- 1University of Baghdad-College of Islamic Sciences, Baghdad, Iraq
- 2Department of Psychology, Faculty of Education, King Khalid University, Abha, Saudi Arabia
- 3Department of Psychology, College of Arts, Zagazig University, Zagazig, Egypt
The utilization of artificial intelligence techniques has garnered significant interest in recent research due to their pivotal role in enhancing the quality of educational offerings. This study investigated the impact of employing artificial intelligence techniques on improving the quality of educational services, as perceived by students enrolled in the College of Pharmacy at the University of Baghdad. The study sample comprised 379 male and female students. A descriptive-analytical approach was used, with a questionnaire as the primary tool for data collection. The findings indicated that the application of artificial intelligence methods was highly effective, and the educational services provided to students were of exceptional quality. The results also showed a strong correlation (correlation coefficient of 0.719) between the use of artificial intelligence techniques and the quality of educational services. This correlation was statistically significant at a confidence level of 99%. The impact of artificial intelligence techniques and their dimensions on the quality of educational services is highly significant at a confidence level of 99%. This suggests that artificial intelligence technologies play a major role in enhancing the quality of educational services. The study emphasizes the importance of creating technologically advanced classrooms equipped with modern devices and equipment to enhance the learning experience and provide an advanced educational environment. It also highlights the significance of effectively addressing students’ complaints and grievances through technical means, such as electronic communication platforms, social media platforms, technical support via the Internet, and smartphone applications. These measures are essential in providing high-quality educational services.
1 Introduction and theoretical background
Given the swift progress in technology, Artificial Intelligence has become indispensable in our everyday lives, especially in higher education. These technologies are employed in different educational domains, including teaching, learning, student assessment, lesson management, and data analysis (McGrath et al., 2023). These technologies strive to improve the quality of educational services by establishing a more dynamic and efficient learning environment. They support teaching personnel in delivering customized instructional material, providing timely feedback, and overseeing student progress (Kohnke et al., 2023). Universities nowadays are broadening their mission beyond the conventional tasks of maintaining heritage, identity, and education, as well as supporting the academic community. They must now stay abreast of technological changes by implementing creative educational and instructional approaches. From this standpoint, everyone must remain informed about technological breakthroughs on a global scale (Kong et al., 2021). The swift progress of information and communication technology in education has necessitated a transition from conventional in-person learning to contemporary e-learning or distance learning, which is readily accessible. This enhances convenience for teachers and students to efficiently and sustainably access global knowledge (Stachowicz-Stanusch and Amann, 2018). Artificial intelligence applications in education vary from automated assessment systems that analyze student performance to personalized learning systems that allow customization of curricula according to the student’s level. Big data analysis tools are also used to extract valuable insights from educational data, helping to make informed decisions that contribute to improving the educational process in general (Luckin and Holmes, 2016). There are also intelligent interactive systems that provide personalized educational support to students. These systems are capable of delivering educational content and individual guidance based on the student’s level and academic progress. They rely on a range of technologies such as natural language processing and machine learning to analyze student responses and provide appropriate instructions (Ussell and Norvig, 2016). Thus, artificial intelligence technologies contribute to enhancing the quality of education by providing personalized learning for each student, effectively analyzing data to improve teaching strategies, and saving time and effort for teachers. These improvements can lead to better academic outcomes and reduce educational gaps between students from different backgrounds (Noor et al., 2022).
1.1 The concept of artificial intelligence
The origins of Artificial Intelligence can be traced back to the 1950s when John McCarthy organized a two-month workshop at Dartmouth College in the United States of America. McCarthy first suggested the phrase “AI” in 1956 in his workshop proposal (Russel and Norvig, 2010). AI is a term that combines the concepts of intelligence and artificiality. Intelligence is the capacity to think, generate novel ideas, perceive, and acquire knowledge, whereas AI is what is produced by humans. AI is a discipline within computer science that focuses on the development and use of intelligent computers capable of performing tasks and exhibiting behaviors similar to those of humans (Verma, 2018). AI refers to computer systems that can imitate human activities such as learning, adapting, studying, self-correcting, and using data to solve complex issues (Popenici and Kerr, 2017). Baker and Smith (2019) offer a comprehensive definition of AI as the utilization of computers to carry out various activities. Comprehensive cognitive knowledge is commonly associated with human minds, especially in the context of learning and solving complex problems (Baker and Smith, 2019). On the other hand, the Organization for Economic Cooperation and Development defines AI as “a machine-based system that enables it to accomplish a specific set of goals determined by humans and to provide predictions, recommendations, or decisions.” It affects real or digital settings (Stolpe and Hallström, 2024). Essentially, it is an AI developed by humans and exhibited by machines, therefore being perceived as a human-made instrument that imitates the cognitive abilities of the human brain (Tai, 2020). Human intelligence encompasses various components, including neural, cognitive, social, emotional, and situational aspects. On the other hand, AI exhibits different levels of complexity and operation. It can be categorized into three levels, namely Artificial Narrow Intelligence (ANI) or Weak Intelligence (Kaplan and Haenlein, 2019). AI, also known as AI, pertains to the utilization of advanced technology for performing certain tasks such as facial and voice recognition or autonomous driving. Artificial General Intelligence (AGI) refers to a more advanced type of intelligence that is capable of autonomous reasoning and problem-solving abilities that go beyond its initial programming. Artificial Superintelligence (ASI) refers to the advanced form of AI that surpasses human capabilities and excels in multiple domains (Benbya et al., 2020).
Table 1 presents a comprehensive overview of applications of artificial intelligence techniques in educational services.
1.2 The concept of quality educational service
Ensuring the quality of educational services is a crucial aspect of the realm of education. Ensuring the excellence of education is vital for the viability of academic institutions. Therefore, quality is acknowledged as a strategic instrument for attaining operational efficiency and enhancing performance among stakeholders. Delivering services of superior quality is extremely challenging and requires significant effort (Syam and Arifin, 2019). In the face of growing competition in the education sector, the provision of high-quality services to beneficiaries has become essential for the ongoing operation and success of educational institutions. High-quality service is a significant draw for students, as it directly impacts their satisfaction. The quality of educational service refers to its ability to meet the needs of students and adhere to established quality standards (Farhan et al., 2022). The quality of educational service is crucial as it serves as a method of communication within and beyond the educational institution, ensuring the provision of vital services to students and meeting their demands. Additionally, it facilitates the development of AI on curricula by implementing a complete quality control system in educational institutions (Haming et al., 2019). The quality of services is a multifaceted term that can be quantified to assess the quality of service offered by a company, educational institution, or individual. The dimensions of the service provided can vary based on its type, but there are five commonly used dimensions in several fields (Yılmaz and Temizkan, 2022). Reliability refers to the ability of an educational institution to deliver the promised service to students, who are the customers of the educational service. Responsiveness, in this context, refers to the educational institution’s commitment to assisting students, providing timely services, and promptly responding to student demands. Tangibility is a factor of educational service quality that relates to the degree to which the service can be objectively measured and explained (Alijanzadeh et al., 2018). Trust in an educational institution’s staff is based on their expertise, respect, and capacity to instil confidence in students through their competence (Zun et al., 2018). Empathy, in the context of educational institutions, pertains to the degree to which these institutions demonstrate concern for the needs and emotions of students. Empathy in educational service encompasses a broad spectrum of characteristics: (a) a comprehensive understanding of the requirements of students, and (b) genuine empathy with students’ emotions. The empathic response to students’ needs is a crucial aspect (Ganbold et al., 2022).
1.3 Discussion of some previous studies
Although many studies have addressed the impact of Artificial Intelligence techniques on the quality of education, this study highlights the unique context of the University of Baghdad, specifically the College of Pharmacy, where economic and technical challenges are faced. This research demonstrates how AI can improve the quality of educational services even in resource-limited environments, representing a new contribution to the existing literature and showcasing the potential of modern technologies to enhance academic performance in developing countries.
