- 1Administrative Science Department, College of Administrative and Financial Science, Gulf University, Sanad, Bahrain
- 2College of Information Technology, University of Bahrain, Zallaq, Bahrain
Methods: This study examines the impact of Artificial Intelligence (AI) on the field of education, with particular focus on its implications for academic integrity and the adoption of comprehensive assessment approaches. This research fits within the specific setting of university students and faculty members in the Kingdom of Bahrain.
A cross-sectional survey was designed to examine the impact Artificial Intelligence (AI) in field of education, with particular focus on its implications for academic integrity and the adoption of comprehensive assessment approaches. A total of 218 participants were randomly selected from 250 employed in this survey study.
Results: Out of 250 invited participants, 203 responded to the survey. This study evaluated the influence of Educational Impact (EI), Policy and Ethics (PE), and Pedagogical Implications (PI) on Academic Outcomes (AO). Results revealed a significant association between EI → AO with a beta of 0.490, t-value of 4.504, and p < 0.001. PI also showed a significant relationship (β = 0.454, t = 2.330, p = 0.010) with more variability. PE’s impact on AO was modest (β = 0.243, t = 1.977, p = 0.024). Overall, EI was the strongest AO predictor. The R2 value was approximately 39%, indicating a good fit.
Conclusion: The research reveals a strong link between the Educational Impact (EI) of AI and academic success in Bahrain’s universities, with EI being the primary predictor. Both Policy and Ethics (PE) and Pedagogical Implications (PI) play crucial roles in this relationship.
1 Introduction
The advancement of technology, especially in the field of artificial intelligence (AI), has revolutionized numerous sectors, including education (Uunona and Goosen, 2023). While AI offers a plethora of advantages such as personalized learning, automated grading, and content recommendation, it also poses challenges, notably in preserving academic integrity (Sok and Heng, 2023). As AI tools become increasingly sophisticated, so too does the potential for students to utilize these technologies to gain undue advantages in their academic pursuits (Javaid et al., 2023).
Academic integrity is the foundation upon which educational institutions are built. It signifies a commitment to and the demonstration of honest scholarly work (McCabe et al., 2006; Poitras Pratt and Gladue, 2022; Sullivan, 2018). However, with the rise of AI-assisted tools, such as essay generators and automated problem solvers, students today are faced with unprecedented temptations to divert from this path of integrity (Ellison and Patel, 2022); Perkins (2023). These AI tools, while developed with the intention of aiding research and providing quicker solutions, can be easily misused (Chiang et al., 2022). This misuse undermines the very purpose of education to promote understanding, critical thinking, and genuine learning.
Furthermore, the issue is not solely about the direct use of AI to cheat. The omnipresence of these tools can inadvertently shape a student’s mindset about the acceptability of seeking shortcuts in learning (Hamman, 2022). This raises a fundamental question about the role of education in the age of AI: How can institutions ensure genuine learning while leveraging the benefits of AI?
While many educators and institutions have been quick to vilify AI as the root cause of a perceived decline in academic integrity, others argue that it is the environment, teaching methodologies, and assessment strategies that need a rethink (Lin et al., 2023). For instance, if assessments are based solely on rote memorization, students might feel inclined to use AI tools as a shortcut, given the lack of depth and understanding required.
Conversely, if the focus shifts to application, critical thinking, and original content creation, AI tools might prove less tempting and less beneficial for students looking to take shortcuts (Currie, 2023). As such, while the tools themselves present challenges, they also force a necessary evolution in pedagogical approaches (Alam et al., 2022).
In exploring this topic, it’s crucial to not only understand the extent and ways in which AI tools can be misused but also to discover innovative strategies educators can adopt to foster environments that deter such behavior and encourage genuine learning.
1.1 Research questions (RQ)
1. What long-term effects could AI-assisted cheating have on the educational landscape, especially with the rise of online and remote learning?
2. To what extent do application-centric assessment methods foster creativity in students compared to traditional assessment methods?
3. How can educational institutions update their academic integrity policies to address the nuances of AI-assisted cheating?
1.2 Research objectives (RO)
1. Analyzing long-term effects of AI-cheating in online learning on academic outcomes and credential value.
2. Evaluate the impact of application-centric assessments versus traditional knowledge-based assessments on student understanding, creativity, and critical thinking.
3. Examine nuances of AI-cheating in education and recommending policy updates.
This research aims to analyze the influence of artificial intelligence (AI) on academic integrity and the shift towards more comprehensive educational assessment methods in the educational sector (Figure 1).
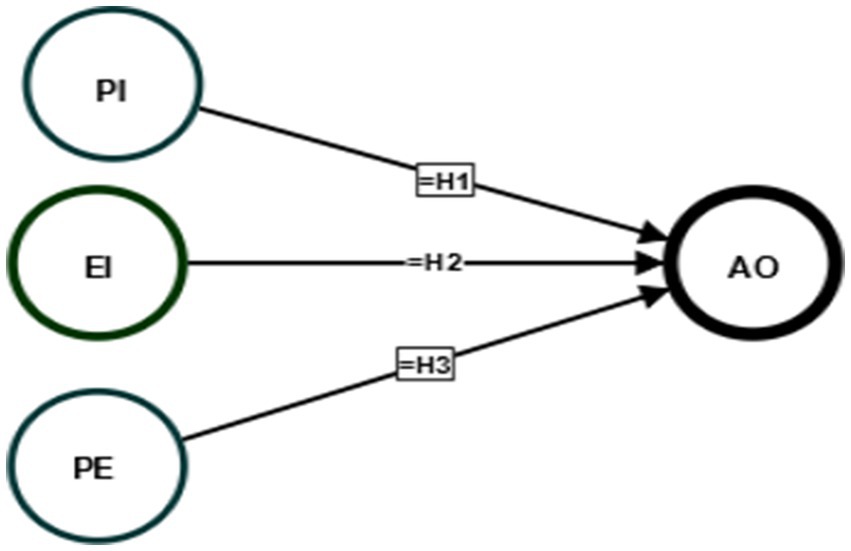
Figure 1. Author’s own conceptual framework and hypotheses model. EI, Educational Impact; PE, Policy and Ethics; PI, Pedagogical Implications; AO, Academic Outcomes.
1.3 Operational definition
1. Pedagogical Implications (PI): The measurable changes in teaching methods that the implementation of AI has brought about are demonstrated by the frequency of using AI tools in lesson plans and the rates at which instructors employ AI-driven instructional strategies.
