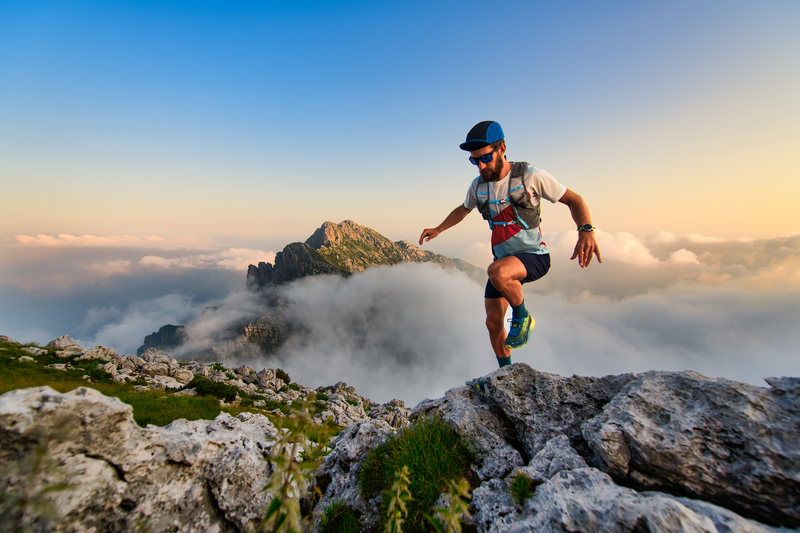
95% of researchers rate our articles as excellent or good
Learn more about the work of our research integrity team to safeguard the quality of each article we publish.
Find out more
SYSTEMATIC REVIEW article
Front. Educ. , 07 February 2025
Sec. Higher Education
Volume 9 - 2024 | https://doi.org/10.3389/feduc.2024.1492308
This article is part of the Research Topic AI's Impact on Higher Education: Transforming Research, Teaching, and Learning View all 9 articles
In an era marked by technological sophistication, Artificial Intelligence (AI) is increasingly being integrated into various fields, including Mechanical Engineering Education (MEE). This review paper presents a systematic examination of scientific publications in this field, spanning from 2018 to 2023. Utilizing the PRISMA framework, 228 research papers were selected and analyzed to identify research gaps and future directions in AI’s application within the MEE discipline. The diverse applications of AI in MEE identified include personalized learning, smart tutoring systems, digitizing engineering drawings, enhancing simulation and assessment, and boosting student motivation and engagement. Additionally, a bibliometric analysis of AI in MEE was conducted, examining its role in different aspects of MEE, interdisciplinary collaboration, geographic distribution, and research focus. Accordingly, the scope of this review encompasses a comprehensive content analysis and bibliometric evaluation of AI applications in MEE. This review systematically identifies current applications of AI, maps research trends, and analyzes publication data to highlight interdisciplinary collaborations and geographical distributions. Furthermore, this study identifies critical research gaps and offers actionable recommendations, emphasizing future directions such as advancing Generative Artificial Intelligence (GAI) applications in MEE and reshaping curricula to integrate AI-based learning tools. The findings provide valuable insights to support stakeholders in evolving MEE to meet industry needs and enhance educational outcomes.
Mechanical Engineering (ME) is a vast field, encompassing a wide range of disciplines such as mechanics, robotics, manufacturing, additive manufacturing (AM), aerospace, and computer-aided design (CAD). ME involves applying engineering principles and methods to solve real-world problems, from the initial stages of design and creation to the introduction of objects into the real world (Prabhu, 2019). Mechanical engineers critically evaluate their work using principles of motion, energy, and force, ensuring that their designs are safe, reliable, and effective. The significance of ME lies in its impact; mechanical engineers address various needs by developing technologies tailored to specific requirements. They are problem solvers who find solutions to challenges across multiple fields, including transportation, climate change, world hunger, healthcare, and more. This versatility is reflected in Mechanical Engineering Education (MEE), which plays a pivotal role in the innovations and challenges of various disciplines. Mechanical Engineers can design a wide array of machines, systems, and processes, from the smallest components to large-scale projects. Consequently, mechanical engineers need a diverse skill set, including problem-solving, creativity, and experiential skills (Prabhu, 2019). As technology evolves, MEE must provide students with an education that aligns with digital advancements, preparing them for the workforce. Students should be equipped with the necessary skills to navigate and address future challenges effectively.
The advent of AI has the potential to significantly facilitate the acquisition of key skills in MEE. By transforming MEE on various levels, AI enhances the educational experience and lessens the burden for both educators and students. It accomplishes this by offering sophisticated facilities such as personalized learning experiences, gamification of the learning process, and the digitalization of educational resources. AI is capable of personalizing education by monitoring students’ performance, providing feedback, offering interfaces for human-computer interaction, and delivering suitable tasks (Zhai et al., 2021). However, the introduction of AI also brings forth concerns about academic integrity, student motivation and engagement, the need for more personalized learning, improved accuracy in engineering drawings and simulations, fault diagnosis in systems, digitization of engineering drawings, assessment, classification, automation of simulations, and the creation of safer learning environments (Cai et al., 2021).
This study is therefore dedicated to exploring and presenting a holistic perspective on the applications of AI in MEE. It achieves this through an in-depth examination of scientific publications focused on this area. By synthesizing and summarizing key findings, methodologies, and recommendations from a broad range of papers, this study offers a valuable resource for researchers, educators, and policymakers who are keenly interested in the integration of AI into MEE. This review paper contributes to the MEE field in several significant ways. First, it provides an extensive overview of the current state of AI in MEE, enabling researchers to identify prevalent themes and research trends within the field. Second, it combines findings and insights from numerous studies, offering a comprehensive perspective on the efficacy of AI in MEE. Additionally, this review pinpoints research gaps and areas that warrant further investigation, thereby guiding future research initiatives.
The methodology used for selecting and analyzing scholarly articles will be elaborated upon in the next section of this study. Subsequently, a detailed content analysis and synthesis of the findings and insights will be presented, highlighting various themes, trends, and prospective research pathways. Moreover, a bibliometric study will be carried out, examining publications related to AI in MEE, the extent of cross-disciplinary collaboration, and the geographical spread of research activities. In conclusion, this review will emphasize the significance of the consolidated findings and recommend future research avenues to propel the AI in MEE field forward.
The research methodology used in this study adopts a structured strategy to collect and examine literature related to the incorporation of AI into MEE. This involves four main stages, as illustrated in Figure 1 and outlined as follows;
This phase involves the identification of relevant search terms and keywords to thoroughly identify significant publications pertinent to the chosen subject, marking the first and critical step in the data collection phase. A collection of existing articles and publications within the AI in MEE sphere was gathered from the Scopus database due to its credibility that results from its comprehensive coverage, quality control, global reach, frequent updates, and accessibility. Through the employment of a set of keywords, including “Mechanical,” “Engineering,” “Education,” “Artificial Intelligence,” “Machine learning,” “Neural network,” “Deep Learning,” and “Augmented reality,” “Computer vision,” “Data Mining,” “Computer Aided Design,” “AI,” “Predictive Analytics,” “Natural Language Processing,” “Computational Linguistics,” “CODEX,” “Reinforcement learning,” and “Chatbots,” the researchers performed a focused search through title, abstract, and keyword sections. This endeavor led to the compilation of 765 papers, covering the period from 2018 to 2023.
The procedure for reviewing literature in this study was influenced by the PRISMA guidelines, acknowledged for their comprehensive and clear methodology in the execution of systematic reviews and meta-analyses (Figure 1). The PRISMA framework provides a systematic approach for the identification, selection, and critical assessment of relevant studies, ensuring the review’s credibility and the ability to replicate its findings (Anon.). Initially, a total of 765 papers were gathered. After removing duplicate entries, 523 documents remained, consisting of 359 conference contributions and 164 review articles, book chapters, and scholarly papers. We then conducted a meticulous evaluation of each document, carefully selecting only those papers that aligned with the study’s objectives and met quality standards. This process led to the exclusion of studies not relevant to our research focus. Ultimately, our final selection included 228 works—comprising 140 conference contributions and 88 review articles, book chapters, and scholarly papers—spanning from 2018 to October 2023, ensuring a robust and relevant dataset for our analysis. Figure 2 displays the incremental growth in the quantity of papers throughout the mentioned timeframe. Scopus database was chosen due to its comprehensive coverage across diverse research fields including engineering, in addition to, its essential role as a reliable source of scientific information.
It functions as a methodical assessment of scholarly literature, primarily through the examination of citations and references within research papers. The 228 papers that were chosen during the literature screening process are encompassed in this bibliometric analysis which includes 88 review papers, book chapters, and journal articles and 140 conference papers. This bibliometric approach allows researchers to systematically examine the impact, trends, and interconnections among scholarly publications, providing insights into the development of research themes and collaborative networks within the field. By analyzing citation patterns, co-authorship relationships, and keyword frequencies, bibliometric analysis helps to identify influential authors, foundational studies, emerging research areas, and collaborative trends. This method thus offers a comprehensive view of the field’s intellectual structure and the evolution of its major themes. In this review, we used co-citation, co-authorship, and co-word maps as key methods to highlight the relationships between studies, authors, and topics. To implement these analyses, VOSviewer was utilized to automatically generate occurrence and co-occurrence matrices, applying similarity measures (e.g., association strength) and post-hoc clustering to group related research areas. This combined approach not only visualizes research clusters but also identifies essential figures and studies, offering a detailed map of how AI is integrated into MEE. These methods were selected to reveal both the breadth of topics covered and the intensity of research collaborations, ensuring a comprehensive and insightful analysis of the literature (Chen H. et al., 2023).