A study titled “The Role of AI Techniques in Improving the Behavior and Practices of Faculty Members When Switching to eLearning in Light of the COVID-19 Crisis” showed that the researcher had a penchant for providing detailed descriptions and conducting thorough analyses. This inquiry evaluated the impact of artificial intelligence (AI) and electronic learning (e-learning) on four educational topics in Saudi Arabia. The findings from the survey of 406 participants demonstrated a significant influence of artificial intelligence on electronic learning (α ≤ 0.05). The consensus among faculty members was that AI and e-learning significantly improved teaching objectives and methodologies during the pandemic. Moreover, the pandemic had a beneficial effect on teaching methodologies. The instructors unanimously concurred that the utilization of e-learning and artificial intelligence significantly enhanced their instructional methodologies as a whole. The study discovered that the utilization of e-learning and artificial intelligence (AI) assists teachers in automating the grading process, ensuring the confidentiality of data, and enhancing the accessibility of information. The study revealed a positive correlation between the average of sample responses in all four fields and acceptance levels. This discovery is of utmost importance considering the current COVID-19 pandemic and the profound impact of e-learning and AI in the field of education (Abdullah, 2021). Another study titled “Impact of AI on Education” explores how artificial intelligence is changing the face of traditional education, highlighting the benefits and risks of its various applications in educational environments. The researcher addresses the use of intelligent tools and technologies in education, such as personalized learning, student assessment, and educational data analysis, to improve the educational process. The study focuses on the impact of artificial intelligence on education from several angles. The author discusses how educational systems adapt to technological innovations and how AI can improve the quality of education and facilitate access to it. The research also highlights potential challenges such as privacy issues and the ethics of using AI, suggesting strategies to overcome them. The study relies on the analysis of previous literature and practical examples to present the impact of artificial intelligence on both teachers and learners and provides recommendations on how to effectively integrate this technology into educational systems (Mureșan, 2023). A study titled “Impact of AI on Assessment Methods in Primary and Secondary Education: Systematic Literature Review” confirmed that artificial intelligence (AI) has the potential to enhance education through various means. AI and its educational applications are an interdisciplinary field that integrates computer science, statistics, psychology, and education. The objective of this review was to consolidate research findings on the application of artificial intelligence (AI) to enhance the assessment of primary and secondary students. This systematic literature review included a total of nine original research publications, which involved a total of 641 participants. These papers were published between 2010 and 2023 and met the specific criteria set for this review. The primary contributions of AI in assessing students at lower levels include predicting their performance, automating evaluations to enhance objectivity through the use of neural networks or natural language processing, analyzing students’ learning processes using educational robots, and identifying factors that enhance the appeal of classes. This study demonstrates the potential and existing application of artificial intelligence (AI) in education, particularly in the assessment of primary and secondary student performance (Martínez-Comesaña et al., 2023). Another study titled “The Effects of AI Applications in Educational Settings: Challenges and Strategies” stated that there is a growing use of AI tools in education. Therefore, it is necessary to conduct a new study to evaluate their effectiveness in informing pedagogical practices. This study examines existing literature to identify the primary barriers to implementing AI models in the field of education. Researchers both advocate for and criticize the use of AI-based academic applications. Between 2018 and 2023, a total of 69 papers were chosen from a pool of 618 articles sourced from diverse academic journals. The report categorizes challenges based on user, operational, environmental, technological, and ethical aspects following a comprehensive evaluation of selected studies. This evaluation recommends utilizing ChatGPT as a supplementary tool for teaching and learning, which involves tailoring and optimizing it specifically for teachers. This study addresses the lack of information on how AI models enhance instruction. The paper examines the impact of artificial intelligence on learning, encompassing innovative recommendations, AI on training with diverse datasets and genres, human involvement, data confidentiality, and mitigating bias. The article concludes by presenting strategic answers to anticipated challenges and proposing tactics to enhance the adoption of ChatGPT and other AI technologies in the field of education. This evaluation can be utilized by policymakers, teachers, technology professionals, and stakeholders to facilitate the adoption of ChatGPT in the field of education. Furthermore, the evaluation establishes the foundation for subsequent investigations (Ali et al., 2023).
Another study titled “Ethics of Artificial Intelligence, Higher Education, and Scientific Research” The study highlights the ethical challenges associated with the use of artificial intelligence (AI) in education, pointing to key issues such as data privacy and algorithmic bias. These challenges combine concerns about the impact of AI on educational decisions and students’ academic outcomes. The findings suggest that the data used to train AI systems can reflect existing biases, leading to unfair decisions in student assessment. Additionally, the study emphasizes the importance of transparency in how data is collected and used, allowing students to have a say in how their personal information is used. It also calls for the need for a comprehensive ethical framework to guide the use of AI responsibly and safely, helping to avoid potential negative effects on individual rights and enhance the overall educational experience. These considerations call for more research to understand how to safely and effectively integrate AI technologies into educational environments, focusing on achieving benefits and enhancing the learning experience for students (Roumate, 2023).
2 Conceptual model and hypothesis development
The following sub-sections are devoted to developing specific hypotheses based on the proposed investigation. The assumed relationships among the studied constructs are graphically shown in Figure 1, representing the conceptual model we will test through our investigation.
2.1 The relationship between AI and quality of educational service
Various international reports indicate that AI in Higher Education (AIED) is currently gaining prominence as a significant field in educational technology, despite its existence for three decades. Nevertheless, universities, colleges, and institutes are still uncertain about how they might derive greater advantages from it and how it can truly influence the teaching and learning process in higher education (Zawacki-Richter et al., 2019). AI plays a vital role in higher education by improving numerous processes and functions, such as developing, leading, supporting, and implementing plans based on scientific research and data analysis, increasing profitability by eliminating unnecessary work, reducing expenses, improving productivity and efficiency, and promoting student loyalty while consistently improving university services (Mukherjee, 2022). It also enhances the efficiency of modifying educational activities to comply with regulatory standards by implementing transformation and revolutionary business re-engineering. Furthermore, AI facilitates informed decision-making by providing analyzed data along with recommended results, directing educational endeavours towards achievement (Habib et al., 2024). Multiple studies have demonstrated the crucial importance of AI in higher education, specifically for faculty members and university students. These technologies provide flexible educational solutions without limitations (Jain and Jain, 2019). Universities globally are experiencing an increase in student enrollment as a result of improved adaptability and effectiveness. Despite the comparatively high cost of implementing AI in colleges, it is considered cost-effective compared to the expenses associated with manual labor (Königstorfer and Thalmann, 2020). Utilizing AI in the long run among university students is more cost-effective compared to traditional educational approaches that involve manual tasks. Developed nations have effectively incorporated AI procedures, while poorer nations are still in the nascent phases. The main reasons for this are poor infrastructure, limited information availability, lack of support from educational institutions, inadequate funding, and insufficient technological capabilities. These factors present obstacles for developing countries aiming to integrate AI into higher education (ElHassan and Arabi, 2024). AI encompasses a wide range of underlying processes and strategies used to establish it. The reliance on technology is substantial, requiring significant investments in educational institution infrastructure to improve technological operations and prepare a specialized workforce (Lui and Lamb, 2018). Although AI has many benefits, it faces various obstacles in educational institutions. One of the main challenges is the potential obsolescence of certain skills due to automation driven by AI. This can occur when tasks are performed more efficiently and accurately by machines, leading to the automation of tasks that used to require human intervention. As a result, human jobs may be displaced (Rahiman and Kodikal, 2024). The use of AI technology can also result in significant expenses, especially for universities, colleges, and institutes that have restricted financial means (Mhlanga, 2020). Individuals, such as faculty members, students, and staff, who do not have access to contemporary personal devices such as computers, smartphones, tablets, internet connectivity, and ICT skills may struggle to successfully utilize AI technology. This highlights the significance of including them in training programs to improve their abilities and synchronize with advancements beneficial to educational institutions (Chen et al., 2020). Finally, numerous AI technologies depend on significant quantities of organized, semi-structured, and unstructured data, which may not always be easily accessible in educational environments (Park et al., 2023). These technologies facilitate students’ access to essential support and aid teachers in identifying deficiencies in the teaching process to improve their performance. Intelligent systems are essential in influencing the way teachers and students engage with information in everyday life and professional settings (Li and Wang, 2023). Based on the above, we derive the following:
H1. AI has a positive effect on the quality of educational services.