2. Policy and Ethics (PE): The criteria and mechanisms for ethical use of artificial intelligence (AI) in education are evaluated based on the existence of institutional regulations, adherence to data privacy laws, and recorded instances of ethical violations related to AI.
3. Educational Impact (EI): The quantifiable effects of AI on student learning experiences, measured by improvements in engagement scores, personalized learning assessments, and the efficiency of AI-generated feedback.
4. Academic Outcomes (AO): The quantifiable outcomes of student performance associated with the use of artificial intelligence, assessed by comparing changes in grades, test scores, and achievement rates before and after the integration of AI.
2 Literature review
The rapid integration of artificial intelligence (AI) into the educational sphere has sparked a flurry of research and debates about its effects on academic integrity. Notably, a consensus on the severity and breadth of AI-induced cheating challenges has yet to be reached, but the existing literature provides valuable insights into the dynamics at play.
AI’s Role in Education: Before understanding its implications on cheating, it’s pivotal to grasp the broader role of AI in education. According to Bühler et al. (2022) AI is heralded as a revolutionary force in personalized learning, adaptive content delivery, and student performance analytics. The promise lies in creating bespoke educational experiences tailored to individual student needs (Alam and Mohanty, 2022; Vandenberghe et al., 2022). However, the same adaptive algorithms that can tailor learning experiences can also be utilized to generate assignment answers or even complete essays (Baidoo-Anu and Owusu Ansah, 2023; Kasneci et al., 2023; Malinka et al., 2023).
Emerging AI Tools and Cheating: Numerous AI tools, ranging from simple calculators to complex problem solvers, are now available at students’ fingertips (Tucker, 2024). Dwivedi et al. (2023) categorized these tools into two: assistive and substitutive. Assistive tools aid students in understanding concepts better, while substitutive ones can replace the student in tasks, leading to a potential breach of integrity (Dhara et al., 2022; Figoli et al., 2022; Mattioli et al., 2022). An alarming trend highlighted by Khosravi et al. (2022) is the burgeoning industry of AI-driven essay mills that offer bespoke essays, often undetectable by traditional plagiarism checkers.
Pedagogical Implications: With AI’s capability to complete assignments, there’s an evident need to rethink assessment strategies (Chan, 2023; Ifelebuegu, 2023). Kaltenboeck et al. (2022) advocate for a shift from knowledge-based assessments to application-centric ones, as AI tools are less adept at mimicking human creativity and critical thinking. This sentiment is echoed by Song et al. (2022) who argue that the emphasis should be on open-book assessments, project-based learning, and real-world problem solving.
Institutional Measures: Educational institutions are not standing idle. Rudolph et al. (2023) detailed how schools and universities are leveraging advanced plagiarism detection tools that use AI themselves to detect artificially generated content. However, Bombaerts et al. (2023) points out that while technology can help, the solution is not solely technological but cultural. Building a strong ethos of academic integrity and ensuring students understand the value of genuine learning are equally crucial (Ateeq et al., 2024b; Fudge et al., 2022; Poitras Pratt and Gladue, 2022).
Student Perception and AI: Perhaps the most overlooked aspect is the student’s viewpoint. Abu Mansour and Abu Shosha (2022) conducted a survey revealing that while students are aware of the ethical implications, they feel an immense pressure to perform, leading them to these tools. This indicates a deeper systemic issue in the current educational landscape’s emphasis on grades over holistic learning (Gambhir et al., 2008; Viberg et al., 2018).
2.1 Artificial intelligence in education: implications for academic integrity and the shift toward holistic assessment
The use of artificial intelligence (AI) in academic settings has significant repercussions. Upholding academic integrity is one of the most important aspects (Perkins and Roe, 2023; Surahman and Wang, 2022; Susilawati et al., 2023). Tools that are powered by artificial intelligence, such as Turnitin, allow advanced detection of plagiarism, which ensures that students’ work is unique (Chaudhry et al., 2023; Owan et al., 2023; Skavronskaya et al., 2023). Concurrently, there is a growing awareness of the ways in which AI may be abused. For example, essay generators powered by AI have the potential to create material that may evade detection by traditional methods (Narayanan and Kapoor, 2024; Rudolph et al., 2023). As a consequence of this, educators are modifying their approaches to the evaluation process (Mhlanga, 2023). The focus is changing from traditional evaluation methods to more holistic ones, such as learning via projects, giving presentations, or working on group projects. These methods not only encourage critical thinking, but they are also difficult to deceive using tools provided by artificial intelligence (Alier et al., 2024; Kenwright, 2023; Williamson and Prybutok, 2024). In conclusion, although AI technologies strengthen efforts to assure academic honesty, they also indirectly drive a turn toward evaluation systems that are more thorough and relevant. Consequently, these hypotheses are displayed in detail to ensure they achieve the objectives of the study. Therefore, this has led to the following hypothesis:
H1 (EI → AO): The integration of AI into education will have a beneficial impact on educational impact (EI), resulting in improved academic outcomes (AO), including increased student engagement and performance.
H2 (PE → AO): To ensure the responsible and equitable application of AI technologies in education, effective policies and ethical guidelines regarding AI (PE) will result in improved academic outcomes (AO).
H3 (PI → AO): Changes made to teaching methods by AI will have a big effect on academic outcomes (AO), which will improve student understanding and performance in school.
3 Methodology
3.1 Ethical consideration
The research was conducted in alignment with the highest ethical standards. Participants voluntarily engaged in the study after receiving comprehensive information regarding its objectives (Ateeq et al., 2022; Habtoor and Ali, 2022). Throughout the investigation, utmost care was taken to ensure the anonymity and confidentiality of all contributors.
3.2 Research design
A research design refers to a systematic framework that is developed to effectively answer the research inquiries and ensure the collection of appropriate information for the purpose of testing the hypothesis that was proposed (Ateeq et al., 2023). The components of this framework are the unit of analysis, research inquiries, tools for data collecting, data analysis methods, and the subsequent presentation and interpretation of experimental findings. It is essential that this strategy be congruent with the research model.
As a result of the substantial sample size of university students and faculty in the several universities Kingdom of Bahrain, quantitative methodologies were implemented in this investigation. Quantitative research is typically based on the traditional, positivist, or empirical paradigm and entails a larger sample size than qualitative research (Al-Fahim et al., 2024). Data collection was conducted using a structured questionnaire survey, which is particularly well-suited for analysis using Partial Least Squares Structural Equation Modelling (PLS-SEM).
