- 1NOVA Information Management School, Universidade Nova deLisboa, Lisbon, Portugal
- 2Facultad de Ingeniería, Universidad Nacional de Chimborazo, Riobamba, Ecuador
The rise of smart learning environments and the transformative potential of the Metaverse are increasingly evident across various sectors, particularly in education. While substantial attention has been directed toward student engagement within the Metaverse, the evolution of teaching methodologies enabled by this technology remains under-examined. This study investigates the influence of teachers’ prior experience with technology on their willingness to adopt the Metaverse in diverse educational contexts. Utilizing a sample of 311 educators from the Brazilian Amazon, partial least squares structural equation modeling (PLS-SEM) was employed to validate the theoretical model. The findings emphasize the significant relationship between previous technological experiences, the mediating role of social influence in adoption, and performance expectations. Furthermore, the results underscore the pivotal role of technological engagement in shaping educators’ perceptions and their readiness to adopt emerging innovations, such as the Metaverse.
1 Introduction
The Metaverse has been a disruptive technological advancement with the potential to revolutionize the area of information systems (Dwivedi et al., 2022). The concept of the Metaverse (Smart et al., 2007) represents a visionary exploration of future technology encompassing the convergence of an enhanced physical reality and a persistent virtual space. In the realm of education, the Metaverse promises a revolutionary shift in educational management models and learning methodologies, offering potential efficiencies surpassing traditional audiovisual materials (Yeh, 2022), virtual reality (VR), and augmented reality (AR) technologies (Wang et al., 2022).
Disruptive technologies like the Metaverse have found extensive use in enhancing professional training across various fields and industries. Schools focused on technology and training have leveraged technologies such as drones, VR, AR, and simulation tools to elevate the quality of education provided (Dwivedi et al., 2022). However, the adoption of these technologies by educators accelerated the integration of digital information and communication technologies (DICT) in education, which has progressed at a relatively slow pace (Wang et al., 2022). However, the lack of consensus regarding the continued use of technology in educational institutions remains evident (Claro et al., 2018).
Prior knowledge of a particular technology plays a crucial role in mitigating potential psychological barriers that users might encounter when encountering a new technology or a similar piece of technology (Wang et al., 2022). The adoption of technology depends on individuals’ characteristics, past experiences, and their readiness to embrace innovation (Parasuraman and Colby, 2015). In such environments, expertise is highly valued and carries social prestige, prior technological experience becomes a potent predictor of social influence. Hence, high-tech knowledge serves as a valuable asset for social interactions and plays a pivotal role in shaping opinion leadership (Arruda Filho et al., 2022; Da Costa-everaldo et al., 2022).
To shed light on the emerging topic of Metaverse among educators in developing contexts, models such as the technology acceptance model (TAM) and the unified theory of acceptance and use of Technology (UTAUT) have been employed to understand the adoption of technological innovations (Venkatesh, 2022). Specifically in the field of education with smart learning environments, the UTAUT model has been widely employed to explain how teachers adopt technology in their classrooms, taking into account psychological factors, such as their apprehension toward innovation (Wang et al., 2022) as well as other factors related to their previous technological experiences (Claro et al., 2018; Devaraj et al., 2008) However, in these acceptance models, prior knowledge is often seen as a consequential influencer of behavioral use patterns (Arruda Filho et al., 2022).
In short, this article makes significant contributions to the literature on technological expertise and the adoption of disruptive innovations in education, particularly in the unique context of the Brazilian Amazon. Initially, it reveals the impact of educators’ previous experiences in adopting technology, mediated by the primary constructions of the UTAUT model. In the distinctive domain of technology and career-oriented education, educators’ past involvement in innovation and the use of technology shape both the quality of their pedagogy and their ability to engage in technology-driven initiatives.
Furthermore, this study explores the potential of virtual worlds to establish immersive learning and assessment environments in educational institutions. This approach appears as an indispensable catalyst to overcome traditional barriers and allow the achievement of pedagogical objectives through immersive experiences, in which students and teachers not only interact with the content but also integrate with it. As a result, they shape and develop significant new skills, such as communication and critical/creative thinking, while exploring a vast universe of knowledge beyond the physical and geographical borders that often challenge access to quality education in the region—a facet especially relevant in the context of the Brazilian Amazon (Massaro et al., 2011).
This article seeks to investigate how these dynamics manifest in the adoption of the Metaverse by educators in the context of the Brazilian Amazon, a region where geographical barriers and the lack of technological infrastructure represent significant challenges to accessing quality education. The study contributes to the literature by analyzing how educators’ prior experiences influence the adoption of technological innovations, mediated by the main constructs of the UTAUT model, and by exploring the role of virtual worlds in establishing immersive and interactive learning environments capable of overcoming traditional barriers.
Finally, this study highlights the adoption of the Metaverse by educators in the Brazilian Amazon, contributing to emerging educational technologies and offering valuable insights into the unique challenges and opportunities presented by such contexts for the adoption of ICT in education, designing a more inclusive educational horizon and dynamic for the region and its communities.
2 Theoretical framework
The choice of the Brazilian Amazon as the context for our study on the adoption of the Metaverse in education is rooted in the exceptional biodiversity, cultural diversity, economic and environmental significance, educational challenges, socioeconomic disparities, and government initiatives of region. This multifaceted context offers a rich and complex backdrop for examining the integration of emerging technologies into education. Insights derived from this context are not only pertinent to Amazon but also have broader implications for technology adoption in education within diverse and dynamic environments globally.
Furthermore, the economic and environmental importance of Amazon cannot be underestimated. The region plays a crucial role in global climate regulation and the conservation of natural resources, making it a strategic location for the implementation of innovative solutions aimed at sustainability and environmental management. However, Amazon also faces significant educational challenges, including the lack of adequate infrastructure and limited access to quality educational resources. These challenges are further exacerbated by socioeconomic disparities, which vary widely within the region and among its communities.
The vast and often remote geographical expanse of the Brazilian Amazon presents unique challenges in delivering quality education. The uneven infrastructure and varying levels of access to educational resources of the region compound these challenges. Investigating the role of technologies like the Metaverse in addressing these hurdles is particularly relevant. It offers an opportunity to explore how technology can potentially bridge geographical gaps and enhance educational access in remote and underserved areas.
The Brazilian Amazon is also characterized by significant socioeconomic disparities, with some areas facing economic disadvantage. This socioeconomic diversity makes it an intriguing context for examining how technology adoption, especially in education, can either mitigate or exacerbate these disparities. Insights from this study can shed light on the potential of emerging technologies to act as equalizers in education, reducing disparities and promoting equitable access to educational opportunities.
2.1 Technology adoption in developing contexts
This analysis focuses on understanding motives and obstacles that impact how teachers adopt different technologies. In addition, it explores psychological predispositions that shape their attitudes when it comes to using said technologies, as well as stimulating or inhibiting feelings that might encourage or discourage technology adoption (Xi et al., 2023).
Parasuraman and Colby (2015) carried out a study to comprehend consumers’ motivation and barriers to the adoption of several technologies, i.e., how psychological predispositions affect an individual’s attitude when using technologies, as they foster motivational or inhibiting feelings for that end (Claro et al., 2018; Devaraj et al., 2008). Technology adoption as a process refers to how likely it is for people to use, in a continued manner, new technologies to achieve their life goals, though mental enablers or inhibitors, which, in turn, determine collectively a person’s predisposition to use new technologies (Parasuraman and Colby, 2015).
According to Rogers et al. (2014), this adoption comes from a series of choices made by an individual, leading him/her to incorporate or not certain innovative practices to their continued use. The author also describes the adoption process occurring in five stages: knowledge, persuasion, decision, implementation, and confirmation. Knowledge refers to how the individual gains comprehension of the workings of an innovation, whereas persuasion refers to when the individual forms a favorable or unfavorable attitude toward an innovation (Williams and Daley, 2021).
