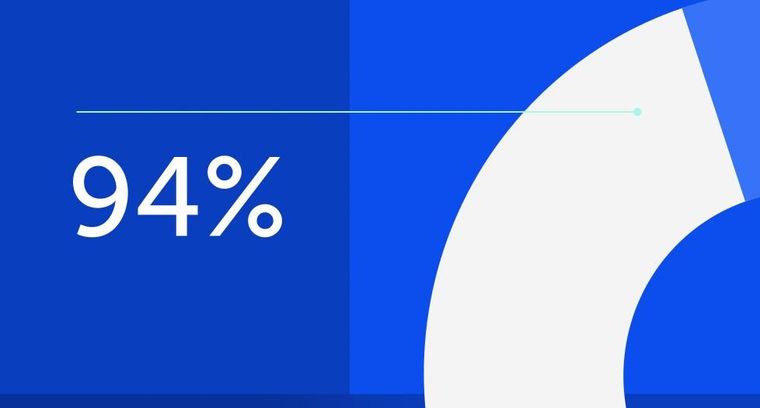
94% of researchers rate our articles as excellent or good
Learn more about the work of our research integrity team to safeguard the quality of each article we publish.
Find out more
ORIGINAL RESEARCH article
Front. Educ., 01 April 2025
Sec. Leadership in Education
Volume 10 - 2025 | https://doi.org/10.3389/feduc.2025.1416761
This article is part of the Research TopicContinuing Engineering Education for a Sustainable FutureView all 19 articles
This manuscript introduces an educational approach by integrating Industry 4.0 and 5.0 technologies within a Smart Factory learning environment at Tecnologico de Monterrey (Tec) and through international collaboration with the Massachusetts Institute of Technology (MIT). It addresses the challenge of adapting engineering education to the demands of smart manufacturing, highlighting the importance of hands-on experience with cyberphysical systems, Additive Manufacturing (AM), Supervisory Control and Data Acquisition (SCADA) integration, and robotics. By integrating the curriculum with Sustainable Development Goals (SDGs), the initiative aims to train engineers capable of contributing to sustainable industrial practices and innovation. The Automation of Manufacturing Systems course provides immersive learning through direct engagement with advanced technologies and methodologies. Students develop technical skills in collaborative robots (cobots), human-machine interfaces (HMI), computer vision and Programmable Logic Controller (PLC) programming. This also develops methodological skills in analytical thinking problem-solving and other competences. The curricula further enhanced social and personal competencies, preparing students to lead in a technologically advanced and sustainable industrial environment. With the emergence of Industry 5.0, which emphasizes sustainability and worker wellbeing, this educational model integrates social behavior science with digital technologies in a student-centered approach. The presented case study highlights the effectiveness of redesigning the course to include immersive learning experiences focused on Industry 4.0 and 5.0 skills. A student survey conducted at the end of the course validated these findings, revealing high satisfaction levels (9.65/10) associated with developing new skills, exploring new concepts, generating new ideas, and promoting innovative thinking. Both the students and the teaching team offered recommendations to further scale and improve the course.
Sectors worldwide are transitioning to Industry 4.0, driving universities to adapt engineering education to include emerging technologies. Industry 4.0 refers to the integration of digital technologies in manufacturing to drive benefit innovation during industrialization stage, relevant components are shown in Table 1. These technologies enable smart factories where machines and systems communicate and optimize production processes autonomously, enhancing three pillars: (1) efficiency, (2) flexibility, and (3) scalability (Culot et al., 2020). In response to this shift, the Tec21 Educational Model, implemented in 2019 across 26 campuses of Tecnológico de Monterrey, redefines traditional education by focusing on developing both disciplinary and transversal competencies through real-world challenges. It organizes the academic experience into blocks that integrate content modules, along with Tec Weeks (Semana Tec), Week 18 (Semana 18), and Tec Semesters (Semestre Tec). Tec Weeks, occurring in weeks 6 and 12, are immersive sessions centered on skills like leadership and entrepreneurship. Week 18, at the semester’s end, emphasizes reflection and feedback, allowing students to evaluate progress and set new goals. The model emphasizes a student-centered relationship with faculty acting as facilitators. It promotes challenge-based learning, interdisciplinary collaboration, and advanced technologies to prepare students for modern industry demands. Central to this model is the “challenge,” a hands-on experience that confronts students with real-world situations to develop competencies in problem-solving and collaboration.
The model also focuses on three core components: Contributions, which include society, economy, and industry; Competencies, such as creativity and adaptability; and Commitments, like integrity and inclusivity, all of which create a challenging, inspiring, and learning environment for students and professors (Figure 1). The integration of Industry 5.0 technologies in Tec21 further enhances students’ technical and socio-emotional skills, preparing them for sustainable industrial environments. By embedding Sustainable Development Goals (SDGs) into the curriculum, the model ensures alignment with global innovation and sustainability priorities. Key elements include:
1. Industry 5.0 integration: This study enables structured collaboration among collaborative robots, automated systems, and students within a Smart Factory, emphasizing resilience by integrating digital twins, ergonomics, and production-flow optimizations. It aligns with SDGs 4, 9, and 10 through practical challenges that merge PLC programming, vision systems, and additive manufacturing to equip students with the technical and socioemotional competencies needed in evolving industrial contexts, reducing inequalities in access to advanced technologies while reinforcing global responsibility.
2. Student 5.0 framework: We propose a holistic model merging Education 5.0, Industry 5.0, and Society 5.0 to prepare students for future challenges.
3. Smart factory implementation: Practical applications, such as the redesigned Automation of Manufacturing Systems course, provide hands-on experience with advanced technologies in a Smart Factory setting.
Figure 1. Framework of TEC 21 Educational Model highlighting contributions, competences, and commitments, along with the dynamic relationship between students, professors, and the learning environment.
To ensure a thorough understanding of Industry 4.0 components, specific modules were introduced where students actively worked with IoT sensors, AI algorithms for predictive maintenance, and collaborative robots for automation tasks. Each component was linked to real industrial applications, such as monitoring production efficiency through IoT networks or using AI to optimize robotic operations. The curricula adaptation involves integrating automation, digital process integration, cyber-physical systems, and the analytics of the information produced (Lu, 2017). According to Nosalska et al. (2020), Industry 4.0 can be divided into six different areas: systems and automation, industrial networks and connectivity, data science, manufacturing technologies, robots, and information technologies (Nosalska et al., 2020). Engineering education now including topics such as internet of things (IoT), cloud computing, Virtual Reality and Augmented Reality (VR/AR), computational simulations, additive manufacturing, Artificial intelligence (AI), data analytics, cybersecurity, robotics, and smart factories are driving the transformation in the contemporary industry. In this way, students are learning new ways of thinking that make them more competitive for the industry, such as, adaptative thinking, cognitive and programming skills, and data analytics (Mian et al., 2020). Moreover, inclusion of Industry 4.0 has proven to emerge new engineering skills such as (1) technical: programming and understanding processes, operations and solving complex problems, (2) methodological: analytical and research skills, (3) social: ability to see the big picture, lead, communicate and start technological changes, and (4) personal: ability to work in complex environments and commitment for lifelong learning (Jerman et al., 2018). Furthermore, another challenge in transforming engineering education relates to the integration of the Sustainable development Goals (SDGs) outlined in the United Nations Agenda 2030. Through a review of the literature, it has been demonstrated a strong correlation between with SDGs 8, 9, and 12 which focus on decent work and economic growth, industry, innovation and infrastructure, and responsible consumption and production, respectively with the industry 4.0 (Beier et al., 2021). Taking this into account, appropriately incorporating these goals in education content can advance manufacturing productivity and enhance competitiveness of companies and regions (Rüßmann et al., 2015).
