- 1School of Arts and Creative Technologies, University of Bolton, Bolton, United Kingdom
- 2Information School, University of Sheffield, Sheffield, United Kingdom
- 3Department of Respiratory Care, Prince Sultan Military College of Health Sciences, Dammam, Saudi Arabia
- 4Division of Medicine, University College London, London, United Kingdom
Background: Generative artificial intelligence (Gen-AI) has emerged as a transformative tool in research and education. However, there is a mixed perception about its use. This study assessed the use, perception, prospect, and challenges of Gen-AI use in higher education.
Methods: This is a prospective, cross-sectional survey of university students in the United Kingdom (UK) distributed online between January and April 2024. Demography of participants and their perception of Gen-AI and other AI tools were collected and statistically analyzed to assess the difference in perception between various subgroups.
Results: A total of 136 students responded to the survey of which 59% (80) were male. The majority were aware of Gen-AI and other AI use in academia (61%) with 52% having personal experience of the tools. Grammar correction and idea generation were the two most common tasks of use, with 37% being regular users. Fifty-six percent of respondents agreed that AI gives an academic edge with 40% holding a positive overall perception about the use in academia. Comparatively, there was a statistically significant difference in overall perception between different age ranges (I2 = 27.39; p = 0.002) and levels of education (I2 = 20.07; p < 0.001). Also, 83% of students believe AI use will increase in academia with over half agreeing it should be integrated into learning. Plagiarism (33%), privacy issues (14%), and lack of clarity by the university (13%) remain the top concerns regarding the use of Gen-AI and other AI tools in academia.
Conclusion: Gen-AI and other AI tools are being used and their use will continue to grow in higher education. While current use is challenging due mainly to plagiarism fear and lack of clarity by the university, most users believe AI should be integrated into the university curriculum.
1 Introduction
Generative artificial intelligence (Gen-AI) has emerged as a transformative force in higher education, with its adoption steadily rising, including in the United Kingdom (Education, 2024). This trend has sparked a range of perceptions and opinions, ranging from optimism regarding its potential to revolutionize the teaching and learning process to concerns about its implications for academic integrity and the traditional roles of educators (Farrelly and Baker, 2023). The integration of Gen-AI into higher education settings has ignited debates surrounding issues such as authorship and the impact on academic standards (Lim et al., 2023).
While some view Gen-AI as a promising tool capable of improving educational experiences through the creation of high-quality learning materials, others express concerns about its impact on the autonomy and pedagogical expertise of educators (Jain and Raghuram, 2024). The rapid advancement of Gen-AI technologies has heightened these discussions, prompting educators, policymakers, and scholars to grapple with its implications for the future of education (İpek et al., 2023; Yusuf et al., 2024).
Lim et al. define Generative Artificial Intelligence as a technology that uses deep learning models to generate human-like content in response to complex and various prompts (Lim et al., 2023). This capability has rendered Gen-AI ubiquitous across various domains, including education, law, policymaking, and content creation (Yusuf and Tambuwal, 2018). Its ability to emulate human-like responses has facilitated its integration into educational practices, offering unique opportunities for personalized learning experiences, content generation, and management (Guan, 2022; Liu et al., 2022; Mao et al., 2024).
Understanding the perception of Gen-AI use in UK higher education is crucial for identifying potential challenges and opportunities in the integration of AI technology in educational settings. As Gen-AI continues to evolve at a rapid pace, it holds the promise of driving innovation and enhancing educational outcomes (Jain and Raghuram, 2024). However, alongside its potential benefits, the widespread adoption of Gen-AI presents a host of challenges that must be addressed through effective policies and measures (Farrelly and Baker, 2023). Failure to navigate these challenges could undermine the integrity of academic practices and exacerbate existing disparities within higher education (Farrelly and Baker, 2023; Mao et al., 2024).
In the coming years, the trajectory of Gen-AI in higher education will be shaped by ongoing debates and efforts to harness its potential while mitigating associated risks (Banh and Strobel, 2023). Understanding the multifaceted nature of perceptions surrounding Gen-AI is essential for informing strategic decisions and ensuring its responsible integration into educational settings (Wu et al., 2020).
Previous studies have delved into the application of artificial intelligence (AI) within educational contexts; however, there remains a notable gap in the literature concerning the specific perception and adoption of Gen-AI within the landscape of UK higher education. While some research has explored perceptions of Gen-AI in education settings, such investigations have primarily been conducted in various global contexts such as Hong Kong and India (Chan and Hu, 2023), leaving a significant gap in understanding the complex dynamics within the UK higher education system.
To bridge this gap in knowledge, the present study aims to investigate the perception of Gen-AI use among students within a UK higher education institution (University of Bolton, Greater Manchester; UK Northwest region). By concentrating on a specific institution within the UK and its unique academic environment, this study aims to provide insights that are both contextually relevant and generalizable which can inform the development of policies and strategies for the effective integration of AI technology in UK higher education. Thus, this study aims to answer the question about whether higher education students in the UK have a positive or negative perception of the use of gen-AI and AI for academic purpose and their expectation regarding integration into learning curriculum.
2 Literature review
2.1 Brief history and trend of AI
AI describes the ability of computer systems to acquire, synthesize data to adapt, self-correct, and perform complex tasks in a human-like mode (Popenici and Kerr, 2017). Indeed, AI has metamorphosed from the early idea of Turing about the integration of intelligent thinking and reasoning into machines (Turing, 1936; Turing, 2009) along with the advancement of technologies with which it can be accessed.
In terms of research output on the use of AI, a recent study showed that AI-related publications rose significantly (>7 times) from the early part of the millennium to over half a million as of 2018. Indeed, this is accompanied by increasing global investment in various field of AI use with increasing demand and potential for usage in all aspect of human life. Further, AI-related research continues to be one of the most funded in academia especially related to the field of computer vision and general machine learning and artificial intelligence (Gardner et al., 2022; Rahkovsky et al., 2021). According to the Artificial Intelligence Index Report 2019, specialization in AI continues to be the most popular among computer science students especially in north America (Perrault et al., 2019). Put together, the trend in the development and integration of AI tools may continue to grow in line with the increasing demand across various fields (Shoham et al., 2018).
Indeed, in recent years, the use of AI has spread across all aspect of the human life including in farming, engineering, healthcare, and most importantly in education (Chen et al., 2022; Chui et al., 2018; Esmaeilzadeh, 2020; Harry and Sayudin, 2023). The use of AI in higher education has gained traction in the past decades, peaking in some countries during the COVID-19 pandemic with the need for digital remote education to reduce the impact of lockdowns around the world (Haris and Al-Maadeed, 2021; Nawaz et al., 2020; Pantelimon et al., 2021).
2.2 How AI is used in education
AI use in higher education have seen overall increase in various aspects including in the improvement of students experience through
i. Targeted and personalized learning: this involves the use of AI-generated contents to modify teaching activities tailored to individual student’s psychological requirements and contextual factors with the possibility of improving knowledge retention, engagement, and boosting creativity (Pataranutaporn et al., 2021). For instance, using virtual instructors (such as anime characters), an increase in students enthusiasm and engagement was observed by a tutor (Edwards, 2020). Aside creating virtual tutor as avatar, other studies have shown that using virtual characters and avatars as a virtual embodiment of students themselves can help personalize learning experience and improve learning outcomes, motivation, and creativity among others (Falloon, 2010; Fitton et al., 2023; Guegan et al., 2016; Hong et al., 2019; Hudson and Hurter, 2016; Ratan et al., 2022).
ii. Supporting students’ wellbeing: Other ways AI have been used in education is as virtual wellbeing counselor. Indeed, mental health apps using AI have received significant attention recently and have been shown to be effective in the treatment of various mental health problems including depression and anxiety (Crosby and Bonnington, 2020; Wu et al., 2021), post-traumatic stress disorder (Goreis et al., 2020), Attention deficit hyperactivity disorder (Moëll et al., 2015), Obsessive-compulsive disorder (Van Ameringen et al., 2017) among others. However, several limitations persist regarding conformation to clinical guideline and lack of government and standard oversight (Radovic et al., 2016; Torous et al., 2019; Torous and Roberts, 2017).