3.3 Sample
The study focused on university students and faculty in several universities in Bahrain for instance Gulf University, examining the implications of Artificial Intelligence in education, specifically its impact on academic integrity and the trend towards holistic assessment. Out of 250 contacted via convenience sampling, 203 participants were deemed sufficient based on Krejcie and Morgan’s 1970 criteria (Ali et al., 2022).
3.4 Survey
The researcher used convenience sampling to obtain statistical data for this research, which took place from August 1st to August 14th, 2023. The researcher specifically targeted those currently working in the educational industry with the electronic distribution of the questionnaire. The objective of this study was to investigate the many consequences of artificial intelligence (AI) in educational settings, particularly its impact on academic honesty and the transition towards multidimensional evaluation methods. The research effectively collected insights from an easily available subgroup of the population using convenience sampling, offering distinctive perspectives on how AI technologies are transforming educational approaches and results.
3.5 Data analysis
The research used SPSS version 28, PLS-SEM, and Amos to analyze data and examine relationships between latent variables. A Cronbach’s alpha of 0.854 confirmed the questionnaire’s reliability and consistency (Ateeq et al., 2023; Qaid et al., 2024).
4 Results
4.1 Background characteristics of the respondents
The study surveyed respondents’ gender, age, and education. Males slightly outnumbered females, representing 54.4% (n = 110) and 44.4% (n = 93) respectively. The age distribution was diverse: 39.9% (n = 81) were aged 20–31, followed by 24.6% (n = 50) in the 31–41 bracket, 13.7% (n = 28) aged 41–50, and 21.6% (n = 44) above 50. Most respondents were undergraduates at 59.1% (n = 120), but a significant 40.8% (n = 83) held post-graduate degrees. In summary, the sample comprised a slight male majority, a larger younger demographic, and a notable proportion of post-graduates (Tables 1–8).
4.2 μ ± SD and rank for the variables
The table presents mean scores and rankings of four educational constructs: Academic Outcomes (AO), Pedagogical Implications (PI), Policy and Ethics (PE), and Educational Impact (EI). Notably, AO, with a mean of 2.900, ranks first, indicating its paramount importance in the evaluated context. PI follows closely in the second rank, despite having a marginally higher mean of 2.941. Third is PE with 2.851, demonstrating its moderate influence. EI, with a mean of 2.78, is perceived as least impactful, ranking fourth. The minor differences in mean scores (a mere 0.120 difference between the highest and lowest) emphasize that all constructs are closely valued. However, the rank does not directly relate to the number of items, suggesting other influencing factors in the ranking process (Ateeq et al., 2024a; Milhem et al., 2024). The data, thus, highlights the nuanced significance of these constructs in the educational arena.
4.3 Multicollinearity test
Multicollinearity arises when explanatory variables in a regression model are highly correlated (Kalnins, 2022). Using variance inflation factor (Ghavifekr and Rosdy, 2015) to detect it, a VIF above 5–10 often indicates significant multicollinearity (Tai and Division, 2022). Analyzing the provided VIFs, all values are below 5, suggesting minimal multicollinearity. The highest VIF is 2.894 for EI6, still well below the concerned levels. Thus, multicollinearity does not appear to be a significant issue in this data (Giacalone et al., 2018).
4.4 Goodness-of-fit of the model
The presented model exhibits a commendable fit based on standard goodness-of-fit indices. The Chi-Square/df is 2.119, below the desired threshold of 5. The RMSEA, at 0.064, is within the favorable limit of 0.08. GFI (0.981), AGFI (0.951), NFI (0.969), TLI (0.915), and CFI (0.925) all surpass the recommended 0.90 threshold. These results suggest that the model aligns well with the data. However, while these metrics provide strong quantitative evidence of fit, a holistic model evaluation should also consider underlying theory and research implications (Ahmed et al., 2020; Ahmed et al., 2019).
4.5 Construct reliability and validity
The table presents the Cronbach’s alpha (α), composite reliability (CR), and average variance extracted (AVE) of four constructs: AO, EI, PE, and PI. Firstly, all constructs show an α greater than 0.7, suggesting good internal consistency (Abro and Salam, 2014; Milhem et al., 2019). In terms of CR, all values exceed the 0.7 threshold, indicating satisfactory reliability, with PI having the highest CR at 0.944 (Al-Fahim et al., 2024). Regarding Average Variance Extracted (AVE), only Average Obstruction (AO) falls below the recommended threshold of 0.5, indicating weak convergent validity. Conversely, the values of PI, EI, and PE exceed the established threshold, likely indicating the presence of issues (Milhem et al., 2024; Milhem et al., 2024). In conclusion, although all variables demonstrate strong reliability, there is need for improvement in the convergent validity of (AO), (EI), (PE).
4.6 Assessment of measurement model
In the field of educational intelligence (AI), the proposed assessment model looks at how different factors, such as educational impact (EI), policy and ethics (PE), pedagogical implications (PI), and academic outcomes (AO), are connected. The path coefficients demonstrate significant interactions among these factors, revealing both the magnitude and direction of their impacts. The observed variables show high loading values, which indicate the reliability and validity of the constructs. Also, the arrows that connect the concepts emphasize the relationships between them, which shows how well the model can understand how AI affects academic honesty and overall evaluation. See Figure 2.
4.7 Discriminant validity
Discriminant validity assesses whether concepts that should be unrelated are, in fact, unrelated. For discriminant validity to be established, the square root of the Average Variance Extracted (AVE) for a given variable should be greater than its correlation with any other variable (Ateeq A.A. et al., 2024; Pehlivan et al., 2023). By examining the provided matrix, we can observe the diagonal, which presumably represents the square root of the AVE for each variable (AO, EI, PE, PI). For instance, AO’s 0.616 is greater than its correlations with EI (0.560), PE (0.438), and PI (0.476). A similar pattern is observed for EI, PE, and PI. Thus, discriminant validity is confirmed for these constructs as thFe square root of each variable’s AVE is greater than its correlation with the other variables (Ali et al., 2023; Ateeq et al., 2024d).