A decision may be considered as an act of engaging in activities leading to a choice of adopting or rejecting an innovation (Parasuraman and Colby, 2015). Implementation encompasses a new idea put to use, in order to occur a confirmation on an individual’s search for positive reinforcement about an already made decision. Finally, it would reinforce such a decision or revert it due to finding conflicting messages (Blut and Wang, 2020).
Additionally, the unique points of the Brazilian Amazon region add new elements to the aspects proposed by Rogers et al. (2014), such as the influence that the environment exerts on individuals. This becomes evident when we consider the demographics of the place, characterized by the precarious quality of infrastructure. This scenario amplifies the digital divide in the region, reflecting a continuous and significant disparity in access to technologies present in smart learning environments such as the Metaverse. Furthermore, this gap deepens given the need to train local educators, who face logistical challenges in obtaining qualifications, as well as difficulties in applying the new knowledge acquired in their daily activities.
Adoption processes have been studied in several contexts of technology use, such as mobile banking (Arruda Filho et al., 2022), educational usage of apps in a Mediascape marked by social networks, and in the context of truly innovative products (Dos Santos et al., 2022). Specifically in relation to the Metaverse environment (Akour et al., 2022), it is affirmed that the adoption process is likely to occur through the perceived usefulness of system being identified by potential users, especially in educational settings.
2.2 Performance expectations
Herein, the central analysis deals with the concept of performance expectations, which refers to a user’s belief that adopting a technology system may improve his/her efficacy. In addition, it explores how such a belief informs the individual’s acceptance level. Moreover, it highlights to which extent performance expectation consists of a substantial indicator of usage intent, exerting considerable influence on technology adoption (Kinshuk et al., 2016).
Performance expectation represents the degree to which an individual assumes that utilizing a system may help him/her to reach a better performance in his/her work (Arruda Filho et al., 2022) Thus, it is possible to understand that the user’s level of belief in the system make performance viable, within a relationship of relative advantage (Venkatesh et al., 2003).
To deepen the comprehension of this construct, it is necessary to understand UTAUT globally, contributing to grasping which factors influence a user to accept and use certain technologies (Wang et al., 2022). Performance expectation is defined as the result a user expects when using a specific technology (Venkatesh and Davis, 1996).
This construct was presented by (Venkatesh et al., 2003) from concepts related to behavioral models, such as perceived usefulness (TAM/TAM2 C-TAM-TPB), extrinsic motivation (MM), work adequacy (MPCU), relative advantage (IDT), and expectation results (SCT) (Venkatesh et al., 2003). Some authors consider performance expectation as the strongest intent indicator, being meaningful to its total measurement points.
In addition, this construct has been employed in the UTAUT model for predicting behavioral intent. In an educational setting, this construct has shown a significant effect on usage intentions and technology acceptance (Compeau and Higgins, 1995). One must acquire previous knowledge of resources through specific training, oriented to a proper applicability guided by a didactic approach. That is why it is understood that performance expectation is defined by how an individual sees a piece of technology as more or less advantageous, basically this being an extrinsic motivation that absorbs relationships from the user’s environment (Garone et al., 2019).
2.3 Effort expectation
Aiming to understand the relationship between a user’s perceived effort when using the system and its following adoption, this paper focuses on the importance of effort expectation. It also explores how such expectation might be an obstacle, especially during the initial stages of new technology adoption.
The easiness degree associated with technology is one of the definitions for effort expectation, i.e., the amount of effort identified at the user’s end when utilizing a system; so, the lower the usage rate, the more complex an innovation is (Venkatesh et al., 2003). Observations point out that effort expectation being an obstacle for new technology acceptance, as user’s thoughts are only defined after a practical experience with it (Davis et al., 1992; Moore and Benbasat, 1991; Thompson et al., 1991).
This construct is often presented as meaningful for one to adhere to new technologies during its initial usage phases, but after a certain while the same construct tends to no longer be of relevance (Blut et al., 2021). Some studies have defended the idea of a lower acceptance being the result of an elevated level of difficulty a piece of technology poses when used by new users, which leads to a drop in levels of satisfaction (Garone et al., 2019).
2.4 Easing conditions
The discussion here aims to understand the interactions between an individual and easing conditions, as well as how such an interaction impacts usage intent substantially. It also explores the ways an organizational and technical assistance plays a positive role in the efficient adoption and use of technology, mainly in educational contexts using smart learning environments.
This construct is defined as the degree to which an individual believes there is an organizational and technical infrastructure that supports the handling, i.e., departures from a user’s notion that the support offered by the organization will influence or not his/her adhesion to a new technology (Thompson et al., 1991; Venkatesh et al., 2003). When analyzed in conjunction with effort expectation, easing conditions do not have a significant influence on usage intent, as they are indirectly absorbed by effort expectation.
Objective factors such as the above are considered external to individuals, being characterized as easing to some extent, or affecting how system usage intentions occur (Warshaw and Davis, 1985). Thus, external factors that might compromise technology applicability should be accompanied by support of its needs, so as to not affect essential behavioral factors.
Within an educational setting, easing conditions as a construct has represented to what degree teachers have observed technologies, identifying existing technical support, institutional policy adhesion, and usage-oriented leadership (Lu et al., 2022). Some instances of research suggest that easing conditions is highlighted through training, information, available materials, and technical support, contributing to a positive effect of technology usage acceptance.
2.5 Anxiety
The central focus here is on understanding of anxiety as apprehension an individual feels when it comes to perceptions on technology use. It also explores how this negative reaction to usage has a considerable effect on a user’s behavior, performance, and learning.
In this context, anxiety is understood as an individual’s fear of directly relating to general perceptions of technology use he/she has, creating tension and nervousness (Compeau and Higgins, 1995). As a variable in an individual’s attention, anxiety leads to several causes of a negative affective reaction regarding computer usage (Dwivedi et al., 2019; Venkatesh et al., 2003).
Research on digital information and communication technologies, and psychology, have emphasized the importance of computer usage anxiety to characterize aspects this usage has, being a variable related to emotion. Hence, anxiety is shown to have a significant impact on a user’s behavior, performance, and learning (Igbaria and Parasuraman, 1989).
2.6 Social influence
The text tackles the concept of social influence in technology adoption and how this construct is related to an individual perception on the significance of adopting a certain technology, especially based on the importance of opinions from an individual’s social circle. In addition, it deals with the individual being acknowledged by people whose credibility impacts the trust and understanding of new products and services.
This is understood as the degree to which an individual imagines others consider his/her use of technology. Thus, social influence is an initial balance point that ends up influencing potential user’s behavior in the face of a new technology. Once voluntary usage comes up, these variables are limited to an interference over perceptions on technologies, even though they do not impact the use in a significant way (Venkatesh et al., 2003).
This construct portrays an emphasis on opinions from individuals who have influence over an individual, mostly relating to system usage intent; as such, an opinion is a relevant factor for a probable adhesion to a new technology. Social influence is understood as having among its characteristics the search for group support by an individual, mainly in obligation contexts (Warshaw and Davis, 1985).
Social influence precedes a comprehensive value of a product/service, which happens through behavioral intentions being created. Such interaction leads an individual to understand it is important for others to believe they are using a new technology that, in turn, corresponds to their expectations (Blut and Wang, 2020). People highly acknowledged by an individual have a greater credibility, which ends up influencing trust and comprehension on a product or a service.
2.7 Previous experience
Focusing on previous experiences, the text tackles the influence this variable has on technology adoption, mainly in the initial stages of a new one being accepted. In addition, how it may lead users to compare their previous experiences and their beliefs on access readiness, assessing its usefulness.
When applied to computer usage and smart learning environments, this construct proves an individual understands how to handle this technology with no proper support (Potosky and Bobko, 1998) This variable is considered to influence several technological notions, important to a new technology usage in its initial moments (Kreijns et al., 2013; Langer et al., 2018). Therefore, it is possible for a user to make a comparison based on prior experiences, specifically his/her beliefs in access readiness and how it is going to turn it into functional technology (Kim and Park, 2018).