Also, AI have been used by faculty staffs in various forms including for
i. Grading and assessment: traditional grading system for the assessment of students’ knowledge retention serves as the benchmark in higher education. Although, useful it suffers the limitation of efficiency and scalability especially in nuanced modern assessment mode such as open-book assessment (Dimari et al., 2024). This is further complicated in situations of high students, number which may result in tutors going for easier to assess but inappropriate assessment mode (Fagbohun et al., 2024). In this situation, AI-based grading system provides a viable alternative which may ensure quality, accuracy, efficiency and fairness (Dimari et al., 2024). Indeed, various studies have reported both the feasibility and promises of AI-integration for automated grading system in higher education (Gao et al., 2024; Hao et al., 2024; Vittorini et al., 2021).
ii. Predictive analytics: for targeted approach to improve students’ engagement and dropout and underperformance. Predictive analysis algorithms are very well applied in higher education institutions using student-related data (past or real-time) to predict academic outcomes and as early warning systems for potential risks of students’ course failure or dropout (Gandomi and Haider, 2015). Generally, insights generated through predictive analytics are useful in the potential design of timely targeted remedial interventions to increase positive learning outcomes (Liz-Domínguez et al., 2019). Various predictive analytical AI or machine learning models are often used including support vector machine, decision tree, K-Nearest Neighbor linear or logistic regression among other usually with the best model chosen based on relative performance (Kumar et al., 2018; Livieris et al., 2016; Oppong, 2023; Sghir et al., 2022; Walia et al., 2020).
iii. For general administration: administrative responsibility is a substantial part of academic responsibilities which in many cases have been reported to have detrimental effect on research output as well as teaching efficiency and consequently students experience (Davidovitch and Cohen, 2024; García-Gallego et al., 2015; Kim, 2019; Martin Iii, 2021; Martin, 2022). Thus, the integration of AI for automated administrative processes could and have been shown to reduce the workload of higher education staffs, freeing up more time for improved teaching, students’ engagement while boosting research output and saving time and resources (Ahmad et al., 2022; Aldosari, 2020; Crompton and Song, 2021; Saaida, 2023). Specific areas where AI has been used in administrative responsibilities include in managing student admissions, financial aid processing, library service, budgeting, and counseling (Dennis, 2018; Zawacki-Richter et al., 2019). Also, studies have shown the potential of Ai in other tedious administrative tasks including human resources (staff recruitment) (Balcioğlu and Artar, 2024; Budhwar et al., 2023; Gong et al., 2022).
3 Methods
3.1 Study design, and ethics
This is a cross-sectional prospective survey aimed to assess the prevalence and perception of gen-AI use among higher education (university) students in the UK. This study was registered, and ethics approval was acquired from the University of Bolton Ethics Committee (AUTOYEHP00207). Data was collected between January and April 2024. The survey was designed electronically using Microsoft Forms and consent was asked following a detailed description of the aim of the survey. Thus, only participants who consented to participate in the survey did so. The response was collected completely anonymously in respect of the GDPR (The General Data Protection Regulation) (Addis and Kutar, 2018). The study was conducted strictly following the Declaration of Helsinki regarding human participants (Shrestha and Dunn, 2020).
3.2 Data collection
All current students at the University of Bolton with active student email addresses were eligible for this study. Generally, this includes adults of 18 years and above irrespective of country of origin. Generative AI use was defined as the utilization of any form of artificial intelligence tool including large language modeling tools (e.g., ChatGPT), grammar correction tools (e.g., Grammarly), and other AI-based tools in any part of academic activities. The survey question included a brief introduction describing the modes of AI included in the definition specifically with reference to those tools used to augment or assist academic activities and learning including ChatGPT, Grammarly, Plagiarisms detection tools. The survey was sent to the central administrative office at the University of Bolton and sent out to all active students’ emails. The survey was resent were sent later and links were also shared during classes to increase engagement with the survey. All active students at the university have a working email which is the primary contact and employed in this study to ensure it reaches the students.
3.3 Survey design and distribution
The survey design involves a thorough literature review on how previous studies assessing perception of students in higher education and includes 7 different sections. Section 1 provides general information and consent to participate while section 2 collects demographic information about the participants. Sections 3 and 4, respectively, measured the general understanding and perceived impact of gen-AI and AI in an academic context. The perception and challenges faced in the use of AI are covered in section 5, while section 6 surveys participants’ expectations and projections of AI use in academia. In general, parts of section 3, 4, 5, and 6 measuring the understanding, impact, perception, expectation, and prospect of AI involves the use of 5-point Likert scale with highest score attributed higher factors visa vice. Sections 7 provides participants with the opportunity to give general thoughts and feedback on the subject. A copy of the survey question is provided as Supplementary Table S1. The survey was distributed to the students via various platforms including email, and social platforms.
3.4 Power calculation
We performed a power calculation to estimate the number of participants needed to draw a significant conclusion with a confidence interval of 95% and a margin of error of 8%. Giving the population of students at the University of Bolton is about 11,000 in 2024 and considering that the survey link was opened by approximately 10% of students, the minimum sample size required for this study to be generalizable was calculated to be 137.
3.5 Statistical analysis
Respondents’ characteristics were summarized using descriptive statistics and presented as counts and percentages. To assess the difference in overall perception of Gen-AI across gender, age, and education level, Pearson Chi-square analysis was performed on the coded 5-point Likert scales of participants’ responses. Statistical analysis was performed using SPSS Statistics 26 (IBM Corp., Armonk, NY). A 2-tailed p-value of < 0.05 was defined as significant in all statistical analyses.
4 Results
4.1 Characteristics of participants
A total of 136 participants consented and responded to this survey out of a total of 140 that confirmed receipt. This is equivalent to a response rate of 97%. Of the 136 responders, 59% (80) identified as male. Further, most responders were between the ages of 18 and 34 (~77%) and studying an undergraduate at the undergraduate level (57%). In general, students in the fields of Engineering and Technology as well as Arts and Design were the most represented (42 and 25% respectively; Table 1).
4.2 Awareness and use of gen-AI
The awareness of participants about the use of gen-AI for academic purposes was measured in this survey. In general, 61% (83/136) of the participants were either highly or moderately familiar with the use of gen-AI for general purposes, with 22% (30/136) and 14% (19/136) being somewhat or slightly familiar with the tools. Also, around 94% (128/136) of the participants were aware of the use of gen-AI for academic purposes, while 52% (71/136) have personally used gen-AI for academic tasks. Specifically, the four most popular tasks for which AI tools have been generally used among respondents who declared using these tools (71/136), were grammar corrections (56%; 40/71), idea generation (55%; 39/71), answering questions (41%; 29/71), paraphrasing and summarizing (37%; 26/71; Figure 1). Indeed, 37% (26/71) of the respondents who answered yes to the usage of Gen-AI and other AI tools (71 participants) reported regular (always or often) use of AI tools, while 61% (43/71) sometimes or rarely use the tools (Table 2).
