As a sparring partner, GPT-4 can help students practice and refine their essay responses in real time, simulating the feedback loop that is essential for effective learning. By delivering ongoing feedback, GPT-4 allows students to address misunderstandings and fill knowledge gaps promptly, helping them to avoid the “Kruger-Dunning effect” (Kruger and Dunning, 1999) and gain a realistic understanding of their capabilities. Additionally, by analyzing performance patterns, GPT-4 can support students in creating customized study plans, targeting areas that need improvement and enhancing study efficiency, much like an Intelligent Tutoring System (Van Lehn, 2011).
However, there are several limitations to consider. As this study represents an early phase of exploring GPT-4’s potential in educational assessment, more comprehensive and authentic testing is necessary to validate its effectiveness in diverse settings. GDPR and ethical considerations also present challenges in handling sensitive data responsibly, especially in providing personalized feedback without compromising student privacy. Additionally, effective use of GPT-4 as a sparring partner requires students to have a certain level of familiarity with chain-of-thought prompting, which may not be intuitive for all learners and could limit the model’s accessibility.
In summary, GPT-4 shows significant potential as a tool for formative assessment and as a sparring partner for students in essay-based exam preparation. Yet, realizing this potential will require careful consideration of ethical standards, AI-directives for higher education, data security, and support for students in engaging with chain-of-thought prompting effectively.
2 What is GPT-4’s capability of grading of six exams (feedback)?
Addressing the second research question, the study found that GPT-4 demonstrated a good capacity for grading essay-based exams according to established rubric criteria, showing potential as a reliable tool for summative assessment. When applied to the six Norwegian-language exams (3 on bachelor and master level, and 3 on PhD-level), GPT-4’s grading aligned closely with human evaluators across key criteria, such as content accuracy, coherence, argumentation, and use of evidence. This indicates that GPT-4 could serve as a valuable aid in grading tasks, supporting consistency and reducing the workload for educators, particularly in environments where grading demands are high.
GPT-4’s performance in grading suggests that it can provide detailed and consistent feedback, adhering to the rubric’s standards while offering specific evaluations in line with human grading practices. By delivering clear, criterion-based feedback, GPT-4 helps students understand their strengths and areas for improvement in a structured manner, reinforcing their academic progress through transparent and actionable feedback. This capability aligns with formative assessment goals, as students receive constructive feedback that can guide future performance.
However, several limitations must be considered. As this is an early-stage investigation into GPT-4’s grading potential, the findings are limited to a small sample size, and further studies are needed to confirm its accuracy across diverse subjects and exam formats. Additionally, ethical concerns surrounding the role of AI in grading need to be addressed, including the potential impact of biases inherent in the model’s training data, which could influence grading fairness. Furthermore, the model’s grading process relies on structured, consistent rubric criteria, which may require additional adjustments to accommodate various educational contexts and specific institutional requirements.
In conclusion, GPT-4 shows promise as a grading tool that can provide reliable, rubric-based evaluations, potentially easing grading burdens for educators and enhancing transparency for students. Nonetheless, a broader application of GPT-4 in grading will require further validation and careful attention to ethical and contextual considerations to ensure fair and accurate assessment outcomes.
3 GPT-4’s capability to suggest measures of improvement (Feed forward)
In addressing the third research question, this study found that GPT-4 demonstrates a promising capability to provide targeted, constructive feed forward aimed at guiding students toward improvement. Through the feed forward process, GPT-4 was able to identify specific areas where student responses could be enhanced, offering actionable suggestions that align with best practices in formative assessment. For instance, GPT-4 frequently highlighted areas for improvement in argument structure, coherence, and depth of analysis, providing students with clear steps to refine their work.
GPT-4’s ability to offer personalized recommendations mirrors aspects of individualized feedback often provided by educators, supporting students in their learning progression. This capability makes GPT-4 a potential tool for formative assessment, helping students recognize and address weaknesses in their responses while encouraging critical engagement with the material.
However, it is important to acknowledge limitations in GPT-4’s feed-forward capability. Although the model can suggest improvements based on patterns in the responses, it may not fully capture the nuanced guidance that a human instructor could provide, especially in complex or abstract topics. Additionally, the quality of GPT-4’s feedback may depend on the academic level (bachelor/master/PhD), clarity and specificity of the initial prompt, meaning that students may need training in effective prompt design to maximize the value of AI-generated feedback.
GPT-4 shows a potential in providing formative feedback to support student improvement on essay-based exams. Yet, realizing its full capability in educational settings will require ongoing refinement and a complementary role alongside human instructors to ensure feedback quality and depth.