One may realize this when considering the expansion the world has been through, followed by increased demands for quicker learning, which inevitably limits users’ access in the face of the complexity and difficulty newer technological products have. Overall, it is possible to understand the direct effect of this construct on behavioral intention, altered according to the degrees of the user’s experience evolution (Burton et al., 2003).
Again, when previous experiences with technological products have compatible characteristics that ground their acceptance, users tend to identify the new product based on its complexity degree. Such a construct generates constructive judgments in relation to the system, festering a greater user interaction with new technologies, resulting in satisfaction (Tlili et al., 2022).
2.8 Conceptual model
The main focus of this section is on introducing a conceptual model that summarizes an explanation of system structures and behaviors. In addition, it deals with hypotheses central to the research and explains their relationship.
The proposed conceptual model was based on relations of theoretical evidence, as they emerge from constructs first presented in the UTAUT model, and empirical observations of every behavioral event sequence related to possible uses of the Metaverse within the field of technological- and career-oriented education.
Due to this type of education being focused on innovation adoption aiming at a quality improvement in teaching and learning processes, this model identifies previous experiences as a preceding construct to social influence and potential users’ expectations of innovation. Thus, such an adoption refers to a real user disposition—in this case, the users being teachers—to employ the Metaverse as a tool in technological education, with their current knowledge about technology as a starting point.
In the context of technological- and career-oriented education, prior knowledge about technology is an important indicator of a teacher’s degree and capabilities to create “connectivity” between theoretical knowledge and crucial practical abilities toward learning (Antonietti et al., 2022). Those aspects enable a professional and social advantage, which makes this characteristic relevant in relation to social influence. In turn, social influence has an effect on performance expectations and an individual’s effort, especially those with fewer to none previous experiences with similar technology usage (Blut and Wang, 2020). It also affects the perception on easing conditions promoted by education institutions under SECTET/PA. On the other hand, this social pressure by teachers who are “opinion leaders” may lead to anxiety, even reducing users’ disposition to innovation (Mohamed et al., 2021).
From the model proposed in Figure 1 previous experience may have an effect on teachers’ social influence and on how much they realize that technology adoption is helpful for their own performance when using education tools on the Metaverse (Dwivedi et al., 2022). Once performance expectation consists of technology usefulness to teaching, it was also considered the most important aspect of adoption (Garone et al., 2019). Thus, teachers not only consider it useful and realize advantages when using education tools on the Metaverse but also should be willing to adopt it. This leads us to formulating the following hypothesis:
H1: Performance expectations on education apps on the Metaverse are going to have a positive effect on adopting this technology for educational ends.
Effort expectation has been shown as relevant in the adoption of disruptive technologies (Tamilmani et al., 2021), such as the Metaverse. This factor ties back to how adoption is related to differentiating advantages during a technological learning process (Bernacki et al., 2020). Hence, simpler technologies—ones that promote quick learning—turn out to be less attractive for potential users (Tamilmani et al., 2021). Therefore, those teachers who wish to distinguish themselves by proper new technology usage will also wish that effort expectation related to technology allows them to have an advantage during the adoption processes. That is why we formulate the second hypothesis:
H2: Effort expectation will positively impact education.
This search for distinction focused on competition is both based on effort expectation and on affecting teachers’ anxiety. In this sense, innovation with low effort expectations might create greater anxiety due to a need for maintenance and/or image rehabilitation for teachers in the technological school network. This, in turn, allows us to formulate the third hypothesis:
H3: Effort expectation will negatively impact teachers’ anxiety.
First, it is necessary to take into consideration psychological barriers created by a lack of equipment, training, support, or even access to truly new technologies. Those are common characteristics in teachers’ perceptions of public education management in Brazil (Bervell and Arkorful, 2020), all of which may affect anxiety among teachers. In this context, a lack of support for a grasp on technology innovation ends up creating a weak demand for teaching quality and technology adoption. In the face of that, the fourth hypothesis is as follows:
H4: Easing conditions will positively affect society.
Anxiety is characterized as apprehension or fear teachers might have in relation to adopting technology innovation in their educational activities (Dwivedi et al., 2019; Mohamed et al., 2021). Hence, it is expected that anxiety has a negative effect on adoption, which leads to the fifth hypothesis:
H5: Anxiety will negatively affect adoption.
Technology adoption processes among teachers have been mediated by a degree of support promoted by education institutions (Akour et al., 2022). Easing conditions are then perceived as structure and resources provided by such organizations. Thus, technology resources may improve teachers’ vision regarding the possibilities of technology innovation. This precise aspect leads to a sixth hypothesis:
H6: Easing conditions will positively affect technology innovation adoption.
Following the model presented in Figure 1, social influence may directly impact potential users’ expectations of technology innovation (Dwivedi et al., 2022). This happens due to teachers being exposed to the opinions of people who are important to them, which may change their perceptions related to innovation possibilities (7). Hence, the next hypothesis is as follows:
H7: Social influence will positively affect performance expectation.
Regarding the impact social influence has on performance, it is necessary to highlight its relevance for teachers’ interactions, approaching aspects such as usability and benefits allowed by technology, in both person and remotely. This process ends up creating an influencing capability over other individuals, so they adopt a certain technology, be it in professional or domestic environments, which, in turn, makes viable an all-encompassing technological support.
H8: Social influence will positively affect effort expectation.
Social influence means the degree of importance a technology user attributes to close people’s opinions, such as friends, relatives, and acquaintances. This scenario possibly affects technology adoption by teachers, as their colleagues’ opinions are themselves relevant positions that inform perceptions of technology adoption in the classroom as being important, even though it may also cause some degree of anxiety. As the ninth hypothesis, it is formulated:
H9: Social influence will positively affect anxiety.
Social influence may have an effect on perceptions about the easing conditions being offered by an educational establishment. This happens due to a process of social exchange within education environments (Kwon et al., 2020), itself associated with pressure from the potential innovation user’s close people; in the face of that, there could be a perception of “support” and “assistance” owing to the information shared. Hence, the tenth hypothesis is as follows:
H10: Social influence will positively affect perceptions of easing conditions.
In this context, previous experience with technology use may be a prior factor to performance expectations, due to possibilities related to objective and/or subjective knowledge of technology (Mullins and Cronan, 2021), allowing the emergence of predictions of real potentialities of innovation usage. As the next hypothesis:
H11: Previous experience will positively affect performance expectation.
In this context, previous experience with technology may classify users as influencers or influenced within social groups, especially in education environments, due to a level of proximity regarding social relationships among the school community (Arruda Filho et al., 2022) As a result, teachers with greater previous knowledge influence other teachers in the process of technology use. Therefore, the twelfth hypothesis is as follows:
H12: Previous experience will positively affect social influence.
Finally, previous experience could have an effect on perceptions of the conditions offered by educational organizations and their management practices. In particular, when it comes to assistance around knowledge and required materials for implementing technological innovations. This leads to the thirteenth hypothesis:
H13: Previous experience will positively affect easing conditions of usage of technological innovation.
This construct first and foremost assesses an individual’s capability of interacting with newer technologies, as he/she is propelled mainly by prior experience. This situation is enacted by comparing users’ experiences, which assures accessibility and technology usage, resulting in a proper increase in adoption.
3 Methodology
Initially, this research is of an exploratory nature, aiming at identifying preexisting latent relationships from the construct called previous experience. In order to do so, a survey was developed from a self-administered closed questionnaire, with a quantitative presentation of results.
Thus, structural equation modeling (SEM) was employed as a predominant statistical technique to understand the conceptual linkages proposed by the study. This multivariate analysis technique allows for a comprehension and explanation of the aforementioned linkages within the conceptual model, as well as the meaning behind each construct (Hair et al., 2014).
3.1 Presentation of the PLS-SEM method
First, the partial least squares structural equation modeling (PLS-SEM) was employed in this study. Again, this technique allows one to analyze the theoretical model being proposed, which is carried out through latent variable testing (external model) and through relations between those variables (internal model).
