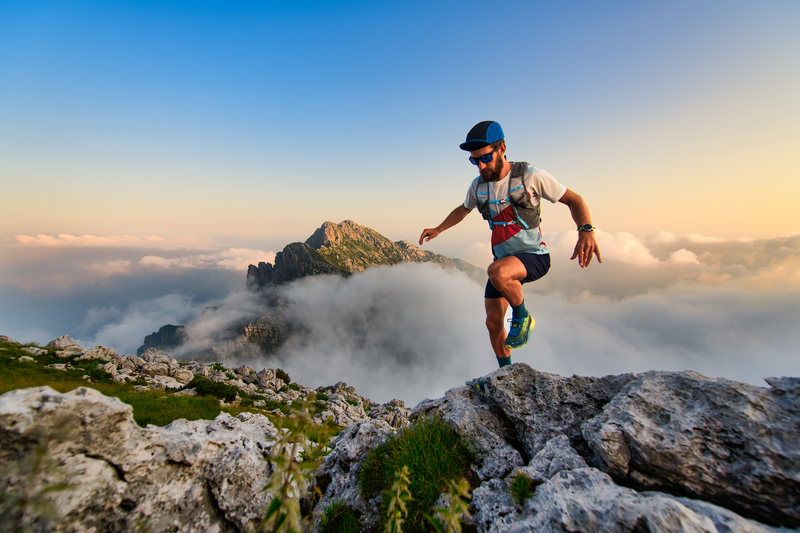
95% of researchers rate our articles as excellent or good
Learn more about the work of our research integrity team to safeguard the quality of each article we publish.
Find out more
ORIGINAL RESEARCH article
Front. Educ. , 17 October 2024
Sec. Mental Health and Wellbeing in Education
Volume 9 - 2024 | https://doi.org/10.3389/feduc.2024.1477659
This article is part of the Research Topic Psychoeducational Approaches to Mental Health for Educators and Students View all 14 articles
COVID-19 has sparked a significant shift in education toward the use of eLearning platforms. Inclusive teachers encounter several challenges when adopting eLearning platforms for education. The purpose of this study was to examine factors influencing inclusive teachers’ acceptance of eLearning platforms according to the unified theory of acceptance and use of technology (UTAUT2) model. Overall, 128 Omanis inclusive teachers completed the survey questionnaire. The findings revealed the general acceptability of eLearning platforms. Higher performance expectancy, facilitating conditions, social influence, and service quality were all significant predictors of the eLearning platform on behavioral intention. Moreover, the findings showed that further support and training are needed for inclusive teachers to use eLearning systems.
In educational systems across the world, inclusive education (IE) is becoming increasingly important. The universal drive to promote inclusive education has progressed due to a rights-based perspective on education and a change in the perception of disability (Chua and Bong, 2024). In inclusive education, all students are treated equitably and have equal access to learning in the regular classroom regardless of students’ differences or students’ unique characteristics, interests, abilities, and learning needs (Alnahdi and Schwab, 2021; Makoelle, 2016). Inclusive teachers are defined as teachers who possess the teaching skills of teaching regular students and students with special educational needs (SEN) in the same classroom (Faragher, 2023). This involves adapting the curriculum content of what is being taught in the classroom, using special tools to help learners, and creating or maintaining a proper learning atmosphere that would enable all learners to feel valued and included (Scanlon et al., 2022). Inclusive teachers consider implementing and teaching inclusive pedagogical practices that can allow every student, especially those with special educational needs (SEN), to have full participation in the learning process (Halder, 2023).
To limit the epidemic in the aftermath of COVID-19, educational institutions, including schools, were obliged to transition from traditional teaching methods to totally remote teaching delivery around the world (Alam, 2021). One of the containment measures taken by governments in various nations was virtual learning through learning management systems (LMSs) as a vital instrument for teaching and learning activities. LMSs, which are widely known as eLearning platforms, arose as a new type of education system that was popularly recognized and was utilized by many people in the world of education to offset the negative repercussions of learning free from disruptions due to school closures (Prasetyo et al., 2021). An online platform allows students and instructors to interact, discuss, and conduct class discussions digitally in the same way that they would in a traditional classroom (Al-Fraihat et al., 2020). Teachers can obtain critical content for their teaching tasks and professional development from the systems/websites; download the material; adhere to the schedule; participate in class discussions; interact with the instructors; and incorporate formative assessment methodologies into eLearning (Camilleri and Camilleri, 2022; Prasetyo et al., 2021). This might contribute to better communication with the students and create an interactive learning environment despite some inherent obstacles brought by this time of crisis (Coman et al., 2020).
Implementing inclusive pedagogical practices through eLearning platforms (LMS) provides substantial benefits for both regular students and those with special educational needs, however, it poses various challenges for including inclusive teachers, such as lack of technological infrastructure and support, lack of online teaching skills, and lack of experience with digital technologies (Camilleri and Camilleri, 2022). This might happen due to several factors. Firstly, the lack of inclusive teachers’ experience in using eLearning platforms. In this regard, the findings of a study conducted by Burić et al. (2021) stated that teachers had to switch to using digital resources and eLearning platforms literally overnight, who had not previously even considered that instruction could be carried out online. Thus, it can be inferred that teachers were unprepared to teach inclusive contexts that could satisfy the requirements of SEN for an entirely online experience (Kozleski, 2020; Rice, 2017). Second, to promote access and equity between different groups of learners, SEN and regular students, inclusive teachers must be proactive in learning to use and modify digital resources in a curriculum, resource design on LMSs, student support systems, and the balance between synchronous and asynchronous learning experiences, which need a long time to adapt their teaching style to the new conditions (Thomas and Bryson, 2021). Finally, these sorts of barriers could be inappropriate policy development issues, teacher attitudes, lack of teacher training, inadequate support, and resources, as well as curriculum issues besides that level of awareness to create activities suitable for all types of students (Mokaleng and Möwes, 2020). Most importantly, for delivering a high-quality education and a successful implementation through eLearning platforms, are teachers’ acceptance and adoption of the platform (Aldossry, 2021). According to Zulherman et al. (2021), using eLearning platforms necessitates knowledge and skills about how users interact with technology.
Considering the aspects, we believe that transitioning to solely eLearning will have a substantial impact on the educational process and inclusive teachers’ acceptance of the use of eLearning platforms in inclusive classrooms, and these beliefs form the foundation of our research. We thought it was important, relevant, and necessary to investigate the factors that promote efficient use of eLearning platforms in inclusive classrooms during the teaching and learning process including educational system quality (ESQ) and service quality (SQ) (Alduaij et al., 2024), as well as factors from the unified theory of acceptance and use of technology (UTAUT2) (Venkatesh et al., 2012), to understand inclusive instructors’ acceptance of using eLearning platforms in their inclusive classes.
The acceptance of eLearning platforms by inclusive instructors is significantly influenced by the perceived educational system quality (ESQ) and service quality (SQ). Educational system quality is a construct that summarizes the following features of the e-learning system: effective communication, diversity of learning styles, evaluation components, curriculum design, technological infrastructure, and pedagogical strategies, ensuring that the platform aligns with the educational goals and needs of diverse learners (Pizarro-Uy and Manapat, 2023). Support system quality concerns the ethical issues, behavioral considerations, legal issues, and promotion of the e-learning system (Ozkan and Koseler, 2009). Service quality, which includes aspects such as providing guidance services, technical support, user-friendliness, staff availability, fair understanding, responsiveness, and ongoing professional development, plays a critical role in instructors’ willingness to adopt and effectively utilize these platforms (DeLone and McLean, 2003; Mathew et al., 2020).
Moreover, the UTAUT2 model has dominated the research landscape in terms of teachers’ acceptance of technology in the classroom, owing to its adaptability to different contexts and flexible samples, ability to explain variance in the intention to use and with the use of technology, and ease of specification within structural equation modelling frameworks (Šumak and Šorgo, 2016). In 2003, UTAUT originally comprised four key factors: performance expectancy, effort expectancy, social influence, facilitating conditions, hedonic motivation, all of which influence the behavioral intention to use technology. This idea was discovered most recently in 2012, when three new UTAUT Model constructs were revealed. Hedonic motivation was the initial structure, followed by price, and finally tradition (Venkatesh et al., 2012). According to Zulherman et al. (2021), the use of Zoom Cloud Meetings during the COVID-19 pandemic was influenced by factors such as ease of use, perceived usefulness, and the extent of remote communication needs. These factors significantly contributed to the spread of utilization of Zoom in educational settings.