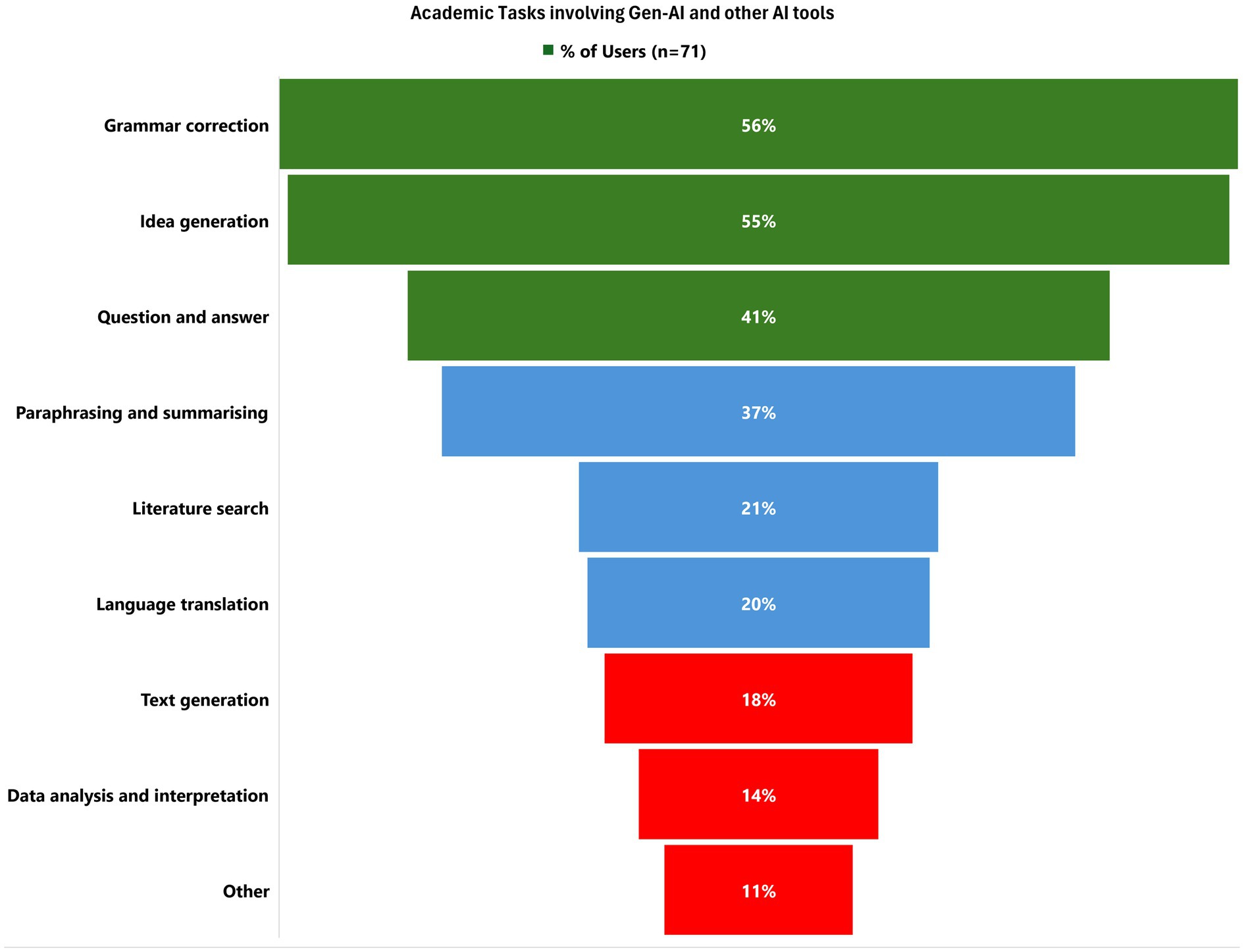
Figure 1. Academic tasks for which Gen-AI (Generative Artificial Intelligence) and AI (Artificial Intelligence) have been used by participants.
4.3 Perception of gen-AI and other AI use for academic purposes
Table 2 shows the respondents’ perception and expectations (prospect) regarding the use of Gen-AI. Overall, 56% (76/136) of the respondents perceived that Gen-AI offers an edge when used for academic purposes with only 15% (20/136) disagreeing with this belief. Also, most respondents (40%) have positive perceptions about the use of Gen-AI and other AI tools in academia with 21% having a negative overall perception of these tools. In terms of overall perception, there was no significant between participants based on gender (I2 = 4.84; p = 0.304). However, there was a significant difference in perceptions of the Gen-AI and other AI tools across different age ranges (I2 = 27.39; p = 0.002; Figure 2) and education level (I2 = 20.07; p < 0.001; Figure 3). Finally, when asked to describe AI in a single word, the overall association was positive (Supplementary Figure S1) with majority of participants using words such as “Innovative,” “Helpful,” “Artificial Intelligence,” and “Good,” while students also used words like “Concerning.”
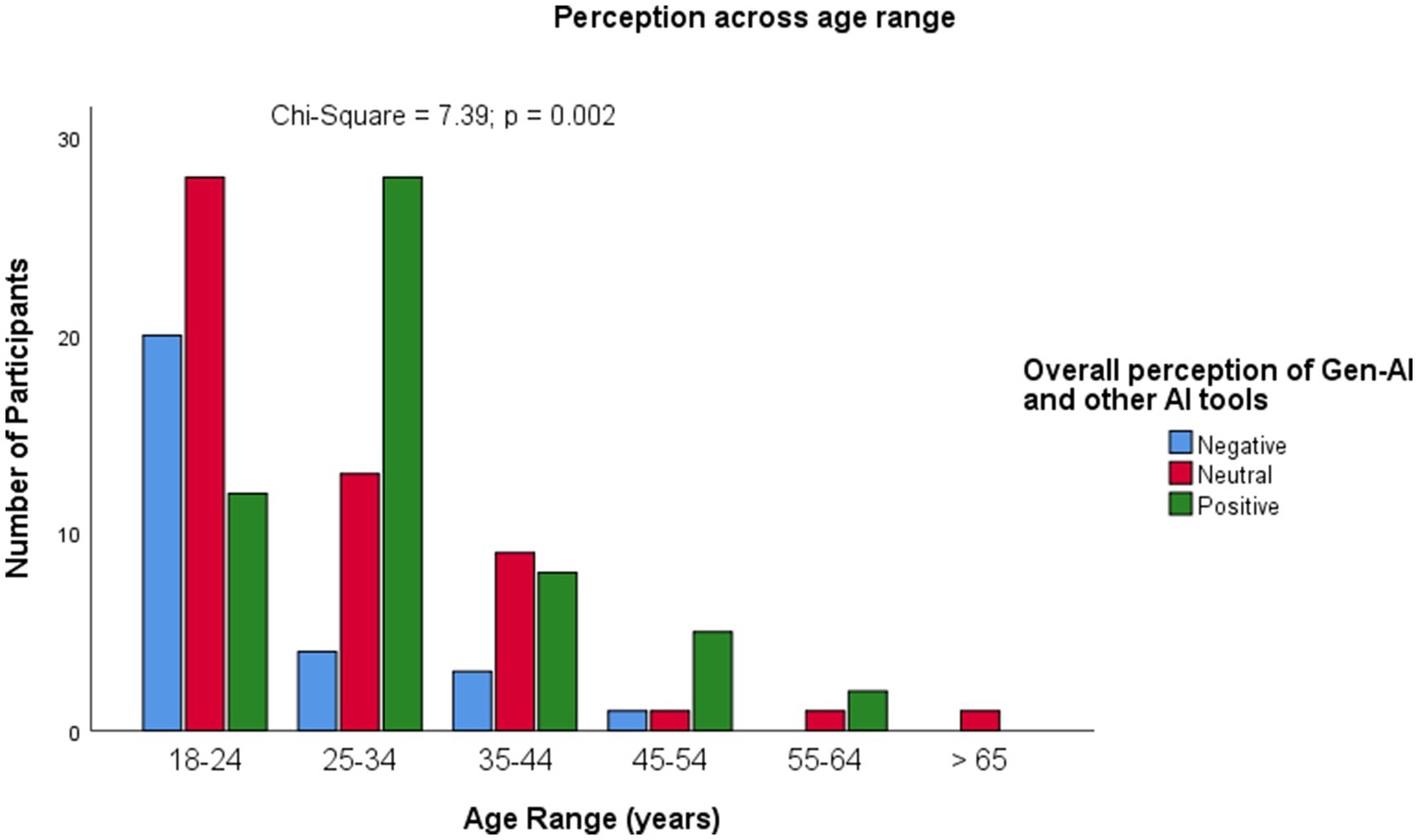
Figure 2. Overall perception of Gen-AI and other AI tools across different age ranges. Gen-AI, Generative Artificial Intelligence.