This phase involves the meticulous review and systematic organization of extensive information, such as scholarly articles, to discern prevalent themes and patterns. A further selection was carried out prior to the content analysis, retaining the most pertinent studies, with a significant proportion being journal articles (50 out of 88 journal papers and 36 out of 140 conference papers). The papers chosen for content analysis predominantly explored AI integrations within MEE, representing the most relevant works pertinent to the objectives of this review. The methodology entailed the classification and thematic grouping of the research articles, facilitating a deeper understanding of AI’s influence on education. This structured approach aids in synthesizing key insights and drawing meaningful conclusions from the amassed data. Our content analysis was conducted through a thematic approach, where we manually reviewed and categorized each selected paper. This process involved identifying recurring themes and subthemes by carefully analyzing the content and focus of each study. This manual approach allowed for a nuanced interpretation, ensuring that the themes accurately represented the insights from the reviewed literature.
The following two sections of this paper present a bibliometric analysis, and a content analysis following the methodological steps described in this section.
In this section, an in-depth bibliometric examination is conducted, focusing on AI within the MEE framework. An improved understanding of the current research and development landscape in AI is pursued by analyzing a wide range of articles and studies, numbering 228 in total, sourced from the Scopus database. Additionally, this analysis is aimed at extracting critical insights, identifying emerging trends, and discerning the broader impacts of AI’s integration into MEE through a meticulous review and evaluation of the literature. Through the utilization of the VOS viewer tool, a nuanced understanding of AI’s current standing in MEE is offered through four distinct types of visual representations. These visual aids, featuring circles each symbolizing a specific research focus, with their size indicating the intensity of activity within that domain, are provided. Moreover, the spatial proximity between circles is signified as the strength of their interrelations, providing a visual image for the interconnected nature of AI research within MEE.
By analyzing the text data from 228 publications selected through literature screening, the most relevant and frequently occurring terms were identified. This analysis, focusing on the titles and abstracts of these publications, aimed to isolate significant terms and establish a network of co-occurrence links among them. Through this process, it was possible to highlight emerging developments and pinpoint the most influential terms in the realm of smart technologies in MEE. From the data processed by VOSVIEWER, a total of 6,534 terms were generated, out of which 104 terms were selected based on a minimum occurrence threshold of 10. Following this, VOSVIEWER calculated the relevance scores for these terms, selecting the top 60% as the most relevant. As a result, 62 terms were illustrated on the map, as shown in Figure 3. According to Eck and Waltman (2018) Terms with high relevance scores were indicative of more specific subjects within the text data, whereas terms with low relevance scores were generally associated with broader concepts. Nonetheless, as demonstrated in Figure 3, the results have revealed a wide variety of research areas within the integration of artificial intelligence in MEE. The interconnected network of key terms indicates that the study of AI within MEE covers a broad spectrum of technological aspects.
As shown in Figure 3, various clusters reveal connections between different themes, the green cluster focus on “technology,” which is closely associated with several key terms, including “Artificial Intelligence” “Robot” “Augmented Reality” “Machine Learning” and also extends to the educational field with terms like “educator” “feedback” “teaching.” This emphasizes a technological focus within MEE literature, suggesting that AI and related technologies play a vital role in advancing the field. Moreover, the direct link between “technology” and “industry” further implies the necessity for MEE programs to align with industry trends, underscoring the importance of preparing students for the workforce through a technology-integrated curriculum. Furthermore, as illustrated in Figure 4, a detailed view of the connections with “technology” is demonstrated, emphasizing the strong connections that “technology” has with other terms across educational and technical aspects. The red cluster focuses on educational themes, with terms such as “teaching,” “active learning,” and “feedback” underscoring on the pedagogical approaches within MEE. Terms like “skill” and “curriculum” in the yellow cluster suggest an ongoing emphasis on developing student competencies and designing curricula that reflect both technological advancements and industry needs.
Figures 3, 4 show how research in MEE intersects technology and education, showing a balance between integrating advanced tools, such as AI, AR, and ML, and enhancing teaching methodologies. The clustering terms demonstrates the multidisciplinary nature of MEE, highlighting the potential of AI and related technologies to transform educational approaches and meet industry expectations. These insights offer a comprehensive view of the literature around the topic and emphasize the potential of technological integration in engineering education.
As shown in Table 1, the terms presented along with their rankings, frequency of occurrences, and relevance scores. Accordingly, it is demonstrated that technology occupies the first ranking with “244” occurrences and “0.4” relevance score, followed by model, and data as the top 3 in the table.
As depicted in Figure 5, the keyword “technology” appears as a central node, with numerous connections extending to related terms, indicating its foundational role in discussions on AI applications. Key terms such as “skill” (yellow cluster) and “accuracy” (green cluster) demonstrate strong associations with technology and AI, revealing that these areas are frequently explored in the context of AI-enhanced skill development and precision in engineering education. In addition, the green cluster involves terms like “machine,” “model,” and “learning,” which connects directly to “technology.” This suggests a focus on ML models and technological frameworks used for educational purposes in MEE. While, the yellow cluster emphasizes “skill” development, underscoring the educational applications of AI to enhance students’ practical and theoretical skills, which are essential in ME fields. Finally, the red and blue clusters represent other themes, such as “robotics” and “curriculum” that are also connected to AI, demonstrating its broader impact across various areas of engineering education.
This visualization highlights the role of AI as a bridge between industry and academia. By linking technology, skill development, and accuracy, AI fosters enhanced learning experiences and prepares students for industry demands. Future investigators and educators can utilize this map to identify well-explores areas, like technology-centered skill enhancement, and to recognize emerging topics that may benefit from further research, such as robotics integration and curriculum innovation. All in all, this figure provides a comprehensive overview of how AI intersects with several educational and industrial themes in MEE, making it a valuable tool for understanding the scope and focus of current research in this field.
By analyzing the bibliographic data from the 228 chosen publications, as illustrated in Figure 6, a total of 2,165 keywords were discovered, from which 50 keywords were selected based on their frequency. A minimum threshold of six occurrences was set for keyword selection. The map displays two categories of keywords: authors’ keywords and index keywords. Authors’ keywords are those specified by the authors of the publications, while index keywords are created by indexes or databases to organize and categorize articles for the purposes of information retrieval and indexing.
As illustrated in Figure 6 and Table 2, “Engineering education” emerged as the keyword with the highest frequency (174 occurrences) and total link strength (725), indicating a strong connection with other keywords in the field. The total link strength here refers to the overall intensity of connections a keyword has, providing insight into its prominence and centrality within the research landscape. This central position indicates that “Engineering education” is a focal point of studies including AI and MEE, bridging multiple research topics and subfields. Following “Engineering education,” “students” and “curricula” are revealed as the second and third most frequent keywords, respectively. This highlights a prominent emphasis on the educational facets of integrating AI into ME, specifically in terms of student engagement and curriculum development. The keyword map in Figure 6 also emphasizes the strong association between “Engineering education” and “artificial intelligence,” highlighting the increasing integration of AI in engineering pedagogies. The map also demonstrates connections between “artificial intelligence” and specific mechanical engineering subfields, including “manufacturing processes” “3D printing” “machine design” “robotics,” and “failure (mechanical).” These links suggest a keen research interest in the way AI can enhance specific technical areas among ME, potentially leading to innovations in design, process optimization, and predictive maintenance. Both (Figure 6) and (Table 2) highlight the multidisciplinary and transformative potential of AI in reshaping both the engineering curriculum and the technical competencies needed in the field.
The co-occurrence network in Figure 7 underscores the central role of “engineering education” in the research on AI applications, with strong linkages to advanced AI technologies like “machine learning” “deep learning” and “neural networks.” This indicates a keen enthusiasm for incorporating these cutting-edge AI methods into engineering curricula. The network also reveals a significant relationship between CAD and “automation,” suggesting a focus on automating CAD processes. However, the co-occurrence map in Figure 7 indicates that the integration of more sophisticated deep learning algorithms into CAD is still in its early stages, as the connection between CAD and “deep learning” appears less pronounced compared to the strong ties between other AI techniques and engineering education.
Figure 7. Network visualization of engineering education keywords: exploring connections between computer-aided design and artificial intelligence.
Furthermore, two supplementary keyword maps were produced. The first map, showcasing the author keywords, features keywords frequently used by authors with a minimum of five occurrences, leading to the identification of 17 authors as illustrated in Figure 8. In this figure, the red cluster includes terms such as “engineering education” and “machine learning,” indicating a strong focus on AI’s role in enhancing educational methodologies. On the other hand, the green cluster connects terms like “robot” and “mechanical design,” indicating an interest in robotics and design-oriented applications of AI. Similarly, the index keyword map (Figure 8), also with a five-occurrence minimum, highlighted 74 authors, demonstrating a more detailed and complex network of keywords. In this figure, “engineering education” stands out as a central node, connected to several sub-themes across different colored clusters, including “augmented reality” and “internet of things” in the blue cluster, emphasizing the role of immersive technologies and internet of things in education, and it is connected with curriculum-related terms, such as “computer aided design” and “curricula” in the yellow cluster, underscoring how AI is integrated into course development’ furthermore, it is connected to keywords related to advanced AI methods, such as “fault diagnosis” and “deep learning” in the red cluster, reflecting AI’s growing applications in predictive maintenance and quality control within MEE. This visual clustering aids in identifying prominent research areas and thematic relationships among keywords used by authors in the field of MEE. Similarly, this analysis of keywords accentuates the emphasis on AI in MEE due to its significant connections with several aspects of ME. By identifying keywords clusters and their thematic associations, these maps provide a comprehensive overview of current research trends, guiding future studies toward areas with enriches academic interests, as well as gaps needing further exploration.