Across these three research questions, this study on GPT-4’s capabilities in Norwegian essay-based assessments aligns with our previous studies of GPT-4 (Krumsvik, 2024a, 2024b, 2024c) and to a certain degree with other recent literature exploring ChatGPT’s application in educational contexts. For instance, studies by Imran and Almusharraf (2023) and Albadarin et al. (2024) emphasize ChatGPT’s effectiveness in generating text and providing feedback, mirroring our findings on GPT-4’s potential to support students in formative assessment by offering constructive, detailed feedback on essay responses. This is particularly relevant for personalized feedback, which both our study and Imran and Almusharraf’s review identify as beneficial for student learning, albeit with ethical considerations around AI’s impact on academic integrity and creativity.
Similarly, the work of Mai et al. (2024) underscores the importance of leveraging ChatGPT’s adaptive support in teaching and learning, with their SWOT analysis identifying strengths and opportunities, as well as threats related to misuse. Our study echoes these findings by highlighting the utility of GPT-4 in grading and feedback while acknowledging the need for careful implementation to maintain ethical standards and ensure responsible AI use.
The systematic review by Bhullar et al. (2024) also resonates with our study’s focus on formative and summative assessment, particularly in discussions on academic integrity and the potential of AI to enhance learning environments. Our findings on GPT-4’s role as a “sparring partner” for students align with Bhullar et al.’s theme of student engagement, while also noting the necessity for structured training in AI use to safeguard academic integrity.
Furthermore, Gao et al. (2024)‘s insights into AI’s potential for rapid, automated assessment reinforce our study’s findings on GPT-4’s grading capabilities, which offer consistency and efficiency in assessment while underscoring the need for ongoing refinement in AI models. And the study also resonates Guo et al.’s (2023) finding where GPT-4 can be viewed as a “team-player” in students learning processes where it offers both one to one tutoring and also contributions in collective settings (as one among several scaffolders around the students learning). Lastly, Ray (2023) provides a broader perspective on ChatGPT’s impact across fields, raising critical ethical questions regarding bias and fairness—concerns also addressed in our study as we consider GPT-4’s potential limitations in diverse educational contexts.
Overall, our study complements this growing body of research by providing a focused examination of GPT-4 in Norwegian educational settings, contributing empirical insights into its application and ethical considerations that align with the broader discourse on ChatGPT’s role in higher education.
The findings from this study have significant implications for both educators and AI developers. For educators, the application of GPT-4 in grading and feedback suggests that AI can serve as a valuable tool in supporting formative and summative assessment processes. By offering timely, detailed, and personalized feedback, AI models like GPT-4 can enhance student learning outcomes, enabling educators to focus more on individual mentorship and less on administrative tasks. Moreover, AI-driven assessment tools could help standardize grading practices, potentially reducing biases and increasing transparency in assessment across educational levels.
For AI developers, this study highlights the need for continual refinement of language models to address language-specific challenges and better align with educational standards. Developers should consider creating more domain specific and adaptable models that can seamlessly operate across various languages and academic fields, accommodating the specific needs of educational assessment. Additionally, developing models with enhanced explainability could improve educators’ confidence in AI tools, as teachers and students could more easily understand the rationale behind AI-generated feedback and grading.
As AI becomes increasingly integrated into educational settings, collaboration between educators and AI developers will be essential. This partnership can ensure that AI tools are designed with the educational context in mind, aligning with curriculum objectives, ethical standards, and cultural considerations. The insights from this study underscore the potential of AI to transform assessment practices in a manner that supports both teaching and learning, while also highlighting the importance of rigorous testing and refinement to fully realize these benefits.
In summary, this study highlights GPT-4’s potential as a multifaceted tool in educational assessment, particularly within Norwegian-language essay-based exams. Through the feed up process, GPT-4 demonstrated an ability to effectively interpret exam content and provide personalized feedback aligned with learning objectives, positioning itself as a supportive sparring partner for students in formative assessment. In terms of feedback, GPT-4 proved capable of grading exams reliably according to rubric criteria, showing consistency with human evaluators and offering detailed, structured evaluations that can enhance both efficiency and transparency in summative assessment. Lastly, through feed forward, GPT-4 exhibited potential in suggesting targeted improvements, guiding students toward better understanding and performance in future assessments.
These findings underscore GPT-4’s role in supporting students and educators alike by delivering detailed feedback and formative guidance. However, realizing GPT-4’s full potential will require further validation, particularly regarding ethical considerations, data privacy, and the adaptability of chain-of-thought prompting. As AI continues to develop, GPT-4 offers a promising step toward more personalized, consistent, and accessible assessment practices, contributing meaningfully to educational innovation.