Considering the evolving needs of the industry for novel engineering programs and their appropriate integration into new career trajectories, it has been suggested preparing new infrastructure where students can enrich their preparation (Fuertes et al., 2021). Tecnologico de Monterrey addresses these evolving industry and educational needs by implementing the Tec21 Educational Model and offered a new class of Automation of Manufacturing System, emphasizing skills-based learning to solve real industry challenges though tools such as a Smart Factory and hands-on learning with Industry 4.0 technologies (e.g., manufacturing, sensing, and controlling tools), preparing students to advance SDGs through digital automation. The course emphasizes the development of automated industrial systems using design and simulation tools and programmable devices, ensuring compliance with performance and quality standards (Rodriguez-Padilla et al., 2023). Key learning outcomes include the ability to apply mechanical, electronic, software, and automatic control technologies and methodologies appropriately, select components based on technical specifications, design automation strategies for processes, systems, and machinery, and analyze the interactions and synergies within mechatronic systems. This course requires prior knowledge in mechatronic design, system modeling, control theory, robotics, and industrial automation (Content of Automation of Manufacturing Systems Course, 2019).
However, the current panorama is shifting beyond merely incorporating, implementing, and adapting the latest Industry 4.0 technologies into engineering curricula. We are now at the threshold of a new era, Industry 5.0, which is poised for implementation. This new chapter in industry, emphasizes in sustainability and the wellbeing of workers (Xu et al., 2021), an aligning closely with Sustainable Development Goals (SDGs) 3, which focuses on good health and wellbeing, and SDG 9, which underscores the importance of industry, innovation, and infrastructure (Kasinathan et al., 2022). By incorporating these two approaches, industry transformation must encompass social behavior science as well as integration of digital information, computation and communication (Froyd et al., 2012). In contrast to Industry 4.0, where cyber-physical systems catalyze digital transformation by having connection between digital and real world (Klotzer et al., 2017). A new approach has been introduced for Industry 5.0, rethinking how information, production, and automation are conceived through virtual simulations to address both physical and social challenges. In this paper, we examine how students interact, learn, and apply state-of-the-art digital technologies within a manufacturing cell featuring collaborative robots (cobots), with particular emphasis on human interaction, ergonomics, and adaptability—elements that align with Industry 5.0 instrumentation (Ivanov, 2023).
The motivation for this work is to align engineering education with the evolving technological landscape of Industry 4.0 and 5.0, integrating advanced technologies like cyber-physical systems, additive manufacturing, and robotics. The redesign of the Automation of Manufacturing Systems course was driven by the need to address the gaps in current engineering education, specifically related to integrating hands-on experiences with Industry 4.0 and 5.0 technologies. Feedback from industry stakeholders highlighted a need for students with practical exposure to technologies such as collaborative robots and digital twins. Therefore, this course was restructured to provide students with the practical skills that align directly with the evolving needs of the industry. It emphasizes a holistic approach that combines traditional and immersive learning experiences to produce well-rounded engineers equipped with technical, social, and personal competencies. The goal is to foster critical thinking, problem-solving, and lifelong learning skills, ensuring students can meet industry demands and lead in technologically advanced, sustainable, and human-centric workplaces. This manuscript introduces an immersive educational paradigm under the Education 5.0 and Industry 5.0 panorama, offering a novel approach to teaching automation in manufacturing systems alongside skills assessment for a Student 5.0.
To rigorously assess the effectiveness of this educational model, this research employs a mixed-methods approach. It begins with a literature review exploring educational models and industry experiences within Industry 4.0 and 5.0, setting the foundation for identifying gaps and best practices in emerging pedagogical methods and curricula. A research group, composed of faculty members and industry partners, collaborated to redesign the Automation of Manufacturing Systems course. This redesign integrates hands-on learning with cyber-physical systems, technical implementation, and simulations aligned with Industry 5.0, demonstrating their application in an educational context. The course evaluation includes learning outcomes and student feedback. Additionally, quantitative and qualitative data were collected through student surveys, competency-based assessments, and observational analysis to measure learning outcomes, student satisfaction, and their understanding of the course. This structured methodology provides a comprehensive evaluation of how immersive learning experiences contribute to student engagement and skill development.
In contrast to a rigid curriculum, the flexible approach of the Tec21 Educational Model allows students multiple opportunities to shape their education through the curricular pathways model. This model offers students the ability to explore, decide, and specialize throughout their academic journey. From the first semester, students can choose a disciplinary area, gaining exposure to both their chosen field and other disciplines, which helps them evaluate various options before making a final decision. This structure reflects a “few entries, many exits” approach, enabling diverse career pathways. In comparison, the shift from a traditional curriculum—focused on theoretical knowledge and individual performance—to the Student 5.0 curriculum demonstrates the evolution toward integrating advanced interdisciplinary modules, sustainability, and human-centric design. Student 5.0 emphasizes holistic problem-solving through integrated projects, such as combining robotics and programming with sustainability challenges, rather than separate courses, aligning education with future industry demands (Table 2).
Education 4.0 has envisioned to define the influence of applying this industrial revolution (Popova-Nowak and Cseh, 2020). It uses emerging technologies and cyber-physical systems to enhance both teaching and learning process, thereby familiarizing young engineers to Industry 4.0 environments educational providers and innovation drives along learning portals, partners, and software support the process (Mourtzis et al., 2018). Meanwhile, Mukul and Büyüközkan stated that students have the autonomy to develop and complement their education through several digital platforms and resources. This environment can be established with a university or crossing borders through cyber-physical systems. Teaching can be carried out creatively in different stages of life with content that is generatively customizable, DIY, or audience-specific, founded on the learning theory is connectivism (Mukul and Büyüközkan, 2023). A study on how personalized content, offer students various options for learning styles, and teach through different project-based learning methods to gain experience in educational environments (Popova-Nowak and Cseh, 2020).
Education 5.0 represents a shift toward personalized learning supported by advanced technologies, aiming to provide adaptable and inclusive educational experiences with a focus on creating student-centered learning environments (Garg et al., 2024). Education 5.0 incorporates the previous phases of Education 4.0 along with the emerging technologies and methodologies from Industry 4.0. It also integrates the well-established frameworks of engineering education offered by universities and educational institutions, complemented by advanced technologies to address real-world challenges related to sustainability and societal wellbeing. This integration would not be possible without the support of accreditation agencies, certifications from industrial companies, and professional educational institutions (Lantada and Lantada, 2022). Education 5.0 introduces several aspects to engineering education, including (1) a holistic approach, emphasizing interdisciplinary engineering for greater impact; (2) a humanistic perspective, promoting education that is shared and distributed for progressive adoption; (3) an ethical guidance, recognizing the importance of ethics, which, while secondary in the era of Industry 4.0 and AI, now demands attention to benefit from experience and ensure ethical considerations are addressed; (4) international experiences, preparing students for diverse, international, and multicultural environments, encouraging exposure to new approaches and unique developments from different organizations; (5) support from technology and assistance from artificial intelligence; (6) enjoyable and enhanced outcomes, where education aims to be “joyful, meaningful, socially iterative, and actively engaging” (Liu et al., 2017) and (7) education for all, aligned with the SDGs to ensure no one is left behind, thus making engineering education widely distributed and accessible (Lantada et al., 2016). The concept of Student 5.0 is introduced as a learned with a holistic formation, with personalized and tech education focusing on socio emotional skills such as critical thinking, creativity, collaboration, and lifelong learning (Education 5.0), strong emphasis on human-machine collaboration, sustainability, innovation and social-ethical responsibility (Industry 5.0), and with the perspective that technology should focus on achieving and enhancing the quality of life for all, global and cultural competences, and empathy for others (Society 5.0). Figure 2 presents an overview of how all elements are connected to create the Student 5.0.