Various studies are available in the extant literature on adopting technology. Among them, UTAUT is a widely accepted model for explaining technology acceptance by users (Venkatesh et al., 2012). UTAUT2 has been extensively applied in school settings, specifically among primary school teachers (e.g., Chen, 2020; Dindar et al., 2021; Jevsikova et al., 2021; Ling et al., 2016; Marcinkovic et al., 2021; Saal et al., 2020), secondary school teachers (Zalah et al., 2017), and high school teachers (e.g., Abusobaih et al., 2021; Holzmann et al., 2020; Zulherman et al., 2021). Moreover, recent studies highlight that a higher perception of ESQ and SRQ leads to increased acceptance and usage of eLearning platforms by inclusive instructors, as these factors directly impact their confidence and perceived ease of integrating technology into their teaching practices (Alduaij et al., 2024; Rosetta et al., 2020). Though studying eLearning platform acceptance of inclusive teachers has been applied to a limited extent during their teaching, particularly in the Oman context, necessitating even more studies about using technology in inclusive classrooms. Drawing on this, the current study investigates inclusive teachers’ acceptance of using eLearning platforms in the classroom.
In doing so, this study contributes to the existing body of knowledge in multiple ways, paving the way to obtain a deeper understanding of the dynamics of eLearning platform adoption in a classroom from the perspective of a developing country. The acceptability of eLearning platforms among inclusive teachers in Oman is the subject of this study, which is the first of its kind. Moreover, enhance the contemporary literature by conducting a more thorough examination into the factors that are deemed accountable for inclusive schoolteachers’ acceptance of an online learning platform in Oman. Finally, they shed light on future trends in classroom development for multi-disciplinary and multi-faceted e-learning. Therefore, the research questions guiding this study are:
Rq1: Is there any effect of performance expectancy (PE), effort expectancy (EE), social influence (SI), facilitating conditions (FC), hedonic motivation (MH), educational system quality (EYQ), and service quality (SQ) on inclusive schoolteachers ‘actual use of the eLearning platform during the COVID-19 pandemic in Oman?
Rq2: Are there any relations between participants in terms of gender, age, and years of technology experiences on behavior intention to using eLearning platforms?
The rest of this paper is laid out as follows: Section two introduces the research framework, which contains the conceptual model and hypotheses. In the third section, the survey, sample composition, and analysis technique are all described. The main results are explained in Section 4, and the relevance of the findings to the existing literature and the managerial implications for service providers are discussed in Section 5. Finally, Section six brings the report to a close and suggests areas for future research.
Figure 1 depicts the study’s proposed conceptual framework, supported by Ain et al. (2016). UTAUT2 was used to create the suggested framework. Given the maturity and breadth of applications for evaluating user acceptability, the researchers employed the most essential factors of the UTAUT2 to explain behavioral intention to actual use of the eLearning platform (Tamilmani et al., 2021). Performance expectancy, effort expectancy, social effect, facilitating conditions, and hedonic motivation are regarded as predictors of intention to use eLearning platforms since the researchers assume high reliability of these factors for an already spread technology and service (Prasetyo et al., 2021).
PE is defined as the degree to which an individual believes that using a system would help them improve their job performance (Venkatesh et al., 2012). In the context of this study, it represents the extent to which inclusive schoolteachers believe that eLearning platforms will enable them to perform their teaching activities much better than before, complete daily lessons, broaden their understanding of studies, assist with task completion, and improve their teaching performance. Several studies examined the effect of performance expectations on schoolteachers’ acceptance of technology use in various contexts and applications. For example, Abusobaih et al. (2021) found that performance expectancy was one of the strongest predictors of teacher acceptance of Lego sets in the classroom. Dindar et al. (2021) discovered that the performance expectancy of K-12 instructors who will utilise LMS in the future was the most significant predictor of behavioral intention. Veiga and Andrade (2021) obtained the same results. Consequently, the following hypothesis is proposed:
H1: Performance expectancy (PE) had a positive effect on inclusive schoolteachers’ behavioural intention (BI) to actual use of the eLearning platform.
EE is one of the factors that influences the intention to use eLearning platforms and technologies (Gunasinghe et al., 2019). EE is defined as “the degree of ease with which technology is utilised and related” (Venkatesh et al., 2003). In line with Davis (1989), an individual’s readiness to adopt a new system is influenced not just by how valuable the system is but also by how simple it is to use and how much it requires free effort. In this study, the aim of EE is to discover schoolteachers’ perspectives on whether learning using an eLearning platform is simple, which necessitates a particular degree of knowledge and skills expectations that can influence teachers’ intentions to use these technologies (Abusobaih et al., 2021; Dahri et al., 2021). Similar results were obtained by Marcinkovic et al. (2021) and Zulherman et al. (2021), who found that the ease with which the instructor views the use of technology in the classroom is a crucial determinant of acceptance for acceptability. The following hypotheses are taken from this study:
H2: Effort expectancy (EE) had a positive effect on inclusive schoolteachers’ behavioural intention (BI) to actual use of the eLearning platform.
Social influence refers to “the degree to which users perceive essential people (such as family and friends) believe they should utilise a specific technology” (Venkatesh et al., 2012). According to a previous study, users are more likely to adopt technology if it has been used by others in their environment. In the context of inclusive education, it represents the degree to which inclusive schoolteachers assign significant weight to the opinions of important people (e.g., school principal, SEN experts, colleagues) when using eLearning platforms. They are more likely to follow their suggestions (Holzmann et al., 2020; Jevsikova et al., 2021; Veiga and Andrade, 2021). In this regard, social influence is one of the factors in the redesigned study model that should be included. The hypothesis is derived as follows:
H3: Social influence (SI) had a positive effect on inclusive schoolteachers’ behavioural intention (BI) to actual use of eLearning platform.
Facilitating conditions (FC) are described by Venkatesh et al. (2003) as an individual’s opinion as to whether the organisation delivers technology that facilitates the use of an eLearning platform. This study examined whether inclusive school instructors met the following criteria for using eLearning platform resources when utilising eLearning platforms to overcome hurdles to their involvement with technology, especially during the early phases of adoption. Positive attitudes toward device functionality or supporting infrastructure may help users feel less anxious about technology. Support workers and their constant guidance, according to Venkatesh et al. (2003), play a critical role in assisting users in overcoming technological challenges. As a result, the amount of assistance inclusive teachers can receive may have an impact on their intention of using the technologies and platforms. Several academics have suggested that supportive settings can improve a teacher’s ability to use technology (Dahri et al., 2021; Zulherman et al., 2021). The hypothesis is derived as follows:
H4: Facilitating conditions (FC) had a positive effect on inclusive schoolteachers’ behavioural intention (BI) to actual use of the eLearning platform.
HM is defined by Venkatesh et al. (2012) as “happiness gained through the use of technology.” Playfulness, pleasure, enjoyment, and entertainment are all examples of hedonic motivation. More specifically, enjoyment refers to how much pleasure a person gets from using technology (Van der Heijden, 2004). Indeed, the significance of hedonic motivation in formalising teachers’ intention to accept technology is well supported in the information systems literature (Alalwan et al., 2017). According to Kang et al. (2015), hedonic incentives determine behavioral intention. In a similar vein, Zulherman et al. (2021) found that during the COVID-19 outbreak, hedonic motivation was the only behavioral intention motivator for teachers using the Zoom platform. As a result, this research claims that interest is necessary to create positive emotions that can play an important role in formalising inclusive teachers’ acceptance of technology. Thus, the following hypothesis was proposed:
H5: Hedonic motivation (HM) had a positive effect on inclusive schoolteachers’ behavioural intention (BI) to actual use of the eLearning platform.