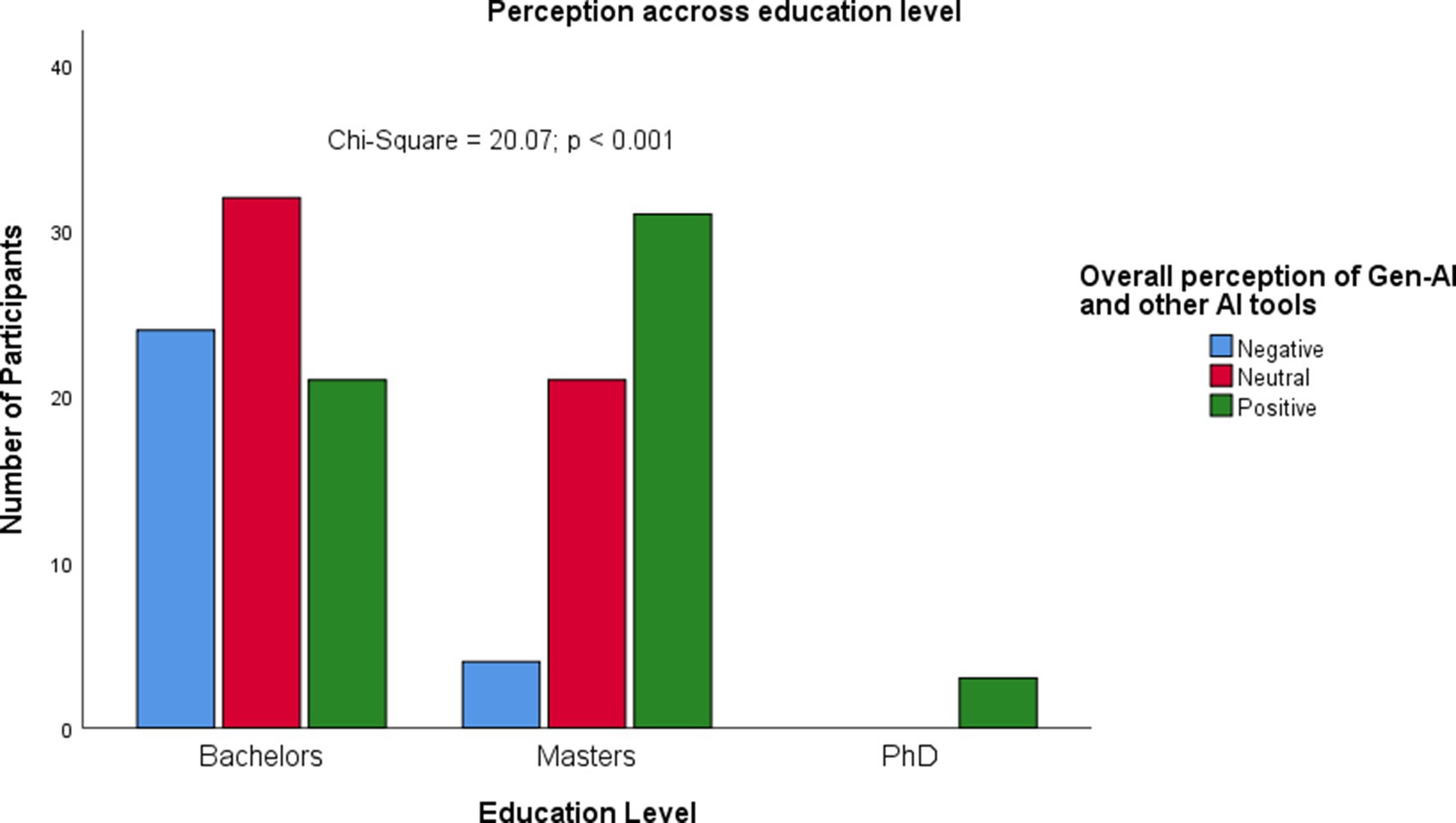
Figure 3. Overall perception of Gen-AI and other AI tools across different education levels. Gen-AI, Generative Artificial Intelligence.
4.4 Prospect and limitation of gen-AI and other AI use for academic purposes
Regarding the use of Gen-AI and other AI tools, most respondents (83%; 112/136) agreed that these tools will be used increasingly for academic purposes in the future. Also, 47% (65/136) of respondents agreed that the use of Gen-AI and other AI tools should be integrated into the university curriculum with 19% disagreeing. In terms of challenges encountered while using AI tools, the most common limitations are linked with plagiarism concerns (33%), followed by data regulatory/privacy issues (14%) and lack of clarity from the university (13%) (Table 3).
5 Discussion
In this cross-sectional prospective survey of higher education students in the UK, we have assessed the perception, expectations, challenges, and prospects of AI use for academic purposes. Our result shows an overall high awareness of Gen-AI and other AI tools for both general and academic use with over half of the participants having a first-hand experience of the tools for their academic works including mostly grammar correction, idea generation, and answering questions. Of the participants who declared using Gen-AI or other AI tools for academic purposes, a third of them regularly use these tools. Further, our results show that more than half of the respondents believed AI use offers academic advantages with 40% having an overall positive perception of the tools (Table 4).
In terms of determinants of perception type, we compared the overall perception of AI and Gen-AI across different genders, age ranges, and levels of study. Results show that while there was no difference in perception across genders, perception regarding the use of AI significantly differed across age and study levels. Specifically, this study shows that participants between the ages of 18 and 24 have the highest proportion of negative perceptions while those between the ages of 25 and 34 years have relatively more positive outlooks regarding the use of Gen-AI or any AI tool for academic purposes. Also, students studying postgraduate degrees (Master’s or Doctoral) have relatively more positive perceptions of AI use compared with those studying a first Bachelor degree. Further, over 80% of respondents believe that AI will grow in popularity as a useful tool in academia with approximately half of participants suggesting the integration into learning curriculum.
These results are similar to previous studies assessing the awareness of AI use in schools (colleges and higher education institutions). For instance, in a survey of 116 educators from a university in the United States, authors reported that “most” of the participants were aware of AI use in higher education (heard of or used) although the proportion of participants that responded being aware of the tools were not given. Also, the authors reported an overall positive perception and sentiment around the use of AI among educators (Ghimire et al., 2024). Also, in a recent, similar study of university students (n = 1,135) in Australia, Kelly et al., reported a high overall awareness (very little knowledge, moderate, or a lot of knowledge) of AI or Gen-AI by students with less than half had used the tools. Further, there was no significant difference in awareness of Gen-AI or AI between different genders or ages although confidence in using these tools differed between the groups (based on gender and age). Intuitively, students ages over 40 were reported to be less likely to use Gen-AI and AI or be confident in using the tools compared with under 25 years old students (Kelly et al., 2023). While the authors did not assess or report the overall difference in perception between the groups, the difference in use and confidence about the use of Gen-AI and AI provides an interesting context. Specifically, this result may be linked with a relatively lower representation of older students in higher education resulting in a relatively higher and statistically significant difference. Alternatively, it could indeed reflect the age difference in AI and general technology adoption that has been reported in previous studies (Chan and Lee, 2023). Also, students at higher levels of education were more likely to have a positive perception of AI. We hypothesize that this may be due to the increased need for creativity (idea generation) as well as the larger volume of writing required in higher levels of university education compared with lower. This may correspond to an increased need to use Gen-AI or other AI tools for idea generation and grammar correction, two of the main uses that most responders in this study have reported (Figure 4).