Figure 2. Student 5.0 holistic integration of Society 5.0, Industry 5.0 and Education 5.0 adapted from Farinha (2012) and Huang et al. (2022).
In this approach, the Teaching Factory (TF) model creates an environment for collaboration between academia and industry, establishing a safe space for education, research, and practical innovation. It also serves as a virtual forum for all manufacturing knowledge, offering a unique experience that integrates current academic training with the needs of industrial partners and the demands of outsourcing manufacturing initiatives (Chryssolouris et al., 2016). However, the Learning Factory (LF) concept, introduced in the United States in 1995, facilitates both formal and informal learning for industry and academia alike. For example, the Vienna University of Technology (VUT) offers an interdisciplinary and practice-oriented approach, making an active enjoyable learning experience in manufacturing and production more engaging in pilot production than passively listening to lectures in the classroom (Jäger et al., 2012). Similarly, at EAFIT University’s Learning Factory, students enhance their learning through the integration of didactic methods with engineering education (Baena et al., 2017). Another instance is the Industry 4.0 technologies Laboratory (I4Tech Lab), which promotes education for Industry 4.0 by creating a realistic environment equipped with a variety of technologies, including Augmented Reality and IoT (Prieto et al., 2019). The previous examples are considered immersive learning environments for comprehensive manufacturing education. Table 3 integrates the objectives of the proposed approach by having the integration of Industry 4.0 and 5.0 technologies with the Education 4.0 and 5.0 skills, learning objectives and how it is approach in our Smart Factory.
Table 3. Integration of Education 4.0 and 5.0 skills with Industry 4.0 and 5.0 technologies in a Smart Factory context.
Building upon these immersive learning environments, advanced technologies like Artificial Intelligence (AI) and Machine Learning (ML) are further transforming education, especially within the framework of Education 5.0. AI and ML enable personalized, adaptive learning experiences that fit individual students’ needs, thereby enhancing the effectiveness of educational outcomes (Kochmar et al., 2020; Kim et al., 2022; Kamalov et al., 2023). This impact is evident in several key areas:
• Personalized learning paths: AI algorithms analyze a student’s performance data across various subjects to create customized learning plans. For example, if a student excels in mathematics but struggles with physics, the system adjusts by providing more challenging math problems while offering additional support and resources in physics. This approach ensures that students are neither bored with content that is too easy nor overwhelmed by content that is too difficult, optimizing their learning potential.
• Adaptive assessment and feedback: Intelligent tutoring systems use ML to assess student responses in real-time. If a student answers a question incorrectly, the system identifies the misconception and provides immediate, targeted feedback. Immediate feedback helps students correct errors promptly, reinforcing learning and preventing the reinforcement of misunderstandings.
• Predictive analytics for student support: ML models analyze data such as attendance, assignment submissions, and engagement in online discussions to predict which students are at risk of falling behind. Educators can proactively reach out to these at-risk students to offer support, thereby improving retention rates and academic performance.
• Language processing and communication: Natural Language Processing (NLP) enables AI chatbots to answer student queries 24/7. For example, a student studying late at night can ask the chatbot to explain a concept or provide resources. This continuous support enhances learning flexibility and accommodates different student schedules.
• Administrative efficiency: AI automates administrative tasks like scheduling, grading multiple-choice assessments, and managing enrollments. Educators can focus more on teaching and mentoring rather than administrative duties, improving the overall educational experience.
The proposed methodology integrates experiential and challenge-based learning components that differ from the traditional ECTS credit allocation, which typically emphasizes lecture hours and theoretical assessments. Considering that 1 Tec credit is approximately equivalent to 1.67 ECTS/1 US credit and that full academic workload at Tec is 18 credits (30 ECTS or 18 US credits) over an 18-week period, this course runs from week 7 to week 18 and carries 12 credits (∼66.6% of a full workload). Through the immersive educational journey offered by our Smart Factory, the course on Automation of Manufacturing Systems prepares students with hands-on experience, preparing them for the complexities of Industry 5.0. The curriculum begins by familiarizing students with fundamental manufacturing concepts such as cycle times, ergonomics, and optimization techniques, including the principles of 5S, 7 wastes and principles of Lean Manufacturing (Hurtado et al., 2023), along with operational and production efficiency. The course was designed to align with SDG 9 (Industry, Innovation, and Infrastructure) by teaching students to use advanced technologies for developing efficient automation solutions. For SDG 4 (Quality Education), the integration of digital twins allowed students to gain practical skills in a simulated environment, which reduced the barriers to accessing physical equipment. Additionally, the project-based learning approach promoted inclusivity, directly contributing to SDG 10 (Reduced Inequalities). As the course progresses, students deepen their technical knowledge by operating collaborative robots (cobots), where they should consider the impacts of having the collaboration between workers and robots (Green et al., 2008), and how to integrate them into the production engineering, acquire skills in Human-Machine Interface (HMI) systems, PLC programming, sensor integration, and explore the functionalities of vision systems. The course culminates in a comprehensive application of this knowledge in the challenge, enabling students to integrate manufacturing systems with automation technologies, placing particular emphasis on sustainable practices and the ergonomic aspects of human-cobot collaboration. An essential part of this educational path involves the virtual creation and application of fixtures, jigs, and poka-yokes, aimed at enhancing and automating production processes in alignment with Design for Manufacturing and Assembly (DFMA) principles. Utilizing Tecnomatix Process Simulate to verify automation projects not only demonstrates the real-world application and improvement of these processes but also provides valuable feedback from students, leading to the continuous improvement of their proposal. This strategy aims to ensure that students develop a thorough grasp of the technical, social, and ergonomic considerations key to Industry 4.0 technologies, preparing them to lead and innovate in the rapidly advancing domain of Industry 5.0.