Educational system quality (ESQ) measures the quality of an educational system, such as interactivity and communication components, attendance, collaboration, assessment content, and a range of learning styles that all contribute to an educational system’s overall excellence. According to Al-Fraihat et al. (2020), the diversity of learning styles, the availability of interaction features and communication tools, and the evaluation materials with provision to students (e.g., assignments and quizzes), all these are very significant in their usage of an eLearning system. When constructing a methodology for measuring the acceptance of eLearning in Iranian institutions, Hassanzadeh et al. (2012) discovered that educational system quality positively and directly affects user satisfaction and, indirectly, the use of the system. Satisfaction and a quality educational system are linked with each other (Kim et al., 2012). These statements demonstrate that the eLearning system can result in users maximising the educational features (e.g., discussion forums, chat rooms, and collaborative learning tools, videoconferences). The following hypotheses are proposed accordingly.
H6: The educational system quality (ESQ) had a positive effect on inclusive schoolteachers’ behavioural intention (BI) to actual use of the eLearning platform.
Serving Quality (SQ) measures the quality of eLearning system services related to assurance, functionality, interactivity, tangibleness, dependability, responsiveness, and empathy (DeLone and McLean, 2003). Delivering services by IT employees in the organisation, whether connected to an information system or an eLearning system, is likely to benefit learners and improve their perceptions of the system. In the realm of information systems, the construct has been employed. In their examination of eLearning system success, Al-Fraihat et al. (2020) substantiated the positive relationship between SQ and perceptions. Simply put, the better eLearning platforms are, the more accurate, engaging, responsive, and dependable they are. The more frequently and over a longer period of time that inclusion schoolteachers employ online learning, the better. As a result, the following hypotheses are put forth:
H7: The service quality (SQ) had a positive effect on inclusive schoolteachers’ behavioural intention (BI) to actual use of the eLearning platform.
Wrzosek et al. (2020) defined behavioral intention as “the degree of the teacher’s intentions to use the eLearning platform in the future.” In the same way, Venkatesh et al. (2003) proposed BI as having a significant positive impact on the utilisation of information technology. Several researchers have found that behavioral intentions have a considerable impact on actual system use, according to Ain et al. (2016). According to DeLone and McLean (2016), actual use refers to the frequency, nature, and length of an individual’s use of an information system’s capabilities. The frequency and duration of use in online learning are also indicated by actual usage (Kim et al., 2007). Therefore, we hypothesized that behavioral intention directly affects actual use, as follows:
H8: Behavioural intention (BI) had a positive effect on inclusive schoolteachers’ actual use of the eLearning platforms.
H9: There are differences in participants’ intentions to actual use of the eLearning platform based on gender, age, and years of technology experience.
For this study, the model’s postulated variables and their relationships were developed from the existing literature of models and theories given in the above-mentioned literature. Figure 1 depicts the proposed extended model.
Employing quantitative research methods, we determine the factors influencing inclusive teachers’ acceptance of using eLearning platforms in online classrooms. A nonprobability, self-selection sampling strategy was used in this investigation. The sample includes 128 high school teachers working in inclusive classrooms from 12 schools supporting students with mental health needs and 8 schools with deaf students in the State of South Al-Batinah, Oman, during the 2021–2022 academic year. Inclusion classrooms refer to educational settings where students with mental health needs or deaf and hard-of-hearing students are integrated with their peers (Ministry of Education in Oman, 2022). The sample size of 128 inclusive high school teachers has been chosen because this is the total number of educators employed in inclusive classrooms in the State of South Al-Batinah, Oman, during the 2021–2022 academic year. The small sample size is therefore a reflection of the actual number of inclusive teachers available in the region and not a result of selective sampling. Inclusive high school teachers were deliberately chosen for this study due to high school being a transitional period where students prepare for higher education or entry into the workforce, making the role of inclusive teachers especially crucial. This focus allows the study to gain insights into the unique challenges and strategies at this educational level, which may differ significantly from those in other educational stages. The participants had previous experience with using the Mansara platform (Ministry of Education in Oman, 2024) to modernize the educational system, particularly in light of technological advancements and the challenges posed by the COVID-19 pandemic in an eLearning environment. Before the collection of data, the ministry of educational authorities’ permission was obtained. The purpose of the research was discussed with inclusive education teachers. The data were collected with a survey questionnaire that was created by Google Forms.
The operationalization of the extended UTAUT2 model relied on the item scales, which stemmed from the corresponding literature for PE, EE, SI, FC, and HM, with additional ESQ, SQ, and BI as shown in Table 1. The relevant measures and scales were adopted and slightly adjusted to illustrate the consistency and validity of the study topics. Changes were made to reflect the eLearning platform’s particular context in the educational setting. The questionnaire was conducted in Oman’s South Al-Batinah region and consisted of three sections: The first part contains the item scales of the UTAUT2 model with an additional two variables: educational system quality (ESQ) and service quality (SRQ), measured on a 5-point Likert scale ranging from 1 (strongly disagree) to 5 (strongly agree). The second section contains the demographic profile questions, namely age, gender, year of technology experience, level of education, and the time spent using an eLearning platform. The items of each construct were constructed in the third section using the UTAUT2 model with two extra variables: (ESQ) and (SQ). A total of 36 items were measured on a 5-point Likert scale, as shown in Table 1. The questionnaire’s theoretical underpinning was the UTAUT2 model. To ensure clarity and legibility, the pilot survey’s recommendations led to the revision and alteration of a few items (see Table 2).
The data was analyzed using the structural equation modelling (SEM) based on the AMOS technique to construct several model assessment metrics using the bootstrapping method. As stated in Table 3, a confirmatory factor analysis (CFA) was used to test the measurement model. The dependability was deemed satisfactory. “Cronbach’s alpha and Composite Reliability” (CR) values ranged from −1.69 to 8.21, and the average variance extracted (AVE) values were greater than 0.50 (between.60 and.79). In terms of multicollinearity risk, the inflation factor (VIF) values were larger than.20 but less than 6 (between 2.52 and 5.39), indicating a non-significant multicollinearity risk (Carrión et al., 2016). As outer weights dropped between.95 and.87, convergent validity was proven, which exceeded the cut-off value of.70 (p < 0.001) (see Figure 1).
The Fornell-Larcker criterion was used to assess discriminant validity. The AVE’s minimum square root value along the diagonal line (0.83) was higher than the latent constructs’ highest correlation value (0.79). The components’ inter-item correlations ranged from.60 to.79, with squared correlations of less than 0.8, meeting scale development standards (Harlow et al., 2002) (see Figure 2).
Figure 2. Structural equation modelling (SEM) (Alavi et al., 2020).
A minimum of 30 respondents is regarded as sufficient for a pilot study (Fan et al., 2016). As a result, the researchers conducted a pilot test on 40 Omani school teachers. 11 respondents are between the ages of 23 and 30, 15 are between the ages of 31 and 40, and the remaining 14 are over the age of 40. PE (0.95), EE (0.94), SI (0.94), FC (0.94), HM (0.94), ESQ (0.94), SQ (0.94), USE (0.94), and BI (0.94) are the average reliability values for all constructs, according to Cronbach’s Alpha Coefficient (0.95). In general, reliability scores above 0.7 indicate that the UTAUT2 constructs have a good level of internal consistency when utilising the SPSS 25 software. As a result, his modified UTAUT2 model can be accepted in the computer technology adoption literature (Xiong et al., 2015).
Several more criteria were used to assess the relevance of the current findings obtained by factor analysis. The Kaiser-Meyer-Olkin (KMO) sampling size adequacy measure, as well as the Barlett’s test, were used to see if 40 instructors were enough to perform a successful factor analysis. The KMO values in this study, as shown in Table 4, were all greater than 0.6, and thus greater than 0.5, which is the recommended minimum standard quantity or value for sufficiency (Wu et al., 2007). Furthermore, the results of the Bartlett’s test were significant, indicating that these 9 core constructs were adequate and fit for component analysis. PE, EE, SI, FC, HM, ESQ, SQ, and USE are the abbreviations for PE, EE, SI, FC, HM, ESQ, SQ, and USE are the five independent variables (exogenous variables), whereas B1 is the only dependent variable (endogenous variable).