Figure 8. Keyword mapping in engineering education: author keywords and index keyword interconnections.
The analysis concerning the geographic origins of the publications reveals significant patterns in global contributions and collaborations. A map illustrating the collaboration between countries was developed, applying a criterion where a country needed to have at least two documents to be considered. Hence, out of 51 countries that have published works, only 32 satisfied this condition, as illustrated in Figure 9.
As also shown Figure 9, a strong concentration is observed in some of the world’s most developed countries, with the United States leading in publication volume, followed closely by China and the United Kingdom. This suggests the emphasis these countries place on research in AI and MEE, likely driven by advanced technological infrastructure, substantial research funding, and a high number of institutions with specialized AI research programs. The green and yellow clusters in the map depict collaborations primarily between the United States, China, and the United Kingdom, suggesting a robust global network where research is frequently shared and co-authored across borders. This collaboration is essential as it enhances the cross-pollination of ideas, standardization of AI methodologies in MEE, and sharing of innovations that address educational challenges internationally. On the other hand, Germany, India, and Australia are placed in smaller nodes which suggests that these countries’ emerging contributions and their interactions with leading countries, yet they are presented on the map which indicates a growing interest in integrating AI into engineering education, potentially offering new insights from various educational and industrial contexts. This network analysis focuses on the international effort toward advancing AI applications in MEE, highlighting the importance of global collaboration in fostering innovation. Furthermore, it also points to geographic areas where research might be less active, suggesting potential opportunities for expanding AI applications in MEE in underrepresented regions.
The top-ranking countries, distinguished by their total link strength are presented in Table 3. This table highlights the most active nations in AI research within MEE, emphasizing both the volume of contributions and the extent of their international collaborations. As shown in the table, a broad geographic distribution of interest, with representation across four continents, including North America, Asia, Europe, and Australia. This widespread engagement reflects the global significance of AI integration into MEE. The table summarizes the countries in a descending order, showing the countries with highest total link strength to the least.
A co-citation link is identified when two entities are both cited by the same document (Eck and Waltman, 2018). Numerous studies have explored the incorporation of AI into MEE, leading to the performance of co-citation analysis to highlight the most significant contributions by authors, as well as the interdisciplinary connections among them, especially regarding their focus on AI integration into MEE. The clusters are color-coded, grouping authors whose work is frequently co-cited, which suggests shared research interests or thematic overlap. For instance, the green cluster might represent the authors focusing on AI applications in the ME education to align with industry needs, while the red cluster could include the researchers who focus on enhancing the pedagogical methods and modernizing course materials through AI. These groupings reveal interdisciplinary connections and areas of focus within the field. A threshold was set, requiring a minimum of six citations for an author to be included, with the selection process capped at 100 authors to ensure the network reflects only the most significant contributions. Figure 10 illustrates the frequency with which authors are cited together within the field, while (Table 4) details the authors who possess the highest total link strength. Overall, this co-citation analysis helps map the intellectual structure of AI research in MEE, showing which authors and ideas are most interconnected and influential. It highlights the collaborative nature of the field and points to core contributors driving interdisciplinary advancements in AI-focused educational research.
From the 228 publications analyzed, the sources were ranked from highest to lowest based on their numbers. As a result, the top 10 sources, as displayed in Figure 11, were identified as those with the highest number of classified papers. According to Figure 12, the leading sources by publication count include the “ASEE Annual Conference and Exposition,” “Journal of Physics,” “Lecture Notes in Mechanical Engineering,” and “Advances in Intelligent Systems and Computing,” which accounted for 31, 9, and 6 publications, respectively.
The examination of the sources reveals a compelling overview of the cross-disciplinary interest with AI applications in MEE. Likewise, the wide array of studies drawn from various fields, including Physics, Mechanical Engineering, and Computer Science, underscores the importance of incorporating AI into MEE. This diversity highlights the necessity for further development and improvement of research in this area.
The variety of document types presented indicates a substantial interest in AI within ME. These documents cover a range of themes and subthemes identified in the content analysis, such as robotics, additive manufacturing, CAD, AI, and more. Additionally, the papers span various interdisciplinary fields within mechanical engineering, including robotics, mechatronics, and simulations. Moreover, numerous documents highlight the significance of introducing new curricula, considering ethical aspects, bridging the gap between industry and academia, and integrating AI into ME industrial systems, as detailed in the content analysis.
Figure 12 presents the themes identified in the analyzed papers, encompassing intelligent systems like VR/AR, AI, and robotics within the MEE sector. It also highlights the necessity for an updated curriculum that integrates these technologies, the application of AI in the mechanical engineering industry, and the effort to close the gap between industrial practices and academic research.
The bibliometric analysis reveals a strong emphasis in the literature on integrating AI technologies into MEE. Analysis of keyword linkages underscores essential connections between education, technology, and skills, highlighting the interdependence of these areas and their critical role in advancing MEE. Additionally, the global distribution of research contributions demonstrates widespread interest, underscoring the importance of expanding research in this field to achieve a more comprehensive understanding of AI’s impact on engineering education. Key findings from this analysis point to emerging themes and research clusters that focus on personalized learning, intelligent tutoring systems, and simulation-based learning, which reflect the evolving landscape of MEE. These observations inform the subsequent content analysis, which will delve into the primary themes and insights identified across the literature, offering a nuanced view of current trends and potential areas for future research.
The primary focus of our exploration is the integration of AI in MEE. In this content review, our emphasis will be on identifying how AI is utilized in MEE, offering a comprehensive overview of scientific publications interested in this field. The key findings of these articles and their future directions that could aid future researchers in delving into this field will be shown. Additionally, this will help in identifying what has been done and what gaps have been found in the exploration of AI in MEE. Furthermore, the advantages provided to the educational experience will be listed. Additionally, various aspects in the field of incorporating AI in MEE will be discussed by this section.
The publications are organized by topic for clarity and relevance. Papers on artificial intelligence in MEE are grouped together, as are studies on virtual/augmented reality, robotics, CAD, and additive manufacturing. This separation helps clarify the contributions and future directions of each technology. Similarly, discussions on the need for a new curriculum, the integration of industry practices into education, and the use of AI in the mechanical engineering industry are each categorized separately. This highlights the importance of incorporating new technologies in MEE. Additionally, breaking down these main topics into subthemes simplifies the explanation and enhances understanding of each specific aspect. The themes and subthemes in this review were categorized through a structured, multi-step process designed to ensure objectivity and relevance. First, the relevant literature was downloaded from the Scopus database and organized in an Excel file for systematic review. Next, each paper was carefully studied to assess its relevance to the scope of our review, and only those papers closely aligned with the study’s objectives were retained for analysis. Following this, an initial scan of the selected papers was conducted to observe recurring patterns, allowing us to identify major and minor themes. To enhance the consistency and rationality of these classifications, we established clear criteria: themes had to appear in at least four different studies to be considered recurring, and subthemes were identified when specific applications or perspectives were frequently associated with a major theme. This process ensured that the themes reflected both the frequency and significance of topics within the literature, rather than subjective interpretation. The remainder of this section discusses the content analysis, providing insights into these themes and their implications for the integration of AI in MEE.
AI applications are diverse, and integrating this technology offers substantial benefits to students, educators, and institutions in achieving their educational objectives. The incorporation of AI can significantly enhance students’ comprehension of information and assist educators in tailoring assignments to align with individual students’ knowledge levels. Furthermore, AI serves as an efficient tool for conducting assessments. Consequently, this section will highlight scientific publications that have explored the integration of AI into MEE, showcasing its multifaceted advantages in the educational landscape (Table 5).
The above table reveals a range of findings from literature that relate to the integration of AI in MEE. The cited papers show that AI can facilitate learning, promote personalized learning, and advocate for student centered learning through the integration of chatbots in mathematical concepts, smart tutoring systems with feedback provider by utilizing deep learning (DL) and reinforcement learning algorithms. As such, this has a positive impact on the creativity and efficiency of educational experience, as well as enhanced students’ motivation, engagement, and industry preparedness. AI plays a transformative role in personalized learning by creating adaptive learning paths that guide students through step-by-step solutions tailored to their unique needs. Data is collected through various channels, including student interactions with course materials, performance metrics, and behavioral data during learning activities. This rich data is then analyzed using machine learning and deep learning algorithms, which identify patterns and adjust content delivery to match individual learning styles and pace. Key algorithms facilitate this personalization: supervised learning algorithms, like linear regression and decision trees, analyze student progress to suggest appropriate learning steps; reinforcement learning algorithms, such as Q-learning and Deep Q-Networks, adaptively refine these paths based on real-time feedback; clustering techniques, like K-means and hierarchical clustering, group students by learning style or performance to provide similar guidance; and collaborative filtering, such as matrix factorization, offers recommendations based on peer learning behaviors. Natural Language Processing (NLP) algorithms, including word embedding and sentiment analysis, enhance personalized interactions by understanding and responding to student language. Finally, neural networks, such as feedforward and recurrent networks, continuously learn from new data to provide increasingly effective and personalized learning experiences. Literature also reveals that some of the studies have delved into the integration of auto-assessment tools in MEE. Accordingly, some have discussed the usage of AI and others have stated the potential of integrating AI within assessment tools to provide better accuracy. Lastly, the publications have discussed the utilization of ML algorithms for the classification of group projects or task allocation, based on student’s expertise within the course of their study which can be often challenging with human bias and complexity. Therefore, AI has shown promising results in theme allocation for tasks and group projects resulting in enhanced learning outcomes.