4.8 R-square
The R-square value for AO stands at 0.389. This indicates that approximately 38.9% of the variability in the dependent variable can be explained by the model, while the remaining 61.1% is unaccounted for. In many contexts, an R-square of 0.389 might be considered moderate, suggesting that while the model has some explanatory power, a significant portion of the variance remains unexplained, which might warrant further investigation or refinement of the model (Faruqe, 2023).
4.9 Effect size F2
In the analysis of f-square values, EI to AO displays a higher value of 0.169, suggesting a more substantial effect on AO compared to PE and PI, which exhibit lower effects of 0.039 and 0.035, respectively. This implies that EI has the most pronounced influence on AO among the predictors.
4.10 Factor loadings of all constructs construct
Table 9 lists factor loadings for various items under four variables: AO, EI, PE, and PI. Analysing the loadings, AO’s items, especially AO4, show strong loadings with AO2 and AO5 following closely. For EI, EI6 has the highest loading, while EI3 stands out as a weak link, potentially indicating less relevance or reliability in that item. PE’s loadings are consistent, with PE2 and PE3 leading; however, PE7 is noticeably weaker and might be less impactful. Lastly, PI items have a tight range of strong loadings, led by PI5. Overall, the majority of items exhibit strong factor loadings, with a few outliers that may require further scrutiny (Alzoraiki et al., 2024; Mede et al., 2022).
4.11 The assessment of the inner model and hypotheses testing procedures
The findings reveal the substantial relationships among the underlying concepts of Educational Impact (EI), Policy and Ethics (PE), Pedagogical Implications (PI), and their impact on Academic outcomes (AO). With a coefficient of 0.388, a high T-value of 4.55, and a p-value of 0.000, the path from EI to AO has a substantial positive impact, demonstrating a statistically significant and robust link. These findings indicate that educational interventions, such as well-designed curriculum, effective teaching approaches, and active student participation, significantly impact academic results. This link highlights the crucial importance of effectively designed educational practices in enhancing student achievement and success. Nevertheless, the correlation between PE and AO, although noteworthy, is less pronounced in comparison to EI. With a coefficient of 0.156, a T-value of 2.04, and a p-value of 0.024, this route suggests that the impact of institutional regulations and ethical practices on academic results is modest. These findings indicate that while policies and ethical principles have a role in influencing the learning environment, their direct influence on student achievement is less significant compared to the instructional techniques themselves. However, strict compliance with effective regulations and ethical principles continues to have a beneficial impact on the general academic achievement of college students. The route from PI to AO shows a modest impact, with a coefficient of 0.218, a T-value of 2.34, and a p-value of 0.010. The aforementioned statement implies that pedagogical strategies, such as instructional innovations, student-teacher interactions, and adaptive teaching techniques, have a significant but not predominant impact on academic achievements. The continued importance of pedagogy in promoting successful learning environments is contingent upon its ability to effectively complement wider educational interventions. Collectively, the results underscore the crucial importance of educational techniques in shaping academic results, with both legislative frameworks and instructional approaches playing supporting but substantial roles. Educational institutions seeking to improve academic achievement should prioritize the quality of education while simultaneously improving policies and teaching methods to provide a comprehensive and efficient learning environment (Table 10).
5 Discussion
Understanding the dynamics between various factors and academic outcomes has long been the subject of numerous studies (Reardon, 2011; Roy, 2023). The current findings throw light on the roles of Educational Impact (EI), Pedagogical Implications (PI), and Policy and Ethics (PE) in shaping Academic Outcomes (AO). This discussion aims to critically analyze the results of these hypotheses in the context of previous research, exploring the nuances of their interrelationships and the implications they hold for the educational realm.
To start, the most dominant predictor of AO in this study was Educational Impact (EI), evidenced by its impressive beta coefficient of 0.490. Historically, many educational researchers have touted the importance of the educational environment, resources, and teaching methodologies in determining students’ academic performance (Howard-Gosse et al., 2023). For instance, Almulla and Al-Rahmi (2023) found a substantial positive correlation between quality teaching resources and improved student outcomes, a sentiment that resonates with the results of H1. Furthermore, Odei-Tettey et al. (2023) highlighted that a well-structured educational system that offers holistic learning experiences significantly improves academic results. Our finding complements these assertions, solidifying the notion that the impact of education has a pronounced effect on AO.
Meanwhile, the influence of Pedagogical Implications (PI) on AO, though significant, demonstrated notable variability. The world of education has witnessed an array of pedagogical shifts over the past few decades, ranging from traditional lecture-based approaches to more interactive, student-centered methodologies (Muscatello, 2023). The significant beta value of 0.454 in H3 is reflective of the importance of pedagogy, which aligns with (Habibi et al., 2023) findings. However, the variability present might be attributed to the multitude of pedagogical strategies available and their differential effectiveness across diverse student populations (Hoffmann et al., 2023). For example, while collaborative learning might prove effective for one group, problem-based learning could be more suited for another. This suggests that while PI remains a key determinant of AO, its effectiveness can vary based on its application and context.
Lastly, the association between Policy and Ethics (PE) and AO, as deduced from H2, seems more moderate when compared with EI and PI. This could stem from the indirect nature of policy and ethics’ impact on academic outcomes. For instance, while schools and institutions may abide by certain educational policies, their translation into classroom practices and eventual student outcomes might not be straightforward (Masters, 2023). Moreover, ethical considerations, such as fairness in assessment and equal opportunities, while foundational, might not show immediate or pronounced effects on AO. Nevertheless, the significance of the PE AO relationship, as evidenced by the p-value of 0.024, cannot be ignored. It accentuates that even the subtler aspects of education, like policy and ethics, play a critical role in determining academic trajectories (Oliveira et al., 2023).
Drawing parallels with previous studies, it’s intriguing to observe the dynamic interplay between these three variables and AO. While each predictor has its unique importance, their collective influence on academic results is undeniable. For future investigations, delving deeper into the intricacies of these relationships can offer granular insights. For instance, exploring which specific elements within EI or PI exert the most influence can help educators fine-tune their strategies for enhanced outcomes.
In conclusion, the present study, in synergy with historical research, underscores the paramountcy of Educational Impact in predicting Academic Outcomes. While Pedagogical Implications and Policy and Ethics have their respective roles, it’s the holistic and encompassing nature of the educational impact that stands out as the most formidable determinant of AO. As educational stakeholders, recognizing and harnessing these insights can pave the way for more informed decisions and strategic implementations in the academic arena.