In the evaluation of the results, the sample data collected for experimental analysis included 128 inclusive high school teachers, 56 (43.8%) male and 72 (56.3%) female participants. As per the age groups of high school teachers, 57 (44.5%) are aged 31–40 years, 37 (28.9%) are aged 41–50 years, and 29 (22.7%) are aged 20–30 years. Based on platform frequency for teaching purposes, 24 (18.8%) respondents used the platform for teaching purposes 1–2 times per month, 42 (32.8%) 3–6 times per month, 23 (18%) 7–12 times per month, 17 (13.3%) more than 12 times, and 22 (17.2%) daily because they were interested in using technology in teaching. Lastly, the time usually spent using an eLearning platform was determined to be less than 1 h using an eLearning platform, 57 (44.5%) using a platform for 1 to 2 h, 35 (27.3%) using a platform for 3 to 4 h, and 10 (7.8%) spending more than 4 h using a platform for their teaching. However, when it comes to online learning platforms, 27 (21.1%) use Manzara and 101 (78.9) use Google Classroom. Table 4 gives details of the demographic information in a nutshell.
The model was created in AMOS, and the diagram is presented below, along with the standardized parameter estimates. The observed variables are represented by squares, whereas the error terms are represented by circles. Three latent variables are assumed, and they are derived using nine confirmatory factor analyses. These latent variables are represented by ovals. After the initial model fitting without any associated error terms, SEM proposed the correlation structure between the error terms of the confirmatory factor analysis. This helps to improve the model’s overall fit.
To answer the first research question, this part uses SEM to determine the link between the constructs proposed by UTAUT2. Table 5 shows the result. In this study, a few of the most frequently used goodness-of-fit markers are observed, as advised by most SEM scholars (Alavi et al., 2020). Both the Non-Normed Fit Index (NFI) and the Comparative Fit Index (CFI) proposed by Schuhen and Schürkmann (2016) must have index values greater than 9, and the Root Mean Squared Approximation of Error (RMSEA) must be less than 0.6. Finally, the Goodness of Fit Index (GFI) must run on a continuum from 0 to 1, and the Root Mean Squared approximation of Error (RMSEA) must be less overall. The CFA results for model fitness in Table 5 show that most of the indexes achieve acceptable levels, indicating that this structural model is well-fit between most of the items in this study and Venkatesh et al. (2012) UTAUT2 model. NFI, GFI, CFI, and RMSEA scores are all admissible in the evaluation model’s nine basic concepts. Factor loadings are substantial, with reliability ranging from 0.94 to 0.95, all over 0.7, and the average variance extracted (AVE), all exceeding 0.5.
Table 5. CFA for the structural equation modelling (Schuhen and Schürkmann, 2016).
Therefore, convergent validity was used in the UTAUT2 model to identify the strengths and limits of the five basic ideas assessed in the study. In fact, most of their actual values were better than the recommended values, implying that the new structural model has strong fit data and is appropriate for usage in Oman.
The degree of fit between the suggested structural framework and the emerging data structure is measured using SEM. According to the CFA results in Table 5, all the NNFI, GFI, CFI, and RMSEA values in this pilot study of 40 respondents are appropriate and acceptable, as they meet the criteria of a good-fit model. NNFI has been noticed and is highly recommended since it is tolerant of a wide range of sample sizes, including small sample sizes. After comparing the updated UTAUT2 model’s fit to a null model as a nested baseline, the NNFI, or Tucker-Lewis index, is calculated. Furthermore, as the null model degrees of freedom are evaluated, NNFI also assesses parsimony. This index requires a value of 0.90 or above to be considered acceptable, and all the NNFI values in Table 5 meet this condition, suggesting a satisfactory match.
The GFI for all the model’s constructs, including “behavioral intention,” is greater than.95, indicating an excellent level of fit. Bentler (1990) suggested the CFI as a non-centrality parameter-based indicator for dealing with the influence and weakness of a limited sample size. This index ranges from 0 to 1, with a cut-off value of.90 or higher indicating that the model is well-fitting. All the major constructs in this study have a perfect 1.00 index, except for SI, which has a.94 index, indicating an acceptable fit.
When evaluating a model fit, one of the most informative criteria is the RMSEA. It also calculates the degree of variation of the estimated covariance matrices from the observed covariance matrices per degree of freedom, not only for the sample but also for the entire population (Xiong et al., 2015). As a result, every value of the fit index estimates better, and the size of the sample has no effect because each RMSEA value ranges from 0 to 1.
The S.E. shows that there is less deviation in the computation of the facilitating condition (FC) by 0.19, while the teachers’ social influence (SI) has less deviation by 0.15. However, performance expectancy (PE) has less deviation by 0.11, while service quality (SQ) has less deviation by 0.17. Likewise, educational system quality (ESQ) has less deviation by 0.31, while hedonic motivation (HM) has less deviation by 0.18. In addition, effort expectancy (EE) has less deviation in the computation by 0.19, whereas actual use of the platform has less deviation by 0.06.
The p-value shows that as for each FC, SI, PE, SQ, and actual use of the platform, the significance value is less than the significance level of the study 0.05. However, for the ESQ, HM, and EE, the p-value shows that the significance value is greater than the significance level of the study 0.05. This result is further verified by the z-score value (C.R.), i.e., −1.69 for FC, 0.71 for ESQ, 1.38 for HM, and 0.78 for EE, which is less than the tabulated Z-value of 1.96. However, 1.99 for SI, 2.42 for PE, 2.12 for SQ, and 8.21 for actual use of the platform, which is greater than the tabulated Z-value of 1.96. As a result, in the current study, the analysis of respondent’s perceptions reveals that educational system quality level, effort expectancy, and hedonic motivation have no significant influence on behavioral intention. However, for the other variables such as facilitating conditions, social influence, performance expectancy, service quality, and actual use of the platform, they have a significant influence on the behavioral intention.
In addition to the good fit model indices, SEM must be used to measure the causal links between the routes. Path analysis was performed on the structural model, specifically on its concept variables, using SEM. The variables are assessed not only for their statistical significance but also for their strength path, which includes standardised coefficients between-1 and 1. The regression results are comparable when standardised, which may help us identify more important components and correlations. Table 6 shows all the correlations between the independent and dependent variables. Actual is one of the eight variables that have the most influence on the BI to be used. As shown in Table 7, the platform has a path coefficient of 0.81 while SQ, SI, PE, FC, and HM have a path coefficient of 0.30, 0.26,0.25, −0.26 and 0.17, respectively. In addition, ESQ and EE, have a path coefficient of 0.14 and.12, respectively. Four of the eight hypotheses (H1, H3, H7, and H8) are found to be statistically significant (p 0.05) in this path analysis model. This shows that the items in this study and the UTAUT2 model are well-matched.
Many potential users are misinformed about SEM and are hesitant to use it because they believe sample sizes should be in the hundreds. Nonetheless, if the variables are reliable, the path will be strong, and there will be no overlaying complications, then samples of lesser size will be sufficient (Civelek, 2018). If the sample size is increased, this could be an appropriate model since the study will be able to explain the causal effect of latent variables like PE, EE, SI, FC, SQ, ESQ, and HM on BI to utilise an eLearning platform among Oman’s teachers. In conclusion, the SEM analysis supported the hypotheses H1, H3, H7, and H8, but not supported H2, H4, H5, and H6.
To answer the second research question, the researcher ran with ANOVA by checking the verification of the homogeneity of variance between the gender, age, and years of technology experience on the behavior intention by using the Levene’s Test. The results obtained are as shown in Table 8.
Table 8 shows that there is no significant difference between male and female for overall behavior intention to use eLearning platforms (F (126) = 0.003, p = 0.96 > 0.05). While the age value was F (124) = 0.35, p = 0.79 > 0.05, demonstrating that there was no significant difference between participants for the overall behavior intention using eLearning platforms. The value for years of technology experience, on the other hand, was F (124) = 1.59, p = 0.19 > 0.05, indicating that there is no significant difference between participants for the overall behavior intention of using eLearning platforms.
To investigate the significant differences between the means of the gender, age, and years of technology experience on behavior intention towards using eLearning platforms, analysis of variance (ANOVA) was used at the level of significance (0.05). The results obtained are as shown in Table 9.