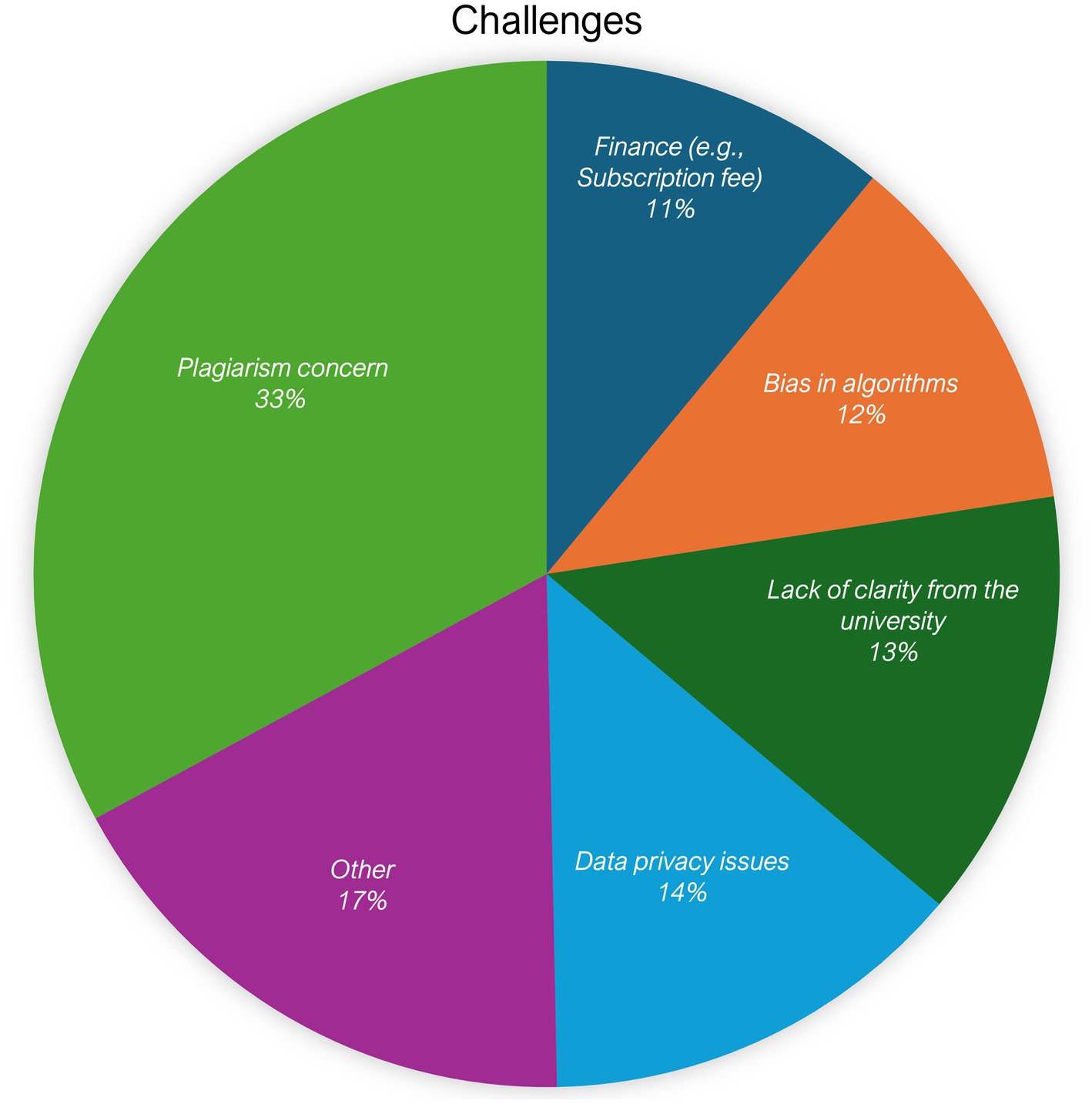
Figure 4. Challenges faced by respondents regarding the use of Gen-AI and AI tools for academic purposes.
The use of AI in higher education has been argued to have the potential to revolutionize various aspects of teaching, learning, and research with specific advantages of increasing access to information, retention of knowledge, and learning outcomes at a relatively lower cost (financial and time) (Klutka et al., 2018). Indeed, various studies have reported the successful application of AI in higher education institutions. For instance, Hannan and Liu reported the successful use of AI in improving enrolment management, students’ learning experience, and student support in higher education institutions (Hannan and Liu, 2023). Also, Jin et al. showed the usefulness of AI in supporting self-regulated online learning in higher education (Jin et al., 2023). Thus, AI may serve as a tool for the improvement of higher education practices and services if integrated well based on research and rigorous stakeholder involvement. This integration may also help in various teaching scenario where face-to-face teaching or learning is not possible. For instance, during global pandemics or cases where online teaching is the only option, AI may be used to augment learning experience (Alqahtani et al., 2022; Diwan et al., 2023; Holstein, 2019; Kim et al., 2020). However, AI also potentially threatens the traditional approach to teaching especially in higher education institutions which may also be in the best position to harness the benefits of these tools in empowering tutors and learners (Bates et al., 2020).
Responders also reported various limitations to the efficient use of AI for academic purposes. Expectedly, fear of plagiarism remains the most reported challenge faced by responders followed by data privacy issues and lack of clarity from the institution regarding the use of Gen-AI and other AI tools for academic works. This corroborates some of the concerns raised in a previous study. Specifically, educators’ main concerns surrounding the use of AI were reported to be mainly the potential for cheating (which may manifest in the form of plagiarism), equity and access as well as the potential to restrict creativity (Ghimire et al., 2024).
This study has several limitations associated with self-reporting and the design of this study is cross-sectional. Specifically, self-reporting is associated with recall bias (Althubaiti, 2016), while cross-sectional studies do not cater to changes in response that may result, for instance, from increased use of Gen-AI and AI tools by participants (Solem, 2015). Particularly, perception may become more positive or negative as participants become more aware of the tools. Another limitation is linked with the Hawthorne effect whereby participants’ behavior (response) is influenced by the fact of being studied whereby, they would have behaved or responded differently were they not involved in a study (Adair, 1984; Sedgwick and Greenwood, 2015). Finally, the sample size and the single-centered location of the study population limit the generalizability of our results. Given the diverse nature of higher education institutions in the UK and the varied demographics of students (Fitzpatrick, 2023), it is essential to examine individual perceptions across different fields to attain a comprehensive understanding of this subject. Each UK region may present unique challenges, opportunities, and attitudes toward Gen-AI integration, necessitating multi-regional investigations to capture the full spectrum of student’s perspectives. Finally, the sample size for this study is low (1 participant lower than the required amount of 137 according to the power calculation), warranting a careful interpretation of the findings in terms of generalization. While the importance of high response rate is cannot be overemphasized (Fosnacht et al., 2017), the low percentage of reach in this study is a result of the inherent difficulty associated with students’ survey which has been extensively described in literature (Dey, 1997; Nair et al., 2008; Van Mol, 2017).
Irrespective of this, the power calculation showed that the sample size is sufficient, and this study provides the basis for future, potentially larger, multicenter, longitudinal studies.
In sum, there is a high awareness of Gen-AI and other AI tools among university students in the UK some of whom use the tools for academic purposes including grammar correction, idea generation, and answering questions. Also, there is a high awareness of Gen-AI and other AI tools among students with an overall positive perception about the tools which depends on age and level of study. Also, various concerns about AI use were raised including plagiarism, data privacy, and lack of clarity by the institution. However, there is a high expectation that AI will become popular in academia and suggestion that they should be incorporated into the curriculum.
As AI becomes more and more inevitably incorporated into all aspects of society, education institutions must consider its use and provide further guidance that allows educators and learners to harness the positive aspects of these innovative tools while protecting the traditional values that make higher education institutions especially the hub of originality, creativity, and integrity.
Data availability statement
The raw data supporting the conclusions of this article will be made available by the authors, without undue reservation.
Ethics statement
The studies involving humans were approved by University of Bolton Ethics Committee (AUTOYEHP00207). The studies were conducted in accordance with the local legislation and institutional requirements. The participants provided their written informed consent to participate in this study.