Quality criteria for the structural model, according to Hair et al. (2014), are related to convergent validity (at the level of items and constructs). Regarding the items, the study observed factorial loads (cross-loading analysis); and regarding the constructs, the average variance extracted (AVE). Convergent validity was established by the Fornell–Larcker criterion and construct reliability (based on Cronbach’s alpha) Hair et al. (2014). To analyze the structural model, the study observed the variance inflation factor (VIF), the predictive power, the exploratory sample (R2 and Q2), and coefficient significance and relevance (Hair et al., 2014).
3.2 Research aspects (target public, sample design, scenarios, and reliability)
This research aim was to understand how previous experience affects social influence and expectations of potential technology users, as well as the easing conditions promoted by the Pará state public education network of technology- and career-oriented schools. The context refers to the possibilities of smart learning environments specifically using the Metaverse in educational activities, with SECTET/PA teachers as its target audience.
Owing to it, a self-administered closed e-questionnaire, available on educação-Tec,1 was sent to the Pará state public education network teachers, who consented to participate in the research after a preliminary contact; the total amount of respondents was 311.
Thus, the sample was characterized as non-probabilistic, in conjunction with a post-hoc analysis of desired statistical power of 0.9, a predicted size effect of f2 = 0.255, and a reliability level of 0.05. The stimulus scenario was based on two videos available on Education in the Metaverse2 and 3DMeet—El Metaverso para la Educación Virtual.3 The goal was to show possibilities brought forth by the Metaverse to develop learning among students. After watching those videos, respondents had access to the questionnaire with 36 questions, split into the respondents’ sociological and psychological profile.
The scale of constructs employed in the conceptual model was adapted from previous studies, as shown in Table 1. Each item required an assessment through an original qualitative statistical scale of 7 points. This scale is a metric employed in models to turn qualitative variables into metrics. Constructs were measured on an assessment scale from 1—hardly to 7—fully. Most constructs have their origin in the UTAUT model.
Data sampling, as illustrated in Figure 2, revealed that 51.7% of respondents were male, with an average age of 44 years. The most frequently family income sits around [R5,100.00 to R10,000.00], accounting for 35.4%. In addition, when asked about their time of service as teachers, it was verified an average of 15.5 years and a median of 15 years.
4 Presentation of results
Initially, factorial loads in the items of reflexive variables were verified, considering what was presented by the model, with the ideal loads being above 0.7. The discriminant variable was also analyzed through the Fornell–Larcker criterion. Hence, Table 2 shows the reliability criteria for the research constructs (Cronbach’s alpha); regarding this criterion, the adequate reference value is 0.7, while the average variance extracted criterion is 0.5, considered as external quality for the proposed model (Hair et al., 2021).The hypotheses were tested via a path model presented by the partial least squares structural equation modeling (PLS-SEM), version 4. It is a model that offers a flexible and powerful approach to examining complex interactions between variables, through a basic bootstrapping procedure, with 5,000 occurrences of change. This multivariate method allows one to estimate models employing cause-and-effect relationships between latent variables, as illustrated by the model in Table 2 (Hair et al., 2014). Thus, Table 3 shows the results obtained by the procedure, along with R2 values for a global effect comprehension. Path coefficients presented in Table 3 show an effect intensity and direction, while R2 values show the level of explanation for the model (Hair et al., 2011). As for the Q2 value in PLS Predict, it compares the PLS path model prediction errors, with simple average predictions (Hair et al., 2019). A collinearity test was also carried out in order to assure trajectory integrity. Hence, the results presented by observed variables (external VIF) fall into the interval [1.471; 4.959], as well as for the constructs (internal VIF), falling into the interval [1; 1.892]. (Hair et al., 2014; Henseler et al., 2015).
Moreover, a model fit testing was conducted, representing an adequacy metric for structural equation modeling, based on the partial least squares method, in which quality indexes saturation show a partial adequacy due to SRMR = 0.056 (adequate values <0.08), and NFT = 0.820 (reference values >0.9), as well as χ2 = 1.124.312 (Henseler et al., 2015).
5 Discussion of results
The conceptual model proposes that previous experience has a preceding effect on performance expectation, social influence, and easing conditions that lead to disruptive technology adoption, such as in the Metaverse case. This happens due to previous experience with technology being able to influence an individual’s several conceptions regarding technology usage (Schophuizen et al., 2021; Luan et al., 2020); in addition, it may create beliefs that guide perception of innovation utility and comparison (relative advantage) (Connolly et al., 2019).
Previous experience, as shown above in Table 3, presents significant and direct effects on the constructs, performance expectation, social influence, and easing conditions. However, it is necessary to verify whether or not it acts as a precursor to the adoption of innovative technologies, considering possible perceptions of the use of smart learning environments, specifically the Metaverse, in educational activities. In this sense, Table 4 shows how this construct works within the proposed conceptual model. Mediations 1 and 2 present a significant indirect path, with a positive structural coefficient. Furthermore, the direct path also shows a positive significant relationship, meaning those relations are complementary partial mediations (Hair et al., 2017). Double partial mediation (see Mediation 1, Table 4) shows that social influence and performance expectations are constructs affected by previous experience. In turn, this fosters trust and a shorter time passage in relation to knowledge of possibilities promoted by technology innovation (Blut and Wang, 2020).
In addition, previous experience is shown to have a direct effect on performance expectations. Even though the Metaverse is a reality that is far from a sizable number of teachers from the Pará state technological public education network, performance expectation could be interpreted as a complementary partial mediator between previous experience and adoption (see mediation 2, Table 4). This relationship means that prior knowledge, resulting from a school technological environment and experiences with technological devices, will be mediated by a perception of “relative advantage” related to smart learning environments, with special emphasis on the possibilities of using the Metaverse in an educational environment.
On the other hand, the mediating effects herein proposed (see mediations 3, Table 4) do not present significance for specific indirect pathways, which shows how those path sequences do not affect adoption in the presented scenario (Henderson and Corry, 2021). Maybe this happens because both anxiety and easing conditions require a closer perception of use implementation and of Metaverse usage in an educational environment. Moreover, easing conditions refer to the degree of technology support a user has from a certain organization (training, equipment, etc.) (Venkatesh et al., 2003). Nevertheless, this scenario might not be considered by teachers in the face of disbelief about the possibilities of technological and technical assistance coming from technology education school management.
Construct performance within the structural model proposed highlights how strong of an effect the previous experience has on social influence, performance expectation, and easing conditions. However, only performance expectation and effort expectations are affected through social influence, contributing to adoption within the system. When construct performance in the model is considered, it is possible to see that only anxiety has a performance below 50%; i.e., this construct does not have a more significant contribution to understanding adoption in a scenario where there are perceptions of Metaverse usage possibilities. However, most constructs have performance above 70%, demonstrating effect explanation capabilities among the proposed constructs (Figure 3).