First, as context, students were immersed in developing the subassembly for the electronics of a custom-made, low-cost extrusion machine that produces microfibers and are measured known as Fiber Extrusion Device (FrED), a product of the Tec-MIT collaboration (Li, 2023). The goal was to automate FrEDs production within a teaching/learning factory environment, such as Tec’s Smart Factory in 7 weeks. Sequences of the learning methodology are shown in Figure 3. Initially, a kick-off meeting with MIT professors and graduate students was launched. Then students received training in various Industry 4.0 technologies during the first 3 weeks in the Smart Factory. The course began by teaching them with hands on training: (1) PLC programming with TIA Portal (PLC 1200, Siemens, Germany), followed by instruction on collaborative robots like the xArm-6 (Ufactory, China), infrared sensors including PNP and NPN, a conveyor belt equipped with a DC motor as an actuator, industrial buttons, and Human Machine Interfaces (HMIs). Additionally, students explored computed vision using Data Logic Pro (Datalogic, Italy), all aimed at understanding how SCADA functions and integrating these elements to automate the manufacturing process. By having all this training students acquire all the skills to attend real word problems (Pinzone et al., 2017). At the same time, (2) theoretical and logical training was given by introducing the students: to the basics of industrial engineering, including the definition of the Product Lifecycle Management (PLM), Diagram of Process Operation (DOP), layout design for the manufacturing cell line, cycle time determination, facility walkthroughs, the implementation of 5S, and the advantages of using fixtures, jigs, and poka-yokes in coordinating manufacturing operations. Quality topics were considered in the application of computed vision for inspection. With these lectures and previous training, students get the knowledge required for automation by having a full perspective of what is required for an industrial solution.
Alongside these phases, (3) students learned virtual simulators training where they ventured into digital twin creation using Tecnomatix Process Simulate (Siemens, Germany) by a series of recorded trainings with typical manufacturing equipment like the one in the Laboratory in 15 h. For the challenge, they first designed the station with SolidWorks, then exported JT files using Autodesk Inventor (Autodesk, United States). Subsequently, they learned to create kinematics, material flow, and virtual commissioning to prepare for the challenge. Moreover, a series of advisory meetings were held to refresh previous knowledge on (1) the use of additive manufacturing and Computer Aided Design (CAD), specifically Filament Fused Fabrication (FFF) and laser cutting, for producing physical station equipment via Computed Aided Manufacturing (CAM), (2) basics of electronics and microcontrollers (i.e., Arduino), and (3) machine elements such as couplings, bearings, aluminum profiles, T-nuts, screws, and other mechanical components.
During the intensive 60-h training program, distributed over 20 h each week, a five-member student team will undertake various specialized roles. This includes one student focusing on design and fabrication, another dedicated to the development of process flow logistics, a third specializing in electronics, a fourth acting as the project manager, and a fifth responsible for control mechanisms. All the students participated in the integration process and collaborated with MIT graduate students to develop their automation and decision-making regarding their assembly tasks. Students tackled the first part of the challenge: designing and developing the station’s elements (storage unit, fixtures, gripper, jigs, etc.) taking 20 h. They were tasked with creating an optimal gripper for their application, determining the placement of parts for assembly, and deciding on the tools for workers and cobots (fixtures, jigs, and poka-jokes). Upon completion, students began to design the automation flow, integrating worker and cobot/station tasks to minimize downtime and prevent production bottlenecks. Following this, the challenge escalated as they were required to provide the final Diagram of Process Operation (DOP) and the automation flow (Quintero-Sanchez et al., 2024). They then initiated simulations using Tecnomatix Process Simulate, where students conducted various simulations to review the process, movements, and interactions between cobot and worker in a virtual environment, aiming for an ergonomic, comfortable, and safe automation setup.
The immersive experience within the Smart Factory aimed to achieve a set of educational goals, meticulously designed to enrich students’ learning journeys, and prepare them for the evolving industrial panorama. The learning objectives were categorized into three primary domains: technical, methodological, and social skills. Technical objectives included mastering PLC programming and using Human-Machine Interface (HMI) systems. Methodological objectives focused on developing problem-solving skills using real-world scenarios, while social objectives included enhancing teamwork through collaborative assignments. Each objective was mapped to specific course activities to ensure measurable outcomes. These goals are outlined as follows:
• Immersive engagement: To provide students with a fully immersive, memorable, and enjoyable experience in the Smart Factory, ensuring that they engage deeply with the learning material and technological environment.
• Technical proficiency: To master technical knowledge critical to Industry 4.0 and foundational industrial engineering concepts, including Product Life Cycle Management. This goal emphasizes the importance of understanding the technological advancements shaping modern manufacturing and production processes.
• Skills development for future education: To develop skills and abilities that align with the principles of Education 4.0 and 5.0, preparing students for a future where technological fluency, adaptability, and continuous learning are paramount.
• Reflection on Sustainable Development Goals (SDGs): To encourage students to reflect on the role of SDGs within the industry and recognize their potential to revolutionize the sector through informed and sustainable practices. This goal aims to highlight the significance of integrating sustainability into industrial operations and the powerful impact of training within an industrial context.
• Application of mechatronics knowledge: To apply the knowledge and skills acquired throughout their Bachelor in Mechatronics Engineering program, allowing students to connect theoretical concepts with practical applications in a meaningful way.
• Virtual and real-world integration: To offer an integral opportunity for practice in a virtual environment through digital twins. This includes a comprehensive understanding of automation design, considering the human element in the process and improving a harmonious relationship between workers and cobots.
To ensure the reliability and validity of our data, we employed a mixed-methods approach that included both quantitative surveys and qualitative observations. (1) For the quantitative component, we used the university’s standardized ECOA system to administer structured student evaluation surveys at the end of the course, gathering data on five key constructs related to teaching effectiveness and learning outcomes: instructor expertise and mastery, student engagement and motivation, learning environment and trust, instructor support and feedback, and overall learning experience. (2) For the qualitative component, we conducted classroom observations and oral argumentative assessments to gain deeper insights into students’ problem-solving approaches, technical decision-making, and teamwork. We then applied thematic analysis to categorize student responses and behaviors into core themes such as technical competence, critical thinking, and collaborative engagement, identifying recurring patterns in how students approached challenges and justified their solutions. To capture real-time insights into the educational experience, we also collected feedback during various project phases. The data analysis combined statistical evaluation of the survey results with thematic interpretation of observational data, allowing us to pinpoint key trends and areas for improvement. Additionally, to assess students’ learning efficiency, we employed both a competency-based assessment—measuring technical knowledge and problem-solving skills relevant to Industry 4.0 and 5.0—and an oral argumentative test, which required students to articulate their engineering decisions, demonstrate real-time problem-solving, and reflect on the practical application of theoretical knowledge. Descriptive statistical analysis was conducted, including the calculation of mean scores, standard deviations, and frequency distributions for each survey item. This allowed us to identify trends in student perceptions and measure performance against established benchmarks.
As demonstrated by Hincapié et al., leveraging digital twins and virtual commissioning has significantly enhanced traditional teaching methods. These implementations simplify the comprehension of technical knowledge, such as PLC programming and debugging of various logic and implementation errors, particularly because the costs associated with mistakes are prohibitively high (Hincapié et al., 2014). Furthermore, the availability of virtual scenarios has proven extremely beneficial in reducing the time from implementation. The practical application of digital twins in the course allowed students to simulate the complete production process, identify potential inefficiencies, and make informed adjustments before physical implementation. For example, students tested different automation flow designs in Tecnomatix Process Simulate, which provided insights into reducing human-cobot interaction times and optimizing workstation layouts for enhanced ergonomics. It also introduces a novel approach to teaching that accommodates different learning styles, including Kinesthetic, Kinesthetic/Visual, Visual, and Auditory (Hincapié et al., 2020) Students were tasked with first creating all virtual components of the challenge, including programming, integrating and downloading files from the different equipment suppliers (cobot, PLC, HMI, cameras, emergency stop, etc.), designing all the components for the manufacturing line (storages with bines, fixtures and jigs, spaces for the tooling, etc.), and validating the proposed layout (Figure 4). They developed simulations in Process Simulation where workers (1 for transporting the FrED for other subassembly and 1 for assembly operations) and the cobot interact preserving the working space for both. This involved ensuring safe distances, considering ergonomics, and defining security spaces according to ANSI RIA and Mexican safety standards (NOMs).