Table 9. ANOVA results for the gender, age, and years of technology experiences on behavior intention to using eLearning platforms.
The value F (1, 126) = 1.71, p = 0.19 > 0.05 in Table 9 indicates that there is no significant difference between the mean of the male and female in the overall behavior intention to use eLearning platforms. However, for the age group, the value F (3, 124) = 5.78, p = 0.001 < 0.05, indicates a significant difference in the overall behavior intention to use eLearning platforms in favor of participants between the ages of 41 and 50. Furthermore, the value for years of technology experience, F (3, 124) = 5.14, p = 0.002 < 0.05, indicates a significant difference in the overall behavior intention to use eLearning platforms in favor of participants with more than 15 years of technology experience. However, for H9, there were differences in participants’ behavior intention to use eLearning platforms based on (gender, age, and years of technology experience), which was supported for age and years of technology experience but not supported for gender.
The purpose of the current study was to investigate the factors influencing inclusive teachers’ acceptance of using eLearning platforms in the classroom in the context of online teaching during the COVID-19 pandemic. The study aimed to investigate the factors influencing inclusive teachers’ acceptance of using eLearning platforms in the classroom during the COVID-19 pandemic. The findings predicted that performance expectancy (PE) had an important effect on teachers’ behavioral intention (BI), as teachers who comprehend what technology can do during online learning and communicate with both groups, students with SEND and general students, are more effective in using the eLearning platform. However, previous studies have reported no significant relationship between performance expectancy and the behavioral intention of teachers to use the platform. Effort expectancy (EE) did not support the hypothesis (H2) set, which could be explained by the COVID-19 teaching requirements and teaching experience, which are new to all-inclusive teachers. The new teaching requirements, such as learning to teach virtually, learning new technology, and adapting lesson plans for virtual and hybrid instruction, may have an impact on experienced teachers who have not had prior mastery experience teaching virtually or hybrid classes or using various virtual platforms. Most inclusive instructors were in their 20s and 40s, so they had significant knowledge of it in their daily lives.
Social influence (SI) had a significantly positive effect on inclusive teachers’ BI to use eLearning platforms (H3), suggesting that the socio-cultural environment might help support and encourage inclusive teachers to adopt new technology and platforms in their classrooms. These results corroborate the results obtained by Dahri et al. (2021), Holzmann et al., 2020, Hsu (2016), Jevsikova et al. (2021), Ling et al. (2016), Lopez-Perez et al. (2019), Marcinkovic et al. (2021), Mohd et al. (2019), Raman and Thannimalai (2021), Turki and Sathiyanarayanan (2018), Veiga and Andrade (2021), and Zulherman et al. (2021). Facilitating conditions (FC) was not a significant predictor of BI to use eLearning platforms for inclusive teachers (H4). However, studies conducted by Abusobaih et al. (2021), Hsu (2016), Jevsikova et al. (2021), and Zulherman et al. (2021) showed that FC has a negative effect on the teachers’ BI to use Lego sets and distance learning technology (DLT). Hedonic motivation (HM) failed to receive support (H5), contrary to our prediction. This result is in contrast with previous studies, which revealed that hedonic motivation has a positive significant effect on teachers’ behavioral intention to adopt and use technology. E-content and a user-friendly environment have the greatest influence on producing delightful learning experiences (Warnecke and Pearson, 2011), and instructional designers should take these characteristics into account. Educational system quality (ESQ) had a negative effect on inclusive teachers’ behavioral intentions (H6), possibly due to their lack of preparedness to conduct online educational activities with students with severe impairments and difficulty controlling devices on their own. Service quality (SQ) was also found to be a significant variable, describing the behavioral intention to use eLearning platforms in the educational context (H7). High-quality services by technical experts should be accessible when needed, and technology has an influence on supporting teachers by providing essential training and instruction on how to use the eLearning system.
Finally, behavioral intention was shown to be highly influential in determining the actual use of the eLearning platform (H8). As conceptualized in the UTAUT2 theory of Venkatesh et al. (2012), this result is in line with previous studies using the same theoretical framework (Ain et al., 2016; Azizi et al., 2020; Chipeva et al., 2018; Raman et al., 2022). The findings of the demographic variables revealed that there are no differences in behavior intention to use eLearning platforms between males and females. This finding is consistent with that of Tarhini et al. (2014), who showed that gender had no significant moderating impact on the desire to adopt and utilize a system. This finding contrasts with a prior study by Venkatesh et al. (2003), which found that males and females make distinct decisions and employ different socially formed cognitive frameworks. This is in accordance with Cacciamani et al. (2018), who said that even though males and females are about equal in number, gender discrepancies have been observed with greater levels of acceptability.
Moreover, the findings demonstrated that participants’ behavior intentions to use eLearning platforms differed by age. According to Altawallbeh et al. (2015), there are age-related differences in behavior intention to utilize eLearning platforms, and it is obvious that age has a significant influence on the drivers of behavior intention. However, there are differences in behavior intention to use eLearning platforms based on years of experience in the use of technology. According to Jevsikova et al. (2021), there is a strong significant on years of technology experience for teachers, in which teachers with 15 years or more accept using eLearning platforms. Consequently, professional development for inclusive teachers is an important component of deploying digital platforms and providing high-quality education (Dahri et al., 2021).
This preliminary investigation’s findings may not be able to give all the answers to the given research questions. As a result, it is necessary to examine the development and modification of more extensive research pioneer models from which the UTAUT2 model is derived. This report seeks to summarise the findings of a few current studies on the factors that influence inclusive teachers’ use of e-learning platforms in Oman. An empirical study was done to test the model. This research makes a multidimensional contribution that includes both theoretical and practical contributions, as seen below.
The study’s first contribution is the development of a multi-dimensional, comprehensive model for assessing the adoption of e-learning platforms. The model was developed based on an intensive review of literature and analysis of the USTAU2 approach for assessing the acceptance of inclusive instructors’ eLearning technologies and platforms. The UTAUT2 model, which synthesises eight current contemporary models of technology acceptance, is chosen as one of the most influential and up-to-date instruments.
Besides that, it has been thoroughly evaluated and used in a variety of disciplines, including educational settings. This study appears to have confirmed the UTAUT2 model’s applicability in an inclusive classroom in Oman. With an extra construct “educational system quality (ESQ)”, and “service quality (SQ)”, UTAUT2 was amended, extended, or even modified to predict and interpret inclusive instructors’ BI to use eLearning platforms. The researchers hope that this new extended and validated extended UTAUT2 model will provide inclusive teachers with a better conceptual framework to assess whether their BI to use eLearning platforms was successful in Omani inclusive classrooms, which is the research’s second contribution (Ain et al., 2016; Azizi et al., 2020; Chipeva et al., 2018; Raman et al., 2022).
Furthermore, leveraging platforms to manage online classes and build customised digital resources and activities to engage both SEN and regular students in online classrooms is a complicated task for teachers. The third contribution of this study is that it presents important theoretical contributions in the inclusive education field and eLearning that practitioners, researchers, and policymakers can use to move forward in developing online learning communities that allow all students to participate in educational settings and beyond.
This is because, on the one hand, most Omani schools began using LMS systems (e.g., Manzara, Google Classroom), and on the other hand, they spend most of their investments in the delivery and use of these platforms to facilitate and support the teaching process. Study findings highlight some vital issues and recommendations that should be considered to improve insights on the acceptance of these platforms. The following are some of the study’s practical contributions to practitioners:
With respect to the fact that many schools in Oman have begun to employ LMS as an emergency option during pandemics, the study’s findings highlight the importance of assessing inclusive teachers on a regular basis. As a result, any flaws or shortcomings must be addressed through ongoing enhancement of these systems.
According to the research findings, inclusive teachers are unmotivated to adopt eLearning platforms. Based on this finding, we can conclude that the educational system and developers must focus on continual motivation to assist inclusive teachers in successfully using the eLearning platform. This can be accomplished by further gamifying the system, making inclusive online activities more relevant. In this regard, it should provide space for inclusive instructors to create instructional practices that fully include students with SEN in conversations and activities, as well as encourage them to collaborate with their peers (Liu et al., 2023).