2.2 The relationship between AI on decision-making and quality of educational service
AI technologies are inherently connected to decision-making and the quality of educational services in multiple ways. Through the analysis of extensive data sets, AI can offer valuable information that assists decision-makers in optimizing their processes (Feng and Tang, 2021). Machine learning approaches can improve the quality of educational services by customizing learning experiences for each student according to their specific needs. This connection has the potential to enhance educational outcomes and boost student satisfaction (Roll and Wylie, 2016). Based on the above, we derive the following:
H2. AI in decision-making has a positive effect on the quality of educational service.
2.3 The relationship between AI on distance learning and quality of educational service
Through the analysis of extensive student data, AI can precisely determine the unique requirements of each student and assign educational materials and experiences that are specifically tailored to their level of knowledge and preferred learning styles (Booyse and Scheepers, 2023). AI enables the development of dynamic and integrated virtual learning environments that provide individualized and relevant instructional information. Students receive instant and significant feedback, which improves learning effectiveness and engagement. By employing machine learning methodologies, educational institutions may accurately measure student performance and forecast their future needs, thereby enhancing educational programs and tailoring them more effectively to meet student demands (Holmes et al., 2019). Based on the above, we derive the following:
H3. AI on Distance Learning has a positive effect on the quality of educational service.
2.4 The relationship between AI in training and quality of educational service
AI can offer guidance to students and provide them with suitable resources to assist in the development and composition of projects and research, tailored to their cognitive abilities and specific requirements (Kuhl et al., 2019). Machine learning and data analytics facilitate the production of thorough reports on students’ performance in project and research writing, simplifying the process for teachers and supervisors to offer the necessary recommendations for enhancing their performance (Chassignol et al., 2018). Based on the above, we derive the following:
H4. AI on Training has a positive effect on the quality of educational service.
3 Materials and methods
This study utilized the descriptive-analytical methodology. The study sample comprised 379 male and female students enrolled at the University of Baghdad’s College of Pharmacy. A hyperlink was generated using Google Forms and subsequently shared with the participants, following their verbal agreement to take part in this research the minimum sample size for the study was calculated using the Steven K. Thompson formula: n = (N*P (1-P))/[N-1*(d2/Z2) + P (1-P)].
Table 2 shows the demographic details of the study sample.
The researchers in this study developed a questionnaire comprising two sections. The first section evaluates artificial intelligence approaches and includes 16 statements divided into four dimensions: curriculum and teaching quality, decision-making, distance learning, and training. The questionnaire for the initial segment was prepared using a previous study (El-Masry, 2022). as a basis. The second section of the questionnaire assesses the calibre of educational services through 20 statements that gauge reliability, trustworthiness, tangibility, and empathy. The questionnaire for the second half was constructed using findings from earlier studies (Aboubakr and Bayoumy, 2022; Alayoubi et al., 2020).
This article conducted an assessment of validity and reliability using Cronbach’s alpha coefficient. The Cronbach’s alpha coefficient is a widely used measure of reliability in previous research. It assesses the internal consistency of responses to questionnaire items among sample members. The Cronbach’s alpha value ranges from 0 to 1, with higher values indicating greater internal consistency. There is agreement among researchers regarding the interpretation of this measure, with an accepted value of ≥0.60.
Furthermore, a normal distribution test is conducted. The normal distribution test assesses the distribution of data and is conducted through many statistical tests, with the Kolmogorov–Smirnov test and the Shapiro–Wilk test being the most significant ones. An examination of multicollinearity between the study variables is also conducted. Multicollinearity is a statistical phenomenon that occurs when there is a strong correlation between the independent variables in a regression model. The objective is to prevent a significant and positive relationship between the variables, which results in the issue of multicollinearity. In such cases, one of the variables needs to be removed during analysis because a high correlation suggests that they are measuring the same phenomenon, and the causal relationship between them is uncertain, as they are both influenced by the same factor. The Variance Inflation Factor (VIF) and Tolerance value are commonly used measures to assess multicollinearity. To determine the absence of multicollinearity, the Tolerance value should be greater than or equal to 0.10, and the inverse of the Variance Inflation Factor should be less than or equal to 10.
Moreover, exploratory factor analysis is conducted. A study was conducted using exploratory factor analysis, a statistical technique used to reduce the number of items. The researcher utilized the KMO test (Kaiser-Meyer-Olkin) to ensure that the study sample size was adequate for conducting the analysis. The KMO value needed to be equal to or greater than 0.50. Additionally, the researcher employed Bartlett’s Test of Sphericity to determine if the relationship between variables was statistically significant. For the relationship to be considered acceptable, the critical value needed to be less than 0.05.
Furthermore, the study variables are analyzed using descriptive statistical analysis. The results of the study variables and their dimensions are interpreted by calculating statistical measures such as mean, standard deviation, and coefficient of variation for the sample members’ answers. The primary and secondary hypotheses regarding the correlation and impact of the variables under research are examined. The aim is to test the hypothesis regarding the correlation between the variables under research.
Assessing the nature and significance of the association between the independent variable (artificial intelligence technology) and the dependent variable (quality of educational services) involves examining the correlation coefficient (r). There are two types of correlation: positive correlation, which occurs when two variables move in the same direction, and negative correlation, which signifies that the two variables move in opposite directions. This study aims to test the hypotheses on the impact of the independent variable, artificial intelligence technology, and its various dimensions on the dependent variable, which is the quality of educational services.
To assess the impact of the independent variable on the dependent variable, it is necessary to test both hypotheses. This can be done using statistical techniques such as regression analysis, which provides the regression coefficient (β) and the coefficient of determination (R2). The regression coefficient measures the strength of the effect, while the coefficient of determination indicates the percentage of the independent variable’s impact on the dependent variable. Additionally, the calculated value of the F-statistic can be compared to the tabular value to determine whether the hypothesis is accepted or rejected.
Furthermore, the study examined the influence of AI curricula on the quality of educational services and its various aspects. An investigation was conducted to examine the influence of AI decision-making on the quality of educational services and its many aspects. An analysis was performed on the influence of remote AI education on the quality and aspects of educational services, as well as the impact of training AI on the quality and aspects of educational services. Additionally, an examination was conducted on the influence of artificial intelligence technologies on the calibre of educational services and its many aspects.
The data was analyzed using the SPSS-24 program. Means and standard deviations, as well as Pearson’s correlation coefficient, were calculated. Based on a confidence interval (0.05).
4 Results
In this section, the validity and reliability of the Questionnaire validity and reliabilityExploratory factor analysis was conducted, which is used to reduce the number of items. The researcher relied on the KMO test (Kaiser Meyer Olkin) for the study sample to be sufficient to conduct the analysis, and its value must be greater than or equal to (0.50), and the (Bartlett’s Test. of Sphericity); for the relationship between variables to be statistically acceptable, the critical value must be less than (0.05).
The Table 3 clearly shows that the value of (KMO) for all dimensions of the independent variable (AI techniques) is higher than 0.50. On the other hand, the values of (Bartlett’s Test) for the dimensions of the independent variable were lower than the significant value of 0.05. The critical value represents the level of significance. Additionally, the proportionality of the study sample was achieved for all items with values higher than the standard limit of 0.50. As a result, exploratory factor analysis was successfully conducted for all values of the dimensions of the independent variable.
Table 4 indicates that the KMO (Kaiser-Meyer-Olkin) values for all dimensions of the dependent variable (quality of educational service) are greater than 0.50. On the other hand, the values of Bartlett’s Test for the dimensions of the dependent variable were less than the significant value of 0.05. The critical value represents the level of significance. Additionally, the study sample achieved proportionality for all items with values higher than the standard limit of 0.50. Consequently, exploratory factor analysis was successfully conducted for all dimensions of the dependent variable.