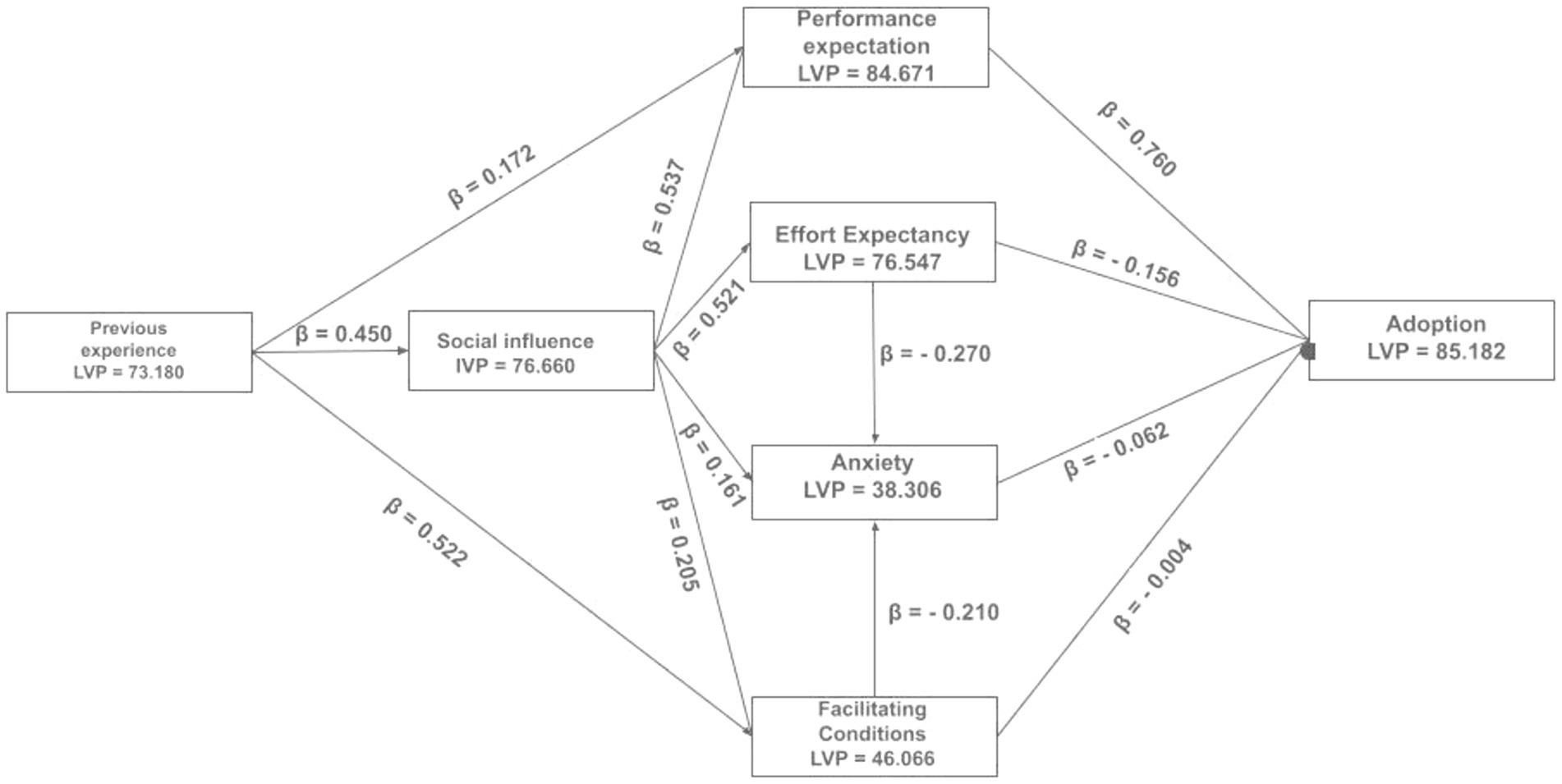
Figure 3. Construct performance and importance within the structural model. Latent variable performance = LVP.
Results then show that previous experience is relevant to disruptive technology adoption, as in the Metaverse case, especially when performance expectation is assessed. In other words, what is being assessed are possible relative advantages regarding this type of technology for developing educational activities (Saritas and Topraklikoglu, 2022), in a technology- and career-oriented education environment.
6 Theoretical and managerial implications
This study initially aims at analyzing previous experience with technology to verify perceptions of Metaverse being adopted as an educational tool, with usage precedents, especially in the realm of the Pará state technology- and career-oriented public education network. Theoretically, prior education has been shown to have significant effects as a moderating (Hakverdi-Can and Dana, 2012) and mediating construct (Peechapol et al., 2018) regarding the relationships that lead to adoption. However, in specific scenarios—such as the phenomenon of truly new results of innovation being adopted, this construct may be presented as a key characteristic for those products/services being successful (Dholakia et al., 2021).
When it comes to perceptions of possible Metaverse applications to education, teachers’ previous experience presented complementary partial mediation (see Table 4) for mediations 2 and 3, which highlights that this construct may precede product performance expectation. In other words, it creates conditions for viability and opportunity understanding; the first is associated with potential innovation advantages, while the second is related to application possibilities, promoting users’ creativity before they effectively come across novelty products presenting disruptive attributes.
In addition, preexisting experience may habilitate users of innovative technological products to be in a position through which they could have influence over other individuals who are part of their social circle. This happens due to the influencing skills in society being conditioned by a series of factors, also encompassing knowledge. This skill may even result in a leadership position in terms of opinion, which, in turn, feeds into expectations related to product performance. Such expectations have a subsequent effect on decision-making over adopting a product.
In a Metaverse context, for both managers and product creators, results point out the importance of seizing previous experience to improve product usability. This occurs due to the fact that experience influences the learning curve for a piece of technology, making it easier the processes of assimilating new concepts and skills related to it.
Moreover, marketing and advertisement managers might employ prior knowledge to create advertisement campaigns in order to show possible uses and applications for Metaverse technologies, seeking to bridge the gap between user experience and users’ needs, such as in the case of teaching and learning, presented in this research scenario.
7 Conclusion, limitations, and recommendations
The main contribution of this paper is the analysis of the preceding effects of previous experience in technology adoption, in a scenario of Metaverse usage for educational means, through the utilization of the UTAUT model. According to this perspective, such a construct showed a complementary partial mediating effect regarding a need for social influence and performance expectation on the Metaverse as an educational tool.
Moreover, although statistically valid in the structural equation modeling, most path analyses did not show previous experience influence when considering easing conditions and anxiety. This might be due to a lack of concrete propositions for technology integration within the teachers’ education environment, which reduces perceptions of technical needs, infrastructure, and professional pressure. Still, influence over effort expectation, easing conditions, and anxiety was evident, as well as an impact on anxiety. This development highlights the importance of taking into account such factors in the planning of training programs for the use of educational technologies in smart learning environments, such as the Metaverse, especially in the school of technological education in the state of Pará, to reduce psychological barriers and improve the quality of teaching.
To do so, a model was developed having previous experience as a behavioral antecedent of latent relationships as proposed in previous research studies. This model was tested through the use of a quantitative method, based on PLS-SEM, with a non-probabilistic sample of 311 teachers working in the Pará state technological- and career-oriented public education network.
Limitations for this study include sample characteristics (non-probabilistic), gathered from a very specific niche (technological- and career-oriented public education teachers), from a single Brazilian state (Pará), where there are not yet education initiatives—be it implemented or being planned—of Metaverse usage for educational means.
Another limitation lies in the Metaverse scenario presentation, due to a scarcity of “real” application of such technology in the field of education, which makes it difficult to understand more effectively previous experience effects related to effort expectation within a psychological sequence as it pertains to this education technology potential users.
Experimental research must be done considering the psychological effects of Metaverse usage in education. Not only that but also it is necessary to deepen an understanding of previous experience effects on this parallel world, which is possible through quantitative and qualitative analysis of technology users and potential users.
For future investigations into the engagement of smart learning environments, specifically the Metaverse with education in the Amazon region, it is crucial to explore this relationship in more depth. A promising path for research is to expand the area of investigation to other states belonging to the Brazilian Amazon, this would make it possible to expand the sample group and the variety of characteristics, increasing the accuracy of the results due to the expansion of the mosaic of participants. Furthermore, carrying out a longitudinal study in parallel with teacher training, that is, monitoring data collection, is essential to analyze whether teachers are developing new skills and digital interactions over time.
The primary contribution of this article lies in the analysis of the antecedent effects the prior experience has on technology adoption within an educational context, with a specific focus on Metaverse use, through the UTAUT model. The results demonstrate that prior experience has a partial mediating effect, particularly regarding social influence (H12) and performance expectancy (H11). This suggests that teachers who are more familiar with technology will be more influenced by their peers and will have fewer doubts about the role of the Metaverse in pedagogical practice (H7).
The study examined Metaverse use in education within the Brazilian Amazon context, employing the UTAUT model to understand how various factors influence the adoption of innovative technologies. The UTAUT model consists of four key constructs: performance expectancy, effort expectancy, social influence, and facilitating conditions, each playing a vital role in the acceptance and utilization of technology.