For the challenge, the students were commissioned with the assembly of the electronics by a custom designed electric gripper powered with Arduino Nano and mechanisms presented the following assembly precedence (Figures 5a,b), such as (1) the supply of materials to the station, (2) accurate positioning of the drivers and the RAMPS shield over the Arduino Mega, and (3) the placement of the power supply (12 V, 5 A) cables and screws. Through observation, it was noted that several issues, including the reordering of the layout, PLC program debugging, review of the automation logic (Figure 5c), and the monitoring of times to reduce cycle time by minimizing downtime, assisted the students in achieving the final solution in Process Simulate before transitioning to real-world application (Figure 5e). This aligns with the principles of Education 5.0, emphasizing the implementation of cyber-physical systems in virtual simulators, dominating Industry 4.0 technologies (Figure 5d) and making such programming accessible to all interested parties.
Figure 5. FrED electronics automation. (a) Operation tree and sequence for the electronics assembly, (b) assembly logics, (c) assembly precedence, (d) Industry 4.0 technologies used, and (e) assembly simulation in Tecnomatix Process Simulate.
According to Figure 5a, the following actions are broken down for manufacturing station:
1. Conveyor Task 1: Transporting the power supply.
2. Robot Task 1: Pick-and-place operation for the Arduino Mega.
3. Robot Task 2: Pick-and-place operation for the RAMPS 1.4.
4. Robot Task 3: Pick-and-place operation for drivers.
5. Inspection Task 1: Electronic components inspection with computed vision.
6. Operator Task 1: Pick-and-place operation for electrical Dupont cables.
7. Operator Task 2: Pick-and-place operation for electronic components onto the platform.
8. Operator Task 3: Connecting electronic components with the power supply.
9. Operator Task 4: Screwing in the power supply.
The implementation of Digital Twin technology within the Smart Factory environment allowed students to create and manipulate virtual models of the Fiber Extrusion Device (FrED). This facilitated real-time simulation and optimization of manufacturing processes. As demonstrated in Figure 5, students were able to identify and rectify inefficiencies in the assembly line, leading to a reduction in cycle time and an improvement in ergonomic safety measures. Figure 6 showcases the iterative approach, reflecting how students continuously improved manufacturing strategies for the placement of the electronic of the plastic extrusion machine. This sequence represents the process flow in both Process Simulate and the physical automation process. It begins with one worker placing the base structure (Figures 7a,e), followed by the conveyor delivering the power supply (Figures 7b,f). The worker then installs the components, places the cables, and secures the elements on the FrED (Figures 7c,g). Finally, once completed, the second worker collects the ready-to-use FrED (Figures 7d,h). The blend of real and simulated environments enabled students to fine-tune a strategy that enhanced human interaction within the Smart Factory lab’s manufacturing cell. Additionally, the essential role of Siemens’ Process Simulate software in the simulation and validation of robotics integration highlights the application of Industry 5.0 concepts. These simulations offered critical insights into the practicality and efficiency of incorporating cutting-edge technologies into tangible systems compared to the test-error with the physical equipment.
Figure 6. Digital twin simulations and physical integration. (a) and (e) Show the placement of the FrED from previous processes; (b) and (f) illustrate the supply of electronic elements from the conveyor; (c) and (g) depict the fixation of these elements by the worker; and (d) and (h) show the second worker retrieving the finished FrED.
By recognizing that working with cyber-physical will help to address emerging challenges such as effective collaboration, trustworthiness, and system complexity, to create human-centered and sustainable environments, capitalizing transdisciplinary frameworks to cover societal, engineering, and sustainability challenges together (Gürdür Broo et al., 2021). The present case study demonstrated an optimization of production processes with a strong emphasis on ergonomics and human-machine collaboration. By having first, the digital twin and simulations in Tecnomatix Process Simulate and the final order for automation, students began the automation process by integrating all elements into the manufacturing cell, as shown in Figure 7.
Firstly, the PLC was implemented as a central control unit where inputs such as infrared proximity sensors, push buttons and information from other devices are processed according to functions and logic configured by the user powered by 24VDC. Therefore, the PLC activates the outputs to power the Direct Current (DC) motor as actuators and communicate with other devices. For material handling in this process, the xArm6 collaborative robot was used to perform these tasks as mentioned above. Additionally, the xArm6 cobot was equipped with an advanced gripper in which an Arduino nano was used to control a servo motor (gripper controller). It is important to mention the students carried out the gripper’s design and implementation and the devices that integrate the manufacturing cell were linked to the Ethernet Module switch to generate a local network with a specific IP for each one.
To validate the result of the process, the artificial vision camera was integrated to carry out the inspection process. Thus, the camera verifies the correct assembly and the correct location of the parts. Additionally, students created and designed the user interface with an HMI, allowing the worker to navigate through different types of information about the process: device status, process flow, process information, and parameterization. Finally, a power supply was used to provide 24VDC to power the devices that integrate into the manufacturing cell. Also, a DC/DC converter was employed to give 5VDC for microprocessor unit for the advanced gripper. The integration of cyber-physical systems within the manufacturing cell provided a hands-on platform for students to engage with collaborative robots (cobots) and programmable logic controllers (PLCs).
Through observation by the teaching team, it was noted that students needed to: create training management for the worker (one student) since, when the process was video recorded, the student had errors and mistakes; plan several subroutines to reduce monotony in the worker’s activities; and conduct assembly tests to increase efficiency and save time during the worker’s tasks. Additionally, it was important to allocate time to students for their own processes and, in the event of scaling to other classrooms, develop an easy way to make connections on the PLC and handle the I/O. Furthermore, even though the students planned their activities using a Gantt chart, it is suggested to establish very specific goals for each period and monitor continuous process improvement through an automated activity system and give the students space to discuss their ideas and make decisions.
On the other hand, the analysis of the study’s findings and their implications for future educational practices can be succinctly presented as follows:
• Evolution toward Education 5.0: Integrate more aspects of Education 5.0, focusing on worker wellbeing and the incorporation of emotions into creating dignified work environments. This aligns with the Sustainable Development Goals (SDGs) related to health and wellbeing and dignified work and equality of opportunities.
• Sustainable Development Goals (SDGs) inclusion: The course aligns with the Sustainable Development Goals by enhancing quality education (SDG 4) through skills-based learning with Industry 4.0 technologies, improving students’ technical and analytical abilities. It also supports industry, innovation, and infrastructure (SDG 9) by teaching students to design and implement automation solutions and promotes reduced inequalities (SDG 10) by providing equal access to educational tools, preparing all students for a competitive, technologically advanced job market.
• Enhancing understanding through VR: Implement VR simulators to allow students to interact directly with the FrED system and its components.
• Strengthening project management: Define communication channels, secure proficiency of control and design change management.