5.1 Limitations
The limitations of the research include a possible bias in the selection of the sample, hence potentially limiting its ability to accurately reflect the wider student population. The potential limitations of relying just on beta coefficients include the possibility of overlooking intricate interactions between factors. Additionally, it should be noted that the research operates on the assumption of a linear relationship between various variables and academic achievements, consequently disregarding the possible impact of non-linear impacts. Finally, it should be noted that there are other external factors, such as socio-economic position and cultural influences, which were not taken into consideration in this research, but might potentially have an effect on AO.
5.2 Recommendations
Considering the complexities presented by artificial intelligence (AI) in upholding academic integrity, it is imperative to embrace a comprehensive approach to evaluation within the realm of education. This method prioritizes the cultivation of authentic learning experiences as opposed to rote memorization. Given the substantial impact of educational approaches on academic achievements and the variation in efficacy depending on their execution, it is imperative for educators and policymakers to emphasize the comprehension of these processes. This will empower individuals to develop tactics that enhance authentic learning experiences and preserve the integrity of academic accomplishments.
6 Conclusion
The incorporation of artificial intelligence (AI) in the field of education has given rise to apprehensions over the preservation of academic integrity, primarily owing to the proliferation of advanced cheating techniques. The use of a holistic assessment approach serves to alleviate these concerns, fostering a comprehensive comprehension of students’ abilities. The integration of technology and education prioritizes authentic learning experiences rather than rote memorization. The research validates the idea that educational influence plays a substantial role in shaping academic achievements, whereas the effectiveness of pedagogical approaches exhibits diverse consequences depending on their implementation. Therefore, it is crucial to comprehend these processes, prompting educators and policymakers to implement comprehensive solutions in order to maximize the effectiveness of learning.
Data availability statement
The original contributions presented in the study are included in the article/supplementary material, further inquiries can be directed to the corresponding author.
Ethics statement
Ethical review and approval was not required for the study on human participants in accordance with the local legislation and institutional requirements. Written informed consent from the participants was not required to participate in this study in accordance with the national legislation and the institutional requirements.
Author contributions
AA: Writing – review & editing, Writing – original draft, Visualization, Validation, Supervision, Software, Resources, Project administration, Methodology, Investigation, Funding acquisition, Formal analysis, Data curation, Conceptualization. MA: Writing – review & editing, Validation, Methodology, Investigation, Funding acquisition. MM: Writing – review & editing, Supervision, Software, Resources, Project administration, Methodology, Investigation, Funding acquisition. RA: Writing – review & editing, Supervision, Software, Resources, Formal analysis, Data curation, Conceptualization.
Funding
The author(s) declare that no financial support was received for the research, authorship, and/or publication of this article.
Conflict of interest
The authors declare that the research was conducted in the absence of any commercial or financial relationships that could be construed as a potential conflict of interest.
Publisher’s note
All claims expressed in this article are solely those of the authors and do not necessarily represent those of their affiliated organizations, or those of the publisher, the editors and the reviewers. Any product that may be evaluated in this article, or claim that may be made by its manufacturer, is not guaranteed or endorsed by the publisher.
Supplementary material
The Supplementary material for this article can be found online at: https://www.frontiersin.org/articles/10.3389/feduc.2024.1470979/full#supplementary-material
References
Abro, Z., and Salam, M. A. (2014). Motivation, work stress and job performance: an analytical study of faculty in higher education institutions of Sindh. JISR Manag. Soc. Sci. Econ. 12, 17–28. doi: 10.31384/jisrmsse/2014.12.2.2
Abu Mansour, S. I., and Abu Shosha, G. M. (2022). Experiences of first-line nurse managers during COVID-19: a Jordanian qualitative study. J. Nurs. Manag. 30, 384–392. doi: 10.1111/jonm.13530
Ahmed, U., Khalid, N., Islam, D. M. Z., and Abro, Z. (2019). Abuse, emotions, and workload in the distribution business: implications for employees’ engagement. Int. J. Adv. Appl. Sci. 6, 90–99.
Ahmed, K., Zain, M., Milhem, M., and Ahmad, A. (2020). The impact of macroeconomic variables on commodity futures prices: an evidence from Malaysian crude palm oil futures. Eur. J. Mol. Clin. Med. 7, 2095–2105.
Alam, S., Albozeidi, H. F., Al-Hawamdeh, B. O. S., and Ahmad, F. (2022). Practice and principle of blended learning in ESL/EFL pedagogy: strategies, techniques and challenges. Int. J. Emerg. Technol. Learn. 17, 225–241. doi: 10.3991/ijet.v17i11.29901
Alam, A., and Mohanty, A. (2022). Business models, business strategies, and innovations in EdTech companies: integration of learning analytics and artificial intelligence in higher education. Paper presented at the 2022 IEEE 6th conference on information and communication technology (CICT).
Al-Fahim, N. H., Ateeq, A. A., Abro, Z., Milhem, M., Alzoraiki, M., Alkadash, T. M., et al. (2024). “Factors influencing the mobile banking usage: mediating role of perceived usefulness” in Digital technology and changing roles in managerial and financial accounting: theoretical knowledge and practical application, vol. 36 (Emerald Publishing Limited), 115–128.
Ali, A. A. A., AlZgool, M., Alzoraiki, M., Milhem, M., and Al-Absy, M. S. M. (2023). Moderating effect of strategic planning on the relationship between career path planning and job performance. Sustain. For. 15:8490. doi: 10.3390/su15118490
Ali, A. A. A., Alzoraiki, M., and Al Aghbas, N. S. M. (2022). Working remotely, social isolation and access to support and communication during COVID-19 its influence on job performance. Int. J. Intell. Hum. Resour. Manag. 3, 23–33.