The analysis of results based on the UTAUT (unified theory of acceptance and use of technology) model provided significant insights into the underlying factors influencing the adoption of disruptive technologies, such as the Metaverse. The application of the UTAUT model enabled the identification of key constructs, including performance expectancy, perceived effort, facilitating conditions, and social influence, as critical factors in the technology adoption process. Additionally, it underscored the pivotal role of prior experience in shaping perceptions of both the usefulness and ease of use of the technology.
Technical terms such as factor loadings, discriminant validity, and construct reliability, demonstrated by Cronbach’s alpha values exceeding 0.7, were crucial in ensuring the external validity of the proposed model. Furthermore, the path coefficients, model fit indices (SRMR = 0.056), and R2 values for understanding the overall effect highlighted the robustness of the tested relationships. Thus, the model not only elucidated the direct and indirect pathways to adoption, as demonstrated by the presented mediations, but also reinforced the significance of partial complementary mediators, such as performance expectancy and social influence, which significantly increased the overall structural coefficient in technology adoption.
Moreover, while effort expectancy has shown a positive impact on the adoption of educational technologies (H2), it has also proven to be significant in influencing teachers’ anxiety (H3). Anxiety, in turn, has a negative effect on Metaverse adoption (H5); although statistically valid in the structural equation model, most path analyses did not show an influence of prior experience when considering facilitating conditions and anxiety. This issue can be attributed to the lack of concrete proposals for integrating technology into teacher training environments, which diminishes perceptions of technical needs, infrastructure, and professional pressure.
However, the influence on effort expectancy, facilitating conditions, and anxiety, as well as the impact of anxiety, became evident. This development underscores the importance of considering these factors in the design of training programs for educational technologies in smart learning environments, such as the Metaverse. This is particularly crucial for technology-focused educational institutions in Pará State, with the aim of reducing psychological barriers and enhancing the quality of education.
Facilitating conditions, such as resources and institutional support, have proven essential for the adoption of technological innovations (H6) and have positively impacted the perception of facilitating conditions (H4). However, teachers’ prior experience did not show a significant influence on these conditions (H13).
Social influence, including the pressure and opinions of colleagues and other close individuals, has demonstrated a positive impact on both performance expectancy (H7) and effort expectancy (H8), thereby reinforcing the role of social interaction in the technological adoption process. However, influence can also increase anxiety (H9) and affect perceptions of facilitating conditions (H10), particularly in environments where technology has not yet been widely adopted.
To achieve this goal, a model was developed with prior experience as a behavioral antecedent of latent relationships, as proposed in previous studies. This model was tested using a quantitative method based on PLS-SEM, with a non-probability sample of 311 teachers working in the public technological and vocational education network in the state of Pará.
7.1 Study limitations
The limitations of this study include the characteristics of the sample (non-probabilistic), which was collected from a very specific niche (teachers in technological and vocational education) within a single Brazilian state (Pará), where there are currently no educational initiatives—either implemented or planned—utilizing Metaverse for educational purposes. These characteristics constrain the generalizability of the findings of the study to other regional contexts or types of educational institutions, potentially limiting the broader applicability of the results.
Another limitation is related to the portrayal of the Metaverse scenario. Due to the scarcity of “real-life” applications of this technology in the field of education, it complicates a more effective understanding of the effects of prior experience on effort expectancy within a psychological sequence for potential users of this educational technology.
7.2 Practical relevance
This study emphasizes the importance of factors such as social influence and performance expectations in the adoption of educational technologies, providing guidelines for the development of teacher training programs. Social influence, for instance, highlights the role of peer interactions in the technology acceptance process, suggesting that collaborative and supportive environments may accelerate the integration of the Metaverse into educational contexts.
Performance expectations underscore the need to clearly demonstrate how the use of the Metaverse can enhance educational outcomes, which may encourage educators’ adoption. Training programs should therefore focus on presenting concrete applications of the technology that are directly relevant to pedagogical practice. Additionally, it is crucial to recognize and address the limitations of this study, particularly those that may affect the generalizability of the results. Understanding these challenges can guide future research and efforts to make the integration of the Metaverse more widespread and effective in various educational contexts.
7.3 Future research
For future investigations into the engagement with smart learning environments, specifically the Metaverse in education within the Amazonian region, it is crucial to explore this relationship in greater depth. A promising path for the research is to expand the area of investigation to include other states within the Brazilian Amazon, which would allow for a larger sample group and a greater variety of characteristics, thereby increasing the accuracy of the results due to the expanded mosaic of participants. In addition, conducting a longitudinal study alongside teacher training—specifically, monitoring data collection—is essential for analyzing whether teachers are developing new digital skills and interactions over time.
To advance the understanding and application of the Metaverse in education, it is crucial to explore new research directions. Expanding the investigation to other states within the Brazilian Amazon will significantly enhance the sample size and diversity of participant characteristics, thereby improving the accuracy of the results.
Longitudinal studies are essential for assessing how prolonged use of the Metaverse influences skill development and the evolution of perceptions over time. Additionally, experimental research can explore the psychological effects of the Metaverse, such as anxiety and motivation, in controlled environments, offering a more detailed understanding of how these variables impact the adoption and effectiveness of the technology.
Contextual studies that explore how cultural and regional factors influence the integration and impact of the Metaverse are crucial. These studies can tailor technology to the specific needs of different educational communities. Additionally, comparative analysis between teaching environments that utilize the Metaverse and those that do not can provide insights into how technology can be adapted to meet the specific needs of diverse educational settings.
Comparative analysis between teaching environments that utilize Metaverse and those that do not can provide valuable insights into its impact on the quality of teaching and students’ academic outcomes. These approaches offer a solid foundation for optimizing the implementation of Metaverse in education, ensuring its relevance and effectiveness across diverse contexts.
To ensure that research has a tangible impact on educational practices and policy development, particularly in remote and disadvantaged regions such as the Brazilian Amazon, it is essential to provide practical recommendations for educators and policymakers. It is essential for teacher training programs to include specific instruction on the use of the Metaverse, adapted to local realities and the pedagogical needs of communities.
Additionally, educational policies should be formulated to support the necessary infrastructure and provide adequate resources for the effective implementation of technology. Collaborating with education and technology experts to develop practical and adaptable guidelines can facilitate the adoption of the Metaverse, ensuring that its benefits are accessible to all educators, regardless of their location. This approach will allow for a more comprehensive analysis and a closer connection between theory and practical application, promoting real and sustainable improvements in education.
Data availability statement
The raw data supporting the conclusions of this article will be made available by the authors, without undue reservation.
Author contributions
YM: Conceptualization, Data curation, Formal analysis, Funding acquisition, Investigation, Methodology, Project administration, Resources, Software, Supervision, Validation, Visualization, Writing – original draft, Writing – review & editing. PV-N: Conceptualization, Data curation, Formal analysis, Funding acquisition, Investigation, Methodology, Project administration, Resources, Software, Supervision, Validation, Visualization, Writing – original draft, Writing – review & editing. DP: Formal analysis, Funding acquisition, Investigation, Methodology, Supervision, Validation, Visualization, Writing – review & editing.
Funding
The author(s) declare that financial support was received for the research, authorship, and/or publication of this article. This study received partial support from national funds through FCT (Fundação para a Ciência e a Tecnologia), under the project—UIDB/04152/2020—Centro de Investigação em Gestão de Informação (MagIC)/NOVA IMS.
Acknowledgments
We gratefully thank the Centro de Investigação em Gestão de Informação (MagIC)/NOVA IMS and Rúben Rumipamba (CNT) for providing useful comments to improve this study.
Conflict of interest
The authors declare that the research was conducted in the absence of any commercial or financial relationships that could be construed as a potential conflict of interest.
Publisher’s note
All claims expressed in this article are solely those of the authors and do not necessarily represent those of their affiliated organizations, or those of the publisher, the editors and the reviewers. Any product that may be evaluated in this article, or claim that may be made by its manufacturer, is not guaranteed or endorsed by the publisher.