Author contributions
AA: Conceptualization, Data curation, Formal analysis, Investigation, Methodology, Project administration, Resources, Software, Validation, Visualization, Writing – original draft, Writing – review & editing. JA: Investigation, Resources, Writing – original draft, Writing – review & editing. TO: Investigation, Resources, Writing – original draft, Writing – review & editing, Conceptualization, Data curation, Formal analysis, Methodology, Project administration, Software, Supervision, Validation, Visualization.
Funding
The author(s) declare that no financial support was received for the research, authorship, and/or publication of this article.
Conflict of interest
The authors declare that the research was conducted in the absence of any commercial or financial relationships that could be construed as a potential conflict of interest.
Publisher’s note
All claims expressed in this article are solely those of the authors and do not necessarily represent those of their affiliated organizations, or those of the publisher, the editors and the reviewers. Any product that may be evaluated in this article, or claim that may be made by its manufacturer, is not guaranteed or endorsed by the publisher.
Supplementary material
The Supplementary material for this article can be found online at: https://www.frontiersin.org/articles/10.3389/feduc.2024.1463208/full#supplementary-material
References
Adair, J. G. (1984). The Hawthorne effect: a reconsideration of the methodological artifact. J. Appl. Psychol. 69:334.
Addis, M. C., and Kutar, M. (2018). “The general data protection regulation (GDPR), emerging technologies and UK organisations: Awareness, implementation and readiness,” in UK academy for information systems conference proceedings 2018. 29. Available at: https://aisel.aisnet.org/ukais2018/29
Ahmad, S. F., Alam, M. M., Rahmat, M. K., Mubarik, M. S., and Hyder, S. I. (2022). Academic and administrative role of artificial intelligence in Education. Sustain. For. 14:1101. doi: 10.3390/su14031101
Aldosari, S. A. M. (2020). The future of higher education in the light of artificial intelligence transformations. Int. J. High. Educ. 9, 145–151. doi: 10.5430/ijhe.v9n3p145
Alqahtani, J. S., Aldhahir, A. M., Al Ghamdi, S. S., Aldakhil, A. M., Al-Otaibi, H. M., AlRabeeah, S. M., et al. (2022). Teaching faculty perceptions, attitudes, challenges, and satisfaction of online teaching during COVID-19 pandemic in Saudi Arabia: A national survey. Front. Educ. 7:1015163. doi: 10.3389/feduc.2022.1015163
Althubaiti, A. (2016). Information bias in health research: definition, pitfalls, and adjustment methods. J. Multidiscip. Healthc. 9, 211–217. doi: 10.2147/JMDH.S104807
Balcioğlu, Y. S., and Artar, M. (2024). Artificial intelligence in employee recruitment. Glob. Bus. Organ. Excell. 43, 56–66. doi: 10.1002/joe.22248
Banh, L., and Strobel, G. (2023). Generative artificial intelligence. Electron. Mark. 33:63. doi: 10.1007/s12525-023-00680-1
Bates, T., Cobo, C., Mariño, O., et al. (2020). Can artificial intelligence transform higher education? Int. J. Educ. Technol. High Educ. 17:42. doi: 10.1186/s41239-020-00218-x
Budhwar, P., Chowdhury, S., Wood, G., Aguinis, H., Bamber, G. J., Beltran, J. R., et al. (2023). Human resource management in the age of generative artificial intelligence: perspectives and research directions on ChatGPT. Hum. Resour. Manag. J. 33, 606–659. doi: 10.1111/1748-8583.12524
Chan, C. K. Y., and Hu, W. (2023). Students’ voices on generative AI: perceptions, benefits, and challenges in higher education. Int. J. Educ. Technol. High. Educ. 20:43. doi: 10.1186/s41239-023-00411-8
Chan, C. K. Y., and Lee, K. K. W. (2023). The AI generation gap: are gen Z students more interested in adopting generative AI such as ChatGPT in teaching and learning than their gen X and millennial generation teachers? Smart Learn. Environ. 10:60. doi: 10.1186/s40561-023-00269-3
Chen, Q., Li, L., Chong, C., and Wang, X. (2022). AI-enhanced soil management and smart farming. Soil Use Manag. 38, 7–13. doi: 10.1111/sum.12771
Chui, M., Manyika, J., Miremadi, M., Henke, N., Chung, R., Nel, P., et al. (2018). Notes from the AI frontier: insights from hundreds of use cases. McKinsey Glob. Inst. 2:267. Available at: https://www.mckinsey.com/west-coast/~/media/McKinsey/Featured%20Insights/Artificial%20Intelligence/Notes%20from%20the%20AI%20frontier%20Applications%20and%20value%20of%20deep%20learning/Notes-from-the-AI-frontier-Insights-from-hundreds-of-use-cases-Discussion-paper.pdf
Crompton, H., and Song, D. (2021). The potential of artificial intelligence in higher education. Revista virtual Universidad Catolica del Norte 62, 1–4. doi: 10.35575/rvucn.n62a1
Crosby, L., and Bonnington, O. (2020). Experiences and implications of smartphone apps for depression and anxiety. Sociol. Health Illn. 42, 925–942. doi: 10.1111/1467-9566.13076
Davidovitch, N., and Cohen, E. (2024). Administrative roles in academia—potential clash with research output and teaching quality? Cogent Educ. 11:2357914. doi: 10.1080/2331186X.2024.2357914
Dennis, M. J. (2018). Artificial intelligence and recruitment, admission, progression, and retention. Enroll. Manag. Rep. 22, 1–3. doi: 10.1002/emt.30479
Dey, E. L. (1997). Working with low survey response rates: the efficacy of weighting adjustments. Res. High. Educ. 38, 215–227. doi: 10.1023/A:1024985704202
Dimari, A., Tyagi, N., Davanageri, M., Kukreti, R., Yadav, R., and Dimari, H. (2024), AI-based automated grading systems for open book examination system: implications for assessment in higher education. International conference on knowledge engineering and communication systems (ICKECS). Chikkaballapur, India. 1–7.
Diwan, C., Srinivasa, S., Suri, G., Agarwal, S., and Ram, P. (2023). AI-based learning content generation and learning pathway augmentation to increase learner engagement. Comput. Educ. 4:100110. doi: 10.1016/j.caeai.2022.100110
Education, D. F. (2024). Generative AI in education: Educator and expert views. Available at: https://www.gov.uk/government/publications/generative-ai-in-education-educator-and-expert-views.