The study finds that educational system quality (e.g., educational aspects in an eLearning system, as well as amenities such as communication tools, chat rooms, interaction, collaborative learning tools, diversity of learning styles, and providing students with assessment resources) has no significant impact on the BI of inclusive teachers, owing to a lack of inclusive teacher preparation in inclusive classrooms to use eLearning platforms. As a result, education policymakers at the ministry level should plan to develop ongoing and sufficient new relevant training programs for inclusive teachers to offer online courses efficiently, such as internet communication skills, to enhance the Omani education system’s quality.
The findings of this study highlight the importance of educational institutions putting forth significant effort to provide inclusive teachers and other institutions in recognising that system characteristics such as ease of use, system reliability, personalization, and integration between system components should be improved to make the system more reliable, user-friendly, personalised, attractive, intuitive, and easy to navigate. As a result, those participating in eLearning in general and LMS will be better able to understand how the system’s use may be expanded, and their willingness to use an eLearning platform will increase.
To sum it up, the primary purpose of this study is to provide a rationale for why some inclusive teachers accept the use of an eLearning platform as a new technology tool, while others stop or leave it. The study’s most significant contribution was the validation of a model that includes a personality trait as a precursor of inclusive teachers’ ideas about technology and platforms in the online classroom. After being validated on a sample of 128 high school inclusive schoolteachers, the model was found to explain a significant amount of variance in the intention and use of technology in the classroom (Mann, 2003).
Although the findings of this study are useful, there are certain limitations to be aware of. To begin with, the survey only included inclusive teachers from Oman schools as respondents. Therefore, we advocate that future studies include a wider variety of diverse groups of eLearning stakeholders to enrich the research with multiple points of view and provide a better understanding of the challenges of acceptability of eLearning platforms for success teaching (e.g., school leadership, SEN teachers, educational designers, and school administration) in a school setting. Future research should also investigate the impact of other aspects such as eLearning platform attitude, leadership support, and self-efficacy on behavioral intention to utilise eLearning platforms.
Furthermore, the effect of moderating variables such as age, gender, and years of technology experience on participants’ acceptance of eLearning platforms for teaching purposes was not considered in this study, in part because the participants in our study were not of the same age and had different years of technology experience. As a result, we recommend that future studies investigate these important factors as moderating variables in the context of UTAUT2 to identify if there is a major effect of age, gender, and years of technology experience on inclusive instructors’ acceptance of eLearning platforms. Finally, the behavioral intention to use an eLearning platform was assessed using a self-reported scale. The accuracy of the results could have been harmed by this manner of data collection. Qualitative research should be employed in future studies to investigate inclusive teachers’ opinions of eLearning platforms, as shown in this study, as it may yield further intriguing results.
The raw data supporting the conclusions of this article will be made available by the authors, without undue reservation.
Ethical review and approval was not required for the study on human participants in accordance with the local legislation and institutional requirements. Written informed consent from the participants was not required to participate in this study in accordance with the national legislation and the institutional requirements.
SA: Writing – review & editing, Methodology, Investigation. AA: Writing – review & editing, Data curation. NM: Writing – review & editing, Methodology, Investigation, Formal analysis. WN: Writing – review & editing, Supervision, Resources, Methodology, Formal analysis. MS: Writing – review & editing, Visualization, Validation, Project administration, Investigation, Data curation. RN: Writing – original draft, Validation, Conceptualization.
The author(s) declare that no financial support was received for the research, authorship, and/or publication of this article.
The authors declare that the research was conducted in the absence of any commercial or financial relationships that could be construed as a potential conflict of interest.
All claims expressed in this article are solely those of the authors and do not necessarily represent those of their affiliated organizations, or those of the publisher, the editors and the reviewers. Any product that may be evaluated in this article, or claim that may be made by its manufacturer, is not guaranteed or endorsed by the publisher.
Abusobaih, A., Havranek, M., and Abdulgabber, M. A.. (2021). “Unified theory of acceptance and use of technology (UTAUT) LEGO sets in education.” Pp. 708–713 In 2021 international conference on information technology (ICIT). IEEE.
Ain, N. U., Kaur, K., and Waheed, M. (2016). The influence of learning value on learning management system use. Inf. Dev. 32, 1306–1321. doi: 10.1177/0266666915597546
Alalwan, A. A., Dwivedi, Y. K., and Rana, N. P. (2017). Factors influencing adoption of Mobile banking by Jordanian Bank customers: extending UTAUT2 with trust. Int. J. Inf. Manag. 37, 99–110. doi: 10.1016/j.ijinfomgt.2017.01.002
Alam, G. M. (2021). Does online technology provide sustainable HE or aggravate diploma disease? Evidence from Bangladesh—a comparison of conditions before and during COVID-19. Technol. Soc. 66:101677. doi: 10.1016/j.techsoc.2021.101677
Alavi, M., Visentin, D. C., Thapa, D. K., Hunt, G. E., Watson, R., and Cleary, M. (2020). Chi-square for model fit in confirmatory factor analysis. J. Adv. Nurs. 76, 2209–2211. doi: 10.1111/jan.14399
Aldossry, B. (2021). Evaluating the Madrasati platform for the virtual classroom in Saudi Arabian education during the time of Covid-19 pandemic. Europ. J. Open Educ. E-Learn. Stud. 6. doi: 10.46827/EJOE.v6i1.3620
Alduaij, M. Y., Alterkait, M. A., and Alainati, S. (2024). Using the Delone and McLean success model to evaluate Moodle’s information system success. Eng. Technol. Appl. Sci. Res. 14, 15008–15015. doi: 10.48084/etasr.7300
Al-Fraihat, D., Joy, M., Masa'deh, R., and Sinclair, J. (2020). Evaluating E-learning systems success: an empirical study. Comput. Hum. Behav. 102, 67–86. doi: 10.1016/j.chb.2019.08.004
Alnahdi, G. H., and Schwab, S. (2021). Inclusive education in Saudi Arabia and Germany: students’ perception of school well-being, social inclusion, and academic self-concept. Eur. J. Spec. Needs Educ. 36, 773–786. doi: 10.1080/08856257.2020.1823163
Altawallbeh, M., Thiam, W., Alshourah, S., and Fong, S. F. (2015). The role of age and gender in the relationship between (attitude, subjective norm and perceived Behavioural control) and adoption of E-learning at Jordanian universities. J. Educ. Pract. 6, 44–54.
Azizi, S. M., Roozbahani, N., and Khatony, A. (2020). Factors affecting the acceptance of blended learning in medical education: application of UTAUT2 model. BMC Med. Educ. 20:367. doi: 10.1186/s12909-020-02302-2
Bentler, P. M. (1990). Comparative fit indexes in structural models. Psychol. Bull. 107, 238–246. doi: 10.1037/0033-2909.107.2.238
Burić, I., Parmač Kovačić, M., and Huić, A. (2021). Transformational leadership and instructional quality during the COVID-19 pandemic: a moderated mediation Analysis. Drustvena Istrazivanja 30, 181–202. doi: 10.5559/di.30.2.01
Cacciamani, S., Villani, D., Bonanomi, A., Carissoli, C., Olivari, M. G., Morganti, L., et al. (2018). Factors affecting students’ acceptance of tablet PCs: a study in Italian high schools. J. Res. Technol. Educ. 50, 120–133. doi: 10.1080/15391523.2017.1409672
Camilleri, M. A., and Camilleri, A. C. (2022). Remote learning via video conferencing technologies: implications for research and practice. Technol. Soc. 68:101881. doi: 10.1016/j.techsoc.2022.101881
Carrión, C. G., Henseler, J., Ringle, C., and Roldán, J. L. (2016). Prediction-oriented modeling in business research by means of PLS path modeling: Introduction to a JBR special section. J. Buss. Res. 69, 4545–4551. doi: 10.1016/j.jbusres.2016.03.048
Chen, H.-J. (2020). Linking role definition rigidity to elementary school teachers’ e-service for in-service teacher development. Comput. Hum. Behav. 107:105990. doi: 10.1016/j.chb.2019.04.014
Chipeva, P., Cruz-Jesus, F., Oliveira, T., and Irani, Z. (2018). Digital divide at individual level: evidence for eastern and Western European countries. Gov. Inf. Q. 35, 460–479. doi: 10.1016/j.giq.2018.06.003
Chua, K. H., and Bong, W. K. (2024). Providing inclusive education through virtual classrooms: a study of the experiences of secondary science teachers in Malaysia during the pandemic. Int. J. Incl. Educ. 28, 1886–1903. doi: 10.1080/13603116.2022.2042403
Coman, C., Țîru, L. G., Meseșan-Schmitz, L., Stanciu, C., and Bularca, M. C. (2020). Online teaching and learning in higher education during the coronavirus pandemic: students’ perspective. Sustain. For. 12:10367. doi: 10.3390/su122410367
Dahri, N. A., Vighio, M. S., Bather, J. D., and Arain, A. A. (2021). Factors influencing the acceptance of Mobile collaborative learning for the continuous professional development of teachers. Sustain. For. 13:13222. doi: 10.3390/su132313222
Davis, F. D. (1989). Perceived usefulness, perceived ease of use, and user acceptance of information technology. MIS Q. 13:319. doi: 10.2307/249008
DeLone, W. H., and McLean, E. R. (2003). The DeLone and McLean model of information systems success: a ten-year update. J. Manag. Inf. Syst. 19, 9–30. doi: 10.1080/07421222.2003.11045748
DeLone, W. H., and McLean, E. R. (2016). Information systems success measurement. Foundations and Trends® in Information Systems. 2, 1–116.