Further research is therefore imperative to identify best practices in personalized learning, to consider aspects such as ethics, gamification for active engagement, and NLP to facilitate human-robot interaction, to make sure that the field of MEE continually aligns with technological advancements and student’s. There is also potential for further research to examine the integration of AI in the field of development of auto-assessment tools since there are insufficient number of studies that have investigated this field. Similarly, the investigation of using AI in assessment tools for better accuracy can be conducted. AI shows promise not only enhancing assessment accuracy but also identifying student retention rates. However, future research should consider ethical implications, AI-assisted peer assessment, NLP for written assessments, simulations for hands-on assessments, and the creation of intelligent grading and feedback systems. Moreover, it is crucial to explore the use of AI in theme allotment and task assignment across all ME courses, considering students’ experience and skills. There is also a pressing need to develop AI techniques, encompassing ML, DL, and reinforcement learning algorithms, that can process larger datasets more effectively, yield more accurate predictions, and precisely gauge student performance.
Virtual Reality (VR) and Augmented Reality (AR) are transforming multiple sectors, including MEE, by providing immersive and interactive experiences. VR offers lifelike simulations that enable hands-on learning, foster collaboration, improve spatial skills, and facilitate the visualization of complex data. Concurrently, AR enhances real-world environments by overlaying digital information, offering features like remote assistance, and enriching traditional laboratory settings. These technologies significantly aid in the comprehension of ME concepts by creating dynamic, interactive learning environments. This section will delve into various publications that have explored the integration of VR and AR into MEE education, highlighting their impactful contributions to the field (Table 6).
The research papers in this section focus on the integration of VR/AR technologies in MEE. The papers show the integration of VR/AR technologies in MEE can simplify to students the visualization of complex engineering drawings and enhances their spatial abilities; also, these technologies can accommodate for higher number of students and provide them a safer environment which can be hazardous in real life. Thus, this resulted in positive impacts on students’ academic journey as it boosted their motivation, understanding, and memory retention in the content of their study. Furthermore, these technologies have developed numerous advantages, including enhanced problem-solving, critical thinking, sustained attention, and reduced cognitive load on learners. Some of the studies have investigated the utilization of VR/AR technologies to encompass all ME laboratories which creates a safer environment to students; moreover, these technologies have been examined for assembly and disassembly of automotive components creating an interactive learning experience. Consequently, this offers students a hands-on experience and reveals a bigger opportunity for industry preparedness. A study has examined the integration of gamification within these technologies to increase engagement and joyfulness. Another study has investigated the integration of AI in VR technology using deep reinforcement learning algorithms to create interactive and intelligent simulations. It can aid learners by controlling objects, providing real-time feedback, and adapting to students’ actions, improving their understanding of complex CAD designs. Nonetheless, the data is retrieved from 3D models and learner interaction with the environment. Hence, AI learns by conducting trial and error, improving the decision making by continuously interacting with the virtual environment by maximizing rewards for correct actions and minimizing penalties for errors.
Further research is imperative to investigate the use of sophisticated AI algorithms, including ML and DL, to facilitate the creation of personalized content, interactive simulations, and the provision of timely feedback all of which contribute to enhanced learning outcomes and a more enriching educational environment. However, the implementation of VR and AR is not without challenges. These include the necessity for faculty training to adeptly handle these technologies, the need for continuous updates and maintenance, ensuring accessibility to technology and internet connectivity, and addressing pertinent privacy and security concerns. Furthermore, there is a need for more comprehensive studies on student motivation and the inclusion of larger sample sizes to validate the impact of these technologies. The employed technologies must align with course delivery objectives, seamlessly integrate AI for customized learning experiences, and incorporate gamification elements to boost engagement and motivation. Additionally, leveraging NLP for personalized simulated environments can further tailor the learning experience to match the students’ pace and course requirements. Hence, a deeper exploration into VR and AR technologies is warranted to fully harness their potential in achieving optimal learning outcomes.
Robotics plays a significant role in reshaping the landscape of MEE. As a multidisciplinary field of engineering, electronics, and computer science, robotics introduces students to the practical applications of theoretical concepts. In MEE, robots serve as powerful tools for hands-on learning, enabling students to delve into control systems, mechatronics, and automation. The integration of robotics and robotics platforms in education not only fosters technical expertise but also cultivates creativity and innovation, preparing students for the evolving challenges in the field of ME. This section will present publications that have discussed the usage of robotics in the MEE (Table 7).
The papers in this section highlight the widespread use of robotics in education to enhance the learning experience, particularly in MEE. Robotics, mechatronics, and AI integration are employed to stimulate students’ interest in ME courses. Robotic educational platforms offer an inclusive educative system with tasks and tutorials in ME courses, and perform several tasks in 3D design, programming, controlling, operating, and planning. Similarly, they can simplify the concepts and enhance the learning experience, as they can provide instructional aid which enhances the learning experience and increases students’ motivation. The usage of robotic kits can be an attractive opportunity to provide students with the hands-on experience which has been revealed to increase the engagement of students and develop better learning outcomes. Publications have shown that the integration of AI within these robotic platforms can add flexibility that accommodates different interests and skillsets in multidisciplinary courses. Other publications have emphasized that the integration of AI can facilitate the simulating process of mechatronics systems and simplify them to students.
The research has focused on specific fields and courses, indicating a need to expand the exploration of robotics education and problem-based learning across various educational settings. Additionally, further studies are necessary to enhance the capabilities and applications of robots, potentially through the use of AI. Integrating robotics with environmental and sustainability initiatives could also promote the use of recycled materials and energy-efficient designs in robotics projects. Finally, employing NLP could improve human-robot interactions, enabling more personalized learning experiences and facilitating interdisciplinary integration.
The dynamic nature of the world, and ongoing technological advancements in industries, necessitates the restructuring of the traditional MEE curriculum. This reshaping ensures that students are equipped with the latest skills, preparing them to face the ever-evolving challenges of modern engineering. In this section, we investigate scientific publications that emphasize the imperative need for reshaping the MEE curriculum (Table 8).
The papers in this section reveal the importance of integrating technologies in educational settings as they can greatly facilitate the learning process. Also, the publications discuss the evolution of delivery methods is essential to keep in pace with technological advancements, including the incorporation of advanced technological tools that can accommodate for both virtual and real worlds. The studies demonstrate frameworks of technologies for educative use to compromise for the rapid shift of educational delivery methods, underscoring the essential nature of adaptability in modern education. In particular, the incorporation of some technologies like Generative Artificial Intelligence (GAI) into MEE must be carefully managed to align with these evolving educational standards. The integration of technologies in the MEE curricula should adhere to the principles set forth by the Engineering Education Accreditation (EEA), with a focus on developing engineering professionals who are not only technically proficient but also globally conscious and capable of adapting to continuous economic and societal shifts. Also, with the utilization of advanced technologies like GAI, a cautious approach is paramount due to their potential for misuse. It is critical to implement strategies that counteract academic misconduct effectively. These strategies should encompass comprehensive education for students on the responsible use of technologies like NLP models, the establishment of clear and concise academic policies regarding technology use, and the integration of these tools in a manner that enhances critical thinking skills. Additionally, employing advanced plagiarism detection software capable of identifying content generated by AI is crucial. Equally important is the promotion of a collaborative learning environment and group work, which together play a vital role in upholding academic integrity while fully leveraging the advantages GAI offers in the academic sphere.
The integration of AI in MEE significantly enhances learning experiences, making education more accessible and interactive through technologies like computer-aided translation and NLP. This shift demands changes in teaching methods, with a focus on adaptability and the integration of digital tools. The careful incorporation of GAI is key, emphasizing the development of globally aware, adaptable engineering professionals. Addressing the potential for AI misuse is crucial, requiring strategies for responsible technology use, promoting critical thinking, and fostering a collaborative learning environment. This approach ensures the effective and ethical use of AI in enhancing MEE.
The field of ME heavily relies on CAD modelers, as nearly every ME process or system incorporates engineering drawings and simulations. These tools enable engineers to materialize any concept, allowing for testing in a cost-effective and safe environment. Consequently, advancing this aspect of ME is crucial, as it significantly contributes to enhancing the efficiency and performance of this subfield. Integrating AI into CAD modelers, engineering drawings, and simulations has the potential to unlock a myriad of benefits. This section will encompass scientific publications that explore this domain within the context of AI (Table 9).