Edwards, C. (2020). Male professor turns himself into anime schoolgirl to teach students remotely during coronavirus lockdown. March 18. The U.S. Sun. Available at: https://www.the-sun.com/lifestyle/tech/556889/male-professor-turns-himself-into-anime-schoolgirl-to-teach-students-remotely-during-coronavirus-lockdown/
Esmaeilzadeh, P. (2020). Use of AI-based tools for healthcare purposes: a survey study from consumers’ perspectives. BMC Med. Inform. Decis. Mak. 20, 1–19. doi: 10.1186/s12911-020-01191-1
Fagbohun, O., Iduwe, N. P., Abdullahi, M., Ifaturoti, A., and Nwanna, O. M. (2024). Beyond traditional assessment: exploring the impact of large language models on grading practices. JAIMLD 2, 1–8. doi: 10.51219/JAIMLD/oluwole-fagbohun/19
Falloon, G. (2010). Using avatars and virtual environments in learning: what do they have to offer? Br. J. Educ. Technol. 41, 108–122. doi: 10.1111/j.1467-8535.2009.00991.x
Farrelly, T., and Baker, N. (2023). Generative artificial intelligence: implications and considerations for higher education practice. Educ. Sci. 13:1109. doi: 10.3390/educsci13111109
Fitton, I., Clarke, C., Dalton, J., Proulx, M. J., and Lutteroth, C. (2023). Dancing with the avatars: Minimal avatar customisation enhances learning in a psychomotor task. 1–16. doi: 10.1145/3544548.3580944
Fitzpatrick, F. (2023). “How can we be inclusive of diverse cultural perspectives in international higher education? Exploring interculturality” in Intersectionality and creative business education. ed. B. S. Nayak (Springer) 5, 93–108. Available at: https://ideas.repec.org/h/spr/sprchp/978-3-031-29952-0_5.html
Fosnacht, K., Sarraf, S., Howe, E., and Peck, L. K. (2017). How important are high response rates for college surveys? Rev. High. Educ. 40, 245–265. doi: 10.1353/rhe.2017.0003
Gandomi, A., and Haider, M. (2015). Beyond the hype: big data concepts, methods, and analytics. Int. J. Inf. Manag. 35, 137–144. doi: 10.1016/j.ijinfomgt.2014.10.007
Gao, R., Merzdorf, H. E., Anwar, S., Hipwell, M. C., and Srinivasa, A. (2024). Automatic assessment of text-based responses in post-secondary education: A systematic review. Comput. Educ. Artif. Intell. 6:100206. doi: 10.1016/j.caeai.2024.100206
García-Gallego, A., Georgantzís, N., Martín-Montaner, J., and Pérez-Amaral, T. (2015). (how) do research and administrative duties affect university professors’ teaching? Appl. Econ. 47, 4868–4883. doi: 10.1080/00036846.2015.1037438
Gardner, A., Smith, A. L., Steventon, A., Coughlan, E., and Oldfield, M. (2022). Ethical funding for trustworthy AI: proposals to address the responsibilities of funders to ensure that projects adhere to trustworthy AI practice. AI Ethics 2, 277–291. doi: 10.1007/s43681-021-00069-w
Ghimire, A., Prather, J., and Edwards, J. (2024). Generative AI in education: A study of Educators' awareness, sentiments, and influencing factors. arXiv:2403.15586. doi: 10.48550/arXiv.2403.15586
Gong, Y., Zhao, M., Wang, Q., and Lv, Z. (2022). Design and interactive performance of human resource management system based on artificial intelligence. PLoS One 17:e0262398. doi: 10.1371/journal.pone.0262398
Goreis, A., Felnhofer, A., Kafka, J. X., Probst, T., and Kothgassner, O. D. (2020). Efficacy of self-management smartphone-based apps for post-traumatic stress disorder symptoms: a systematic review and meta-analysis. Front. Neurosci. 14:3. doi: 10.3389/fnins.2020.00003
Guan, Y. (2022). Student Education management strategy based on artificial intelligence information model under the support of 5G wireless network. Comput. Intell. Neurosci. 2022:4709146. doi: 10.1155/2022/4709146
Guegan, J., Buisine, S., Mantelet, F., Maranzana, N., and Segonds, F. (2016). Avatar-mediated creativity: when embodying inventors makes engineers more creative. Comput. Hum. Behav. 61, 165–175. doi: 10.1016/j.chb.2016.03.024
Hannan, E., and Liu, S. (2023). AI: new source of competitiveness in higher education. Competitiveness Rev. 33, 265–279. doi: 10.1108/CR-03-2021-0045
Hao, J., von Davier, A. A., Yaneva, V., Lottridge, S., von Davier, M., and Harris, D. J. (2024). Transforming assessment: the impacts and implications of large language models and generative AI. Educ. Meas. Issues Pract. 43, 16–29. doi: 10.1111/emip.12602
Haris, R. M., and Al-Maadeed, S. (2021). COVID-19 lockdown-challenges to higher education in Qatar. 267–273. doi: 10.1109/ISCAIE51753.2021.9431774
Harry, A., and Sayudin, S. (2023). Role of AI in Education. Interdicip. J. Hum. (Injurity) 2, 260–268. doi: 10.58631/injurity.v2i3.52
Holstein, K. (2019). Designing real-time teacher augmentation to combine strengths of human and AI instruction. Unpublished doctoral dissertation, Carnegie Mellon University.
Hong, S. W., Park, J., and Cho, M. (2019). Virtual vs. actual body: applicability of anthropomorphic avatars to enhance exploratory creativity in architectural design education. Archit. Sci. Rev. 62, 520–527. doi: 10.1080/00038628.2019.1669526
Hudson, I., and Hurter, J. (2016). Avatar types matter: Review of avatar literature for performance purposes. 9740, 14–21. doi: 10.1007/978-3-319-39907-2_2
İpek, Z. H., Gözüm, A. I. C., Papadakis, S., and Kallogiannakis, M. (2023). Educational applications of the ChatGPT AI system: A systematic review research. Educ. Process 12, 26–55. doi: 10.22521/edupij.2023.123.2
Jain, K., and Raghuram, J. (2024). Gen-AI integration in higher education: Predicting intentions using SEM-ANN approach. Educ. Inf. Technol. 1–41. doi: 10.1007/s10639-024-12506-4
Jin, S.-H., Im, K., Yoo, M., Roll, I., and Seo, K. (2023). Supporting students’ self-regulated learning in online learning using artificial intelligence applications. Int. J. Educ. Technol. High. Educ. 20:37. doi: 10.1186/s41239-023-00406-5
Kelly, A., Sullivan, M., and Strampel, K. (2023). Generative artificial intelligence: University student awareness, experience, and confidence in use across disciplines. J. Univ. Teach. Learn. Pract. 20. doi: 10.53761/1.20.6.12
Kim, K.-N. (2019). Teachers’ administrative workload crowding out instructional activities. Asia Pacific J. Educ. 39, 31–49. doi: 10.1080/02188791.2019.1572592
Kim, J., Merrill, K., Xu, K., and Sellnow, D. D. (2020). My teacher is a machine: understanding students’ perceptions of AI teaching assistants in online education. Int. J. Hum. Comput. Interact. 36, 1902–1911. doi: 10.1080/10447318.2020.1801227
Klutka, J., Ackerly, N., and Magda, A. J. (2018). Artificial intelligence in higher education: Current uses and future applications. Louisville: Learning House.
Kumar, A. D., Selvam, R. P., and Kumar, K. S. (2018). Review on prediction algorithms in educational data mining. Int. J. Pure Appl. Math. 118, 531–537. Available at: https://www.acadpubl.eu/jsi/2018-118-7-9/articles/8/77.pdf
Lim, W. M., Gunasekara, A., Pallant, J. L., Pallant, J. I., and Pechenkina, E. (2023). Generative AI and the future of education: Ragnarök or reformation? A paradoxical perspective from management educators. Int. J. Manag. Educ. 21:100790. doi: 10.1016/j.ijme.2023.100790
Liu, Y., Chen, L., and Yao, Z. (2022). The application of artificial intelligence assistant to deep learning in teachers' teaching and students' learning processes. Front. Psychol. 13:929175. doi: 10.3389/fpsyg.2022.929175
Livieris, I., Mikropoulos, T., and Pintelas, P. (2016). A decision support system for predicting students’ performance. Themes Sci. Technol. Educ. 9, 43–57. Available at: https://files.eric.ed.gov/fulltext/EJ1130918.pdf
Liz-Domínguez, M., Caeiro-Rodríguez, M., Llamas-Nistal, M., and Mikic-Fonte, F. A. (2019). Systematic literature review of predictive analysis tools in higher education. Appl. Sci. 9:5569. doi: 10.3390/app9245569
Mao, J., Chen, B., and Liu, J. C. (2024). Generative artificial intelligence in Education and its implications for assessment. TechTrends 68, 58–66. doi: 10.1007/s11528-023-00911-4
Martin, Q. (2022). From faculty to administration: preparing the next generation of academic leaders. Perspect. Policy Pract. High. Educ. 26, 109–114. doi: 10.1080/13603108.2021.2016513
Martin Iii, Q. (2021). Look before you leap: making the transition from administration to faculty. Int. J. Acad. Dev. 26, 110–115. doi: 10.1080/1360144X.2020.1795665
Moëll, B., Kollberg, L., Nasri, B., Lindefors, N., and Kaldo, V. (2015). Living SMART—A randomized controlled trial of a guided online course teaching adults with ADHD or sub-clinical ADHD to use smartphones to structure their everyday life. Internet Interv. 2, 24–31. doi: 10.1016/j.invent.2014.11.004
Nair, C. S., Adams, P., and Mertova, P. (2008). Student engagement: the key to improving survey response rates. Qual. High. Educ. 14, 225–232. doi: 10.1080/13538320802507505
Nawaz, N., Gomes, A. M., and Saldeen, M. A. (2020). Artificial intelligence (AI) applications for library services and resources in COVID-19 pandemic. Artif. Intell. 7, 1951–1955. doi: 10.31838/jcr.07.18.244
Oppong, S. O. (2023). Predicting students’ performance using machine learning algorithms: A review. Asian J. Res. Comput. Sci. 16, 128–148. doi: 10.9734/AJRCOS/2023/v16i3351
Pantelimon, F.-V., Bologa, R., Toma, A., and Posedaru, B.-S. (2021). The evolution of AI-driven educational systems during the COVID-19 pandemic. Sustain. For. 13:13501. doi: 10.3390/su132313501
Pataranutaporn, P., Danry, V., Leong, J., Punpongsanon, P., Novy, D., Maes, P., et al. (2021). AI-generated characters for supporting personalized learning and well-being. Nat. Mach. Intell. 3, 1013–1022. doi: 10.1038/s42256-021-00417-9
Perrault, R., Shoham, Y., Brynjolfsson, E., Clark, J., Etchemendy, J., Grosz, B., et al. (2019). The AI index 2019 annual report. Stanford, CA: AI Index Steering Committee, Human-Centered AI Institute, Stanford University.