The raw data supporting the conclusions of this article will be made available by the authors, without undue reservation.
RK: Conceptualization, Formal analysis, Investigation, Methodology, Resources, Software, Validation, Writing – original draft, Writing – review & editing.
The author(s) declare that no financial support was received for the research and/or publication of this article.
The author would like to thank the three reviewers of this article for their constructive feedback, which has helped improve the manuscript, the validity community for providing valuable insights for this case study, and others who assisted me in revising the manuscript.
The author declares that the research was conducted in the absence of any commercial or financial relationships that could be construed as a potential conflict of interest.
The author(s) declared that they were an editorial board member of Frontiers, at the time of submission. This had no impact on the peer review process and the final decision.
The author(s) declare that Generative AI was used in the development of this manuscript. GPT-4 (OpenAI, 2024) was employed in this article to examine the exam questions and translation of exam questions and rubrics from Norwegian to English, and Gemini Advanced (Google, 2024) was employed as one of validity communities. The GPT-4’s output was manually examined, edited, and reviewed by the author.
All claims expressed in this article are solely those of the authors and do not necessarily represent those of their affiliated organizations, or those of the publisher, the editors and the reviewers. Any product that may be evaluated in this article, or claim that may be made by its manufacturer, is not guaranteed or endorsed by the publisher.
The Supplementary Material for this article can be found online at: https://www.frontiersin.org/articles/10.3389/feduc.2025.1444544/full#supplementary-material
1. ^This is based on the grade scale (University of Bergen, 2018) and the “sensorveiledning” for the MMR-course.
2. ^This is why we trained and developed a chatbot for PhD-student working with their PhD thesis in November 2023 (see here: https://chatgpt.com/g/g-T6wJuA5tr-writing-the-synopsis-companion-for-phd-candidates).
Albadarin, Y., Saqr, M., Pope, N., and Tukiainen, M. (2024). A systematic literature review of empirical research on ChatGPT in education. Discov. Educ. 3:60. doi: 10.1007/s44217-024-00138-2
Bhullar, P. S., Joshi, M., and Chugh, R. (2024). ChatGPT in higher education - a synthesis of the literature and a future research agenda. Educ. Inf. Technol. 29, 21501–21522. doi: 10.1007/s10639-024-12723-x
Bloom, B. (1984). The 2 sigma problem: the search for methods of group instruction as effective as one-to-one tutoring. Educ. Res. 13, 4–16. doi: 10.3102/0013189X013006004
Creswell, J. W., and Guetterman, T. C. (2021). Educational research: Planning, conducting and evaluating quantitative and qualitative research. 6th Edn. Harlow: Pearson.
Gao, R., Merzdorf, H. E., Anwar, S., Hipwell, M. C., and Srinivasa, A. R. (2024). Automatic assessment of text-based responses in post-secondary education: a systematic review. Comput. Educ. Artif. Int. 6:100206. doi: 10.1016/j.caeai.2024.100206
Google. (2024). Gemini advanced (version 1.5 pro). Google. Available online at: https://support.google.com/gemini/answer/14517446
Guo, B., Zhang, X., Wang, Z., Jiang, M., Nie, J., Ding, Y., et al. (2023). How close is ChatGPT to human experts? Comparison corpus, evaluation, and detection. ArXiv. doi: 10.48550/arXiv.2301.07597
Hattie, J., and Timperley, H. (2007). The power of feedback. Rev. Educ. Res. 77, 81–112. doi: 10.3102/003465430298487
Hesse-Biber, S., Rodriguez, D., and Frost, N. A. (2015). Qualitatively Driven Approach to Multimethod and Mixed Methods Research. I. S. Hesse-Biberog and B. Johnson, The Oxford Handbook of Multimethod and Mixed Methods Research Inquiry (s. 3–20) (Oxford Library of Psychology). Oxford: Oxford University Press.
Imran, M., and Almusharraf, N. (2023). Analyzing the role of ChatGPT as a writing assistant at higher education level: a systematic review of the literature. Contemp. Educ. Technol. 15:ep464. doi: 10.30935/cedtech/13605
Johnson, R. B., and Christensen, L. (2017). Quantitative, Qualitative, and Mixed Research. In: Educational Research: Quantitative, Qualitative, and Mixed Approaches. eds. R. B. Johnson and L. Christensen, 6th ed, US: SAGE Publications Inc. 2, 29–56.