The papers highlight the impactful integration of AI in CAD for ME, showcasing its effectiveness in enhancing learning, concept demonstration, and addressing spatial analysis challenges in drawing interpretation. AI, particularly through ML and DL algorithms, plays a crucial role in the digitization of engineering drawings, improving the accuracy and efficiency of detecting, classifying, and converting elements to 3D models. The digitization process involves several steps. First, preprocessing is applied, which includes binarization, noise reduction, and thinning. Subsequently, vectorization converts raster images into scalable and editable graphics, utilizing techniques like morphological operation and line detection to identify shapes and lines. AI plays a key role in shape and symbol detection through the usage of deep learning algorithms, classifying symbols by learning from labeled data and using graph-based approaches to detect connections. Feature extraction and classification then apply statistical and structural features to refine the digitized symbols, removing distortions, completing broken lines, and normalizing shapes. In the contextualization stage, AI infers relationships between symbols and analyzes how components are connected, utilizing both shape recognition and contextual rules. This process aids learners by recognizing mistakes in their CAD designs, suggesting corrections, automating design adjustments, and optimizing the design. Also, AI assists in the transition from 2D drawings to 3D CAD models by recognizing and reconstructing geometric shapes. This digitization not only enhances accessibility and preserves documents but also increases efficiency by automating the identification and interpretation of drawing elements. AI’s integration into CAD is praised for automating design processes, analyzing vast data, fostering creativity, enhancing decision-making, and reducing design-related costs and time. Despite the challenges and time consumption in using CAD modelers, AI’s role in automating and optimizing the design process is recognized as a significant advantage. The papers further identify the potential for future research in AI’s role in manufacturing and product design, especially in developing algorithms for new product generation and advancing manufacturing research through 3D CAD model data analysis.
Several gaps have been identified in the digitization of engineering drawings. These include a scarcity of annotated examples, the absence of domain-specific datasets, and a lack of guidelines for interpreting drawings. There is also a need for further research on the contextualization of digitized information from specific types of engineering drawings, a lack of standardization across engineering drawings, and limited testing in the application of machine vision and ML techniques. Additional gaps include a limited scope of investigation, the need for a more judicious selection of ML models, and issues with generalizability. Consequently, further research in this area is imperative. This research should focus on extending to more complex shapes, enhancing accuracy, integrating with CAD modeling, exploring ML models, and addressing existing limitations. Moreover, the development of hybrid approaches that combine heuristic-based methods and document image recognition with DL techniques, the creation of specialized datasets, the introduction of advanced testing methods, the contextualization of digitized information, and the integration of emerging technologies like DL are also critical. Importantly, there is a significant need for continued research in implementing techniques that facilitate the generation of new products using CAD modelers.
AM, commonly known as 3D printing, holds great significance as it transforms drawings into tangible 3D models. This process notably aids in the enhancement of students’ spatial abilities and offers them enriched hands-on experience, thereby bolstering their skills, and deepening their knowledge base. Consequently, the advancement of AM is crucial, and this can be achieved by integrating AI, which promises to bring a multitude of benefits to these processes (Table 10).
The papers in this section were examined to illustrate the critical role of AM in MEE, particularly robotics education and to show how the integration of AI can positively impact these technologies enhancing the learning experience. The papers highlight the benefits of AM in MEE, as AM accelerates the design process and enables rapid prototyping and iterative refinement of robotic components. Moreover, AM’s capacity to create complex and functional systems due to its adeptness in producing complex geometric and employing lightweight materials, makes it an invaluable tool in the ME field. Thus, the findings have concluded that this not only allow students to quickly test and adjust their designs but also significantly improve their understanding and practical skills in designing and manufacturing robotic systems, as it provides them hands-on experience, effectively preparing them for future industry challenges, as well as it cultivates creativity, boosts confidence, enhances design capabilities, and deepens understanding of core of ME concepts.
The integration of AI in AM significantly optimizes the process by controlling and enhancing various aspects. AI contributes to AM by improving process control, offering real-time monitoring, and enabling predictive outcome modeling. It also aids in designing new materials, reducing waste and production time, and decreasing costs through ML, DL, and reinforcement learning algorithms. Furthermore, AI is involved in detecting defects during AM process, showcasing its comprehensive utility in enhancing the efficiency and quality of AM. Additionally, AI strategically chooses processing parameters, optimizing product design, and notably accelerating delivery timeline from production to application.
Optimizing AM through the utilization of advanced AI techniques, such as improved ML models, is of great significance. Additionally, fostering a collaborative environment between humans and machines can significantly enhance the printing process, with generative AI playing a key role in customizing printed materials to meet specific individual needs. Moreover, further exploration into the use of AI for advanced simulation and modeling in AM processes is warranted. Ultimately, future research in this domain is crucial to identify and establish best practices in the integration of AI within the context of AM.
As mentioned in the previous section, it is important for institutions to get students to acknowledge the industry, so they develop the required skills in using specific technologies during their academic journey which will prepare for the workforce. This section discusses the publications that have focused on combining industry and education to bridge the gap between them (Table 11).
AI technologies are rapidly evolving and finding applications in numerous fields, including both industrial and educational sectors. It is, therefore, crucial to examine scientific publications that focus on this area of interest. These publications are helpful in bridging the gap between industry and academia, and they aim to equip students with competencies needed to utilize in industry technologies effectively. This preparation is vital for students entering the workforce, as it promises to yield significant advantages in the industry’s future. The papers in this section highlight the importance of integrating AI in the educational context of ME since students encounter equivalent challenges that are present in the industry. They also emphasize the importance of bridging the gap between academia and industry to bridge the gap between them providing students an enhanced preparedness for the industry and better practical skills. The publications have discussed the cruciality of students acknowledging AI and its uses in some of ME applications as employees in industry, as well as the importance of implementing a curriculum that can accommodate for such technology. Furthermore, the papers have highlighted the enhanced learning experience that has resulted by the usage of AI and VR.
However, there is a need for further exploration of improved digital simulations, and educational institutions should consider integrating AI technologies into their curricula. Such integration will familiarize students with industry standards, enhancing their knowledge and skills in engineering systems. Additionally, the curriculum should place greater emphasis on hands-on experience and practical exposure to industrial practices, ensuring students are well-prepared for the demands of the industry.
This section delves into the integration of AI within the ME industry, which plays an essential role in narrowing the gap between industry and academic learning, as illustrated in Table 12. This table categorizes relevant publications into three distinct areas: AI in the ME industry, AI in ME systems, and AI in the manufacturing industry. The significance of this classification lies in its comprehensive coverage of current industry practices. By familiarizing institutions and stakeholders with these practices, it enables them to equip students with relevant, industry-aligned knowledge and skills. This approach not only enhances students’ understanding of real-world applications of their studies but also effectively prepares them for their future roles in the workforce, thereby effectively bridging the industry-education divide.
The development of technology and the progress of industries necessitate an examination of AI technologies used in industry 4.0 to determine the content of future curricula for ME students. The publications in this section emphasize the utilization of AI in many industries including manufacturing and aerospace industries, as well as in mechatronics systems. AI is being utilized for numerous purposes, i.e., enhance work efficiency, automate repetitive tasks, diagnose defects, provide predictive solutions, develop safer environment, and predict and evaluate mechanical properties using ML and DL algorithms. Furthermore, AI has boundless usage in manufacturing industry as to assist manufacturers in visualizing the challenges that were drastically difficult to acknowledge, uncovering concealed bottlenecks, and identifying unprofitable production lines. Also, AI improves accuracy of rotating machinery, reduces time and cost, enables online status detection and remote monitoring of mechanical equipment, and increases efficiency of detection. In addition, the incorporation of AI in Aerospace field has brought several benefits including enhanced automation in aerospace systems, intelligent robotics, autonomous control systems for unmanned vehicles, better sensor technology, and valuable educational opportunities with hands-on experience. Also, it is used for many purposes including image and speech recognition, predictive maintenance, and recommendation systems. Additionally, the publications have stated the potential of AI in mechatronics systems such as quality control, optimization of processes, providing autonomous systems, decision-making, and analyze data gathered from sensors to predict future damages. Industry’s main objective is to achieve higher levels of efficiency, product quality, and productivity. Therefore, some of the future directions of the industry is to focus on the development of smart manufacturing, advanced robotics, digital twins, and predictive maintenance.
Nevertheless, the acknowledgment of the industry advancements in utilizing technologies is crucial for institutions as their main objective is to prepare students to the work field; thus, it is crucial to study industry and their advancement which will get the students with more familiarity regarding the technologies utilized.
The incorporation of AI in industry has brought diverse benefits as it has optimized the processes and has increased efficiency. Secondly, it has created a safer environment for employees as it has automated several operations. Also, it has helped in creating a valuable opportunity for educational and training by providing a hands-on experience with more enhanced technologies. Nonetheless, the advantages that AI has brought to industry reveals the cruciality of this technology to be integrated in many fields including MEE. Thus, this can enhance the learning experience and benefit students, educators, and institutions in a wide range of opportunities. Accordingly, it is important to address unresolved issues, advance AI techniques, and integrate AI with more technologies.
The conceptual framework presented below (Figure 13) inspired by Bahroun et al. (2023), offers a detailed visualization and categorization of publications that discuss the diverse applications of AI in MEE across various domains. The framework features a spider graph with a central dark black circle connecting to a two-tier structure: the initial tier represented by dark gray circles and the second tier by light gray circles. The black circle represents the overarching theme of “AI in MEE,” the initial tier highlights primary themes of AI applications, while the second tier is linked to secondary themes. For instance, “3D Printing” is linked to themes like “Accelerated material research” and “Mathematical chatbots,” showcasing areas where AI can enhance materials research and provide educational tools. The size of the circles in the spider graph varies, with larger circles indicating themes that are more prevalent and prominent. Conversely, smaller circles suggest fewer scientific publications have considered the theme they represent. This graphical representation provides a comprehensive overview for future researchers, offering insights into which areas require more development and enhancement, which have been less explored, and which exhibit the most significant gaps in the literature. Consequently, researchers in the field can target their investigations toward these areas, potentially contributing to their growth and development.