In addition, Cronbach’s alpha coefficient was computed to assess the questionnaire’s reliability for both portions and the overall score. The findings are displayed in Table 5.
The results displayed in the table are evident. The Cronbach’s alpha (α) coefficients for part 1 and part 2 were found to be 0.716 and 0.737, respectively. These values are considered acceptable as they exceed the threshold of 0.70. Additionally, the α coefficient for the overall score of the questionnaire was calculated to be 0.726. Based on these α coefficient values, it can be concluded that the questionnaire is reliable and it was used the normal distribution test is used to assess the distribution of data. It involves several statistical tests, with the Kolmogorov–Smirnov test and the Shapiro test being the most significant. The results of these tests are presented in Table 6.
It is clear from the results shown in Table 6 that the value of the significance level (Sig.) is greater than 0.05 for both tests and the two variables studied, and therefore this indicates that the data is distributed normally and it was used multicollinearity test between study variables is a statistical phenomenon that occurs when there is a strong correlation between the independent variables in a regression model. The objective is to prevent a significant and positive relationship between the variables, which results in the issue of multicollinearity. In such cases, one of the variables needs to be removed during analysis as a high correlation suggests that they are measuring the same phenomenon. Ultimately, the relationship between them remains unknown, as they are both assessed using the same metric. The Variance Inflation Factor (VIF) and Tolerance value are frequently employed measures to assess multicollinearity. To confirm the absence of multicollinearity, the VIF and Tolerance value should be equal to or greater than 0.10, and the inverse of the VIF should be less than or equal to 10 in Table 7:
The variance inflation factor (VIF) values for the independent variables, as shown in Table 7, ranged from 2.007 to 2.418. These values are considered acceptable as they are below 10. Additionally, the tolerance values for the independent variables ranged from 0.414 to 0.498, which is greater than 0.10. This indicates that there is no issue with multicollinearity.
and it was used Descriptive statistical analysis of study variables The following is a presentation of the interpretation of the results of the sample members’ answers about the study variables and their dimensions by performing statistical measures (mean, standard deviation, and coefficient of variation). Table 8 shows the categories of arithmetic means as follows:
The analysis was conducted of the study sample’s answers to the independent variable (AI techniques) in Table 9, which presents an analysis of the responses related to the independent variable (Artificial Intelligence techniques): We notice that “AI on curricula” achieved the highest mean (4.94) with the lowest coefficient of variation (7.64%), indicating that this dimension has high agreement among respondents regarding the importance of AI’s impact on curricula. Meanwhile, “AI on decision-making” comes in first place in terms of importance with a mean of (4.28) and a lower coefficient of variation (7.61%), meaning that the use of AI techniques in decision-making is of great importance to respondents. Distance learning achieved a mean of (4.37) and a relatively high coefficient of variation (9.13%), indicating some variations among respondents regarding the impact of AI in this area. Training came with a mean of (4.02) and a coefficient of variation of (8.11%), suggesting that this aspect receives relatively less focus compared to the other dimensions.
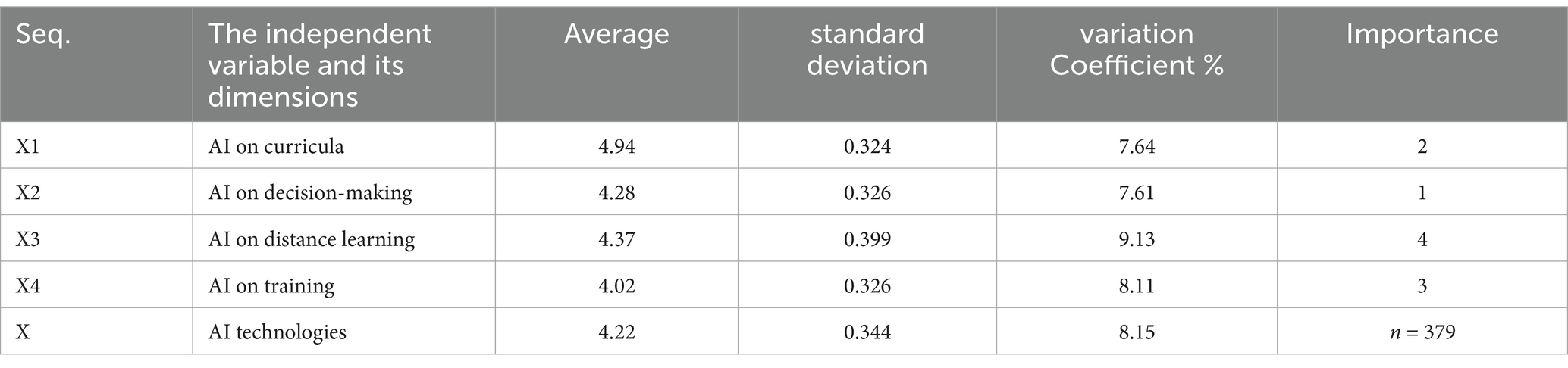
Table 9. Mean, standard deviation, and coefficient of variation for the independent variable and its dimensions.
The analysis was conducted of the study sample’s answers to the dependent variable (quality of educational service) in Table 10, which presents an analysis of the responses related to the dependent variable (quality of educational services): We note that the “Tangibility” dimension received the highest average (4.99) and the lowest coefficient of variation (1.78%), indicating that the quality of equipment and infrastructure in educational institutions is considered excellent and highly agreed upon among respondents. “Responsiveness” received an average of (4.78) and a coefficient of variation of (4.29%), indicating a high level of satisfaction regarding the institutions’ ability to quickly handle student complaints and needs.”Reliability” received an average of (4.37) and a low coefficient of variation (6.84%), indicating good trust between students and the institution’s administration. As for “Empathy” and “Dependability,” they achieved high averages (4.61 and 4.52 respectively) with relatively higher variation in responses.
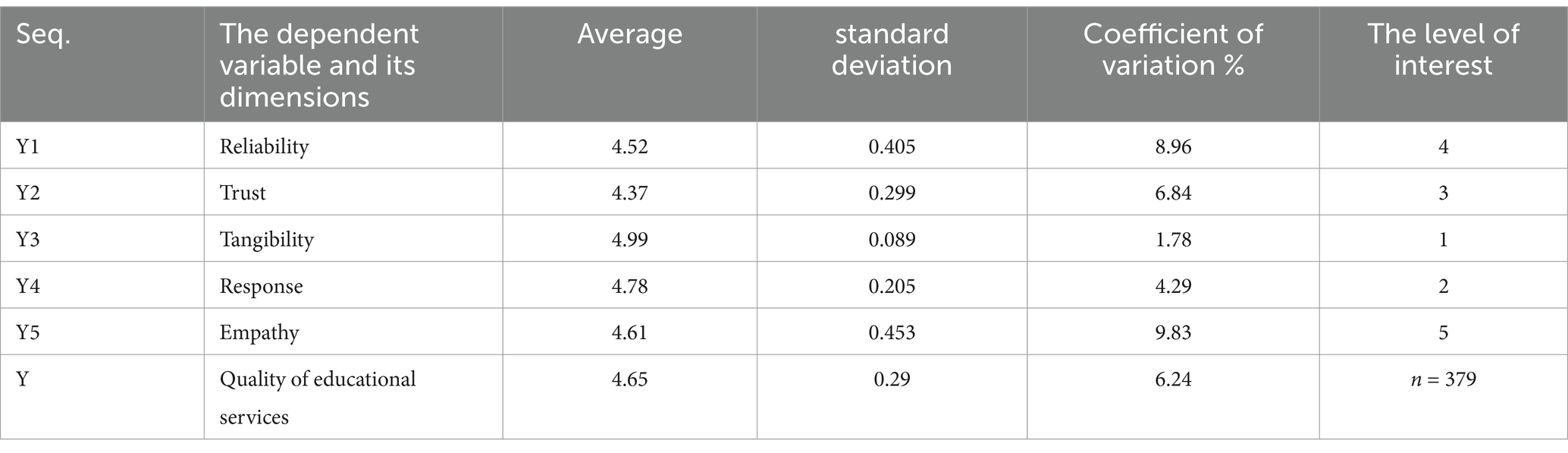
Table 10. Mean, standard deviation, and coefficient of variation for the dependent variable and its dimensions.