Popenici, S. A. D., and Kerr, S. (2017). Exploring the impact of artificial intelligence on teaching and learning in higher education. Res. Pract. Technol. Enhanc. Learn. 12:22. doi: 10.1186/s41039-017-0062-8
Radovic, A., Vona, P. L., Santostefano, A. M., Ciaravino, S., Miller, E., and Stein, B. D. (2016). Smartphone applications for mental health. Cyberpsychol. Behav. Soc. Netw. 19, 465–470. doi: 10.1089/cyber.2015.0619
Rahkovsky, I., Toney, A., Boyack, K. W., Klavans, R., and Murdick, D. A. (2021). AI research funding portfolios and extreme growth. Front. Res. Metrics Anal. 6:630124. doi: 10.3389/frma.2021.630124
Ratan, R., Klein, M. S., Ucha, C. R., and Cherchiglia, L. L. (2022). Avatar customization orientation and undergraduate-course outcomes: actual-self avatars are better than ideal-self and future-self avatars. Comput. Educ. 191:104643. doi: 10.1016/j.compedu.2022.104643
Saaida, M. B. E. (2023). AI-driven transformations in higher education: opportunities and challenges. Int. J. Educ. Res. Stud. 5, 29–36. doi: 10.5281/zenodo.8164414
Sedgwick, P., and Greenwood, N. (2015). Understanding the Hawthorne effect. BMJ 351:h4672. doi: 10.1136/bmj.h4672
Sghir, N., Adadi, A., and Lahmer, M. (2022). Recent advances in predictive learning analytics: A decade systematic review (2012-2022). Educ. Inf. Technol. (Dordr.), 1–35. doi: 10.1007/s10639-022-11536-0
Shoham, Y., Perrault, R., Brynjolfsson, E., Clark, J., Manyika, J., Niebles, J. C., et al. (2018). The AI index 2018 annual report. AI Index Steering Committee, Human-Centered AI Initiative.
Shrestha, B., and Dunn, L. (2020). The declaration of Helsinki on medical research involving human subjects: A review of seventh revision. J. Nepal Health Res. Counc. 17, 548–552. doi: 10.33314/jnhrc.v17i4.1042
Solem, R. C. (2015). Limitation of a cross-sectional study. Am. J. Orthod. Dentofacial Orthop. 148:205. doi: 10.1016/j.ajodo.2015.05.006
Torous, J., Andersson, G., Bertagnoli, A., Christensen, H., Cuijpers, P., Firth, J., et al. (2019). Towards a consensus around standards for smartphone apps and digital mental health. World Psychiatry 18:97. doi: 10.1002/wps.20592
Torous, J., and Roberts, L. W. (2017). Needed innovation in digital health and smartphone applications for mental health: transparency and trust. JAMA Psychiatry 74, 437–438. doi: 10.1001/jamapsychiatry.2017.0262
Turing, A. M. (1936). On computable numbers, with an application to the Entscheidungsproblem. J. Math 58:5.
Turing, A. M. (2009). “Computing machinery and intelligence,” in Parsing the turing test. eds. R. Epstein, G. Roberts, and G. Beber Dordrecht: Springer.
Van Ameringen, M., Turna, J., Khalesi, Z., Pullia, K., and Patterson, B. (2017). There is an app for that! The current state of mobile applications (apps) for DSM-5 obsessive-compulsive disorder, posttraumatic stress disorder, anxiety and mood disorders. Depress. Anxiety 34, 526–539. doi: 10.1002/da.22657
Van Mol, C. (2017). Improving web survey efficiency: the impact of an extra reminder and reminder content on web survey response. Int. J. Soc. Res. Methodol. 20, 317–327. doi: 10.1080/13645579.2016.1185255
Vittorini, P., Menini, S., and Tonelli, S. (2021). An AI-based system for formative and summative assessment in data science courses. Int. J. Artif. Intell. Educ. 31, 159–185. doi: 10.1007/s40593-020-00230-2
Walia, N., Kumar, M., Nayar, N., and Mehta, G. (2020). Student’s academic performance prediction in academic using data mining techniques. Proceedings of the international conference on innovative computing & communications (ICICC) 2020. Available at SSRN: https://ssrn.com/abstract=3565874 (Accessed April 1, 2020).
Wu, E. H.-K., Lin, C.-H., Ou, Y.-Y., Liu, C.-Z., Wang, W.-K., and Chao, C.-Y. (2020). Advantages and constraints of a hybrid model K-12 E-learning assistant chatbot. IEEE Access 8, 77788–77801. doi: 10.1109/ACCESS.2020.2988252
Wu, A., Scult, M. A., Barnes, E. D., Betancourt, J. A., Falk, A., and Gunning, F. M. (2021). Smartphone apps for depression and anxiety: a systematic review and meta-analysis of techniques to increase engagement. NPJ Digit. Med. 4:20. doi: 10.1038/s41746-021-00386-8
Yusuf, A., Pervin, N., and Román-González, M. (2024). Generative AI and the future of higher education: a threat to academic integrity or reformation? Evidence from multicultural perspectives. Int. J. Educ. Technol. High. Educ. 21:21. doi: 10.1186/s41239-024-00453-6
Yusuf, A., and Tambuwal, N. I. (2018). Integrating educational Technology in Teaching: current perceptions and practices in Sokoto State, Nigeri. Arab J. Qual. Educ. 5, 7–16. doi: 10.12816/0051288
Keywords: artificial intelligence, generative AI, higher education, machine learning, pedagogy
Citation: Arowosegbe A, Alqahtani JS and Oyelade T (2024) Perception of generative AI use in UK higher education. Front. Educ. 9:1463208. doi: 10.3389/feduc.2024.1463208
Edited by:
Halvdan Haugsbakken, Østfold University College, NorwayCopyright © 2024 Arowosegbe, Alqahtani and Oyelade. This is an open-access article distributed under the terms of the Creative Commons Attribution License (CC BY). The use, distribution or reproduction in other forums is permitted, provided the original author(s) and the copyright owner(s) are credited and that the original publication in this journal is cited, in accordance with accepted academic practice. No use, distribution or reproduction is permitted which does not comply with these terms.
*Correspondence: Tope Oyelade, dC5veWVsYWRlQHVjbC5hYy51aw==