The content analysis in this review offers a structured overview of AI-driven tools and methodologies that can significantly enhance the pedagogical design of engineering education. By categorizing AI applications across various educational contexts, this review provides educators and curriculum developers with practical insights into integrating AI to create more effective, personalized, and industry-aligned learning experiences. The analysis reveals AI’s potential to transform traditional teaching methods through adaptive learning systems, automated assessment tools, and simulation-based learning environments that support hands-on and experiential approaches. These insights are particularly valuable for designing courses that respond to individual learning needs, facilitating adaptive feedback and intelligent tutoring. Furthermore, the content analysis underscores the role of AI in fostering essential technical and analytical skills, preparing students for the technologies they will encounter in the workforce. By integrating these AI applications, educational institutions can enhance student motivation, engagement, and preparedness for industry, making the curriculum more dynamic and aligned with technological advancements in engineering fields.
However, the integration of AI in MEE is also accompanied by its challenges and limitations. Ethical concerns, such as data privacy and potential biases in AI-driven assessments, must be carefully addressed to ensure responsible application. Furthermore, the need for substantial technological infrastructure, including powerful computing systems and specialized software, poses a barrier to widespread AI adoption. Faculty training is also crucial, as instructors must be equipped to effectively use AI tools and adapt their teaching methods accordingly. Without proper training, faculty resistance to change and unfamiliarity with AI could hinder successful integration. Addressing these limitations is essential for realizing AI’s full potential in MEE and ensuring that it enhances the learning experience rather than detracts from it.
AI technology is rapidly evolving, and its applications are expanding across various fields, including MEE, where it is transforming education at different levels. In MEE, AI is utilized for a variety of purposes such as personalized learning, smart tutoring systems, digitizing engineering drawings, simulations, fault diagnosis, and more. The incorporation of AI in MEE has raised several concerns, calling for enhanced accuracy, more personalized learning approaches, improved simulations, increased efficiency, and academic integrity. These concerns are driving changes in the curriculum, elevating it to new heights. A thorough literature review has resulted in the analysis of 228 publications, with the most relevant papers being discussed in detail, showcasing their findings, and outlining future research directions.
First, a bibliometric analysis of AI applications in MEE has been conducted. This analysis began with an initial poll of 765 articles sourced from the Scopus database. After a meticulous process of literature screening and the removal of duplicates, the focus narrowed down to 228 publications specifically addressing AI in MEE, spanning the years 2018 to 2023. This comprehensive bibliometric analysis yielded several key insights. The results highlighted a range of interdisciplinary ME subfields globally incorporating AI. Moreover, it revealed a significant volume of scientific publications dedicated to this domain. Additionally, numerous authors have notably contributed to the discourse on integrating AI into MEE, bringing diverse perspectives and insights to the field.
Following the bibliometric analysis, the next step involved conducting a content analysis to thoroughly investigate the application of AI in MEE. This analysis yielded significant findings, highlighting the diverse applications of AI across various aspects of MEE, including manufacturing, AM, thermodynamics, simulations, and engineering drawings. Additionally, it brought attention to areas such as smart tutoring systems, classification, and personalized learning. AI tools like VR and AR have been noted for their substantial role in developing students’ spatial skills and enhancing their motivation and engagement. Similarly, robotics and robotic platforms have proven beneficial in student learning within numerous robotic courses and other science education domains. Moreover, the integration of AI in robots and VR/AR technologies has shown positive impacts, enhancing their functionality. Furthermore, the reviewed scientific publications have revealed the numerous benefits that AI brings to MEE, with many papers stressing the importance of incorporating these technologies into the ME curriculum.
The promising outcomes that AI has brought to MEE have been notably highlighted in various studies. This review delves deep into the potential that AI unfolds in enriching MEE. Building on these findings, it suggests several future research directions that could further explore and expand the integration and impact of AI in this field.
Integrating GAI and Large Language Models (LLMs) like ChatGPT into the MEE curriculum offers a transformative opportunity to revolutionize this field. By leveraging LLMs that utilize NLP, educational approaches in MEE can be enhanced with personalized learning, intelligent tutoring systems, and effective feedback mechanisms. For example, ChatGPT, known for its advanced NLP capabilities, can be especially beneficial for MEE courses, facilitating tailored explanations of complex engineering concepts and improving interactive learning experiences. The vast potential of such technologies includes assisting in the instruction of challenging topics, providing instant feedback, and simulating real-world engineering problem scenarios. Specifically, GAI tools offer the potential to transform several aspects of the MEE curriculum. In simulations, AI can create complex engineering scenarios, enabling students to engage with real-world problem-solving in virtual environments. For coding exercises, these tools can provide instant feedback and code suggestions, accelerating learning for computational tasks. In research support, AI can assist students with literature reviews, trend analysis, and insights into emerging fields. For theoretical and computational problem-solving, AI offers step-by-step guidance on complex concepts and calculations, making abstract topics more accessible and interactive. However, these applications come with challenges, such as ethical risks (e.g., potential misuse and biases), accuracy concerns, faculty resistance, and a need for specialized training. Additionally, there are practical barriers, including possible reductions in critical thinking, technical limitations, accessibility, and compatibility issues with existing software. Addressing these challenges will be crucial to fully harnessing the educational benefits of GAI in a responsible and effective manner.
The future of MEE stands on the brink of significant advancements, primarily driven by the integration of AI. A crucial step forward involves developing theoretical guidelines or conceptual frameworks to assist educators and stakeholders in effectively implementing AI models, such as GAI, within the MEE curriculum. A key focus area is the automation of AM processes, where the use of machine learning algorithms is set to boost efficiency and performance. Additionally, advanced deep learning and reinforcement learning algorithms are expected to transform automated simulations, particularly in generating new products through 3D CAD model analysis. Another promising development is the creation of intelligent tutoring systems in mechanical engineering. These systems, which integrate GAI models with multimedia elements like animations and simulations, aim to simplify complex concepts for students. Efforts are also being made to enhance the precision of digitizing engineering drawings, utilizing AI for more accurate innovations. Moreover, addressing ethical considerations in using AI, particularly models like ChatGPT in educational settings, is essential for ensuring their responsible application. Finally, strengthening the connection between industry and academia is vital. This connection aligns academic learning with industry practices, equipping students with the skills needed to tackle real-world engineering challenges and meet industry standards.
The original contributions presented in the study are included in the article/supplementary material, further inquiries can be directed to the corresponding author/s.
MA: Conceptualization, Data curation, Formal analysis, Investigation, Methodology, Software, Visualization, Writing – original draft, Writing – review & editing. VA: Conceptualization, Formal analysis, Investigation, Methodology, Supervision, Validation, Writing – review & editing. ZB: Conceptualization, Data curation, Investigation, Methodology, Supervision, Validation, Writing – review & editing.
The author(s) declare that no financial support was received for the research, authorship, and/or publication of this article.
The authors declare that the research was conducted in the absence of any commercial or financial relationships that could be construed as a potential conflict of interest.
All claims expressed in this article are solely those of the authors and do not necessarily represent those of their affiliated organizations, or those of the publisher, the editors and the reviewers. Any product that may be evaluated in this article, or claim that may be made by its manufacturer, is not guaranteed or endorsed by the publisher.
Afanasyev, A., Voit, N., Ionova, I., Ukhanova, M., and Yepifanov, V. (2018). International conference on interactive collaborative learning. Cham: Springer.
Ali, M.A.M., Al-Yousif, S., Mohammed, M.N., Al-Sanjary, O.I., and Abdullah, M.I., (2018). IEEE Conference on Systems, Processes & Control (ICSPC). IEEE. 84–88.
Ao, H., Zhang, H., and Yan, H., (2021). Information communication Technologies Conference (ICTC). IEEE. 353–357.
Auerbach, J. E., Concordel, A., Kornatowski, P. M., and Floreano, D. (2019). Inquiry-based learning with RoboGen: an open-source software and hardware platform for robotics and artificial intelligence. IEEE Transac. Learn. Technol 12, 356–369. doi: 10.1109/TLT.2018.2833111
Awuor, N. O., Weng, C., Matere, I., Chen, J., Pusspitasari, D., and Tran, K. N. P. (2022). The effect of 3D-stereogram mobile AR on engineering drawing course outcomes among first-year vocational high schoolers with different spatial abilities: a Bloom’s taxonomy perspective. Interact. Learn. Environ. 1–19. doi: 10.1080/10494820.2022.2158477
Ayub, M.A., Yusoff, N., and Heinmann, B. World Congress on Science, Engineering and Technology (WCOSET) 2021 (2023). 2571. doi: 10.1063/5.0115801
Bahroun, Z., Anane, C., Ahmed, V., and Zacca, A. (2023). Transforming education: a comprehensive review of generative artificial intelligence in educational settings through bibliometric and content analysis. Sustain. For. 15: 1–40. doi: 10.3390/su151712983
Barroquillo, M.D., Duque, P.S.L., Bellosillo, E.S., Espanola, J.M.V., Magon, S.S., Manuel, M.C.E., et al. (2021). IEEE International Conference on Automatic Control and Intelligent Systems (I2CACIS). IEEE. 241–246.