The Correlations between the study variables can be determined through The results presented in Table 11 demonstrate a significant positive correlation of high moral importance between AI techniques and the dependent variable, quality of educational services. The correlation coefficient of 0.719 at a confidence level of 99% confirms the validity of the main hypothesis. There exists a notable association between AI technology and the quality of educational services. The table that there is a robust positive correlation, with statistical significance, between the AI on AI on curricula and all dimensions of the dependent variable, both individually and overall. The correlation coefficient is 0.724, indicating a strong relationship. This correlation is observed with a confidence level of 99% about the quality of educational services in general. The table demonstrates a substantial positive connection with high moral importance between the field of AI on decision-making and the dimensions of the dependent variable, both branched and overall. The correlation coefficient between the quality of educational services and the total achieved was 0.777, with a confidence level of 99%.
The findings are displayed in the table. There is a robust and positive association of great moral importance between the field of distant learning and the dimensions of the dependent variable, which is the quality of educational services. The correlation coefficient achieved a value of 0.734, with a confidence level of 99%. The study findings presented in Table 11 indicate a robust and positive connection between the field of training and the dimensions of the dependent variable, which is the quality of educational services, with significant moral significance. The correlation coefficient achieved a value of 0.708, with a confidence level of 99%.
In addition, the researchers conducted a regression analysis to determine the regression coefficient (β) and the coefficient of determination (R2). The coefficient of determination measures the percentage of the effect of the independent variable on the dependent variable. The researchers also compared the calculated value of (F) with its tabular value to determine whether to accept or reject the hypothesis.
The impact of the AI Curricula on the quality of educational services can be determined through Table 12. The results indicate that the coefficient of determination (R2) is 0.524, which means that 52.4% of the changes in the quality of educational services can be attributed to the AI Curricula. The remaining 47.6% is attributed to unknown factors that were not included in the model. An increase in the AI curricula by one unit results in an improvement in the quality of educational services. This relationship is supported by a regression coefficient (β) of 0.007, indicating a positive correlation. Additionally, the calculated (t) value of 2.260 exceeds the tabulated value of 1.960 at a significance level of 0.05, providing further evidence of the relationship. Furthermore, the calculated (F) value of 5.107 is greater than the tabulated value of 3.84 at a significance level of 0.05, with a confidence level of 95%. The impact of AI curriculum on educational services is evident in all aspects of the quality of educational services.
The impact of AI decision-making on the quality of educational services can be determined through Table 13. The results indicate that the coefficient of determination (R2) is 0.604, which means that 60.4% of the changes in the quality of educational services can be attributed to AI decision-making. The remaining 39.6% is attributed to unknown factors that were not included in the model. An increase in AI decision-making by one unit results in an improvement in the quality of educational services. This relationship is supported by a regression coefficient (β) of 0.081, indicating a positive correlation. Additionally, the calculated (t) value of 3.625 exceeds the tabulated value of 1.960 at a significance level of 0.05, providing further evidence of the relationship. Furthermore, the calculated (F) value of 13.138 is greater than the tabulated value of 3.84 at a significance level of 0.01, with a confidence level of 99%. The impact of AI decision-making on educational services is evident in all aspects of the quality of educational services.

Table 13. Results of the impact of AI decision-making on the quality of educational services and its dimensions.
The impact of AI Distance Learning on the quality of educational services can be determined through Table 14. The results indicate that the coefficient of determination (R2) is 0.539, which means that 53.9% of the changes in the quality of educational services can be attributed to AI distance learning. The remaining 46.1% is attributed to unknown factors that were not included in the model. An increase in AI Distance Learning by one unit results in an improvement in the quality of educational services. This relationship is supported by a regression coefficient (β) of 0.008, indicating a positive correlation. Additionally, the calculated (t) value of 2.324 exceeds the tabulated value of 1.960 at a significance level of 0.05, providing further evidence of the relationship. Furthermore, the calculated (F) value of 5.731 is greater than the tabulated value of 3.84 at a significance level of 0.05, with a confidence level of 95%. The impact of AI Distance Learning on educational services is evident in all aspects of the quality of educational services.
The impact of AI training on the quality of educational services can be determined through Table 15. The results indicate that the coefficient of determination (R2) is 0.501, which means that 50.1% of the changes in the quality of educational services can be attributed to AI training. The remaining 49.9% is attributed to unknown factors that were not included in the model. An increase in AI training by one unit results in an improvement in the quality of educational services. This relationship is supported by a regression coefficient (β) of 0.021, indicating a positive correlation. Additionally, the calculated (t) value of 1.963 exceeds the tabulated value of 1.960 at a significance level of 0.05, providing further evidence of the relationship. Furthermore, the calculated (F) value of 3.958 is greater than the tabulated value of 3.84 at a significance level of 0.05, with a confidence level of 95%. The impact of AI training on educational services is evident in all aspects of the quality of educational services.
The impact of AI technologies on the quality of educational services can be determined through Table 16. The results indicate that the coefficient of determination (R2) is 0.517, which means that 51.7% of the changes in the quality of educational services can be attributed to AI technologies. The remaining 49.3% is attributed to unknown factors that were not included in the model. An increase in AI technologies by one unit results in an improvement in the quality of educational services. This relationship is supported by a regression coefficient (β) of 0.116, indicating a positive correlation. Additionally, the calculated (t) value of 2.655 exceeds the tabulated value of 1.960 at a significance level of 0.05, providing further evidence of the relationship. Furthermore, the calculated (F) value of 7.049 is greater than the tabulated value of 3.84 at a significance level of 0.01, with a confidence level of 99%. The impact of AI technologies on educational services is evident in all aspects of the quality of educational services.
5 Discussion
The results indicate a significant positive correlation (correlation coefficient of 0.719) at a confidence level of 99% between AI techniques and educational service quality. This suggests a strong and meaningful relationship between the two variables. The results revealed a robust and meaningful positive correlation between the curriculum and all aspects of the dependent variable, both individually and collectively. The correlation coefficient of 0.724 indicates a strong relationship, and the statistical significance at a confidence level of 99% confirms the presence of a significant association between the curriculum and educational service quality. There is also a strong positive relationship between the decision-making field and the dimensions of the dependent variable, both individually and collectively. This relationship has a high moral significance. The total correlation coefficient between the decision-making field and educational service quality is 0.777, which is statistically significant at a confidence level of 99%. Therefore, there is a clear and significant relationship between the decision-making field and educational service quality.
The results demonstrated a robust and statistically significant positive correlation (correlation coefficient of 0.734) between AI on distance learning and the dimensions of educational service quality. This indicates a statistically significant relationship between AI on distance learning and educational service quality. The results also demonstrate a robust and statistically significant positive correlation (correlation coefficient of 0.708) between the training field and the dimensions of the dependent variable of educational service quality. This indicates a significant relationship between the training field and educational service quality.
During the assessment of the influence of AI techniques on the quality of educational services and its various aspects, the findings reveal a determination coefficient (R2) value of 0.517. This indicates that AI on the curriculum contributes 51.7% to the variations in the quality of educational services. The remaining proportion (48.3%) is ascribed to unidentified variables that were not accounted for in the model. An increase in the value of AI approaches by one unit results in an improvement in the quality of educational services, as indicated by the regression coefficient (β) of 0.116. The computed value of (t) (2.655) exceeded the tabulated value of (1.960) at a significance level of (0.05). The computed value of (F) (5.107) exceeds the critical value of (3.84) at a significance level of (0.05) and a confidence level of 95%. The previous results demonstrate that AI approaches have a substantial influence on all aspects of the quality of educational services. Therefore, the first hypothesis is confirmed:
H5: AI has a positive effect on the quality of educational services.