A descriptive statistical analysis was performed on the scores obtained from 40 students enrolled in the class for both activities, assessing competency mastery (C1–C7) as presented in Table 4. In Evidence 1, the perfect median score and low variability highlight consistent success in evaluating and implementing advanced automation technologies. In Evidence 2, students demonstrated high proficiency in analyzing system interactions and proposing technological solutions, as reflected in the high average scores.
The results and feedback gathered from the Student Opinion Survey from Tec known as ECOA offer insightful reflections from students who participated in this immersive experience, highlighting the impactful learning outcomes and personal growth achieved through the course. Incorporating the ECOA student evaluation of the professor at the end of the Course at Tecnologico de Monterrey into our analysis, the feedback previously summarized aligns remarkably with the principles of Education 5.0, particularly in its focus on challenge-based learning, personal growth, and industry readiness. In the specific query, “The professor challenged me to give my best (develop new skills, new concepts and ideas, think differently, etc.),” students assigned a high rating of 9.65 out of 10, with a standard deviation of 1.26, echoing the core tenets of Education 5.0 that advocate for personalized, challenge-based, and lifelong learning approaches. This educational model emphasizes the importance of adapting to students’ needs, an aspect students highlighted in praising the instructor’s human-first approach, summarized aptly as “first human and then teacher.” The methodological integration and cutting-edge resources and international collaboration not only prepared students to be competitive but also instilled a motivation for excellence and continuous learning, qualities essential for navigating the complexities of the modern workforce. Feedback such as “worthy of praise” and “excellent professor” encapsulates the course’s transformative impact, demonstrating a strong correlation between the instructor’s challenge-based pedagogy and the innovative, holistic educational paradigm proposed by Education 5.0. This approach not only creates technical skill proficiency, personal development, and industry preparedness but also profoundly impacts students’ readiness for future challenges, marking a significant stride toward realizing the ambitious goals of Education 5.0. High ratings in Instructor Expertise 9.35 and Learning Environment 9.08 reflect a positive and supportive classroom atmosphere. Slightly lower scores in Instructor Feedback 8.89 suggest areas for improvement in providing timely and detailed feedback (see Table 5).
This challenge serves as an inspiring opportunity to develop enjoyable experiences that captivate students’ attention and commitment toward a unified goal: the production of a FrED. Such an endeavor closely mirrors potential future challenges in the actual industrial sector. This initiative can be adapted to various environments, and, with software support, it can even proceed without complete access to Industry 4.0 technologies, by having a remote factory where the students 5.0 can run factory simulations within university settings. Moreover, it offers a framework that companies could adopt to test automation strategies before full-scale implementation. In essence, it provides universities with secure platforms for industry collaboration, where students are empowered to devise innovative solutions for diverse manufacturing challenges.
Furthermore, the positive feedback regarding the development of soft skills highlights the effectiveness of our holistic educational approach. By fostering teamwork, communication, and problem-solving abilities, we prepare students for the collaborative and dynamic nature of modern industrial environments (Jerman et al., 2018). The alignment with SDGs reinforces the societal relevance of our educational model, ensuring that students are not only technically proficient but also socially responsible and ethically aware. This dual focus addresses the growing demand for engineers who can contribute to sustainable and inclusive industrial practices (Beier et al., 2021). Our findings also suggest that the Student 5.0 framework effectively bridges the gap between theoretical knowledge and practical application, facilitating a seamless transition from academia to industry. This is particularly pertinent as industries increasingly seek graduates who are adept at navigating complex technological landscapes and can lead innovation initiatives (Lu, 2017; Nosalska et al., 2020). Future research should explore the scalability of our model across different educational institutions and its long-term impact on student career trajectories. Additionally, integrating emerging technologies such as Virtual Reality (VR) and Augmented Reality (AR) could further enhance the immersive learning experience, providing even more robust training platforms for students.
Although the findings highlight notable advancements in developing Industry 5.0 competencies, several biases may limit the broader applicability of these results. First, the study drew on a relatively small group of students who were already highly motivated, which could lead to more favorable evaluations than those seen in a larger or more diverse population (Tashakkori and Teddlie, 2010). Second, the course was conducted at a single institution with specific technological and facilities resources, making its replication in other contexts potentially challenging. Furthermore, relying on self-reported surveys at the course conclusion can introduce social desirability bias, where participants might rate their experience more positively or respond in ways they believe are expected. Finally, a Hawthorne effect may have emerged, as students knew they were participating in a novel educational initiative and could have changed their behavior accordingly. Recognizing these limitations can guide future research toward larger, more varied samples, as well as additional mixed-methods assessments (e.g., longitudinal studies, focus groups) to enrich the robustness and generalizability of these outcomes.
The immersive design and compressed timeframe of the course may not fully capture how competencies evolve over longer academic periods or in more diverse, real-world manufacturing contexts spanning multiple industry types. While the specialized equipment and software reflect state-of-the-art technology, their availability is not universal; institutions or regions with fewer resources may face challenges in replicating these conditions (Mourtzis et al., 2018). Moreover, the study design did not incorporate a control group or a longitudinal follow-up, thereby limiting the capacity to assess sustained learning effects and to isolate the influence of the redesigned curriculum from other factors. Because industry feedback was gathered primarily from the course’s direct stakeholders, broader validation from external industrial partners—along with cross-institutional comparisons—would bolster generalizability and practical relevance. Future research might also investigate the scalability of this model in low-resource environments or with a more diverse student population to better understand the adaptability of this approach.
In conclusion, this case study is a compelling example of how educational programs are evolving to impart students with the future-oriented skills demanded by Industry 5.0. By immersing students in a transformative educational experience focused on hands-on learning and virtual simulations, this study effectively imparts future-oriented skills essential for navigating modern industrial environments. The tangible outcomes, evidenced by the acquisition of specific skills such as PLC programming, robotics integration, and HMI design, underscore the practical application of the competencies. Our work conclusions highlight the following:
• The redesign of the Automation of Manufacturing Systems course addresses the need for practical skills aligned with Industry 4.0 demands and the principles of Education 5.0.
• The course integrates hands-on experiences with IoT, AI, and collaborative robotics, ensuring students are equipped for real-world applications in smart manufacturing.
• The concept of Student 5.0 promotes a holistic approach to education, emphasizing not only technical competencies but also social, methodological, and personal skills essential for collaboration and innovation.
This study exemplified the requirements of Industry 5.0 and prepared Student 5.0 participants to thrive in a more dynamic and proactive educational approach, with positive feedback from students confirming the achievement of the stated objectives. Additionally, the qualitative analysis of the competence assessment confirmed that students were fully immersed and successfully acquired both the technical knowledge and soft skills included in this course. Our educational model offers a scalable framework for engineering institutions to incorporate advanced technologies and sustainability principles into their curricula. Graduates equipped with both technical and socio-emotional skills are better prepared to lead and innovate in technologically advanced and sustainable workplaces. Open problems and future research include investigating the scalability of our educational model across different institutions and industrial contexts, assessing the long-term impact on student career trajectories and industry performance, exploring the integration of emerging technologies like Virtual Reality (VR), Augmented Reality (AR), and Artificial Intelligence (AI) to enrich learning experiences, and extending our framework to other engineering disciplines to address complex industrial challenges through interdisciplinary collaboration. Moreover, the emphasis on human-centered manufacturing in the educational journey highlights the pivotal role in enhancing competitiveness in an organizational context.