Dindar, M., Suorsa, A., Hermes, J., Karppinen, P., and Näykki, P. (2021). Comparing technology acceptance of K-12 teachers with and without prior experience of learning management systems: a Covid-19 pandemic study. J. Comput. Assist. Learn. 37, 1553–1565. doi: 10.1111/jcal.12552
Fan, Y., Chen, J., Shirkey, G., John, R., Wu, S. R., Park, H., et al. (2016). Applications of structural equation modeling (SEM) in ecological studies: an updated review. Ecol. Process. 5:19. doi: 10.1186/s13717-016-0063-3
Faragher, R. M. (2023). Individual student characteristics, abilities and personal qualities and the Teacher’s role in improving mathematics learning outcomes. Evol. Res. Teach. Math. 22, 227–253. doi: 10.1007/978-3-031-31193-2_8
Gunasinghe, A., Hamid, J. A., Khatibi, A., and Azam, S. M. F. (2019). The adequacy of UTAUT-3 in interpreting Academician’s adoption to e-learning in higher education environments. Interact. Technol. Smart Educ. 17, 86–106. doi: 10.1108/ITSE-05-2019-0020
Halder, S. (2023). “Preparing teacher educators for inclusive classroom” in The routledge handbook of inclusive education for teacher educators: Issues, considerations, and strategies. eds. S. Halder, S. Dada, and R. Banerjee, et al. Taylor & Francis. 3–24.
Harlow, L. L., Burkholder, G. J., and Morrow, J. A. (2002). Evaluating attitudes, skill, and performance in a learning-enhanced quantitative methods course: a structural modeling approach. structural equation modeling: a multidisciplinary. Jour. 9, 413–430. doi: 10.1207/s15328007sem0903_6
Hassanzadeh, A., Kanaani, F., and Elahi, S. (2012). A model for measuring E-learning systems success in universities. Expert Syst. Appl. 39, 10959–10966. doi: 10.1016/j.eswa.2012.03.028
Holzmann, P., Schwarz, E. J., and Audretsch, D. B. (2020). Understanding the determinants of novel technology adoption among teachers: the case of 3D printing. J. Technol. Transf. 45, 259–275. doi: 10.1007/s10961-018-9693-1
Hsu, L. (2016). Diffusion of innovation and use of Technology in Hospitality Education: an empirical assessment with multilevel analyses of learning effectiveness. Asia Pac. Educ. Res. 25, 135–145. doi: 10.1007/s40299-015-0244-3
Jevsikova, T., Stupurienė, G., Stumbrienė, D., Juškevičienė, A., and Dagienė, V. (2021). Acceptance of distance learning technologies by teachers: determining factors and emergency state influence. Informatica 32, 517–542. doi: 10.15388/21-INFOR459
Kang, M., Liew, B. Y. T., Lim, H., Jang, J., and Lee, S. (2015). Investigating the determinants of mobile learning acceptance in Korea using UTAUT2. In Emerging issues in smart learning. Springer Berlin Heidelberg. 209–216.
Kim, H.-W., Chan, H. C., and Gupta, S. (2007). Value-based adoption of Mobile internet: an empirical investigation. Decis. Support. Syst. 43, 111–126. doi: 10.1016/j.dss.2005.05.009
Kim, K., Trimi, S., Park, H., and Rhee, S. (2012). The impact of CMS quality on the outcomes of E-learning Systems in Higher Education: an empirical study. Decis. Sci. J. Innov. Educ. 10, 575–587. doi: 10.1111/j.1540-4609.2012.00360.x
Kozleski, E. B. (2020). Disrupting what passes as inclusive education: predicating educational equity on schools designed for all. Educ. Forum 84, 340–355. doi: 10.1080/00131725.2020.1801047
Ling, L. W., Ahmad, W. F. W., and Singh, T. K. R.. (2016). “Factors influencing behavioral intention to use computers among teachers.” Pp. 120–125 In 2016 3rd international conference on computer and information sciences (ICCOINS). IEEE.
Liu, T., Oubibi, M., Zhou, Y., and Fute, A. (2023). Research on online teachers’ training based on the gamification design: a survey analysis of primary and secondary school teachers. Heliyon 9, e15053–e15018. doi: 10.1016/j.heliyon.2023.e15053
Lopez-Perez, V. A., Ramirez-Correa, P. E., and Grandon, E. E. (2019). Innovativeness and factors that affect the information technology adoption in the classroom by primary teachers in Chile. Inform. Educ. 18, 165–185. doi: 10.15388/infedu.2019.08
Makoelle, T. M. (2016). “Who is an inclusive teacher?” in Inclusive teaching in South Africa. ed. T. M. Makoelle African Sun Media, 57–66.
Mann, S. J. (2003). E-learning in the 21st century-a framework for research and practice. Innov. Educ. Teach. Int. 40:313.
Marcinkovic, B., Abersek, B., and Pesek, I.. (2021). “The satisfaction of primary school teachers with the introduction and use of MS teams in distance education.” Pp. 694–698 In 2021 44th international convention on information, communication and electronic technology (MIPRO). IEEE.
Mathew, S., Jose, A., G, R., and Chacko, D. P. (2020). Examining the relationship between E-service recovery quality and e-service recovery satisfaction moderated by perceived justice in the banking context. BIJ 27, 1951–1980. doi: 10.1108/BIJ-07-2019-0323
Ministry of Education in Oman. (2022). Oman’s Ministry of Education organises special exhibition for people with disabilities. Times of Oman. Retrieved September 16, 2024, Available at: https://timesofoman.com/article/115182-omans-ministry-of-education-organises-special-exhibition-for-people-with-disabilities
Ministry of Education in Oman. (2024). New educational platform to be launched in partnership with Ooredoo to enhance digital learning. Times of Oman. Accessed September 16, 2024, Available at: https://timesofoman.com/article/3016290/oman/education/new-educational-platform-to-be-launched-in-oman
Mohd, F., Yahya, W. F. F., Jalil, M. A., Ismail, S., Noor, N. M. M., and Hasan, M. N. (2019). User acceptance testing (UAT) for the development and evaluation of an automated learning style detection system. In AIP Conference Proceedings. AIP Publishing. 2138.