The results indicate that the determination coefficient (R2) for the impact of curricula on the quality of educational services is 0.524, which means that AI on curriculum accounts for 52.4% of the variations in the quality of educational services. The remaining proportion (47.6%) is ascribed to unidentified variables that were not accounted for in the model. An increase of one unit in the value of curriculum results in a corresponding increase in the quality of educational services, as indicated by the regression coefficient (β) of 0.007. The computed value of (t) (2.260) exceeded the critical value (1.960) at a significance level of (0.05), while the computed value of (F) (5.107) shows that it surpasses the critical value (3.84) at a significance level of (0.05) with a confidence level of 95%. The previous results demonstrate that AI on curriculum has a substantial influence on all aspects of educational service quality, except the reliability component. Therefore, the second hypothesis is confirmed:
H6: AI in curricula has a positive effect on the quality of educational services.
However, during the examination of the influence of decision-making on the quality of educational services and its aspects, the findings reveal that the coefficient of determination (R2) has a value of 0.604, indicating that the combined effect of AI on decision-making is responsible for 60.4% of the variations observed in the quality of educational services. The remaining proportion (39.6%) is ascribed to unidentified variables that were not accounted for in the model. An increase in decision-making value by one unit results in an improvement in the quality of educational services, as indicated by the regression coefficient (β) of 0.008. The calculated t-value (3.625) exceeded the tabulated value (1.960) at a significance level of (0.05), indicating statistical significance. Similarly, the estimated F-value (13.138) indicates significance at a confidence level of 95%. The previous results demonstrate that decision-making has a substantial influence on all aspects of educational service quality, except reliability and responsiveness. Therefore, the third hypothesis is confirmed:
H7: AI in decision-making has a positive effect on the quality of educational services.
However, in the study examining the effect of distance education on the quality of educational services and its various aspects, the findings reveal a determination coefficient (R2) value of 0.539, indicating that AI on distance education contributes to 53.9% of the educational services. The remaining proportion (46.1%) is ascribed to unidentified variables that were not accounted for in the model. An increase in the value of distance education by one unit results in an improvement in the quality of educational services, as indicated by the regression coefficient (β) of 0.008. The computed value of (t) (2.324) exceeded the tabulated value of (1.960) at a significance level of (0.05). The computed value of (F) (5.731) demonstrates statistical significance with a confidence level of 95%. Furthermore, the previous results demonstrate that distance education has a substantial influence on all aspects of the quality of educational services, except for reliability, tangibility, and responsiveness. Therefore, the fourth hypothesis is confirmed:
H8: AI in distance education has a positive effect on the quality of educational services.
In contrast, the test examining the effect of training on the quality of educational services and its various aspects revealed a determination coefficient (R2) value of 0.501. This indicates that training contributes to 50.1% of the changes observed in the quality of educational services. The remaining proportion (49.9%) is ascribed to unidentified variables that were not accounted for in the model. An increase in the value of training by one unit results in an improvement in the quality of educational services, as indicated by the regression coefficient (β) of 0.021. The computed value of (t) (1.964) exceeded the tabulated value of (1.960) at a significance level of (0.05). The computed value of (F) (3.958) demonstrates statistical significance with a confidence level of 95%. The previous results demonstrate that AI in training has a substantial influence on all characteristics of educational service quality, except tangibility and empathy. Therefore, the fifth hypothesis is confirmed:
H9: AI in training has a positive effect on the quality of educational services.
Artificial intelligence technologies have a crucial impact on enhancing the quality of educational services. They achieve this by automating fundamental tasks in higher education, tailoring educational programs to meet the specific requirements of undergraduate and graduate students, identifying and addressing weaknesses in lectures and AI on curricula delivered by instructors, aiding instructors and students in creating personalized training courses based on their individual needs, monitoring students’ progress, and notifying teachers of any issues in students’ performance or decline. Additionally, AI-supported educational programs enable students to learn and acquire essential skills regardless of their location.
The text explores previous research that has investigated the influence of artificial intelligence in higher education. Our study agrees with the study titled “The Use of Artificial Intelligence Techniques and Their Impact on Improving the Higher Education Outcomes of Business Administrative Colleges.” In their studies, they examined how artificial intelligence tools might enhance higher education outcomes in Business Administrative Colleges at the universities featured in the research community. The study sample included 130 academic responders from the universities under consideration. Research indicates that employing AI approaches (e.g., expert systems, neural networks) significantly enhances higher education outputs in Business Administrative Colleges. Artificial intelligence technologies aid in identifying graduates capable of modernization and professional development across numerous domains of work. These technologies enhance graduates’ labour market skills and equip them with new abilities to fulfil their tasks. The report suggests that institutions should prioritize expert systems technology to enhance higher education outcomes through computer-based curriculum reformulation (Atieh et al., 2023).
Moreover, our study aligns with the study titled “Effect of Artificial Intelligence on Learning Quality & Practices in Higher Education.” This study provided a background and listed the primary research topics and objectives. Professional and personal qualities needed for organizational growth are developed by AI. The learning systems use this strategy to boost student productivity and efficiency as needed. During and after COVID, online learning has been a key medium for education and skill development. Student problem-solving skills improve via enhanced cognitive quality. This study used a secondary qualitative strategy to gain knowledge from relevant peer-reviewed journals. To assess artificial intelligence’s role in higher education, thematic analysis was conducted by evaluating the research articles (Rajesh et al., 2022). According to international assessments, Artificial Intelligence in Education (AIEd) is a growing field within educational technology. Although AI has been around for thirty years, educators are still unsure how to use technology to improve higher education teaching and learning. The benefits and constraints of AI in higher education are examined in this research. It also discusses how emerging technologies affect student learning and institutional evolution. To provide equal, high-quality education, the article presents instances of AI in education. First, the article explores how AI might improve learning outcomes by helping higher education systems use data to promote fairness and quality. Finally, we propose guidelines for AI in education to initiate discussions about its benefits, potential, and hazards for sustainable development. Research consistently demonstrates that AI applications offer a multitude of advantages in improving the quality of education. This is accomplished by providing prompt assistance, tailored instruction, and promoting enhanced engagement between students and teachers. The ongoing research in the College of Pharmacy aligns with these prevailing patterns, to harness AI technology to enhance educational services and provide a sophisticated, tailored learning experience for students.
The results of this study align with previous research, which collectively indicates that AI technologies have a beneficial effect on the quality of education and the enhancement of the learning experience.
“Although the results indicate a clear and positive impact of Artificial Intelligence techniques on the quality of educational services, the study has some biases and limitations. One of the main limitations is that the study sample was restricted to students from the College of Pharmacy only, which may limit the generalizability of the results to other colleges or universities. Furthermore, issues related to technological infrastructure, unstable internet services, can affect students’ interactions with AI-based educational tools, creating disparities in their experiences with technology”.
5.1 Conclusion and future direction
The current study’s findings determined that the institution should enhance its commitment to providing students with intelligent training platforms and programs that specify learning methodologies, such as Coursera, edX, Khan Academy, Udemy, LinkedIn Learning, and Duolingo. It is vital to enhance and advance the intelligent technology employed at the institution for decision-making purposes, such as utilizing management information systems (MIS) to gather, retain, and scrutinize data about students. There is a requirement to improve the Internet service for college students, as well as facilitate the availability and downloading of programs for the electronic student system on students’ mobile devices. Furthermore, we must intensify our efforts to offer scholarships and grants to college students who are pursuing studies in cutting-edge AI technologies, the realm of education, and the provision of student services.
Therefore, these findings emphasize the need to incorporate a dedicated methodological course on AI into the college’s curriculum. This would be a crucial measure in enhancing students’ abilities and equipping them to stay abreast of future technological advancements. Additionally, it is imperative to enhance the Educational Guidance Unit’s engagement in fostering students’ concepts related to investing in AI technologies within their respective areas of expertise. This study has certain limitations as it employed a descriptive approach rather than an intervention study. Therefore, we suggest that other researchers investigate students from other colleges and universities in Iraq and implement interventions using AI approaches to enhance the quality of educational services offered to university students.