Future studies aiming to replicate or extend this educational model might be guided by the following research questions:
1. How does an immersive Smart Factory environment influence the development and retention of core Industry 5.0 competencies among engineering students over time? Exploring both immediate and long-term skill retention would clarify how rapidly evolving industries can be integrated into curricula while maintaining knowledge longevity.
2. Which elements of the integrated Education 5.0 framework most effectively bridge theoretical knowledge and practical application? Identifying specific pedagogical strategies—such as digital twins, virtual reality, or adaptive feedback—would help educators refine course design for maximum impact.
3. What factors influence the transferability and success of this model across different universities and resource-constrained environments? Investigating variables like technology availability, local industry partnerships, and institutional support may shed light on how to adapt the approach without compromising educational quality.
4. To what extent do these acquired Industry 5.0 competencies enhance graduates’ employability, adaptability, and contributions to sustainable industrial practices? Longitudinal tracking of alumni outcomes could reveal how effectively students translate their training into professional settings that prioritize sustainability and human-centered manufacturing.
The raw data supporting the conclusions of this article will be made available by the authors without undue reservation.
ER-C: Conceptualization, Data curation, Formal analysis, Investigation, Methodology, Project administration, Supervision, Validation, Visualization, Writing – original draft, Writing – review & editing. MA-R: Formal analysis, Investigation, Methodology, Project administration, Software, Validation, Writing – original draft. JG-Á: Conceptualization, Formal analysis, Investigation, Methodology, Project administration, Writing – original draft, Writing – review & editing. DQ-S: Conceptualization, Data curation, Formal analysis, Investigation, Methodology, Software, Validation, Writing – original draft, Writing – review & editing. AO-E: Conceptualization, Data curation, Formal analysis, Investigation, Methodology, Project administration, Supervision, Writing – original draft, Writing – review & editing. AV-M: Conceptualization, Funding acquisition, Resources, Visualization, Writing – review & editing.
The author(s) declare that financial support was received for the research and/or publication of this article. The research was possible with the financial support of the Tecnologico de Monterrey Mechatronics Department and the Institute of Future Education through the Writing Lab.
The authors acknowledge the support of the Massachusetts Institute of Technology through the FrED Factory Lab, with special thanks to Brian Anthony, Russel Bradley and José Pacheco for their invaluable contributions. The authors acknowledge the financial and technical support of the Writing Lab of the Institute for the Future of Education at Tecnológico de Monterrey.
The authors declare that the research was conducted in the absence of any commercial or financial relationships that could be construed as a potential conflict of interest.
The handling editor PC declared a shared affiliation with the authors at the time of review.
All claims expressed in this article are solely those of the authors and do not necessarily represent those of their affiliated organizations, or those of the publisher, the editors and the reviewers. Any product that may be evaluated in this article, or claim that may be made by its manufacturer, is not guaranteed or endorsed by the publisher.
Baena, F., Guarin, A., Mora, J., Sauza, J., and Retat, S. (2017). Learning factory: the path to industry 4.0. Procedia Manuf. 9, 73–80. doi: 10.1016/J.PROMFG.2017.04.022
Beier, G., Niehoff, S., and Hoffmann, M. (2021). Industry 4.0: a step towards achieving the SDGs? A critical literature review. Discov. Sustain. 2, 1–21. doi: 10.1007/S43621-021-00030-1
Chryssolouris, G., Mavrikios, D., and Rentzos, L. (2016). The teaching factory: a manufacturing education paradigm. Proc. CIRP 57, 44–48. doi: 10.1016/J.PROCIR.2016.11.009
Content of Automation of Manufacturing Systems Course (2019). Available online at: https://samp.itesm.mx/Materias/VistaPreliminarMateria?clave=MR2007B&lang=EN (Accessed July 19, 2024).
Culot, G., Nassimbeni, G., Orzes, G., and Sartor, M. (2020). Behind the definition of industry 4.0: analysis and open questions. Int. J. Prod. Econ. 226:107617. doi: 10.1016/J.IJPE.2020.107617
Farinha, L. (2012). Triple Helix conference. 10TH 2012. Emerging triple Helix models for developing countries: from conceptualization to implementation, 324.
Froyd, J. E., Wankat, P. C., and Smith, K. A. (2012). Five major shifts in 100 years of engineering education. Proc. IEEE 100, 1344–1360. doi: 10.1109/JPROC.2012.2190167
Fuertes, J. J., Prada, M. Á., Rodríguez-Ossorio, J. R., González-Herbón, R., Pérez, D., and Domínguez, M. (2021). Environment for education on industry 4.0. IEEE Access 9, 144395–144405. doi: 10.1109/ACCESS.2021.3120517
Garg, A., Babu, B. V., and Balas, V. E. (Eds). (2024). Advances in Technological Innovations in Higher Education: Theory and Practices (1st ed). CRC Press. doi: 10.1201/9781003376699
Green, S. A., Billinghurst, M., Chen, X., and Chase, J. G. (2008). Human-robot collaboration: a literature review and augmented reality approach in design. Int. J. Adv. Robot. Syst. 5, 1–18. doi: 10.5772/5664
Gürdür Broo, D., Boman, U., and Törngren, M. (2021). Cyber-physical systems research and education in 2030: Scenarios and strategies. J Ind Inf Integr. 21:100192. doi: 10.1016/J.JII.2020.100192
Hincapié, M., de Jesús Ramírez, M., Valenzuela, A., and Valdez, J. A. (2014). Mixing real and virtual components in automated manufacturing systems using PLM tools. Int. J. Interact. Des. Manuf. 8, 209–230. doi: 10.1007/S12008-014-0206-7
Hincapié, M., Valdez, A., Güemes-Castorena, D., and Ramírez, M. (2020). Use of laboratory scenarios as a strategy to develop smart factories for industry 4.0. Int. J. Interact. Des. Manuf. 14, 1285–1304. doi: 10.1007/S12008-020-00696-3
Huang, S., Wang, B., Li, X., Zheng, P., Mourtzis, D., and Wang, L. (2022). Industry 5.0 and society 5.0—comparison, complementation and co-evolution. J. Manuf. Syst. 64, 424–428. doi: 10.1016/J.JMSY.2022.07.010
Hurtado, C. V., Castro, O. J. L., De La Garza Inzunza, E. E., and Saravia, R. G. (2023). Applying the lean manufacturing tools 5S and 7 wastes for the learning and development of undergraduate students. IEEE global engineering education conference, EDUCON 2023-May.
Ivanov, D. (2023). The Industry 5.0 framework: viability-based integration of the resilience, sustainability, and human-centricity perspectives. Int. J. Prod. Res. 61, 1683–1695. doi: 10.1080/00207543.2022.2118892
Jäger, A., Mayrhofer, W., Kuhlang, P., Matyas, K., and Sihn, W. (2012). The “learning factory”: an immersive learning environment for comprehensive and lasting education in industrial engineering. Proceedings of the 16th world multi-conference on systemics, cybernetics and informatics, 237–242.