Belapurkar, G., Chauhan, A., Panwar, A., Fernandes, V., and Arya, K., (2019). IEEE International Conference on Teaching, Assessment and Learning for Engineering (TALE). IEEE. 1–6.
Bello-Robles, J.C., Suarez, O.J., and Garcia, A.P., (2021). 19th LACCEI International Multi-Conference for Engineering, Education, and Technology. LACCEI Inc. 1–6.
Bencheva, N., and Kostadinov, N., (2023). 32nd Annual Conference of the European Association for Education in Electrical and Information Engineering (EAEEIE). IEEE.
Bharadwaj, A., Xu, Y., Angrish, A., Chen, Y., and Starly, B., (2019). ASME 2019 14th international manufacturing science and engineering conference Erie, Pennsylvania, USA.
Bi, S. (2020). Journal of Physics Conference Series. IOP Publishing Ltd. 1550, 1–4. doi: 10.1088/1742-6596/1550/3/032036
Boboc, R. G., Chiriac, R., and Antonya, C. (2021). How augmented reality could improve the Student’s attraction to learn mechanisms. Electronics. 10:1–24. doi: 10.3390/electronics10020175
Boya-Lara, C., Saavedra, D., Fehrenbach, A., and Marquez-Araque, A. (2022). Development of a course based on BEAM robots to enhance STEM learning in electrical, electronic, and mechanical domains. Int. J. Educ. Technol. Higher Educ. 19, 1–23. doi: 10.1186/s41239-021-00311-9
Brazina, J., Stepanek, V., Holub, M., Vetiska, J., and Bradac, F., (2022). 2022 20th International Conference on Mechatronics - Mechatronika (ME). IEEE.
Brezeanu, T., and Lazarou, E., (2020). eLearning sustainment for never-ending learning Proceedings of the 16th International Scientific Conference. University Publishing House. 2, 328–334.
Bula, I., Hoxha, V., and Hajrizi, E., (2018). IFAC (International Federation of Automatic Control). Elsevier.
Cai, W., Grossman, J., Lin, Z. J., Sheng, H., Wei, J. T., Williams, J. J., et al. (2021). Bandit algorithms to personalize educational chatbots. Machine Learn. 110, 2389–2418. doi: 10.1007/s10994-021-05983-y#citeas>
Caridade, C. M. R. (2023). International conference on mathematics and its applications in science and engineering. Cham: Springer.
Castelli, K., and Giberti, H. (2019). Additive manufacturing as an essential element in the teaching of robotics. Robotics 8, 1–13. doi: 10.3390/robotics8030073
Chen, D., Chen, Y., Wen, K., Deng, W., Lu, S., and Chen, X., (2023). International conference on innovative technologies and learning. Springer.
Chen, H., Tsang, Y. P., and Wu, C. H. (2023). When text mining meets science mapping in the bibliometric analysis: a review and future opportunities. Int. J. Engin. Business Manage. Springer. 15, 1–15.
Chen, D., You, C., and Su, M. (2020). Development of professional competencies for artificial intelligence in finite element analysis. Interact. Learn. Environ. 30, 1265–1272. doi: 10.1080/10494820.2020.1719162
Choong, L.M., and Cheng, W.K., (2021). 2021 international conference on Computer & Information Sciences (ICCOINS). IEEE.
Chuang, S., Chen, C., and Wu, E.H., (2022). 2022 IEEE 4th Eurasia conference on IOT, communication and engineering (ECICE). IEEE.
Cordero-Guridi, J., Cuautle-Gutierrez, L., Alvarez-Tamayo, R., and Caballero-Morales, S. (2022). Design and development of a I4.0 engineering education laboratory with virtual and digital technologies based on ISO/IEC TR 23842-1 standard guidelines. Appl. Sci. 12, 1–13. doi: 10.3390/app12125993
Coronado, P. D. U., Demeneghi, J. A. A., Ahuett, H., Orta, P., and Martinez, M. (2022). Representation of machines and mechanisms in augmented reality for educative use. Int. J. Interact. Design Manufactur. 16, 643–656. doi: 10.1007/s12008-022-00852-x
Dagman, A., and Warmefjord, K. (2022). An evidence-based study on teaching computer aided Design in Higher Education during the COVID-19 pandemic. Educ. Sci. 12, 1–13. doi: 10.3390/educsci12010029
Eck, N.J.V., and Waltman, L., (2018). VOSviewer manual. Available at: https://www.vosviewer.com/documentation/Manual_VOSviewer_1.6.20.pdf
Faria, A. C. C., and Barbalho, C. M. (2023). Mechatronics: a study on its scientific constitution and association with innovative products. Appl. System Innov. 6, 1– 42. doi: 10.3390/asi6040072
Garces, J. M., Almagro, C. V., Lunghi, G., Castro, M. D., Buonocore, L. R., Prades, R. M., et al. (2021). MiniCERNBot educational platform: antimatter factory mock-up missions for problem-solving STEM learning. Sensors 21. doi: 10.3390/s21041398
Grisales-Palacio, V. H., and Garcia-Zaragoza, U. (2022). Connecting industry and academy through cyber-physical Systems for Disruptive Education in machine automation. Singapore: Springer.
Grodotzki, J., Ortelt, T. R., and Tekkaya, A. E. (2018). Remote and virtual labs for engineering education 4.0 achievements of the ELLI project at the TU Dortmund University. Procedia Manufactur. 26, 1349–1360. doi: 10.1016/j.promfg.2018.07.126
Guo, L., (2023). International conference on applied intelligence and sustainable computing (ICAISC) 2023. IEEE.
Guo, A. X. Y., Cheng, L., Zhan, S., Zhang, S., Xiong, W., Wang, Z., et al. (2022). Biomedical applications of the powder-based 3D printed titanium alloys: a review. J. Materials Sci. Technol. 125, 252–264. doi: 10.1016/j.jmst.2021.11.084
Hernandez-Chavez, M., Cortes-Caballero, J. M., Perez-Martinez, A. A., Hernandez-Quintanar, L. F., Roa-Tort, K., Rivera-Fernandez, J. D., et al. (2021). Development of virtual reality automotive lab for training in engineering students. Sustainability 13: 1–17. doi: 10.3390/su13179776
Hsia, C., Lai, C., and Su, Y. (2020). Impact of using ARCS model and problem-based leaning on human interaction with robot and motivation. Library Hi Tech 40, 963–975. doi: 10.1108/LHT-07-2020-0182
Hsieh, S., and Li, Q., (2018). 2018 ASEE Annual Conference & Exposition Proceedings. Texas A&M University.
Huang, J., Wensyeen, S., and Funk, M. (2023). Experiential speculation in vision-based AI design education: designing conventional and progressive AI futures. Int. J. Des. 17: 1–17. doi: 10.57698/v17i2.01
Jaakma, K., and Kiviluoma, P. (2019). Auto-assessment tools for mechanical computer aided design education. Heliyon 5:1–9. doi: 10.1016/j.heliyon.2019.e02622
Johnson, N. S., Vulimiri, P. S., To, A. C., Zhang, X., Brice, C. A., Kappes, B. B., et al. (2020). Invited review: machine learning for materials developments in metals additive manufacturing. Addit. Manuf. 36:1–30. doi: 10.1016/j.addma.2020.101641
Jovanovic, V.M., McLeod, G., Alberts, T.E., Tomovic, C., Popescu, O., Batts, T., et al. (2019). ASEE Annual conference & exposition. The American Society for Engineering Education (ASEE).
Kahangamage, U., and Leung, R. C. K. (2019). Remodelling an engineering design subject to enhance students’ learning outcomes. Int. J. Technol. Design Educ. 30, 799–814. doi: 10.1007/s10798-019-09519-3
Kesler, D., Arntz, A., Friedhoff, J., and Eimler, S.C., (2020). IEEE international conference on artificial intelligence and virtual reality (AIVR) 2020. IEEE.
Kibrete, F., and Woldemichael, D. E. (2023). International conference on advances of science and technology. Cham: Springer.
Klaric, S., Lockley, A., and Pisacic, K. (2022). The application of virtual tools in teaching dynamics in engineering. Tehnički Glasnik. 17, 98–103. doi: 10.31803/tg-20220303040401
Kuzilek, J., Zdrahal, Z., and Fuglik, V. (2021). Student success prediction using student exam behaviour. Futur. Gener. Comput. Syst. 125, 661–671. doi: 10.1016/j.future.2021.07.009
Lesage, J., Brennan, R., Eaton, S. E., Moya, B., McDermott, B., Wiens, J., et al. (2024). Exploring natural language processing in mechanical engineering education:implications for academic integrity. Int. J. Mechan. Engin. Educ. 52, 88–105. doi: 10.1177/03064190231166665
Lima, J., Kalbermatter, R.B., Braun, J., Brito, T., Berger, G., and Costa, P., (2022). IEEE Latin American robotics symposium, LARS 2022 Latin American robotics symposium (LARS), 2022 Brazilian symposium on robotics (SBR), and 2022 workshop on robotics in education (WRE). IEEE.
Lin, M., Shang, L., and Zhang, Y. (2020). Journal of Physics Conference Series. 1633. IOP Publishing.
Liu, H., Cao, S., Tan, W., and Gong, J. (2021a). International symposium on advances in informatics. Electron. Educ. 62–65.