Abbreviations
AR, Augmented reality; AVE, Average variance extracted; CC, Construct reliability; DICT, Digital information and communication technologies; VD, Discriminant validity; SCT, Expectation results; MM, Extrinsic motivation; CF, Factor loading; LVP, Latent variable performance; PLS-SEM, Partial least squares structural equation modeling; IDT, Relative advantage; ICT, Information and communication technologies; SECTET/PA, Secretariat of Science, Technology, and Higher, Professional, and Technological Education of Pará; SEM, Structural equation modeling; TAM, Technology acceptance model; UTAUT, Unified theory of acceptance and use of technology; VIF, Variance inflation factor; VR, Virtual reality; MPCU, Work adequacy.
Footnotes
References
Akour, I. A., Al-Maroof, R. S., Alfaisal, R., and Salloum, S. A. (2022). A conceptual framework for determining metaverse adoption in higher institutions of gulf area: an empirical study using hybrid sem-ann approach. Comput. Educ. Artif. Intell. 3:100052. doi: 10.1016/j.caeai.2022.100052
Antonietti, C., Cattaneo, A., and Amenduni, F. (2022). Can teach- ers’ digital competence influence technology acceptance in vocational education? Comput. Hum. Behav. 132:107266. doi: 10.1016/j.chb.2022.107266
Arruda Filho, E. J. M., Nogueira, A. C. L., and Costa, E. M. S. (2022). Social influence effect on consumers’ intention to adopt mobile banking services. Inf. Syst. Manag. 39, 269–285. doi: 10.1080/10580530.2021.1965678
Bernacki, M. L., Greene, J. A., and Crompton, H. (2020). Mobile technology, learning, and achievement: advances in understanding and measuring the role of mobile technology in education. Contemp. Educ. Psychol. 60:101827. doi: 10.1016/j.cedpsych.2019.101827
Bervell, B., and Arkorful, V. (2020). LMS-enabled blended learning utilization in distance tertiary education: establishing the relationships among facilitating conditions, voluntariness of use and use behaviour. Int. J. Educ. Technol. High. Educ. 17, 1–16. doi: 10.1186/s41239-020-0183-9
Blut, M., Chong, A., Tsiga, Z., and Venkatesh, V. (2021). Meta-analysis of the unified theory of acceptance and use of technology (utaut): challenging its va- lidity and charting a research agenda in the red ocean. J. Assoc. Inf. Syst. 23, 13–95. doi: 10.17705/1jais.00719
Blut, M., and Wang, C. (2020). Technology readiness: a meta- analysis of conceptualizations of the construct and its impact on technology usage. J. Acad. Mark. Sci. 48, 649–669. doi: 10.1007/s11747-019-00680-8
Burton, S., Sheather, S., and Roberts, J. (2003). Reality or perception? The effect of actual and perceived performance on satisfaction and behavioral intention. J. Serv. Res. 5, 292–302. doi: 10.1177/1094670503005004002
Claro, M., Salinas, Á., Cabello-Hutt, T., San Martín, E., Preiss, D. D., Valenzuela, S., et al. (2018). Teaching in a digital environment (tide): defining and measuring teachers’ capacity to develop students’ digital information and communication skills. Comput. Educ. 121, 162–174. doi: 10.1016/j.compedu.2018.03.001
Compeau, D. R., and Higgins, C. A. (1995). Computer self- efficacy: development of a measure and initial test. MIS Q. 19, 189–211. doi: 10.2307/249688
Connolly, S., Miller, C., Lindsay, J., and Bauer, M. (2019). Providers’ attitudes toward telemental health via videoconferencing: findings from a systematic review. In: 2019 annual research meeting. AcademyHealth.
Da Costa-everaldo, E. M. S., Esteves, A. M., and Bezerra, T. C. (2022). A influência social como antecedente da continuidade de uso de plataformas de aulas remotas: Modelo conceitual a partir da visão de professores do ensino superior.
Davis, F. D., Bagozzi, R. P., and Warshaw, P. R. (1992). Extrinsic and intrinsic motivation to use computers in the workplace 1. J. Appl. Soc. Psychol. 22, 1111–1132. doi: 10.1111/j.1559-1816.1992.tb00945.x
Devaraj, S., Easley, R. F., and Crant, J. M. (2008). Research note—how does personality matter? Relating the five-factor model to technology acceptance and use. Inf. Syst. Res. 19, 93–105. doi: 10.1287/isre.1070.0153
Dholakia, R. R., Costa, E. M. S., Gammarano, I. J. L., and Arruda Filho, E. J. M. (2021). Antecedents of brazilian consumers’ intentions towards virtual grocery shopping. J. Cust. Behav. 20, 221–242. doi: 10.1362/147539221X16356770010721
Dos Santos, A. O., Cogo, S. B., Sehnem, G. D., Monteiro, A. S., Dallabrida, G. S., and Scopel, M. F. (2022). Uso de tecnologias educacionais do plano de parto junto às gestantes: revisão integrativa de literatura. Res. Soc. Dev. 11, –e38311125218. doi: 10.33448/rsd-v11i1.25218
Dwivedi, Y. K., Hughes, L., Baabdullah, A. M., Ribeiro-Navarrete, S., Giannakis, M., Al-Debei, M. M., et al. (2022). Metaverse beyond the hype: multidisciplinary perspectives on emerging challenges, opportunities, and agenda for research, practice and policy. Int. J. Inf. Manag. 66:102542. doi: 10.1016/j.ijinfomgt.2022.102542
Dwivedi, Y. K., Rana, N. P., Jeyaraj, A., Clement, M., and Williams, M. D. (2019). Re-examining the unified theory of acceptance and use of technology (utaut): towards a revised theoretical model. Inf. Syst. Front. 21, 719–734. doi: 10.1007/s10796-017-9774-y
Garone, A., Pynoo, B., Tondeur, J., Cocquyt, C., Vanslambrouck, S., Bruggeman, B., et al. (2019). Clustering university teaching staff through utaut: implications for the acceptance of a new learning management system. Br. J. Educ. Technol. 50, 2466–2483. doi: 10.1111/bjet.12867
Hair, J. F., Hult, G. T. M., Ringle, C. M., Sarstedt, M., Danks, N. P., Ray, S., et al. (2021). “An introduction to structural equation modeling” in Partial least squares structural equation modeling (PLS-SEM) using R: A workbook. ed. J. F. Hair (Berlin: Springer), 1–29.
Hair, J. F. Jr., Sarstedt, M., Hopkins, L., and Kuppelwieser, V. G. (2014). Partial least squares structural equation modeling (pls-sem): an emerging tool in business research. Eur. Bus. Rev. 26, 106–121. doi: 10.1108/EBR-10-2013-0128
Hair, J. F., Ringle, C. M., and Sarstedt, M. (2011). Pls-sem: indeed a silver bullet. J. Mark. Theory Pract. 19, 139–152. doi: 10.2753/MTP1069-6679190202
Hair, J. F., Risher, J. J., Sarstedt, M., and Ringle, C. M. (2019). When to use and how to report the results of pls-sem. Eur. Bus. Rev. 31, 2–24. doi: 10.1108/EBR-11-2018-0203
Hair, J. F., Sarstedt, M., Ringle, C. M., and Gudergan, S. P. (2017). Advanced issues in partial least squares structural equation modeling. Thousand Oaks, CA: SAGE Publications.
Hakverdi-Can, M., and Dana, T. M. (2012). Exemplary science teachers’ use of technology. Turk. Online J. Educ. Technol. 11, 94–112.