Kruger, J., and Dunning, D. (1999). Unskilled and unaware of it: how difficulties in recognizing one’s own incompetence lead to inflated self-assessments. J. Pers. Soc. Psychol. 77, 1121–1134. doi: 10.1037/0022-3514.77.6.1121
Krumsvik, R. J. (2024a). GPT-4's capabilities of formative and summative assessment in Norwegian medicine exam-an intrinsic case study (June 06, 2024). Available online at: https://ssrn.com/abstract=4948469 or (Accessed June 6, 2024).
Krumsvik, R. J. (2024b). Artificial intelligence in nurse education – a new sparring partner? GPT-4 capabilities of formative and summative assessment in National Examination in anatomy, physiology, and biochemistry. Nordic J. Digit. Lit. 19, 172–186. doi: 10.18261/njdl.19.3.5
Krumsvik, R. J., Berrum, E., and Jones, L. Ø. (2018). Everyday digital schooling – implementing tablets in Norwegian primary school. Examining outcome measures in the first cohort. Nordic J. Digital Liter. 13, 152–176. doi: 10.18261/issn.1891-943x-2018-03-03
Krumsvik, R. J., Berrum, E., Jones, L. Ø., and Gulbrandsen, I. P. (2021). Implementing tablets in Norwegian primary schools. Examining outcome measures in the second cohort. Front. Educ. 6:642686. doi: 10.3389/feduc.2021.642686
Krumsvik, R. J. (2024c). Chatbots and academic writing for doctoral students. Educ Inf Technol. doi: 10.1007/s10639-024-13177-x
Mai, D. T. T., Da, C. V., and Hanh, N. V. (2024). The use of ChatGPT in teaching and learning: a systematic review through SWOT analysis approach. Front. Educ. 9:1328769. doi: 10.3389/feduc.2024.1328769
Maxwell, J. A. (2009). Qualitative research design: An interactive approach. 2nd Edn. London: SAGE Publications.
Norman, D. (1999). Affordance, conventions, and design. Interactions 6, 38–43. doi: 10.1145/301153.301168
OpenAI (2023). ChatGPT (GPT-4) (march 2023 – March 2024 version) [large language model]. Available online at: https://chatgpt.com/
Ray, P. P. (2023). ChatGPT: a comprehensive review on background, applications, key challenges, bias, ethics, limitations and future scope. Int. Things Cyber Phys. Syst. 3, 121–154. doi: 10.1016/j.iotcps.2023.04.003
Shute, V. J. (2008). Focus on formative feedback. Rev. Educ. Res. 78, 153–189. doi: 10.3102/0034654307313795
University of Bergen (2018). Grading scale. Available at: https://www.uib.no/en/student/126257/grading-scale (Accessed May 20, 2023).
University of Bergen (UiB) (2018). Grading scale. Available at: https://www.uib.no/en/for-students/exams-and-submissions/grades-and-diploma/grading-scale. (Accessed April 13, 2024).
Van Lehn, K. (2011). The relative effectiveness of human tutoring, intelligent tutoring systems, and other tutoring systems. Educ. Psychol. 46, 197–221. doi: 10.1080/00461520.2011.611369
Wisniewski, B., Zierer, K., and Hattie, J. (2020). The power of feedback revisited: A meta-analysis of educational feedback research. Front. Psychol. 10:3087. doi: 10.3389/fpsyg.2019.03087
Keywords: GPT-4, formative assessment, summative assessment, essay based exams, Norwegian language, case study, educational innovation
Citation: Krumsvik RJ (2025) GPT-4’s capabilities in handling essay-based exams in Norwegian: an intrinsic case study from the early phase of intervention. Front. Educ. 10:1444544. doi: 10.3389/feduc.2025.1444544
Received: 05 June 2024; Accepted: 04 March 2025;
Published: 19 March 2025.
Edited by:
Davide Girardelli, University of Gothenburg, SwedenReviewed by:
Vanessa Scherman, International Baccalaureate (IBO), NetherlandsCopyright © 2025 Krumsvik. This is an open-access article distributed under the terms of the Creative Commons Attribution License (CC BY). The use, distribution or reproduction in other forums is permitted, provided the original author(s) and the copyright owner(s) are credited and that the original publication in this journal is cited, in accordance with accepted academic practice. No use, distribution or reproduction is permitted which does not comply with these terms.
*Correspondence: Rune Johan Krumsvik, UnVuZS5rcnVtc3Zpa0B1aWIubm8=
Disclaimer: All claims expressed in this article are solely those of the authors and do not necessarily represent those of their affiliated organizations, or those of the publisher, the editors and the reviewers. Any product that may be evaluated in this article or claim that may be made by its manufacturer is not guaranteed or endorsed by the publisher.
Research integrity at Frontiers
Learn more about the work of our research integrity team to safeguard the quality of each article we publish.