Liu, H., Tan, W., Li, H., Gong, J., and Liu, X. (2021b). J. Phys. Conf. Ser. 1–7. doi: 10.1088/1742-6596/640/1/012013
Ma, Z., Liao, H., Gao, J., Nie, S., and Geng, Y. (2023). Physics-informed machine learning for degradation modeling of an electro-hydrostatic actuator system. Reliabil. Engin. Syst. Safety 229, 1–14. doi: 10.1016/j.ress.2022.108898
Mamedova, L., Rukovich, A., Likhouzova, T., and Vorona-Slivinskaya, L. (2023). Online education of engineering students: educational platforms and their influence on the level of academic performance. Educ. Inf. Technol. 28, 15173–15187. doi: 10.1007/s10639-023-11822-5
Mane, A., Adhikari, R., Gadgil, S., and Raykar, N., (2019). International conference on nascent technologies in the Engineering Field (ICNTE). IEEE.
Mogylenko, O., Selvaggio, A., Upadhya, S., Grodotzki, J., and Tekkaya, A. E. (2020). Augmented reality application for the Mobile measurement of strain distributions. Cross Reality Data Sci. Engin. 1231, 235–245. doi: 10.1007/978-3-030-52575-0_19
Moreno-Garcia, C. F., Elyan, E., and Jayne, C. (2018). New trends on digitisation of complex engineering drawings. Neural Comput. Applic. 31, 1695–1712. doi: 10.1007/s00521-018-3583-1
Okuno, H.R.M., Benitez, G.S., and Castillo, R.C., (2020). ICIET 2020: 2020 8th international conference on information and education technology. The Association for Computing Machinery (ACM).
Pando Cerra, P., Fernández Álvarez, H., Busto Parra, B., and Castaño Busón, S. (2023). Boosting computer-aided design pedagogy using interactive self-assessment graphical tools. Comput. Applic. Engin. Educ. 31, 26–46. doi: 10.1002/cae.22569
Patange, G.S., and Pandya, A.B., (2023). 2nd international conference and exposition on advances in mechanical engineering (ICoAME 2022). Elsevier.
Peloquin, J., Kirillova, A., Rudin, C., Brinson, L. C., and Gall, K. (2023). Prediction of tensile performance for 3D printed photopolymer gyroid lattices using structural porosity, base material properties, and machine learning. Mater. Des. 232, 1–15. doi: 10.1016/j.matdes.2023.112126
Polhmann, T., Parras-Burgos, D., Cavaz-Martinez, F., Canavate, F. J. F., Nieto, J., and Fernandez-Pacheco, D. G. (Eds.) (2020). Proceedings of the XXIX international Congress INGEGRAF, 20–21 June 2019, Logroño, Spain. Cham: Springer.
Pop, G. M., Crisan, L. A., and Tripa, M. (2019). The effectiveness of ICT tools for engineering education: ISO checker. Cham: Springer.
Prabhu, T., (2019). Principles of Mechanical Engineering: Vital Concepts of Mechanical Engineering, 3rd ed. Nestfame Creations Pvt Ltd.
Probst, A., Ebner, M., and Cox, J. (2019). International conference on interactive collaborative learning. Cham: Springer.
Qu, J., Zheng, L., Kang, Y., and Ma, B. (2022). Design and implementation of teaching assistant system for mechanical course based on Mobile AR technology. Int. J. Adv. Comput. Sci. Applic. 13, 83–90. doi: 10.14569/IJACSA.2022.0130410
Ravikumar, P., McDonald, B., Shin, I., Zbeeb, K., and Puneeth, N. (2019). Enhancing learning of kinematics and fatigue failure theories using CAD modeler. Singapore: Springer.
Razaviarab, N., Sharifi, S., and Banadaki, Y.M., (2019). Proceedings of the SPIENano-, bio-, info-tech sensors and 3D Systems III. International Society for Optics and Photonics (SPIE).
Rizvi, S. H. M., and Abbas, M. (2023). From data to insight, enhancing structural health monitoring using physics-informed machine learning and advanced data collection methods. Engin. Res. Express 5, 1–28. doi: 10.1088/2631-8695/acefae
Rodríguez-Martín, M., Rodríguez-Gonzálvez, P., Sánchez-Patrocinio, A., and Sánchez, J. R. (2019). Short CFD simulation activities in the context of fluid-mechanical learning in a multidisciplinary student body. Appl. Sci. 9:4809. doi: 10.3390/app9224809
Rossoni, M., Spadoni, E., Carulli, M., Barone, C., Colombo, G., and Bordegoni, M. (2024). Virtual reality in education to enable active learning and hands-on experience. Computer Aided Design Applic. 21, 258–269. doi: 10.14733/cadaps.2024.258-269
Sanchez, S., Rengasamy, D., Hyde, C. J., Figueredo, G. P., and Rothwell, B. (2021). Machine learning to determine the main factors affecting creep rates in laser powder bed fusion. J. Intelligent Manufact. 32, 2353–2373. doi: 10.1007/s10845-021-01785-0
Sawatzki, C.G., and Muraleedharam, R., (2021). 2021 ASEE ANNUAL conference. The American Society for Engineering Education (ASEE).
Scaravetti, D., and Francois, R. (2021). Implementation of augmented reality in a mechanical engineering training context. Computers 10, 1–15. doi: 10.3390/computers10120163
Sha, Z., Lu, Y., McComb, C., Yang, Z., Tran, A., Liu, D., et al. (2022). Promoting data science in mechanical engineering research and education: An exploration of the hackathon mechanism. Cham: Springer.
Sheng, H., and Wang, P. (2023). Evaluation of pipeline steel mechanical property distribution based on Multimicromagnetic NDT method. IEEE Trans. Instrum. Meas. 72, 1–15. doi: 10.1109/TIM.2023.3241060
Shyr, W., Yang, F., Liu, P., Hsieh, Y., You, C., and Chen, D. (2019). Development of assessment indicators for measuring the student learning effects of artificial intelligence-based robot design. Comput. Applic. Engin. Educ. 27, 863–868. doi: 10.1002/cae.22118
Singhal, I., Tyagi, B., Chowdhary, R., Saggar, A., Raj, A., Sahai, A., et al. (2022). Augmenting mechanical design engineering with additive manufacturing. Springer Nature.
Tsai, Y, Wang, C, Peng, H, Huang, H., and Ching-Piao, T., (2018). International conference on innovative technologies and learning. Springer Nature.
Tudic, V., Kralj, D., Hoster, J., and Tropcic, T. (2022, 5). Design and implementation of a ball-plate control system and Python script for educational purposes in STEM technologies. Sensors 22. doi: 10.3390/s22051875
Vogel-Heuser, B., Land, K., Hujo, D., and Kruger, M. (Eds.) (2022). IEEE international conference on automation science and engineering (CASE) Mexico city. Mexico: IEEE.
Wang, T., and Ahmad, R. (Eds.) (2020). Proceedings of the Latin American symposium on industrial and robotic systems (LASIRS), held in Tampico, Mexico on October-November 30-01 2019, Springer. Cham: Springer Nature.
Wei, Z., and Berry, C. (2018). ASEE Conference and Exposition. American Society for Engineering Education (ASEE). 1–19.
Win, L. L., Abdul Aziz, F., Hairuddin, A. A., Abdullah, L. N., Yap, H. J., Saito, H., et al. (2022). Effectiveness on training method using virtual reality and augmented reality applications in automobile engine assembly. ASEAN Engin. J. 12, 83–88. doi: 10.11113/aej.v12.18009
Yengui, M. H., and Stechers, C. (2022). IEEE International Conference on Industrial Engineering and Engineering Management. IEEE. 32–36.
Yuan, F., Shen, X., Wu, J., and Yang, W. (2023). 2023 2nd international symposium on control engineering and robotics (ISCER) 2023. IEEE. 270–273. doi: 10.1109/ISCER58777.2023.00054
Zhai, X., Chu, X., Chai, C. S., Jong, M. S. Y., Istenic, A., Spector, M., et al. (2021). A review of artificial intelligence (AI) in education from 2010 to 2020. Complexity 2021, 1–18. doi: 10.1155/2021/8812542
Keywords: mechanical engineering, education, artificial intelligence, machine learning, educational automation
Citation: Alghazo M, Ahmed V and Bahroun Z (2025) Exploring the applications of artificial intelligence in mechanical engineering education. Front. Educ. 9:1492308. doi: 10.3389/feduc.2024.1492308
Received: 06 September 2024; Accepted: 20 December 2024;
Published: 07 February 2025.
Edited by:
Xinyue Ren, Old Dominion University, United StatesReviewed by:
Y. P. Tsang, Hong Kong Polytechnic University, Hong Kong SAR, ChinaCopyright © 2025 Alghazo, Ahmed and Bahroun. This is an open-access article distributed under the terms of the Creative Commons Attribution License (CC BY). The use, distribution or reproduction in other forums is permitted, provided the original author(s) and the copyright owner(s) are credited and that the original publication in this journal is cited, in accordance with accepted academic practice. No use, distribution or reproduction is permitted which does not comply with these terms.
*Correspondence: Zied Bahroun, emJhaHJvdW5AYXVzLmVkdQ==
Disclaimer: All claims expressed in this article are solely those of the authors and do not necessarily represent those of their affiliated organizations, or those of the publisher, the editors and the reviewers. Any product that may be evaluated in this article or claim that may be made by its manufacturer is not guaranteed or endorsed by the publisher.
Research integrity at Frontiers
Learn more about the work of our research integrity team to safeguard the quality of each article we publish.