Jerman, A., Bach, M. P., and Bertoncelj, A. (2018). A bibliometric and topic analysis on future competences at smart factories. Mach. Des. 6:41. doi: 10.3390/MACHINES6030041
Kamalov, F., Santandreu Calonge, D., and Gurrib, I. (2023). New era of artificial intelligence in education: towards a sustainable multifaceted revolution. Sustain. For. 15:12451. doi: 10.3390/SU151612451
Kasinathan, P., Pugazhendhi, R., Elavarasan, R. M., Ramachandaramurthy, V. K., Ramanathan, V., Subramanian, S., et al. (2022). Realization of sustainable development goals with disruptive technologies by integrating Industry 5.0, Society 5.0, smart cities and villages. Sustain. For. 14:15258. doi: 10.3390/SU142215258
Kim, J., Lee, H., and Cho, Y. H. (2022). Learning design to support student-AI collaboration: perspectives of leading teachers for AI in education. Educ. Inf. Technol. (Dordr) 27, 6069–6104. doi: 10.1007/S10639-021-10831-6
Klotzer, C., Weibenborn, J., and Pflaum, A. (2017). “The evolution of cyber-physical systems as a driving force behind digital transformation” in Proceedings − 2017 IEEE 19th conference on business informatics, CBI 2017, 5–14.
Kochmar, E., Do Vu, D., Belfer, R., Gupta, V., Serban, I. V., and Pineau, J. (2020). Automated personalized feedback improves learning gains in an intelligent tutoring system. Artif. Intell. Educ. 12164:140. doi: 10.1007/978-3-030-52240-7_26
Lantada, A. D., and Lantada, A. D. (2022). “Engineering education 5.0: strategies for a successful transformative project-based learning” in Insights into global engineering education after the birth of Industry 5.0. IntechOpen. doi: 10.5772/intechopen.102844
Lantada, A., Muñoz-Guijosa, J. M., Tanarro, E. C., Otero, J. E., and Sanz, J. L. M. (2016). Engineering education for all: strategies and challenges. Int. J. Eng. Educ. 32. Available at: https://www.ijee.ie/latestissues/Vol32-5B/02_ijee3303ns.pdf
Li, R. (2023). Design and manufacturing of the filament collection and diameter measurement systems of fiber extrusion device for educational purposes. Available online at: https://dspace.mit.edu/handle/1721.1/147910 (Accessed April 6, 2024).
Liu, C., Solis, S. L., Jensen, H., Hopkins, E., Neale, D., Zosh, J., et al. (2017). Neuroscience and learning through play: A review of the evidence (research summary). The LEGO Foundation, DK.
Lu, Y. (2017). Industry 4.0: a survey on technologies, applications and open research issues. J. Ind. Inf. Integr. 6, 1–10. doi: 10.1016/J.JII.2017.04.005
Mian, S. H., Salah, B., Ameen, W., Moiduddin, K., and Alkhalefah, H. (2020). Adapting universities for sustainability education in industry 4.0: channel of challenges and opportunities. Sustain. For. 12:6100. doi: 10.3390/SU12156100
Mourtzis, D., Vlachou, E., Dimitrakopoulos, G., and Zogopoulos, V. (2018). Cyber-physical systems and education 4.0 –the teaching factory 4.0 concept. Proc. Manuf. 23, 129–134. doi: 10.1016/J.PROMFG.2018.04.005
Mukul, E., and Büyüközkan, G. (2023). Digital transformation in education: a systematic review of education 4.0. Technol. Forecast Soc. Change 194:122664. doi: 10.1016/J.TECHFORE.2023.122664
Nosalska, K., Piątek, Z. M., Mazurek, G., and Rządca, R. (2020). Industry 4.0: coherent definition framework with technological and organizational interdependencies. J. Manuf. Technol. Manag. 31, 837–862. doi: 10.1108/JMTM-08-2018-0238
Pinzone, M., Fantini, P., Perini, S., Garavaglia, S., Taisch, M., and Miragliotta, G. (2017). Jobs and skills in industry 4.0: an exploratory research. IFIP Adv. Inf. Commun. Technol. 513, 282–288. doi: 10.1007/978-3-319-66923-6_33
Popova-Nowak, I. V., and Cseh, M. (2020). The relationship between knowledge management and organizational learning with academic staff readiness for education 4.0. Eurasian J. Educ. Res. 14, 299–331. doi: 10.1177/1534484315596856
Prieto, M. D., Sobrino, Á. F., Soto, L. R., Romero, D., Biosca, P. F., and Martínez, L. R. (2019). Active learning based laboratory towards engineering education 4.0. IEEE international conference on emerging technologies and factory automation, ETFA 2019-September, 776–783.
Quintero-Sanchez, D. I., de la Garza-Inzunza, E. E., García-Avila, J., and Ramirez-Cedillo, E. (2024). Collaborative smart manufacturing with process operation diagrams: a case study from Tec’s smart factory. IEEE international conference on industrial engineering and engineering management, 1257–1261.
Rodriguez-Padilla, C., Vazquez-Hurtado, C., and Ramirez-Cedillo, E. (2023). Manufacturing cell framework based on TEC21 educational model: a successful case of study. 2023 IEEE IFEES world engineering education forum and global engineering deans council: convergence for a better world: A call to action, WEEF-GEDC 2023- proceedings.
Rüßmann, M., Lorenz, M., Gerbert, P., Waldner, M., Justus, J., Engel, P., et al. (2015). Industry 4.0: the future of productivity and growth in manufacturing industries. Boston Consulting Group, 9, 54–89.
Tashakkori, A., and Teddlie, C. (2010). Putting the human back in “human research methodology”: the researcher in mixed methods research. J. Mix Methods Res. 4, 271–277. doi: 10.1177/1558689810382532
Keywords: Industry 5.0, Education 5.0, Society 5.0, Student 5.0, Automation, Smart Factory, Manufacturing Systems, Innovation in Education
Citation: Ramírez-Cedillo E, Armendáriz-Rodríguez M, García-Ávila J, Quintero-Sánchez D, Ortiz-Espinoza AA and Vargas-Martínez A (2025) Student 5.0: immersive learning in next-gen Automation of Manufacturing Systems courses for the Industry 5.0 era. Front. Educ. 10:1416761. doi: 10.3389/feduc.2025.1416761
Received: 13 April 2024; Accepted: 14 March 2025;
Published: 01 April 2025.
Edited by:
Patricia Caratozzolo, Institute for the Future of Education, Tecnologico de Monterrey, MexicoReviewed by:
Andra Diaconescu, Politehnica University of Timișoara, RomaniaCopyright © 2025 Ramírez-Cedillo, Armendáriz-Rodríguez, García-Ávila, Quintero-Sánchez, Ortiz-Espinoza and Vargas-Martínez. This is an open-access article distributed under the terms of the Creative Commons Attribution License (CC BY). The use, distribution or reproduction in other forums is permitted, provided the original author(s) and the copyright owner(s) are credited and that the original publication in this journal is cited, in accordance with accepted academic practice. No use, distribution or reproduction is permitted which does not comply with these terms.
*Correspondence: Erick Ramírez-Cedillo, ZXJpY2tyYW1jZUB0ZWMubXg=
Disclaimer: All claims expressed in this article are solely those of the authors and do not necessarily represent those of their affiliated organizations, or those of the publisher, the editors and the reviewers. Any product that may be evaluated in this article or claim that may be made by its manufacturer is not guaranteed or endorsed by the publisher.
Research integrity at Frontiers
Learn more about the work of our research integrity team to safeguard the quality of each article we publish.