Henderson, J., and Corry, M. (2021). Teacher anxiety and technology change: a review of the literature. Technol. Pedagog. Educ. 30, 573–587. doi: 10.1080/1475939X.2021.1931426
Henseler, J., Ringle, C. M., and Sarstedt, M. (2015). A new criterion for assessing discriminant validity in variance-based structural equation modeling. J. Acad. Mark. Sci. 43, 115–135. doi: 10.1007/s11747-014-0403-8
Igbaria, M., and Parasuraman, S. (1989). A path analytic study of individual characteristics, computer anxiety and attitudes toward microcomputers. J. Manag. 15, 373–388. doi: 10.1177/014920638901500302
Kim, B., and Park, M. J. (2018). Effect of personal factors to use icts on e-learning adoption: comparison between learner and instructor in developing countries. Inf. Technol. Dev. 24, 706–732. doi: 10.1080/02681102.2017.1312244
Kinshuk, C., Chen, N.-S., Cheng, I.-L., and Chew, S. W. (2016). Evolution is not enough: revolutionizing current learning environments to smart learning environments. Int. J. Artif. Intell. Educ. 26, 561–581. doi: 10.1007/s40593-016-0108-x
Kreijns, K., Vermeulen, M., Kirschner, P. A., Buuren, H., and Acker, F. V. (2013). Adopting the integrative model of behaviour prediction to explain teach- ers’ willingness to use ict: a perspective for research on teachers’ ict usage in pedagogical practices. Technol. Pedagog. Educ. 22, 55–71. doi: 10.1080/1475939X.2012.754371
Kwon, K.-J., Mai, L.-W., and Peng, N. (2020). Determinants of con- sumers’ intentions to share knowledge and intentions to purchase on s-commerce sites: incorporating attitudes toward persuasion attempts into a social exchange model. Eurasian Bus. Rev. 10, 157–183. doi: 10.1007/s40821-019-00146-5
Langer, M., König, C. J., and Fitili, A. (2018). Information as a double edged sword: the role of computer experience and information on applicant reactions towards novel technologies for personnel selection. Comput. Hum. Behav. 81, 19–30. doi: 10.1016/j.chb.2017.11.036
Lu, J., Schmidt, M., Lee, M., and Huang, R. (2022). Usability research in educational technology: a state-of-the-art systematic review. Educ. Technol. Res. Dev. 70, 1951–1992. doi: 10.1007/s11423-022-10152-6
Luan, H., Geczy, P., Lai, H., Gobert, J., Yang, S. J., Ogata, H., et al. (2020). Challenges and future directions of big data and artificial intelligence in education. Front. Psychol. 11:580820. doi: 10.3389/fpsyg.2020.580820
Massaro, G., Mantovani, A. M., and da Silva Rodrigues, M. (2011). Aplicações educacionais em 3D para os processos de ensino e aprendizagem da área de anatomia no second life. RENOTE 9:25127. doi: 10.22456/1679-1916.25127
Mohamed, N., Sharif, D., and Muhayiddin, M. (2021). Literature review on technology acceptance model: the enhanced variables of venkatesh’s utaut model on students’ acceptance of use on online distance learning. In: AIP conference proceedings, No. 2347. AIP Publishing.
Moore, G. C., and Benbasat, I. (1991). Development of an instrument to measure the perceptions of adopting an information technology innovation. Inf. Syst. Res. 2, 192–222. doi: 10.1287/isre.2.3.192
Mullins, J. K., and Cronan, T. P. (2021). Enterprise systems knowledge, beliefs, and attitude: a model of informed technology acceptance. Int. J. Inf. Manage. 59:102348. doi: 10.1016/j.ijinfomgt.2021.102348
Parasuraman, A., and Colby, C. L. (2015). An updated and streamlined technology readiness index: tri 2.0. J. Serv. Res. 18, 59–74. doi: 10.1177/1094670514539730
Peechapol, C., Na-Songkhla, J., Sujiva, S., and Luangsodsai, A. (2018). An exploration of factors influencing self-efficacy in online learning: a sys- tematic review. Int. J. Emerg. Technol. Learn. 13:64. doi: 10.3991/ijet.v13i09.8351
Potosky, D., and Bobko, P. (1998). The computer understanding and experience scale: a self-report measure of computer experience. Comput. Hum. Behav. 14, 337–348. doi: 10.1016/S0747-5632(98)00011-9
Rogers, E. M., Singhal, A., and Quinlan, M. M. (2014). “Diffusion of innovations” in An integrated approach to communication theory and research (Abingdon: Routledge), 432–448.
Saritas, M. T., and Topraklikoglu, K. (2022). Systematic literature review on the use of metaverse in education. Int. J. Technol. Educ. 5, 586–607. doi: 10.46328/ijte.319
Schophuizen, M., Kreijns, K., Stoyanov, S., Rosas, S., and Kalz, M. (2021). Does project focus influence challenges and opportunities of open online education? A sub-group analysis of group-concept mapping data. J. Comput. High. Educ., 33, 255–280. doi: 10.1007/s12528-020-09264-w
Smart, J., Cascio, J., Paffendorf, J., Bridges, C., Hummel, J., Hurst-House, J., et al. (2007). A cross-industry public foresight project. Proceeding Metaverse Roadmap Pathways, 3DWeb, pp. 1–28.
Tamilmani, K., Rana, N. P., and Dwivedi, Y. K. (2021). Consumer acceptance and use of information technology: a meta-analytic evaluation of utaut2. Inf. Syst. Front. 23, 987–1005. doi: 10.1007/s10796-020-10007-6
Thompson, R. L., Higgins, C. A., and Howell, J. M. (1991). Personal computing: toward a conceptual model of utilization. MIS Q., 125–143. doi: 10.2307/249443
Tlili, A., Huang, R., Shehata, B., Liu, D., Zhao, J., Metwally, A. H. S., et al. (2022). Is metaverse in education a blessing or a curse: a combined content and bibliometric analysis. Smart Learn. Environ. 9, 1–31. doi: 10.1186/s40561-022-00205-x
Venkatesh, V. (2022). Adoption and use of ai tools: a research agenda grounded in utaut. Ann. Oper. Res. 1, 1–12. doi: 10.1007/s10479-020-03918-9
Venkatesh, V., and Davis, F. D. (1996). A model of the antecedents of perceived ease of use: development and test. Decis. Sci. 27, 451–481. doi: 10.1111/j.1540-5915.1996.tb01822.x
Venkatesh, V., Morris, M. G., Davis, G. B., and Davis, F. D. (2003). User acceptance of information technology: toward a unified view. MIS Q. 1, 425–478. doi: 10.2307/30036540
Wang, Y., Su, Z., Zhang, N., Xing, R., Liu, D., Luan, T. H., et al. (2022). A survey on metaverse: Fundamentals, security, and privacy. IEEE Communications Surveys and Tutorials.
Warshaw, P. R., and Davis, F. D. (1985). Disentangling behavioral intention and behavioral expectation. J. Exp. Soc. Psychol. 21, 213–228. doi: 10.1016/0022-1031(85)90017-4
Williams, M., and Daley, S. (2021). Innovation in dementia education within undergraduate healthcare programmes: a scoping review. Nurse Educ. Today 98:104742. doi: 10.1016/j.nedt.2020.104742
Xi, N., Chen, J., Gama, F., Riar, M., and Hamari, J. (2023). The challenges of entering the metaverse: an experiment on the effect of extended reality on workload. Inf. Syst. Front. 25, 659–680. doi: 10.1007/s10796-022-10244-x
Keywords: Metaverse, social influence, technology adoption, technological engagement, technology in education
Citation: Mendonça YVS, Vinueza-Naranjo PG and Pinto DC (2024) Employing structural equation modeling to discern teachers’ perspectives and their embrace of the Metaverse within the classroom setting. Front. Educ. 9:1461646. doi: 10.3389/feduc.2024.1461646
Edited by:
Mahir Pradana, Telkom University, IndonesiaReviewed by:
Mahendra Fakhri, Telkom University, IndonesiaTendai Charles, British University in Dubai, United Arab Emirates
Copyright © 2024 Mendonça, Vinueza-Naranjo and Pinto. This is an open-access article distributed under the terms of the Creative Commons Attribution License (CC BY). The use, distribution or reproduction in other forums is permitted, provided the original author(s) and the copyright owner(s) are credited and that the original publication in this journal is cited, in accordance with accepted academic practice. No use, distribution or reproduction is permitted which does not comply with these terms.
*Correspondence: Paola G. Vinueza-Naranjo, cGFvbGFnLnZpbnVlemFAdW5hY2guZWR1LmVj