Ali, A. A. A., and Habtoor, N. (2022). Islamic work ethics and its impact on enhancing organizational commitment in Bahraini private educational universities. Int. J. Intell. Hum. Resour. Manag. 3, 33–42. doi: 10.46988/IJIHRM.03.02.2022.005
Alier, M., García-Peñalvo, F., and Camba, J. D. (2024). Generative artificial intelligence in education: From deceptive to disruptive. Int. J. Interact. Multimedia Artif. Intell. 8, 5–14. doi: 10.9781/ijimai.2024.02.011
Almulla, M. A., and Al-Rahmi, W. M. (2023). Integrated social cognitive theory with learning input factors: the effects of problem-solving skills and critical thinking skills on learning performance sustainability. Sustain. For. 15:3978. doi: 10.3390/su15053978
Al-refaei, A. A.-A., Ali, H. B. M., Ateeq, A. A., and Alzoraiki, M. (2023). An integrated mediating and moderating model to improve service quality through job involvement, job satisfaction, and organizational commitment. Sustain. For. 15:7978. doi: 10.3390/su15107978
Alzoraiki, M., Ahmad, A. R., Ateeq, A., and Milhem, M. (2024). The role of transformational leadership in enhancing school culture and teaching performance in Yemeni public schools. Front. Educ. 9:1413607. doi: 10.3389/feduc.2024.1413607
Ateeq, A., Abdulrazaq, A. Y., Alzoraiki, M., Milhem, M., and Al Astal, A. (2024a). “Assessing the influence of work-related pressure on employee performance: a case study of solidarity insurance Company in the Kingdom of Bahrain” in The AI revolution: driving business innovation and research: Volume 1 (Switzerland: Springer), 331–338.
Ateeq, A., Alaghbari, M. A., Al-refaei, A. A.-A., and Ahmed, A. Y. (2024b). Sustainable solutions: the impact of green technologies in university operations. Paper presented at the 2024 ASU international conference in emerging technologies for sustainability and intelligent systems (ICETSIS).
Ateeq, A. A., Ali, R., Milhem, M., Alzoraiki, M., Al Meer, S., and Ajlan, N. A. M. (2024). “The impact of online learning on students’ outcomes at Gulf University in the Kingdom of Bahrain” in Information and communication technology in technical and vocational education and training for sustainable and equal opportunity: business governance and digitalization of business education (Switzerland: Springer), 13–25.
Ateeq, A., Al-Refaei, A. A.-A., Alzoraiki, M., Milhem, M., Al-Tahitah, A. N., and Ibrahim, A. (2024c). Sustaining organizational outcomes in manufacturing firms: the role of HRM and occupational health and safety. Sustain. For. 16:1035. doi: 10.3390/su16031035
Ateeq, A., Alzoraiki, M., Milhem, M., and Al-Absy, M. (2023). Impact of employee loyalty on job performance: mediating role of job satisfaction on the example of Zain company, Bahrain. Management 21, 470–481.
Ateeq, A., Ayyash, M. M., Milhem, M., Alzoraiki, M., and Alzaghal, Q. K. (2024d). From textbooks to Chatbots: the integration of ChatGPT in modern university pedagogy. J. Theor. Appl. Inf. Technol. 102.
Ateeq, A., Ebrahim, S., and Al-Ghatam, R. (2022). The impact of stress and its influencing factors among dentists during the COVID-19 pandemic in Kingdom of Bahrain. F1000Research 11:930. doi: 10.12688/f1000research.110841.1
Baidoo-Anu, D., and Owusu Ansah, L. (2023). Education in the era of generative artificial intelligence (AI): understanding the potential benefits of ChatGPT in promoting teaching and learning. J. AI 7, 52–62. doi: 10.61969/jai.1337500
Bombaerts, G., Anderson, J., Dennis, M., Gerola, A., Frank, L., Hannes, T., et al. (2023). Attention as practice: Buddhist ethics responses to persuasive technologies. Glob. Philos. 33:25. doi: 10.1007/s10516-023-09680-4
Bühler, M. M., Jelinek, T., and Nübel, K. (2022). Training and preparing tomorrow’s workforce for the fourth industrial revolution. Educ. Sci. 12:782. doi: 10.3390/educsci12110782
Chan, C. K. Y. (2023). A comprehensive AI policy education framework for university teaching and learning. Int. J. Educ. Technol. High. Educ. 20, 1–25. doi: 10.1186/s41239-023-00408-3
Chaudhry, I. S., Sarwary, S. A. M., El Refae, G. A., and Chabchoub, H. (2023). Time to revisit existing student’s performance evaluation approach in higher education sector in a new era of ChatGPT—a case study. Cogent Educ. 10:2210461. doi: 10.1080/2331186X.2023.2210461
Cheah, C. S. (2020). Factors contributing to the difficulties in teaching and learning of computer programming: a literature review. Contemp. Educ. Technol. 12:ep272. doi: 10.30935/cedtech/8247
Chiang, F. K., Zhu, D., and Yu, W. (2022). A systematic review of academic dishonesty in online learning environments. J. Comput. Assist. Learn. 38, 907–928. doi: 10.1111/jcal.12656
Currie, G. M. (2023). Academic integrity and artificial intelligence: Is ChatGPT hype, hero or heresy? Semin. Nucl. Med. 53, 719–730. doi: 10.1053/j.semnuclmed.2023.04.008
Dhara, S., Chatterjee, S., Chaudhuri, R., Goswami, A., and Ghosh, S. K. (2022). “Artificial intelligence in assessment of” in Artificial intelligence in higher education: a practical approach.
Dwivedi, Y. K., Kshetri, N., Hughes, L., Slade, E. L., Jeyaraj, A., Kar, A. K., et al. (2023). “So what if ChatGPT wrote it?” Multidisciplinary perspectives on opportunities, challenges and implications of generative conversational AI for research, practice and policy. Int. J. Inf. Manag. 71:102642. doi: 10.1016/j.ijinfomgt.2023.102642
Ellison, A. M., and Patel, M. V. (2022). Success in navigating your student research experience: moving forward in STEMM : Springer Nature.
Faruqe, F. (2023). ATIAS: a model for AI technology acceptance, combining the user's AI trust and the intention to use AI systems : The George Washington University.
Figoli, F. A., Mattioli, F., and Rampino, L. (2022). Artificial intelligence in the design process: the impact on creativity and team collaboration : FrancoAngeli.
Fudge, A., Ulpen, T., Bilic, S., Picard, M., and Carter, C. (2022). Does an educative approach work? A reflective case study of how two Australian higher education enabling programs support students and staff uphold a responsible culture of academic integrity. Int. J. Educ. Integr. 18, 1–20. doi: 10.1007/s40979-021-00099-1
Gambhir, M., Broad, K., Evans, M., and Gaskell, J. (2008). Characterizing initial teacher education in Canada: Themes and issues.