The findings from the study on the impact of artificial intelligence (AI) in education provide valuable insights that contribute significantly to the broader field of AI in education. First, they demonstrate the effectiveness of AI techniques in enhancing educational quality, particularly in areas such as curriculum development, decision-making, distance learning, and training. This aligns with existing literature that emphasizes the transformative potential of AI in educational settings, promoting personalized learning experiences and improving student engagement.
By confirming that AI positively influences various dimensions of educational service quality, the study supports the notion that integrating AI into educational practices can lead to more adaptive and effective learning environments. This is crucial as educational institutions worldwide strive to meet the diverse needs of students. The results reinforce the importance of investing in AI technologies to facilitate better educational outcomes and address challenges in traditional teaching methodologies.
The field of using artificial intelligence in education is wide and growing, and many research opportunities can be explored in the future. By conducting more research, we can better understand how to leverage these technologies to improve the quality of education and meet the changing needs of students. Researchers can focus on specific areas in the future, such as comparing the experience of the University of Baghdad with that of other universities in Iraq and the Arab region to identify best practices and determine gaps. The scope of the study can also be expanded to include other universities in Iraq or other Arab countries to generalize the results. Additionally, studying how to use artificial intelligence to improve the distance learning experience, assess student performance more accurately and effectively, and design customized curricula for each student presents valuable opportunities for future research.
Data availability statement
The original contributions presented in the study are included in the article/supplementary material, further inquiries can be directed to the corresponding author.
Ethics statement
Written informed consent was obtained from the individual(s) for the publication of any potentially identifiable images or data included in this article.
Author contributions
NF: Methodology, Supervision, Visualization, Writing – review & editing. BS: Writing – original draft, Writing – review & editing. MZ: Conceptualization, Data curation, Formal analysis, Funding acquisition, Investigation, Methodology, Project administration, Resources, Software, Supervision, Validation, Visualization, Writing – review & editing. BA: Conceptualization, Data curation, Formal analysis, Funding acquisition, Investigation, Methodology, Project administration, Resources, Software, Supervision, Validation, Visualization, Writing – original draft.
Funding
The author(s) declare that no financial support was received for the research, authorship, and/or publication of this article.
Conflict of interest
The authors declare that the research was conducted in the absence of any commercial or financial relationships that could be construed as a potential conflict of interest.
Publisher’s note
All claims expressed in this article are solely those of the authors and do not necessarily represent those of their affiliated organizations, or those of the publisher, the editors and the reviewers. Any product that may be evaluated in this article, or claim that may be made by its manufacturer, is not guaranteed or endorsed by the publisher.
References
Abdullah, S. H. (2021). The role of AI techniques in improving the behavior and practices of faculty members when switching to eLearning in light of the covid-19 crisis. Int. J. Educ. Pract. 9, 687–714. doi: 10.18488/journal.61.2021.94.687.714
Aboubakr, R. M., and Bayoumy, H. M. (2022). Evaluating educational service quality among dentistry and nursing students with the SERVQUAL model: a cross-sectional study. J. Taibah Univ. Med. Sci. 17, 648–657. doi: 10.1016/j.jtumed.2022.01.009
Alayoubi, M. M., Al Shobaki, M. J., and Abu-Naser, S. S. (2020). Strategic leadership practices and their relationship to improving the quality of educational service in Palestinian universities. Int. J. Business Market. Manag. 5, 11–26. Available at: http://www.ijbmm.com/paper/Mar2020/8340436031.pdf
Ali, O., Murray, P., Momin, M., Dwivedi, Y., and Malik, T. (2023). The effects of AI applications in educational settings: challenges and strategies. Technol. Forecast. Soc. Chang. 199, 123076–121625. doi: 10.1016/j.techfore.2023.123076
Alijanzadeh, M., Fattahi, H., Veisi, F., Alizadeh, B., Khedmatgozar, Z., and Gholami, S. (2018). Assessment of educational service quality gap: the students’ perspectives. Educ. Res. Med. Sci. 7, 1–7. doi: 10.5812/erms.80246
Atieh, K. A. F. T., Ahmad, G. M. S. A., Awwad, M. A. D. Q., and Al Shobaki, M. J. (2023). The use of artificial intelligence techniques and their impact on improving the higher education outcomes of business administrative colleges in Palestinian universities. Int. J. Eng. Inform. Syst. 7, 83–92. Available at: https://philpapers.org/rec/ATITUO
Baker, T., and Smith, L. (2019). Educ-AI-tion rebooted? Exploring the future of AI in schools and colleges. Retrieved from Nesta Foundationwebsite. Available at: https://media.nesta.org.uk/documents/Future_of_AI_and_education_v5_WEB.pdf
Benbya, H., Davenport, T. H., and Pachidi, S. (2020). AI in organizations: current state and future opportunities. MIS Q. Exec. 19, 1–15. doi: 10.2139/ssrn.3741983
Booyse, D., and Scheepers, C. B. (2023). Barriers to adopting automated organisational decision-making through the use of AI. Manag. Res. Rev. 47, 64–85. doi: 10.1108/MRR-09-2021-0701
Chassignol, M., Khoroshavin, A., Klimova, A., and Bilyatdinova, A. (2018). AI trends in education: a narrative overview. Proc. Comput. Sci. 136, 16–24. doi: 10.1016/j.procs.2018.08.233
Chen, L., Chen, P., and Lin, Z. (2020). AI in education: a review. IEEE Access 8, 75264–75278. doi: 10.1109/ACCESS.2020.2988510
Crompton, H., and Burke, D. (2023). Artificial intelligence in higher education: the state of the field. Int. J. Educ. Technol. High. Educ. 20:22. doi: 10.1186/s41239-023-00392-8
ElHassan, B. T., and Arabi, A. A. (2024). "Ethical forethoughts on the use of AI in medicine", international journal of ethics and systems. Int. J. Ethics Syst. 1. doi: 10.1108/IJOES-08-2023-0190
El-Masry, O. N. (2022). The role of AI technologies in improving the quality of services provided to students at the University of Jordan from their perspective. J. Faculty Educ. 38, 265–290. Available at: https://journals.ekb.eg/article_271572_94972089fc62b48df38e16a53b28a6d0.pdf
-Farhan, L. A., Luay, L. M., Fattah, M., and Abdel, A. L. M. (2022). AI and its impact in the quality of educational service. Alkut university college journal, 124–135. Available at: https://js.alkutcollege.edu.iq/article_19556.html
Feng, X., and Tang, X. (2021). Learning analytics: improving educational decision making. J. Educ. Data Mining 13, 45–60. doi: 10.1234/jedm.v13i2.234
Ganbold, B., Park, K., and Hong, J. (2022). Study of educational service quality in Mongolian universities. Sustain. For. 15:580. doi: 10.3390/su15010580
Goodfellow, I., Bengio, Y., and Courville, A. (2016). Deep learning : MIT Press. Available at: https://e-hir.org/upload/pdf/hir-22-351.pdf
Habib, S., Vogel, T., Anli, X., and Thorne, E. (2024). How does generative AI impact student creativity? J. Creat. 34, 100072–100077. doi: 10.1016/j.yjoc.2023.100072
Haming, M., Murdifin, I., Syaiful, A. Z., and Putra, A. H. P. K. (2019). The application of SERVQUAL distribution in measuring customer satisfaction of retails company. J. Distrib. Sci. 17, 25–31. doi: 10.15722/jds.17.2.201902.25
Holmes, W., Bialik, M., and Fadel, C. (2019). AI in education promises and implications for teaching and learning. Center for Curriculum Redesign. Available at: https://discovery.ucl.ac.uk/id/eprint/10139722/
Jain, S., and Jain, R. (2019). Role of AI in higher education—an empirical investigation. IJRAR-Int. J. Res. Anal. Rev. 3, 146–152. doi: 10.46843/jiecr.v3i2.88
Kaplan, A., and Haenlein, M. (2019). A brief history of AI: on the past, present, and future of AI. Calif. Manag. Rev. 61, 5–14. doi: 10.1177/0008125619864925
Kohnke, L., Moorhouse, B. L., and Zou, D. (2023). Exploring generative AI preparedness among university language instructors: a case study. Comput. Educ. AI 5:100156. doi: 10.1016/j.caeai.2023.100156
Kong, S. C., Cheung, W. M. Y., and Zhang, G. (2021). Evaluation of an AI literacy course for university students with diverse study backgrounds. Comput. Educ. AI 2:100026. doi: 10.1016/j.caeai.2021.100026
Königstorfer, F., and Thalmann, S. (2020). Applications of AI in commercial banks – a research agenda for behavioral finance. J. Behav. Exp. Financ. 27, 100352–100310. doi: 10.1016/J.JBEF.2020.100352
Kuhl, P., Hannemann, T., and Gall, J. (2019). Personalized learning with artificial intelligence: applications and ethics. IEEE Trans. Learn. Technol. 12, 305–315. doi: 10.1109/TLT.2018.2868679
Li, F., and Wang, C. (2023). AI and edge computing for teaching quality evaluation based on 5G-enabled wireless communication technology. J. Cloud Comput. 12:45. doi: 10.1186/s13677-023-00418-6
Likert, R. (1932). Atechique for the measurement of attitudes, Archie’s of psychology. New York: Columbia University press.