Mokaleng, M., and Möwes, A. D. (2020). Issues affecting the implementation of inclusive education practices in selected secondary schools in the Omaheke region of Namibia. J. Curric. Teach. 9:78. doi: 10.5430/jct.v9n2p78
Mtebe, J. S., Mbwilo, B., and Kissaka, M. M. (2016). Factors influencing teachers’ use of multimedia enhanced content in secondary schools in Tanzania. Int. Rev. Res. Open Distrib. Learn. 17:2280. doi: 10.19173/irrodl.v17i2.2280
Ouajdouni, A., Chafik, K., and Boubker, O. (2021). Measuring E-learning systems success: data from students of higher education institutions in Morocco. Data Brief 35:106807. doi: 10.1016/j.dib.2021.106807
Ozkan, S., and Koseler, R. (2009). Multi-dimensional students’ evaluation of e-learning Systems in the Higher Education Context: an empirical investigation. Comput. Educ. 53, 1285–1296. doi: 10.1016/j.compedu.2009.06.011
Pizarro-Uy, A. C. D., and Manapat, C. L. (2023). Evaluating the culture of online learning and its effectiveness amidst the Covid-19 pandemic. Rev. Integ. Bus. Econ. Res. 12, 136–147.
Prasetyo, Y. T., Ong, A. K. S., Concepcion, G. K. F., Navata, F. M. B., Robles, R. A. V., Tomagos, I. J. T., et al. (2021). Determining factors affecting acceptance of E-learning platforms during the COVID-19 pandemic: integrating extended technology acceptance model and DeLone & McLean IS success model. Sustain. For. 13:8365. doi: 10.3390/su13158365
Raman, A., and Thannimalai, R. (2021). Factors impacting the Behavioural intention to use E- learning at higher education amid the Covid-19 pandemic: UTAUT2 model. Psychol. Sci. Educ. 26, 82–93. doi: 10.17759/pse.2021260305
Raman, A., Thannimalai, R., Rathakrishnan, M., and Ismail, S. N. (2022). Investigating the influence of intrinsic motivation on behavioral intention and actual use of Technology in Moodle Platforms. Int. J. Instr. 15, 1003–1024. doi: 10.29333/iji.2022.15157a
Reychav, I., Ndicu, M., and Wu, D. (2016). Leveraging social networks in the adoption of mobile technologies for collaboration. Comp. Human Behav. 58, 443–453. doi: 10.1016/j.chb.2016.01.011
Rice, M. F. (2017). Describing K-12 online teachers’ online professional development opportunities for students with disabilities. Online Learn. 21, 103–121. doi: 10.24059/olj.v21i4.1274
Rosetta, A., Priska, M. A., Muslim, E., and Rafi, M.. (2020). “Evaluating the success of E-learning systems and strategy creation: the perspective of students in Universitas Indonesia.” Pp. 11–17 In 2020 the 4th international conference on education and E-learning. New York, NY, USA: ACM.
Saal, P. E., Graham, M. A., and van Ryneveld, L. (2020). Integrating educational Technology in Mathematics Education in economically disadvantaged areas in South Africa. Comput. Sch. 37, 253–268. doi: 10.1080/07380569.2020.1830254
Scanlon, G., Radeva, S., Pitsia, V., Maguire, C., and Nikolaeva, S. (2022). Attitudes of teachers in Bulgarian kindergartens towards inclusive education. Teach. Teach. Educ. 112:103650. doi: 10.1016/j.tate.2022.103650
Schuhen, M., and Schürkmann, S. (2016). “Construct validity with structural equation modelling” in International handbook of financial literacy. eds. C. Aprea, E. Wuttke, K. Breuer, K. K. Noi, P. Davies, and B. Greimel-Fuhrmann, et al. (Singapore: Springer Singapore), 383–396.
Šumak, B., and Šorgo, A. (2016). The acceptance and use of interactive whiteboards among teachers: differences in UTAUT determinants between pre- and post-adopters. Comput. Hum. Behav. 64, 602–620. doi: 10.1016/j.chb.2016.07.037
Tamilmani, K., Rana, N. P., Wamba, S. F., and Dwivedi, R. (2021). The extended unified theory of acceptance and use of technology (UTAUT2): a systematic literature review and theory evaluation. Int. J. Inf. Manag. 57:102269. doi: 10.1016/j.ijinfomgt.2020.102269
Tarhini, A., Hone, K., and Liu, X. (2014). Measuring the moderating effect of gender and age on E-learning acceptance in England: a structural equation modeling approach for an extended technology acceptance model. J. Educ. Comput. Res. 51, 163–184. doi: 10.2190/EC.51.2.b
Thomas, M., and Bryson, J. R. (2021). Combining proximate with online learning in real-time: ambidextrous teaching and pathways towards inclusion during COVID-19 restrictions and beyond. J. Geogr. High. Educ. 45, 446–464. doi: 10.1080/03098265.2021.1900085
Turki, A. M., and Sathiyanarayanan, M.. (2018). “Importance of Mobile learning in improving the quality of educational-information Systems in the Saudi Arabian High Schools.” Pp. 1–5 In 2018 21st Saudi computer society National Computer Conference (NCC). IEEE.
Van der Heijden, H. (2004). User acceptance of hedonic information systems. MIS Q. 28:695. doi: 10.2307/25148660
Veiga, F. J., and Andrade, A. M. V. d. (2021). Critical success factors in accepting Technology in the Classroom. Int. J. Emerg. Technol. Learn. (IJET) 16:4. doi: 10.3991/ijet.v16i18.23159
Venkatesh, V., Morris, M. G., Davis, G. B., and Davis, F. D. (2003). User acceptance of information technology: toward a unified view. MIS Q. 27:425. doi: 10.2307/30036540
Venkatesh, V., Thong, J. Y., and Xu, X. (2012). Consumer acceptance and use of information technology: extending the unified theory of acceptance and use of technology. MIS Q. 36:157. doi: 10.2307/41410412
Warnecke, E., and Pearson, S. (2011). Medical students’ perceptions of using e-learning to enhance the acquisition of consulting skills. Australasian Med. J. 4, 300–307. doi: 10.4066/AMJ.2011.73
Wrzosek, N., Zimmermann, A., and Balwicki, Ł. (2020). Doctors’ perceptions of E-prescribing upon its mandatory adoption in Poland, using the unified theory of acceptance and use of technology method. Healthcare 8:563. doi: 10.3390/healthcare8040563
Wu, Yu-Lung, Tao, Yu-Hui, and Yang, Pei-Chi. (2007). “Using UTAUT to explore the behavior of 3G Mobile communication users.” Pp. 199–203 In 2007 IEEE international conference on industrial engineering and engineering management. IEEE.
Xiong, B., Skitmore, M., and Xia, B. (2015). A critical review of structural equation modeling applications in construction research. Autom. Constr. 49, 59–70. doi: 10.1016/j.autcon.2014.09.006
Zalah, I., Greener, S., and Gill, D. (2017). Factors that influence SAUDI secondary teachers’ acceptance of e-learning technologies. In Edulearn17 Proceedings. IATED. 5749–5759.
Keywords: eLearning platform, UTAUT2 model, inclusive education, schoolteachers, class room activity
Citation: Almaki SH, Al Mazrouei AK, Mafarja N, Naseem W, Sial MA and Naveed RT (2024) Factors influencing inclusive teachers’ acceptance to adopt eLearning platforms in classroom: a case study in Oman. Front. Educ. 9:1477659. doi: 10.3389/feduc.2024.1477659
Received: 08 August 2024; Accepted: 26 September 2024;
Published: 17 October 2024.
Edited by:
Sarfraz Aslam, UNITAR International University, MalaysiaReviewed by:
Namood-e Sahar, Quaid-i-Azam University, PakistanCopyright © 2024 Almaki, Al Mazrouei, Mafarja, Naseem, Sial and Naveed. This is an open-access article distributed under the terms of the Creative Commons Attribution License (CC BY). The use, distribution or reproduction in other forums is permitted, provided the original author(s) and the copyright owner(s) are credited and that the original publication in this journal is cited, in accordance with accepted academic practice. No use, distribution or reproduction is permitted which does not comply with these terms.
*Correspondence: Samah Hatem Almaki, ZHIuc2FtYWhAY2l0eS5lZHUubXk=
Disclaimer: All claims expressed in this article are solely those of the authors and do not necessarily represent those of their affiliated organizations, or those of the publisher, the editors and the reviewers. Any product that may be evaluated in this article or claim that may be made by its manufacturer is not guaranteed or endorsed by the publisher.
Research integrity at Frontiers
Learn more about the work of our research integrity team to safeguard the quality of each article we publish.