Ghavifekr, S., and Rosdy, W. A. W. (2015). Teaching and learning with technology: effectiveness of ICT integration in schools. Int. J. Res. Educ. Sci. 1, 175–191. doi: 10.21890/ijres.23596
Giacalone, M., Panarello, D., and Mattera, R. (2018). Multicollinearity in regression: an efficiency comparison between L p-norm and least squares estimators. Qual. Quant. 52, 1831–1859. doi: 10.1007/s11135-017-0571-y
Habibi, A., Yaakob, M. F. M., and Al-Adwan, A. S. (2023). M-learning management system use during COVID-19. Inf. Dev. 39, 123–135. doi: 10.1177/02666669211035473
Habtoor, N. H., and Ali, E. S. (2022). Human side of total quality management: a critical review. Int. J. Intell. Hum. Resour. Manag. 3, 27–32. doi: 10.46988/IJIHRM.03.02.2022.004
Hamman, J. J. (2022). Pastoral virtues for artificial intelligence: Care and the algorithms that guide our lives : Rowman & Littlefield.
Hoffmann, J., De France, K., Seibyl, J., Orleck-Jetter, R., Gualda, R. C., and Brackett, M. A. (2023). The role of originality, distancing, and tentative language in effective cognitive reappraisal among adolescents. Think. Skills Creat. 49:101377. doi: 10.1016/j.tsc.2023.101377
Howard-Gosse, A., Bergey, B. W., and Deacon, S. H. (2023). The reading challenges, strategies, and habits of university students with a history of reading difficulties and their relations to academic achievement. J. Learn. Disabil. 57, 91–105.
Ifelebuegu, A. (2023). Rethinking online assessment strategies: authenticity versus AI chatbot intervention. J. Appl. Learn. Teach. 6.
Javaid, M., Haleem, A., Singh, R. P., Khan, S., and Khan, I. H. (2023). Unlocking the opportunities through ChatGPT tool towards ameliorating the education system. BenchCouncil Trans. Benchmarks Stand. Eval. 3:100115. doi: 10.1016/j.tbench.2023.100115
Kalnins, A. (2022). When does multicollinearity bias coefficients and cause type 1 errors? A reconciliation of Lindner, Puck, and Verbeke (2020) with Kalnins (2018). J. Int. Bus. Stud. 53, 1536–1548. doi: 10.1057/s41267-022-00531-9
Kaltenboeck, M., Choukri, S. B., Lieske, C., Lynn, T., Rigau, G., Heuschkel, M., et al. (2022). Project European language equality (ELE) Grant agreement no. LC-01641480–101018166 ELE coordinator Prof. Dr. Andy way (DCU) co-coordinator prof. Dr. Georg Rehm (DFKI) start date, duration 01-01-2021, 18 months.
Kasneci, E., Seßler, K., Küchemann, S., Bannert, M., Dementieva, D., Fischer, F., et al. (2023). ChatGPT for good? On opportunities and challenges of large language models for education. Learn. Individ. Differ. 103:102274. doi: 10.1016/j.lindif.2023.102274
Kenwright, B. (2023). Exploring the power of creative AI tools and game-based methodologies for interactive web-based programming. arXiv preprint arXiv:2308.11649.
Khosravi, H., Shum, S. B., Chen, G., Conati, C., Tsai, Y.-S., Kay, J., et al. (2022). Explainable artificial intelligence in education. Comput. Educ. Artif. Intell. 3:100074.
Lin, C.-C., Huang, A. Y., and Yang, S. J. (2023). A review of AI-driven conversational chatbots implementation methodologies and challenges (1999–2022). Sustain. For. 15:4012. doi: 10.3390/su15054012
Malinka, K., Peresíni, M., Firc, A., Hujnák, O., and Janus, F. (2023). On the educational impact of ChatGPT: is artificial intelligence ready to obtain a university degree? Paper presented at the proceedings of the 2023 conference on innovation and Technology in Computer Science Education V. 1.
Masters, G. N. (2023). Building a world-class learning system: Insights from some top-performing school systems.
Mattioli, F., Rampino, L., and Figoli, F. A. (2022). “Artificial intelligence in the design process: the impact on creativity and team collaboration” in Artificial Intelligence in the design process, 1–105.
McCabe, D. L., Butterfield, K. D., and Trevino, L. K. (2006). Academic dishonesty in graduate business programs: prevalence, causes, and proposed action. Acad. Manag. Learn. Edu. 5, 294–305. doi: 10.5465/amle.2006.22697018
Mede, N. G., Schäfer, M. S., Metag, J., and Klinger, K. (2022). Who supports science-related populism? A nationally representative survey on the prevalence and explanatory factors of populist attitudes toward science in Switzerland. PLoS One 17:e0271204. doi: 10.1371/journal.pone.0271204
Mhlanga, D. (2023). Open AI in education, the responsible and ethical use of ChatGPT towards lifelong learning. Education, the responsible and ethical use of ChatGPT towards lifelong learning (February 11, 2023).
Milhem, M., Ateeq, A., Ali, R., and Alzoraiki, M. (2024). “Corporate social responsibility: a multidimensional approach to sustainable growth and community engagement—the case of Almarai Company” in The AI revolution: driving business innovation and research: Volume 1 (Switzerland: Springer), 143–151.
Milhem, M., Ayyash, M. M., Ateeq, A., Alzaghal, Q., and Alzoraiki, M. (2024). Emotional intelligence in leadership: Its role in fostering employee engagement in Palestine’s ICT sector. Probl. Perspect. Manag. 22, 338–352. doi: 10.21511/ppm.22(1).2024.28
Milhem, M. M., Muda, H., and Ahmed, K. (2019). The effect of perceived transactional leadership style on employee engagement in Palestinian ICT industry. J. Adv. Res. Bus. Manag. Stud. 14, 42–51.
Milhem, M., Tahayna, A. M. A., Ayyash, M. M., Ali, A. A. A., Al-Zoraiki, M., and Alkadash, T. (2024). “The mediating role of organizational culture in the relationship between workforce diversity and turnover intention among employees in Palestine” in Intelligent systems, business, and innovation research (Switzerland: Springer), 807–816.
Muscatello, V. S. (2023). Promoting inclusivity with student-centered instructional approaches: evaluation of a course redesign of undergraduate research methods in psychology. Los Angeles: University of California.
Narayanan, A., and Kapoor, S. (2024). AI Snake oil: what artificial intelligence can do, what it can’t, and how to tell the difference. Princeton University Press.