Luckin, R., and Holmes, W. (2016). Intelligence unleashed: an argument for AI in education. UCL Knowledge Lab: London, UK.
Lui, A., and Lamb, G. W. (2018). AI and augmented intelligence collaboration: regaining trust and confidence in the financial sector. Inform. Commun. Technol.Law 27, 267–283. doi: 10.1080/13600834.2018.1488659
Martínez-Comesaña, M., Rigueira-Díaz, X., Larrañaga-Janeiro, A., Martínez-Torres, J., Ocarranza-Prado, I., and Kreibel, D. (2023). Impact of AI on assessment methods in primary and secondary education: systematic literature review. Revista de Psicodidáctica (English ed.) 28, 93–103. doi: 10.1016/j.psicoe.2023.06.002
McGrath, C., Pargman, T. C., Juth, N., and Palmgren, P. J. (2023). University teachers' perceptions of responsibility and AI in higher education-an experimental philosophical study. Comput. Educ. AI 4:100139. doi: 10.1016/j.caeai.2023.100139
Mhlanga, D. (2020). Industry 4.0 in finance: the impact of AI (AI) on digital financial inclusion. Int. J. Finan. Stud. 8, 1–45. doi: 10.3390/ijfs8030045
Mukherjee, A. N. (2022). Application of AI: benefits and limitations for human potential and labor-intensive economy–an empirical investigation into pandemic ridden Indian industry. Manag. Matters 19, 149–166. doi: 10.1108/MANM-02-2022-0034
Mureșan, M. (2023). Impact of Artificial Intelligence on Education. In Proceedings of the 32nd International RAIS Conference on Social Sciences and Humanities (Scientia Moralitas Research Institute) pp. 81–85.
Noor, N., Rao Hill, S., and Troshani, I. (2022). Developing a service quality scale for artificial intelligence service agents. Eur. J. Mark. 56, 1301–1336. doi: 10.1108/EJM-09-2020-0672
Ocaña-Fernández, Y., Valenzuela-Fernández, L. A., and Garro-Aburto, L. L. (2019). Inteligencia artificial y sus implicaciones en la educación superior. Propósitos Y Representaciones 7, 536–568. doi: 10.20511/pyr2019.v7n2.274
Park, J., Teo, T. W., Teo, A., Chang, J., Huang, J. S., and Koo, S. (2023). Integrating AI into science lessons: teachers’ experiences and views. Int. J. STEM Educ. 10:61. doi: 10.1186/s40594-023-00454-3
Popenici, S. A., and Kerr, S. (2017). Exploring the impact of AI on teaching and learning in higher education. Res. Pract. Technol. Enhanc. Learn. 12, 22–13. doi: 10.1186/s41039-017-0062-8
Rahiman, H. U., and Kodikal, R. (2024). Revolutionizing education: AI empowered learning in higher education. Cogent Educ. 11, 1–24. doi: 10.1080/2331186X.2023.2293431
Rajesh, E., Shreevamshi, D. V., Krishna, S., and Maguluri, L. (2022). The effect of the artificial intelligence on learning quality & practices in higher education. J. Posit. School Psychol. 6, 2371–2378. Available at: http://journalppw.com
Roll, I., and Wylie, R. (2016). Evolution and revolution in artificial intelligence in education. Int. J. Artif. Intell. Educ. 26, 582–599. doi: 10.1007/s40593-016-0110-3
Roumate, F. (2023). “Ethics of artificial intelligence, higher education, and scientific research” in Artificial intelligence in higher education and scientific research: Future development (Singapore: Springer Nature Singapore), 129–144.
Stachowicz-Stanusch, A., and Amann, W. (2018). AI at universities in Poland. Organizacja i Zarządzanie: Journal kwartalnik naukowy 2, 65–82. doi: 10.29119/1899-6116.2018.42.6
Stolpe, K., and Hallström, J. (2024). AI literacy for technology education. Comput. Educ. Open 6:100159. doi: 10.1016/j.caeo.2024.100159
Syam, A. R., and Arifin, S. (2019). Quality of educational Services in Islam Perspective. In WESTECH 2018: Proceedings of 1st workshop on environmental science, society, and technology, WESTECH 2018, December 8th, 2018, Medan, Indonesia (Vol. 439). European Alliance for Innovation.
Tai, M. C. (2020). The impact of AI on human society and bioethics. Tzu chi Med. J. 32, 339–343. doi: 10.4103/tcmj.tcmj_71_20
Ussell, S., and Norvig, P. (2016). Artificial intelligence: a modern approach. 3rd Edn: Pearson. Available at: https://thuvienso.hoasen.edu.vn/handle/123456789/8967
Verma, M. (2018). AI and its scope in different areas with special reference to the field of education. Online Submission, 3(1), 5–10. Available at: https://files.eric.ed.gov/fulltext/ED604401.pdf
Woolf, B. P., Lane, H. C., Chaudhri, V. K., and Kolodner, J. L. (2013). AI grand challenges for education. AI Mag. 34, 66–84. doi: 10.1609/aimag.v34i4.2490
Yılmaz, K., and Temizkan, V. (2022). The effects of educational service quality and socio-cultural adaptation difficulties on international students’ higher education satisfaction. SAGE Open 12, 1–18. doi: 10.1177/215824402210783
Zawacki-Richter, O., Marín, V. I., Bond, M., and Gouverneur, F. (2019). Systematic review of research on AI applications in higher education–where are the educators? Int. J. Educ. Technol. High. Educ. 16, 1–27. doi: 10.1186/s41239-019-0171-0
Keywords: artificial intelligence, AI on curricula, AI on decision making, AI on distance learning, AI on training, quality of educational services
Citation: Farhan ND, Sadiq BH, Zwayyer MH and Arnout BA (2024) The impact of using artificial intelligence techniques in improving the quality of educational services/case study at the University of Baghdad. Front. Educ. 9:1474370. doi: 10.3389/feduc.2024.1474370
Edited by:
Huichun Liu, Guangzhou University, ChinaReviewed by:
Sohaib Ahmed, Bahria University, PakistanHanan Alahmari, King Khalid University, Saudi Arabia
Tahani Asiri, Ministry of Education, Saudi Arabia
Copyright © 2024 Farhan, Sadiq, Zwayyer and Arnout. This is an open-access article distributed under the terms of the Creative Commons Attribution License (CC BY). The use, distribution or reproduction in other forums is permitted, provided the original author(s) and the copyright owner(s) are credited and that the original publication in this journal is cited, in accordance with accepted academic practice. No use, distribution or reproduction is permitted which does not comply with these terms.
*Correspondence: Bareq Habeeb Sadiq, YmFyaXEuaEBjb2lzLnVvYmFnaGRhZC5lZHUuaXE=