Odei-Tettey, K., Ayivor, A. E. A., and Dodzi, E. (2023). Mudling through intersectionality theory for leadership futures: pathways toward policies for reinventing gender spaces for educational leadership in two male schools in Ghana. Br. J. Educ. 11, 28–60. doi: 10.37745/bje.2013/vol11n72860
Oliveira, T., Nada, C., and Magalhães, A. (2023). Academic migration and epistemological value: exploring the experience of migrant academics in Portugal. Educ. Sci. 13:720. doi: 10.3390/educsci13070720
Owan, V. J., Abang, K. B., Idika, D. O., Etta, E. O., and Bassey, B. A. (2023). Exploring the potential of artificial intelligence tools in educational measurement and assessment. Eurasia J. Math. Sci. Technol. Educ. 19:em2307. doi: 10.29333/ejmste/13428
Pehlivan, D., Aras, S., Glaze, D. G., Ak, M., Suter, B., and Motil, K. J. (2023). Development and validation of parent-reported gastrointestinal health scale in MECP2 duplication syndrome. Springer. Available at: https://link.springer.com/article/10.1186/s13023-024-03022-2#citeas
Perkins, M. (2023). Academic integrity considerations of AI large language models in the post-pandemic era: ChatGPT and beyond. J. Univ. Teach. Learn. Pract. 20:07. doi: 10.53761/1.20.02.07
Perkins, M., and Roe, J. (2023). Decoding academic integrity policies: a corpus linguistics investigation of AI and other technological threats. Springer. Available at: https://link.springer.com/article/10.1057/s41307-023-00323-2
Poitras Pratt, Y., and Gladue, K. (2022). “Re-defining academic integrity: Embracing indigenous truths” in Academic integrity in Canada: An enduring and essential challenge (Cham: Springer International Publishing), 103–123.
Qaid, E. H., Ateeq, A. A., Abro, Z., Milhem, M., Alzoraiki, M., Alkadash, T. M., et al. (2024). “Conceptual framework in attitude factors affecting Yemeni university lecturers’ adoption of E-government” in Information and communication technology in technical and vocational education and training for sustainable and equal opportunity: business governance and digitalization of business education (Springer), 345–358. Available at: https://link.springer.com/chapter/10.1007/978-981-99-7798-7_29
Reardon, S. F. (2011). The widening academic achievement gap between the rich and the poor: new evidence and possible explanations. Whither Opportunity 1, 91–116.
Roy, S. K. (2023). YouTube's influential factors for academic achievement: a two-stage approach. Telemat. Inform. Rep. 10:100060. doi: 10.1016/j.teler.2023.100060
Rudolph, J., Tan, S., and Tan, S. (2023). ChatGPT: bullshit spewer or the end of traditional assessments in higher education? J. Appl. Learn. Teach. 6. doi: 10.37074/jalt.2023.6.1.9
Sarstedt, M., Ringle, C. M., and Hair, J. F. (2021). “Partial least squares structural equation modeling” in Handbook of market research (Springer), 587–632. Available at: https://link.springer.com/referenceworkentry/10.1007/978-3-319-57413-4_15
Skavronskaya, L., Hadinejad, A., and Cotterell, D. (2023). Reversing the threat of artificial intelligence to opportunity: a discussion of ChatGPT in tourism education. J. Teach. Travel Tour. 23, 253–258. doi: 10.1080/15313220.2023.2196658
Sok, S., and Heng, K. (2023, 2023). ChatGPT for education and research: a review of benefits and risks. Cambodian J. Educ. Res. 3, 110–121.
Song, Z., Shah, N., Guo, J., and Zhu, Q. (2022). Applying project-based learning to improve computer networks courses: an experience report. Paper presented at the 2022 IEEE global engineering education conference (EDUCON).
Sullivan, W. M. (2018). Work and integrity: the crisis and promise of professionalism in America. J. Am. Coll. Dent. 85.
Surahman, E., and Wang, T. H. (2022). Academic dishonesty and trustworthy assessment in online learning: a systematic literature review. J. Comput. Assist. Learn. 38, 1535–1553. doi: 10.1111/jcal.12708
Susilawati, E., Lubis, H., Kesuma, S., Pratama, I., and Khaira, I. (2023). Exploring the antecedents of student academic integrity: the impact of using digital technology automated short essay scoring (ASES) assessment models in learning. Eurasian J. Educ. Res. 103, 125–144.
Tucker, L. G. (2024). Applications of AI in primary and secondary education : Universitat Politècnica de Catalunya. Available at: https://upcommons.upc.edu/handle/2117/413223
Uunona, G. N., and Goosen, L. (2023). “Leveraging ethical standards in artificial intelligence technologies: a guideline for responsible teaching and learning applications” in Handbook of research on instructional technologies in health education and allied disciplines (IGI Global), 310–330.
Vandenberghe, B., Gerling, K., Geurts, L., and Vanden Abeele, V. (2022). Maker technology and the promise of empowerment in a Flemish School for Disabled Children. Paper presented at the proceedings of the 2022 CHI conference on human factors in computing systems.
Viberg, O., Hatakka, M., Bälter, O., and Mavroudi, A. (2018). The current landscape of learning analytics in higher education. Comput. Hum. Behav. 89, 98–110. doi: 10.1016/j.chb.2018.07.027
Keywords: Artificial Intelligence, academic integrity, Educational Impact, Policy and Ethics, Pedagogical Implications, Academic Outcomes
Citation: Ateeq A, Alzoraiki M, Milhem M and Ateeq RA (2024) Artificial intelligence in education: implications for academic integrity and the shift toward holistic assessment. Front. Educ. 9:1470979. doi: 10.3389/feduc.2024.1470979
Edited by:
Raman Grover, Consultant, Vancouver, CanadaReviewed by:
Crystal Chambers, East Carolina University, United StatesVicki S. Napper, Weber State University, United States
Copyright © 2024 Ateeq, Alzoraiki, Milhem and Ateeq. This is an open-access article distributed under the terms of the Creative Commons Attribution License (CC BY). The use, distribution or reproduction in other forums is permitted, provided the original author(s) and the copyright owner(s) are credited and that the original publication in this journal is cited, in accordance with accepted academic practice. No use, distribution or reproduction is permitted which does not comply with these terms.
*Correspondence: Ali Ateeq, ZHIuYWxpLmF0ZWVxQGd1bGZ1bml2ZXJzaXR5LmVkdS5iaA==
†ORCID: Ali Ateeq, https://orcid.org/0000-0002-8138-7766